Flash and JavaScript are required for this feature.
Download the video from iTunes U or the Internet Archive.
Topics covered: Conversion of continuous-time to discrete-time signals through sampling; Digital differentiaion, half-sample delay; Demonstration: sampling a continuous-time signal, filtering sequences with a low-pass filter, reconstructing a continuous-time signal using bandlimited interpolation.
Instructor: Prof. Alan V. Oppenheim
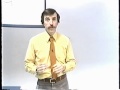
Lecture 18: Discrete-Time P...
[MUSIC PLAYING]
PROFESSOR: Last time, we began the discussion of discreet-time processing of continuous-time signals. And, as a reminder, let me review the basic notion. The idea was that we convert from a continuous-time signal to a sequence through an operation which I represent as a continuous to discrete time converter. And then that sequence is used as the input to an appropriate discreet-time system. And after appropriate discreet-time processing, that sequence is converted back to a continuous-time signal through an operation which I label as a discrete to continuous time converter.
Now, in the lecture last time, we carried out some analysis which related for us the spectra in the first step of this operation. Namely in the transformation from a continuous-time time signal to a sequence. And let me, by the way, draw your attention to the fact that, in the real world, this operation is essentially implemented by what you would typically label as an analog to digital converter, if in fact the discreet-time processing is being done digitally. Now, it's important to emphasize that it's not exactly what an analog to digital converter does, but, in some sense at least, you should think of this mapping from continuous-time to discreet-time in very much the same way that one would think of an analog to digital converter. And the mapping back then corresponds, in some sense, to what would happen with a digital to analog converter.
Well, let me review what is involved in the mapping from the continuous-time signal to the sequence. And, let me stress again that, this operation is basically-- in the continuous to discreet-time conversion-- a two-step process. In the first part of the process, the continuous-time signal is modulated with impulse train, where the period of the impulse train is capital T. And so we have a continuous-time time impulse train signal which captures the samples of the original continuous-time signal.
That impulse train is then put through an operation which, essentially, re-labels the samples so that the sample values, the impulse areas, are re-labeled as sequence values. And the result of that conversion is then the sequence x of n. So the overall process, then, is a sampling process, followed by what is simply, in this box, a re-labeling process. And, although as I indicated just a minute ago, that is essentially what an analog to digital converter does. An analog to digital converter doesn't necessarily carry it out in those two steps, but particularly, in terms of carrying through an analysis, thinking of it as a two-step process is particularly convenient.
Now we talked last time about what this mapping from continuous-time to discreet-time means, both in the time domain, and terms of the spectra. And in particular, in the time domain we begin with the continuous-time signal, which is then sampled with an impulse train and converted to a sequence by simply generating a sequence whose values are the areas of the impulses. And I stress the fact that what this corresponds to, essentially, is a normalization of the time axis, essentially, by dividing the time axis by capital T.
In the frequency domain, then, we had the spectrum of the original signal, which, because of the sampling process, is replicated at integer multiples of the sampling frequency omega sub s, or 2 pi over capital T. And then, in converting the impulses to a sequence, we are essentially normalizing the frequency axis, so that the frequency 2 pi over capital T gets re-labeled as 2 pi. And the resulting discreet-time spectrum looks like I indicate here. Which really is nothing more than a frequency scaling corresponding to the associated time scaling. So the mapping from the impulse train spectrum to the discreet-time spectrum corresponds to a mapping specified by capital omega equal to small omega times capital T.
And equivalently, it's the frequency 2 pi over capital T, which is, of course, the sampling frequency which gets normalized to the frequency 2 pi. And so, in the frequency domain, there is a frequency normalization associated with the fact that corresponding to this spectrum is a time sequence or discreet-time sequence, as I showed previously, and the discreet-time sequence is related to the original continuous-time signal through a time normalization. In other words, these sequence values are simply samples of the continuous-time signal with the time axis renormalized.
Now, what we want to consider-- this is the conversion from continuous-time to discreet-time-- what we want to consider now is the overall system which implements not just the conversion, but filtering, and then coming back out of the conversion back to continuous-time. So let's look at the overall system. And the overall system, of course, as I've stressed several times in the past, consists of first, the sampling process, conversion to an impulse train, and the impulse train converted to a sequence.
That sequence is then processed through our discreet-time filter. And after the discreet-time time processing, the result of that is converted back to an impulse train. So this resulting process sequence is then converted back to an impulse train. And then, finally, we carry out the desampling process by simply using a low-pass filter with a cutoff associated with the sampling frequency that we used.
Now, typically in a system like that-- which implements discreet-time processing of continuous-time time signals-- we need to ensure in one way or another that the bandwidth of the input is sufficiently limited, so that we avoid aliasing. One way to do that is to force it one way or another, or simply know that our signal satisfies the bandwidth constraint. Although, a fairly typical thing to do in addition to this sampling process is to include what is referred to an anti-aliasing filter. In other words, this is a filter that would band-limit the input at at least half the sampling frequency, so that we are guaranteed, then, that there is no aliasing that's carried out in this process. And it's important to stress that, in this kind of processing-- discreet-time processing of continuous-time signals-- except in certain special situations, it's very important to avoid aliasing because we're going to want to do a reconstruction after we do the sampling and processing.
OK, now, this is the sequence of steps in the time domain. Let's examine what happens as a consequence of this in the frequency domain. Well, let's choose some type of simple representative spectrum. And, of course, what's important about it is that the spectrum we choose is band-limited, or that there's an anti-aliasing filter. And it's not the shape, of course, that is critical.
And as we work our way through the system, this is the continuous-time spectrum. After sampling, that spectrum is replicated at multiples of the sampling frequency-- integer multiples of the sampling frequency-- and so there would be another one over here, and another one over here, et cetera. And then, in converting to a discreet-time sequence, there is the associated frequency normalization, so that the sampling frequency gets normalized to a frequency of 2 pi. OK, now, at that point, where we are in the system is at this point, so that we've converted to a sequence. We now want to carry out some filtering, and then, after that filtering, convert back to a continuous-time signal.
All right, so, here we are at the spectrum associated with the sequence. And now, the processing that we're carrying out is linear time and variant filtering in the discreet-time domain. And what that corresponds to, then, is multiplying this spectrum by the filter frequency response. And I've chosen a particular shape. And again, it's not the shape that's important to the discussion, but the fact, for example, that it has a particular cutoff frequency, which we will track as we work through this.
And so now, the spectrum of y of n, the output of the digital filter, is the product of this spectrum, and the Fourier transform, or frequency response, of the digital filter.
Now, in working our way through, we're going to take the output of the filter and undo the two-step process. So we now want to take that sequence, convert it to an impulse train, and then take that impulse train and desample through a low-pass filter. So, here we are now at the output of the digital filter. We then convert that to an impulse train. Well that's really undoing the original time normalization.
And so, what that means, is that we are undoing the frequency normalization. In particular, we're dividing the frequency axis by capital T. Whereas, this point in y of omega was 2 pi, now it's 2 pi over capital T. What that means is that, equivalently, we're multiplying this spectrum by the frequency response of the digital filter, but now linearly-scaled in frequency, so that what was a cutoff frequency of omega sub c is now cutoff frequency of omega sub c, divided by capital T.
So now, the next step in the process is the reconstructing low-pass filter. And what that extracts is simply the portion of this periodic spectrum around the origin. And so finally, then, the spectrum of the output of the overall system will be the spectrum of the input multiplied by a frequency response, which is the digital filter frequency response frequency scaled by dividing that digital filter frequency axis by capital T.
OK, now, what we can ask is, we've got this processing-- we've converted to discreet-time, and we've gone back to continuous-time, and one can ask now what equivalent, overall continuous-time system does that correspond to? In other words, if we-- that, of course, is a continuous-time system, it's a continuous-time input and continuous-time time output-- and the overall system, then, would be one that would give us exactly the same output spectrum as we're getting. Well, what is that?
What we have is an output spectrum, which is the product of the input spectrum and the digital filter frequency characteristic frequency-scaled. And so, in fact, the resulting continuous-time time filter is simply the digital filter with an appropriate frequency scaling. In other words, with the frequency axis divided by capital T. So said another way, if we show here the frequency response of the original digital filter, then the corresponding continuous-time filter would be this, frequency-scaled.
And then, because of the associated low-pass filtering and the reconstruction, we would select out just one of these periods-- in particular, the portion around the origin. And the essential consequence of that is that the corresponding continuous-time filter, then, is given by this. And these two are related simply by a linear scaling of the frequency axis. And note that, where the digital filter has a cutoff frequency of omega sub c, the continuous-time filter has a cutoff frequency of omega sub c divided by capital T.
So that's the linear frequency scaling. And, by the way, plant away for now-- and we'll return to this point later-- the observation that even if the digital filter frequency response is fixed, which we would assume it is, by changing the sampling frequency, in fact, what we're able to do is affect a linear scaling all of the equivalent continuous-time filter.
OK, well, this is pretty much the process and the analysis, but to highlight a number of the issues and emphasize these points, what I'd like to do is illustrate some of this with a videotape demonstration that, in fact, was made originally as part of another course-- a course devoted entirely to digital signal processing, which essentially is discreet-time time processing, whether or not it's related to continuous-time signals. And what I'd like to now focus on are some of the details of that demonstration.
In the demonstration, the specific impulse response that is used for the digital filter, or discreet-time filter, is the one that I show here. And the associated frequency response is the frequency response of a discreet-time, low-pass filter, as I indicate below. And the cutoff frequency of that filter-- as I indicate, the filter was designed as a discreet-time filter with a cutoff frequency of pi over 5.
And let me just draw your attention to the fact that pi over 5 is also a 10th of 2 pi. And so in fact the digital or discreet-time filter cutoff frequency is a 10th of 2 pi. And as I'll stress again shortly, remember that, in the frequency normalization or unnormalization, 2 pi represents, in effect, the sampling frequency. And so the consequence of that is that the cutoff frequency really, is going to be associated with a 10th of the sampling frequency. But, for now, keep in mind that it's just simply a 10th of 2 pi.
Now, the equivalent continuous-time system, in terms of the impulse response, is, of course, a band-limited interpolation of the impulse response associated with the discreet-time filter. And in the frequency domain, the frequency response is correspondingly a time-scaled, frequency scaled version of the frequency response. So, in fact, in the frequency domain and in the time domain related to the continuous-time signal, the associated impulse response is what I indicate here-- a band-limited interpolation of the discreet-time impulse response and time scale, in fact. And the frequency response-- following the discussion that we've previously gone through-- is a frequency-scaled version of the one associated with the digital filter.
Well, the first thing that I'll want to look at is the impulse response. And when we do, let me just indicate that in the actual implementation things are slightly different than they are associated with the ideal analysis. In particular, in converting from a discreet-time sequence to the continuous-time signal, whereas this way of looking at it is convenient in the context of the analysis, in fact, the way it's done is using a more or less standard digital to analog converter.
And what a digital to analog converter does, as I indicated in the previous lecture, is to convert the sequence not to an impulse train but, in fact, to go directly through a zero-order hold. And so, usually what comes out of a digital to analog converter is a staircase type of signal associated with a zero-order hold. And then, the result of that is low-pass filtered to do the reconstruction.
So what we'll want to look at, then, is that reconstruction, first with just an impulse input. And so, what we'll see after the low-pass filter, for the impulse response, is a smooth curve like this. But also, as part of the demonstration, what I'll do, just to show the zero-order hold, is to take the low-pass filter out temporarily and then put it back in. So first, let's just look at the filter impulse response.
What we see here is the impulse response of the overall system. And we observe, for one thing, that it's a symmetrical impulse response. In other words, corresponds to a linear phase filter. We could also look at the impulse response before the desampling low-pass filter-- let's take out the desampling low-pass filter slowly-- and what we observe is, basically, the output of the digital to analog converter.
Which, of course, is a staircase, or boxcar, function, not an impulse train. In the real world, the output of a D to A converter, generally, is a boxcar type of function. We can put the desampling filter back in now and notice that the effect of the desampling filter is, basically, to smooth out the rough edges in the boxcar output from the D to A converter.
OK, so, that's the impulse response of the system. Now, what I'd like to show is the frequency response of the system. And to measure the frequency response, of course, what we can do is put a sine wave into the system and look at the sinusoidal output.
So, in particular now, what will happen is that, with the system, we will put in a continuous-time sinusoid, which is sampled, converted to a sequence. The sampled continuous-time sinusoid is a discreet-time sinusoid. That goes through the digital filter and gets attenuated, or amplified appropriately. And then the output of that is converted back-- and that's, again, a sinusoidal output-- that gets converted back to a continuous-time sinusoid. Theoretically, as I indicate here-- but again, as we just saw, really, represented by a zero-order hold, followed by a low-pass filter. So, that's the overall operation, with one modification from the diagram that we have here.
In this particular diagram I've included and anti-aliasing filter. In fact, in the demonstration there is no anti-aliasing filter. And so, in fact, the input is a sinusoidal input which is not band-limited by virtue of an anti-aliasing filter. It's only, of course, band-limited appropriately if we choose the sinusoidal frequency that way.
So, there is no anti-aliasing filter, and this is the system. And one consequence of that is that, in fact, if we sweep the input sinusoid only up to half the sampling frequency, we'll see no aliasing. But if we let it sweep past that, we're going to get aliasing.
Now, in the demonstration, the sampling rate that's picked for this part of the demonstration is a 20 kilohertz sampling rate. That means, based on the sampling theorem, that as long as the input frequency is below 10 kilohertz we get no aliasing. When the input frequency goes beyond 10 kilohertz , that higher frequency is going to get aliased down into a lower frequency.
A consequence of that, then, is that as we go through the processing, and we demonstrate the frequency response of the system, what we'll see in the output is no aliasing when the input is below 10 kilohertz. As the input sweeps past 10 kilohertz-- when we let it, which we will eventually in the demonstration-- then, in fact, that frequency, as it finally shows up here, will begin to be aliased down into a lower frequency. Another way of thinking about that is that, when we watch the frequency response of the system, as we look at the digital filter frequency response, what we're sweeping as we go from 0 up to 10 kilohertz in the input frequency is this portion of the frequency response.
As we sweep from 10 kilohertz out to 20 kilohertz, what we'll see is this portion of the frequency response. In other words, we'll see it periodically replicated. Or, if we look at the corresponding continuous-time frequency response, what it means, really, is that sweeping from 0 to 10 kilohertz is moving up this way. And then sweeping from 10 kilohertz to 20 kilohertz on the input, really because of the aliasing, reflects itself in the digital filter by looking back toward lower frequencies. And so the continuous-time filter sweeps back down from 10 kilohertz back to 0.
OK, so, that's what we'll see, and we'll see it in several different ways as explained in the demonstration. So now let's look at the frequency response of the filter.
Now what we'd like to illustrate is the frequency response of the equivalent continuous-time filter. And we can do that by sweeping the filter with sinusoidal input. So, what we'll see in this demonstration is, on the upper trace, the input sinusoid, on the lower trace, the output sinusoid, using a 20 kilohertz sampling rate, and a sweep from 0 to 10 kilohertz. In other words, a sweep from 0 to, effectively, pi, in terms of the digital filter.
So what we'll observe as the input frequency increases, is that the output sinusoid will have, essentially, constant amplitude up to the cutoff frequency of the filter, and then approximately zero amplitude past. So let's now sweep the filter frequency response. And there is the filter cutoff frequency.
Now, we can also observe the filter frequency response in several other ways. One way in which we can observe it is by looking, also, at the amplitude of the output sinusoid as a function of frequency, rather than as a function of time. And so we'll observe that on the left-hand scope.
While on the right-hand scope, we'll have the same trace the we just saw, namely two traces-- the upper trace is the inputs sinusoid, the lower trace is the output sinusoid. And, in addition to observing the frequency response, let's also listen to the output sinusoid and observe the attenuation in the output as we go from the filter passband to the filter stopband. Again, a 20 kilohertz sampling rate and a sweep range from 0 to 10 kilohertz.
Now, of course, we're in the filter stopband. Now, if we increase the sweep range from 10 kilohertz the 20 kilohertz, so that the sweep range is equal to the sampling frequency, in essence, that corresponds to sweeping out the digital filter from 0 to 2 pi. And, in that case, we'll begin to see some of the periodicity in the digital filter frequency response. So let's do that now with a 20 kilohertz sampling rate and a sweep range of 0 to 20 kilohertz.
Now as we come near 2 pi, we get back the past-band. And, finally, back to a 0 to 10 kilohertz sweep, so that we're again sweeping only from 0 to pi with regard to the digital filter.
Now, an important observation is that, with the digital or discreet-time filter cutoff frequency fixed as I've indicated here-- and I remind you that what the cutoff frequency is, is a 10th of 2 pi-- with that cutoff frequency fixed, because of the normalization that we get as we come back to a continuous-time filter, in fact, what we have is a cutoff frequency that is dependent on the sampling frequency or on the sampling period. And, more specifically, since the discreet-time, or digital, filter or has a cutoff frequency which is a 10th of 2 pi, the normalization, as you recall, is that 2 pi, in discreet-time frequency, corresponds to omega sub s, the sampling frequency, in terms of continuous-time frequency. The consequence is that this cutoff frequency, in fact, is 1/10 of-- not 2 pi now because of the normalization-- it's 1/10 of the sampling frequency.
So, consequently, as we change the sampling frequency, what will happen is that, even with the discreet-time filter cutoff fixed, the cutoff frequency of the equivalent continuous-time filter will change. Now, that's what I want to demonstrate. But let me again stress and ask you to keep in mind that this demonstration is done without an anti-aliasing filter in.
And we are going to be changing the sampling frequency and, so keep in mind that, as we look at this, as the input frequency sweeps past half the sampling frequency-- whatever sampling frequency we happen to be looking at-- then, because of the fact that there's no anti-aliasing filter we'll get aliasing. In other words, the frequency and the digital filter, or discreet-time filter, as we sweep the input frequency up, will move up in frequency until we get past half the sampling frequency and then essentially will move back down in frequency. Consequently, what we'll get, then-- or what we'll see-- are periodic replications of the frequency response when we swept past half the sampling frequency.
All right, so now, let's look at the same digital filter, but the frequency response, as we change the sampling frequency. Now, what we would like to demonstrate is the effect of changing the sampling frequency. And we know that the effective filter cutoff frequency is tied to the sampling frequency and, for this particular filter, corresponds to a 10th of the sampling frequency. Consequently, if we double the sampling frequency, we should double the effective filter passband width, or double the filter cutoff frequency.
And, so, let's do that now. Again a 0 to 10 kilohertz sweep range, but a 40 kilohertz sampling frequency. And we should observe that the filter cutoff frequency has now doubled out to four kilohertz. Now, let's begin to decrease the filter sampling frequency. So from 40, let's change the sampling frequency to 20 kilohertz. We should see the cutoff frequency cut in half.
Now, we can go even further. We can cut the sampling frequency down to 10 kilohertz. And remember that the sweep range is 0 to 10 kilohertz. So now we'll be sweeping from 0 to 2 pi. So as we get close to 2 pi, we'll see the passband again. And, now, let's cut down the sampling frequency even further, to 5 kilohertz. Here we are at 2 pi. And then at 4pi.
All right, so, that illustrates the effect of changing the sampling frequency. Now let's conclude this demonstration of the effect of the sampling frequency on the filter cutoff frequency by carrying out some filtering on some live audio. What we'll watch, in this case, is the output audio waveform as a function of time on the single tray scope, and also we'll listen to the output. We'll begin it with a 40 kilohertz sampling rate, then reduce that to 20 kilohertz, 10 kilohertz, and then 5 kilohertz. And in each of those cases, the effective filter cutoff frequency, then, is cut in half from 4 kilohertz, to 2 kilohertz, to 1 kilohertz, and then to 500 cycles.
So let's begin with a 40 kilohertz sampling frequency, or an effective filter cutoff frequency of 4 kilohertz. Now, let's reduce that a 20 kilohertz sampling frequency, or a 2 kilohertz filter. Then a 10 kilohertz sampling frequency. And, finally, a 5 kilohertz sampling frequency corresponding to a 500 cycle equivalent analog filter. Alright, now, let's finally conclude by returning to a little higher quality ragtime by changing the sampling frequency back to 40 kilohertz.
Alright, well, hopefully what you've seen in the demonstration and in this lecture gives you a sense and a feeling for the analysis and the use of discreet-time filters for processing continuous-time signals. And as you may be aware, and as I've tried to indicate previously in the past, this, in fact, is one very important-- but not the only-- but one very important context in which discreet-time filtering is used. And this, in fact, is an area that is developing rapidly because of the fact that microprocessors, digital technology, computers, et cetera afford considerable flexibility in carrying out digital processing of signals. And when digital processing is used, that naturally corresponds to implementing the processing and analyzing it in discreet-time.
Now, in the next lecture we'll be continuing on another aspect-- developing another aspect-- of sampling. And, in particular, what we'll be talking about is sampling of discreet-time signals. As I'll indicate there, one of the contexts in which discreet-time sampling, in fact, plays an important role is in the context in which we are processing continuous-time signals using discreet-time processing. Where, in fact, one step that we might want to take-- in addition to the steps so we've talked about here-- is an additional sampling process following whatever kinds of filtering that we do.
Well, that's a discussion and a topic that we'll be going into in the next lecture. Thank you.
Free Downloads
Video
- iTunes U (MP4 - 87MB)
- Internet Archive (MP4 - 87MB)
Subtitle
- English - US (SRT)