Flash and JavaScript are required for this feature.
Download the video from iTunes U or the Internet Archive.
Topics covered: Linearity, symmetry, time shifting, differentiation and integration, time and frequency scaling, duality, Parseval's relation; Convolution and modulation properties and the basis they provide for filtering, modulation, and sampling; Polar representation, magnitude and phase, Bode plots; Use of transform methods to analyze LTI systems characterized by differential and difference equations; Cascade and parallel form realization: first- and second-order systems.
Instructor: Prof. Alan V. Oppenheim
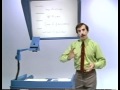
Lecture 9: Fourier Transfor...
Related Resources
Fourier Transform Properties (PDF)
The following content is provided under a Creative Commons license. Your support will help MIT OpenCourseWare continue to offer high quality educational resources for free. To make a donation, or view additional materials from hundreds of MIT courses, visit MIT OpenCourseWare at ocw.mit.edu.
PROFESSOR: In the last two lectures, we saw how periodic and non periodic signals could be represented as linear combinations of complex exponentials. And this led to the Fourier series representation, in the periodic case, and it led to the Fourier transform representation in the aperiodic case. And then, in fact, what we did was to incorporate the Fourier series within the framework of the Fourier transform.
What I'd like to do in today's lecture is look at the Fourier transform more closely, in particular with regard to some of its properties.
So let me begin by reminding you of the analysis and synthesis equations for the Fourier transform, as I've summarized them here.
The synthesis equation being an equation the tells us how to build the time function out of, in essence, a linear combination of complex exponentials. And the analysis equation telling us how to get the amplitudes of those complex exponentials from the associated time function.
So essentially, in the decomposition of x of t as a linear combination of complex exponentials, the complex amplitudes of those are, in effect, the Fourier transform scaled by the differential and scaled by 1 over 2 pi.
As I indicated last time, the Fourier transform is a complex function of frequency. And in particular, the complex function of frequency has an important and very useful symmetry property.
The symmetry of the Fourier transform, when x of t is real, is what is referred to as conjugate symmetric. In other words, if we take the complex function, f of omega, and its complex conjugate, that's equivalent to replacing omega by minus omega.
And a consequence of that, if we think in terms of the real part of the Fourier transform, the real part is an even function of frequency, and the magnitude is an even function of frequency. Whereas the imaginary part is an odd function of frequency, and the phase angle is an odd function of frequency.
So we have this symmetry relationship that, for x of t real, if we think of either the real part or the magnitude of the Fourier transform, it's even symmetric. And the imaginary part, or the phase angle, either one, is odd symmetric. In other words, if we flip it, we multiply by a minus sign.
Let's look at an example, in the context of an example that we worked last time for the Fourier transform.
We took the case of a real exponential of the form e to the minus at times the step. And the Fourier transform, as we found, was of the algebraic form 1 over a plus j omega. And, incidentally, the Fourier transform integral only converged for a greater than 0. In other words, for this exponential decaying.
And I illustrated the magnitude and angle, and what we see is that e to the minus at is a real time function, therefore its magnitude should be an even function of frequency, and indeed it is. And its phase angle, shown below, is an odd function of frequency.
So in fact, although I stressed last time that the complex exponentials is required to build a time function require exponentials of both positive and negative frequencies, for x of t real what we see is that, because of these symmetry properties, either for the real and imaginary or magnitude and angle, we can specify the Fourier transform for, let's say only positive frequencies, and the symmetry, then, implies, or tells us, what the Fourier transform, then, would be for the negative frequencies.
This same example, the decaying exponential, demonstrates another important and often useful property of the Fourier transform. Specifically, let's rewrite, algebraically, this example as I indicate here.
So we have, again, the exponential, whose Fourier transform is 1 over a plus j omega. And if I just simply divide numerator and denominator by a, I can rewrite it in the form shown here. And what we notice is that in the time function we have a term of the form a times t, and in the frequency function we have a term of the form omega divided by a.
Or equivalently, we could think of the time function, which I show here, and as the parameter a gets smaller, the exponential gets spread out in time. Whereas its Fourier transform, or the magnitude of its Fourier transform, has the inverse property that as a gets smaller, in fact, this scales down in frequency.
Well, this is a general property of the Fourier transform, namely the fact that a linear scaling in time generates the inverse linear scaling in frequency. And the general statement of this time frequency scaling is what I show at the top of the transparency, namely the equation that if we scale the time function in time, then we apply an inverse scaling in frequency.
This, in fact, is probably a result that you're already possibly familiar with, in somewhat of a different context. Essentially, you could think of this as an example, or a generalization, rather, of the notion that if, let's say I had a signal that was recorded on a tape player, and if I play the tape back at, let's say, twice the speed, which means that I'm compressing the time axis linearly by a factor of 2. Then, in fact, what happens is that the frequencies that we observe get pushed up by a factor of 2.
And, in fact, let me illustrate that. I have here a glockenspiel, and anyone who loves a parade certainly knows what a glockenspiel is. And this particular glockenspiel has three a's, separated each by an octave. There's a middle a, a high a, and a low a.
And what I've done is to record the middle a on a tape at 7 and 1/2 inches per second. And what I'd like to demonstrate is that as we play that back, either at twice or half speed, the effective note gets moved down or up by an octave.
So let me first play the note at the speed at which it was recorded. And so what we'll hear is the middle a as I've recorded it. And let me just start the tape player.
Let me stop it, and hopefully what you heard is the same note from the tape recorder as the note that I played on the glockenspiel.
Now I'll rewind the tape, and we'll go back to the beginning of that portion. And now, if I change the tape speed to half the speed, so from 7 and 1/2 inches per second, I'll change the tape speed to 3 and 3/4 inches per second. And now when I play the tape back, because of the inverse relationship between time and frequency scaling, we're now scaling in time by stretching out, we would expect the frequencies to be lowered by a factor of 2. We should now expect the taped note to be an octave lower, matching this lower a. So let's just play that.
Let me stop it, and, again, we'll rewind the tape. Go back to the beginning, and now we'll play this at twice the speed. So I'll change from 3 and 3/4 to 15 inches per second. And now when I play it, we would expect that to match the upper note. And let's just do that.
Although that's a result that, intuitively, probably makes considerable sense, in fact, what that is is an illustration of the inverse relationship between time scaling and frequency scaling. And also, by the way, it was finally my opportunity to play the glockenspiel on television.
In addition, there is another very important relationship between the time and frequency domains, namely what is referred to as a duality relationship. And the duality relationship between time and frequency falls out, more or less directly, from the equations, the analysis and synthesis equations.
In particular, if we look at the synthesis equation, which I repeat here, and the analysis equation, which I repeat below it, what we observe is that, in fact, these equations are basically identical, except for the fact that in the top integral we have things as a function of omega, in the bottom integral as a function of t, and there's a factor of 1 over 2 pi, and, by the way, a minus sign.
You can look at the algebra more carefully at your leisure, but essentially what this says is that if x of omega is the Fourier transform of a time function x of t, then, in fact, x of t is very much like the Fourier transform of x of omega. In fact, it's the Fourier transform of x of minus omega to account for this minus sign. And, by the way, there's just an additional factor of 1 over 2 pi.
So the duality relationship which follows from these two equations, in fact, says that if x of t and x of omega are a Fourier transform pair, if x and X are a Fourier transform pair, then X, in fact, has a Fourier transform which is proportional to x turned around.
This duality in the continuous time Fourier transform is very important. It's very useful. It, by the way, is not a duality that surfaced in the Fourier series, because, as you recall, the Fourier series begins with a continuous time function and in the frequency domain generates a sequence, which would just naturally have problems associated with it if we attempted to interpret a duality.
And we'll see, also, that in the discrete time case, one of the important differences between continuous time and discrete time Fourier transforms is the fact that in continuous time we have duality, in the discrete time Fourier transform we don't.
Let's illustrate this with an example. Here are, in fact, two examples of Fourier transform pairs taken from examples in the text. The top one being example 4.11 from the text, and it's a time function which is a sine x over x type of function. And its Fourier transform corresponds to a rectangular shape in the frequency domain.
There's also another example in the text, the example that precedes this one, which is example 4.10. And in example 4.10, we begin with a rectangle, and its Fourier transform is of the form of a sine x over x function.
So in fact, if we look at these two examples together, what we see is the duality very evident. In other words, if we take this time function and instead think of a frequency function that has the same form, then we simply interchange the roles of time and frequency in the other domains. So the fact that these two correspond means that these two correspond.
Of course in this particular example, because of the fact that we picked a symmetric function, an even function, in fact, the additional twist of the time axis being reversed didn't show up in duality with this example.
One thing this says, of course, is that essentially any time you've calculated the Fourier transform of one time function, then you've actually calculated the Fourier transform of two time functions. Another one being the dual example to the one that you just calculated.
Also somewhat related to duality is what is referred to as Parseval's relation for the continuous time Fourier transform. And essentially, what Parseval's relationship says, as a summary of it, says that the energy in a time function and the energy in its Fourier transform are proportional, the proportionality factor being a factor of 2 pi.
That's summarized here. What's meant by the energy is, of course, the integral of the magnitude squared of x of t. And the statement of Parseval's relation is that that integral, the energy in x of t, is proportional to this integral, which is the energy in x of omega.
Although we've incorporated the Fourier series within a framework of the Fourier transform, Parseval's relation needs to be modified slightly for Fourier series, because of the fact that a periodic signal has an infinite amount of energy in it, and, essentially, that form of Parseval's relationship for the periodic case would say infinity equals infinity, which isn't too useful.
However, it can be modified so that Parseval's relationship to the periodic case says, essentially, that the energy in one period of the periodic time function is proportional with, this is the proportionality factor, proportional to the sum of the magnitude squared of the coefficients.
In other words, the energy in one period is proportional to the energy in the sequence that represents the Fourier series coefficients.
There are lots of other properties, and they're developed in the text and in the study guide. A number of properties that we want to make particular use of during this lecture, and in later lectures, are ones that I summarize here. And I won't demonstrate the proofs, but principally focus on some of the interpretation as the lecture goes on.
The first property that I have listed here is what's referred to as the time shifting property. And the time shifting property says, if I have a time function with a Fourier transform x of omega, if I shift that time function in time, then that corresponds to multiplying the Fourier transform by this factor.
As you examine this factor, what you can see is that this factor has magnitude unity and it has a phase, which is linear with frequency, and a slope of minus t0.
So a statement to remember, that will come up many times throughout the course, is that a time shift, or a displacement in time, corresponds to a linear change in phase and frequency.
Another property and, in fact, a pair of properties that we'll make reference to as we turn our attention toward the end of this lecture to solving differential equations using the Fourier transform, is what's referred to as the differentiation property and its companion, which is the integration property.
The differentiation property says, again, if we have a time function with Fourier transform x of omega, the Fourier transform of the time derivative of that corresponds to multiplying the Fourier transform by a linear function of frequency. So here it's a linear amplitude change that corresponds to differentiation.
At first glance, what you might think is that the integration property is just the reverse of that. If for the differentiation property you multiply by j omega, then for integration you must divide by j omega. And that's almost correct, except not quite. And the reason for the not quite is that recall that if you differentiate, what happens, of course, is that you lose a constant. And if we have a time function that's some finite energy signal plus a constant, differentiating will destroy the constant. The integration property, in essence, tries to bring that back.
So the integration property, which is the inverse of the differentiation property, says that we divide the transform by j omega, and then if, in fact, there was a constant added to x of t, we have to account for that by inserting an impulse into the Fourier transform.
And the final property that I want to draw your attention to on this view graph is the linearity property, which is very straightforward to demonstrate from the analysis and synthesis equations, which simply says if x1 of omega is the Fourier transform x1 of t, and x2 of omega is the Fourier transform of x2 of t, then the Fourier transform of a linear combination is a linear combination of the Fourier transforms.
Let me emphasize, also, that these properties, for the most part, apply both to Fourier series and Fourier transforms because, in fact, what we've done is to incorporate the Fourier series within the framework of the Fourier transform.
We'll be using a number of these properties shortly, when we turn our attention to linear constant coefficient differential equations. However, before we do that I'd like to focus on two additional major properties, and these are what I refer to as the convolution property and the modulation property. And in fact, the convolution property, as I'm about to introduce it, forms the mathematical and conceptual basis for the whole notion of filtering, which, in fact, will be a topic by itself in a set of lectures, and, in fact, is a chapter by itself in the textbook.
Similarly, what I'll refer to as the modulation property, again, will occupy its own set of lectures as we go through the course, and, in fact, has its own chapter in the textbook.
Let me just indicate what the convolution property is. And what the convolution property tells us is that the Fourier transform of the convolution of two time functions is the product of their Fourier transforms.
So it says, for example, that if I have a linear time invariant system, and I have an input x of t, an impulse response h of t, and the output, of course, being the convolution, then, in fact, if I look at this in the frequency domain, the Fourier transform of the output is the Fourier transform of the input times the Fourier transform of the impulse response.
You can demonstrate this property algebraically by essentially taking the convolution integral and applying the Fourier transform and doing the appropriate interchanging of the order of integration, et cetera. But what I'd like to draw your attention to is a somewhat more intuitive interpretation of the property. And the intuitive interpretation stems from the relationship between the Fourier transform of the impulse response and what we've referred to as the frequency response.
Recall that one of the things that led us to use complex exponentials as building blocks was the fact that they're eigenfunctions of linear time and variant systems. In other words, if we have a linear time invariant system, and I have an input which is a complex exponential, the output is a complex exponential of the same frequency multiplied by what we call the frequency response. And, in fact, the expression for the frequency response is identical to the expression for the Fourier transform of the impulse response. In other words, the frequency response is the Fourier transform of the impulse response.
Now in that context, how can we interpret the convolution property? Well, remember what I said at the beginning of the lecture, when I pointed to the synthesis equation and I said, in essence, the synthesis equation tells us how to decompose x of t as a linear combination of complex exponentials. What are the complex amplitudes of those complex exponentials?
In terms of our notation here, the complex amplitude of those complex exponentials is x of omega, or proportional to x of omega, in particular it's x of omega, d omega, and then a factor of 2 pi.
As this signal goes through this linear time invariant system, what happens to each of those exponential is each one gets multiplied by the frequency response at the associated frequency. What comes out is the amplitude of the complex exponentials that are used to build the output.
So in fact, the convolution property simply is telling us that, in terms of the decomposition of the signal, in terms of complex exponentials, as we push that signal through a linear time invariant system, we're separately multiplying by the frequency response, the amplitudes of the exponential components used to build the input. And that sum, in turn, is the decomposition of the output in terms of complex exponentials.
I understand that going through that involves a little bit of sorting out, and I strongly encourage you to try to understand and interpret the convolution property in those conceptual terms, rather than simply by applying the mathematics to the convolution integral and seeing the terms match up on both sides.
As I indicated, the convolution property forms the basis for what's referred to as filtering. And this is a topic that we'll be treating in a considerable amount of detail after we've also gone through a discussion of the discrete time Fourier transform in the next several lectures.
However, what I'd like to do is just indicate, now, a little bit of the conceptual ideas involved. Essentially, conceptually, what filtering, as it's typically referred to, corresponds to is modifying separately the individual frequency components in a signal. The convolution property told us that if we look at the individual frequency components, they get multiplied by the frequency response, and so what that says is that we can amplify or attenuate any of those components separately using a linear time invariant system.
For example, what I've illustrated here is the frequency response of what is commonly referred to as an ideal low pass filter. What an ideal low pass filter does is to pass exactly frequencies in one frequency range and eliminate totally frequencies outside that range. Another filter which is not so ideal might, for example, attenuate components in this band but not totally eliminate them.
In terms of filtering, we can think back to the differentiation property and, in fact, interpret differentiator as a filter. Recall that the differentiation property said that the Fourier transform of the differentiated signal is the Fourier transform of the original signal multiplied by j omega. So what that says, then, is that if we have a differentiator, the frequency response of that is j omega. In other words, the Fourier transform of the output is j omega times the Fourier transform of the input.
And so the frequency response of the differentiator looks like this, in terms of its magnitude. And what it does, of course, is it amplifies high frequencies and attenuates low frequencies.
Let me just, to cement some of these ideas, illustrate them in the context of one kind of signal, namely a signal which, in fact, is a spatial signal rather than a time signal. And this also gives me an opportunity to introduce you to our colleague, J. B. J. Fourier.
So if we could look at our colleague, Mr. Fourier, who, by the way, is not only a person who had tremendously brilliant insights, and his insights, in fact, have led to forming the foundation of the developments that is the basis for this course. He was also a very interesting, fascinating person. And there's a certain amount of historical discussion about Fourier and his background, which you might enjoy reading in the text.
In any case, what you're looking at, of course, is a signal. And the signal is a spatial signal, and it has high frequencies and low frequencies. High frequencies corresponding to things that are varying rapidly spatially, and low frequencies varying slowly spatially.
And so, for example, we could low pass filter this picture simply by asking the video crew if they could be slightly defocus it. And what you see as the picture is defocused, if you could hold it there, is that we've lost edges, which is the rapid variation. And what we've retained is the broader, slow variation. And now let's take out the defocusing low pass filter and go back to a focused image.
What we can also consider is what would happen if we looked at the differentiated image. And there are several ways we can think about this. One is that a differentiator-- Of course the output of a differentiator is larger where the discontinuity, or where the variation, is faster.
And so we would expect the edges to be enhanced if, in fact, we differentiated the image. Or if we interpret differentiation in the context of our filter, then what we're saying is that, in effect, what's happening is that the differentiator is accentuating the high frequencies because of the frequency shape of the differentiator.
Recall that this all fits together as a nice package. We expect intuitively that differentiation will enhance edges. When we talked about square waves and we saw how the Fourier series built up a square wave, we saw that it was the high frequencies that were required in order to build up the sharp edges. And so either viewed as a filter, or viewed intuitively, we would expect that the differentiated image would, in fact, attenuate this slowly varying background and amplify the rapidly varying edges.
So let's look again at our original image, just to remind you of the fact that there are edges, of course, and there is a more slowly varying background. And now let's look at the result of passing that through a differentiator. And, as I think is very evident in the resulting image, clearly it's the edges that are retained and the slower background variations are destroyed, which is consistent with everything that we've said.
I've emphasized that we'll be returning to a much broader discussion of filtering at a later point in the course. I'd now like to comment on another property, which is also, as I indicated, a topic in its own right, and which really is the dual property to the convolution property, and, in fact, could be argued directly from duality. And that is what's referred to as the modulation property.
The convolution property told us that if we convolve in the time domain, we multiply in the frequency domain. And we know that time and frequency domains are interchangeable because of duality, so what that would suggest is that if we multiply in the time domain, that would correspond to convolution in the frequency domain.
And, in fact, that is exactly what the modulation property is. I have it summarized here that if we multiply a time function by another time function, then in the frequency domain we convolve. Whereas the convolution property is just the dual of that, namely convolving in the time domain corresponds to multiplication in the frequency domain.
The convolution property is the basis, as I indicated, for filtering. The modulation property, as I've summarized it here, in fact, is the entire basis for amplitude modulation systems as used almost universally in communications.
And what the modulation property, as we'll see when we explore it in more detail, tells us is that if we have a signal with a certain spectrum, and we multiply by a sinusoidal signal whose Fourier transform is a set of impulses, then in a frequency domain we convolve. And that corresponds to taking the original spectrum and translating it, shifting it in frequency up to the frequency of the carrier, namely the sinusoidal signal. And as I said, we'll come to that in much more detail in a number of lectures.
We've seen a number of properties, and I indicated sometime earlier when we talked about differential equations, that, in fact, it's the properties of the Fourier transform that provide us with a very useful and important mechanism for solving linear constant coefficient differential equations. And what I'd like to do now is illustrate the procedure, the basis for that, and I think what we'll do is illustrate it simply in the context of several examples.
What I've indicated is a system with an impulse response h of t, or frequency response h of omega. And, of course, we know that in the time domain it's described through convolution, in the frequency domain it's described through multiplication. And I, in essence, am assuming that we're talking about a linear time invariant system. And we're also going to assume then it's characterized by a linear constant coefficient differential equation, where we're going to impose the condition that it's causal linear and time invariant, or equivalently that the initial conditions are consistent with the initial rest.
And it's because of the fact that we're assuming that it's a linear time invariant system that we can describe it in the frequency domain through the convolution property, and we can use the properties of the Fourier transform.
So let's take, as our example, a first order differential equation as I indicate here. So the derivative of the output plus a times the output is equal to the input. And now we can use the differentiation property. If we Fourier transform this entire expression, the differentiation property tells us that the Fourier transform of the derivative of the output is the Fourier transform of the output multiplied by j omega. And linearity will let us write the Fourier transform of this as a times y of omega. And since these are added together, and since we have the linearity property, these are added together. And x of omega is the Fourier transform of x of t.
So what we've used is the differentiation property, and we've used the linearity property.
We can solve this equation for y of omega. The Fourier transform of the output in terms of x of omega, the Fourier transform of the input, and a simple algebraic step gets us to this expression. So the Fourier transform of the output is 1 over j omega plus a times the Fourier transform of the input. And I've just simply repeated that equation up here.
So far this is algebra, and the question is, now, how do we interpret this? Well, we know that the Fourier transform of the output is the Fourier transform of the input times the Fourier transform of the impulse response of the system, namely the frequency response. So, in fact, if we think of h of t and h of omega as a Fourier transform pair, it's the convolution property that lets us equate this term with h of omega. So here we're using the convolution property.
So we know what the Fourier transform of the impulse response is, namely 1 over j omega plus a. We may have, for example, wanted in our problem, instead of getting the frequency response, to get the impulse response. And there are a variety of ways that we can do this. We can attempt to go through the inverse Fourier transform expression. But in fact, one of the most useful ways is formally called the inspection method. Informally it's called, if you worked it out going one way, then you ought to remember the answer so that you know how to get back method.
So what that says is, remember that we worked an example, and in fact I showed you the example earlier in the lecture, that the Fourier transform of e to the minus at times the step is 1 over j omega plus a? So what is the inverse Fourier transfer of 1 over j omega plus a? Well, it's e to the minus at times a unit step. And that's just simply remembering, in essence, this particular transform pair.
I've drawn, graphically, the magnitude of the Fourier transform here. And below it we have the impulse response. The impulse response is, as I just indicated, e to the minus at times u of t. Now let's go back up and look at the magnitude of the frequency response. And given just the little bit of discussion that we had previously about filtering, you should be able to infer something about the filtering characteristics of this simple, first order differential equation. In particular, if you look at that frequency response, the frequency response falls off with frequency and so what it tends to do is attenuate high frequencies and retain low frequencies.
So in fact, you could think of the defocusing that we did on the image of Fourier, you could think of that, approximately, as similar to the kind of filtering action that you would get by passing a signal through a first order differential equation.
Just to illustrate one additional step in both evaluating inverse transforms and using Fourier transform properties to solve linear constant coefficient differential equations, let's take the same example and, rather than finding the impulse response, let's find the response to another exponential input.
We could, of course, do that using the convolution integral. We've just gotten the impulse response, and we could put that through the convolution integral to get the response to this input. But let's do it, instead, by going back to the differential equation.
And so here I'm taking a differential equation. I'll choose, just to have some numbers to work with, a equal to 2. And now I'll choose an exponential on the right hand side, e to the minus t times u of t.
And again, we Fourier transform the equation. And we can remember this particular Fourier transform pair. It's just the one we worked out previously, now with a equal to 1. Clearly we're getting a lot of mileage out of that example. And now, if we want to determine what the output y of t is, we can do that by solving for y of omega and then generating the inverse Fourier transform.
Let's solve this algebraically for y of omega, and that gets us to this expression. And this is not a Fourier transform that we've worked out before. And this is the second part to the inspection procedure. What we have is a Fourier transform which is a product of two terms, each of which we can recognize.
And what we can consider doing is expanding that out in a partial fraction expansion, namely as a sum of terms. Because of the linearity property associated with the Fourier transform, the inverse transform is then the sum of the inverse transform of each of those terms.
So if we expand this out in a partial fraction expansion, and you can just verify that if you add these two together you'll get back to where we started. We now have the sum of two terms, and if we now recognize, by inspection, the inverse Fourier transform of this, we see that it's simply minus e to the minus 2t times the unit step. This one is plus e to the minus t times the unit step.
And so, in fact, it's the sum of these two terms which are the inverse transforms of the individual terms in the partial fraction expansion that then give us the output. So this is y of t, which is the sum of these two exponentials, and this is the inverse Fourier transform of y of omega as we calculated it previously.
Hopefully you're beginning to get some sense, now, of how powerful and also beautiful the Fourier transform is. We've seen already a glimpse of how it plays a role in filtering, modulation, how its properties help us with linear constant coefficient differential equations, et cetera.
What we will do, beginning with the next lecture, is develop a similar set of tools for the discrete time case. And there are some very strong similarities to what we've done in continuous time, also some very important differences. And then, after we have the continuous time and discrete time Fourier transforms, we'll then see how the concepts involved and the properties involved lead to very important and powerful notions of filtering, modulation, sampling, and other signal processing ideas. Thank you.
Free Downloads
Video
- iTunes U (MP4 - 108MB)
- Internet Archive (MP4 - 108MB)
Subtitle
- English - US (SRT)