Flash and JavaScript are required for this feature.
Download the video from iTunes U or the Internet Archive.
Topics covered: Analyzing Data
- Intention to treat (ITT) and Treatment on treated (ToT)
- Choice of outcomes and covariates
- External validity
- Cost-effectiveness
Instructor: Shawn Cole
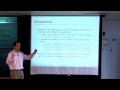
8: Analyzing Data
Related Resources
Lecture slides (PDF)
The following content is provided under a Creative Commons license. Your support will help MIT OpenCourseWare continue to offer high quality educational resources for free. To make a donation or to view additional materials from hundreds of MIT courses, visit MIT OpenCourseWare at ocw.mit.edu.
SHAWN COLE: Great. It's a real pleasure to be here and thank you for listening to me. This is perhaps the capstone lecture of the course or at least the last lecture of the course. And I'm going to try to pick up right where Michael left off talking about intention to treat and moving on to treatment of the treated. But with any luck, they'll be some time at the end where we can have more general discussions or questions if people are still interested about particular topics or have questions. And I'll stay after class to talk to people. And I think you have my email on the slides. So feel free to get in touch with me at any time. We've got this great project. We want to evaluate it or write JPAL.
So what are we going to do today? Look at some challenges to randomized evaluations. So these problems. So basically, when people don't do what you assign them to do because you can't control them. You can control undergraduates in a lab, but in the real world it's a lot harder.
Then we're going to talk about sort of how do you choose which effects to report in your study? So you've got your study, you did a bunch of household surveying, what do you want to report? How credible are your results?
Then we'll spend some time talking about external validity, which is sort of the question, OK, I have a study that I think is internally valid. We did the randomization correctly and the results we think are legitimate. But how much can that tell us about the greater world around us?
And finally, we'll conclude by talking about cost effectiveness. Which, as economists, is very important to us. So we may have a program that's effective, but how do we compare whether we should spend our precious aid or budget dollars on that particular program as composed to a host of other programs?
And so I usually teach at HBS in the case method, which is very different than a lecture format because it's basically the students always talking and me refereeing. I'm not going to do that today, but I'm very comfortable with interruptions, or questions, or requests for clarification, et cetera. And I think we all stay more engaged if there's more discussion. And so that's super valuable. So that's the outline for today.
And the slides up here are going to differ a little bit from the slides that you have printed out because there was some last minute optimization and re-coordination.
So the basic problem, which I think Michael talked about, is that individuals are allocated to treatment groups and they don't receive treatment, or individuals are allocated to the assignment group, but somehow managed to get treatment. So you talked about students didn't show up at school, so they didn't get the treatment on the treatment day. Or the program said you can't give this program, you can't give de-worming medicine to girls over the age of 13 because of health reasons. They may be pregnant and we don't know how the de-worming medicine affects pregnancy. So what do you do?
You came up with the solution of estimating the program effect, ITT. Which is to use the original assignment. So we have our baseline survey, our baseline list of schools or people and we flipped our coins or run our [UNINTELLIGIBLE] code to randomize. So then it would just evaluate them no matter what actually happened and them. We count them in the treatment group or we count them in the control group. And that gives us our intention to treat estimate.
And so the interpretation of that is, what was the average effect on an individual in the treated population relative to an individual in the comparison population? And you've already covered this with Michael? This is just a review. So is this the right number to look for? Well, if you're thinking about putting in a de-worming program, you have to realize that people aren't going to be at school when the government shows up to administer the de-worming program to all their students. And that's that.
So maybe we can just pause for a second and think about some programs that if you're an [? IPARA ?] you think you're going to be involved with, or if you're involved in an NGO, the type of program you're running. And maybe a few people can volunteer what the intent to treat estimate might look like in their evaluation and whether it's something they care about. Put this into practice.
I should be comfortable with long pregnant pauses as well. Any examples? Excellent.
AUDIENCE: So one of the projects that we're working on is looking at the impact of financial education in low income communities in New York City. Obviously we're trying to measure the impact that financial education has, but we're working specifically with a certain NGO to implement that education. Whatever estimate we get that's the intention to treat will just be measuring the impact of the program.
SHAWN COLE: And so what are the compliance problems you anticipate there?
AUDIENCE: Maybe people not showing up for classes or not following through on whatever they're asked to do in the education.
SHAWN COLE: Or people in the control group could get on the internet and download the government's financial literacy program and study very industriously themselves and learn. The intention to treat will tell you what the program does and you could imagine that if only 15% of the people turn up for your meetings, you're going to have a pretty small difference between the treatment group and the comparison group. That'll be an accurate measure of the program, but that's not going to tell us much about how important financial literacy is in making financial decisions. So if maybe your outcome variable is what interest rate do people borrow at, or do they pay their credit cards back on time, you might not find much effect. From that we can't conclude that financial education doesn't affect credit card repayment behavior. We just have to conclude that this particular program we delivered wasn't very effective. So that's an example. Any other examples? The point is hopefully pretty clear. Great.
So I don't know if you went through these calculations. Yeah, OK. It's absolutely simple. You just take the average in this one, average in this one, and it's the difference. So it's not rocket science. So it relates to the actual program and it gives us an estimate of the program's impact.
I guess the second method we're going to talk about, which you talked about in your learning groups this morning too, is treatment on the treated. And maybe you could motivate this by telling the joke about the econometricians or statisticians who went hunting. Have you heard this?
So the first one aims at a deer and shoots and misses 10 meters to the left. And the second one aims at the deer and misses 10 meters to the right. And the third one says, yes, we got it.
So the intention to treat is giving us the average program effect, but maybe we care more about what's the effect of knowing financial literacy? What's the effect of actually changing people's understanding of financial literacy? And that's where the treatment on the treated estimate can provide some assistance.
So again, we went back to the worming example which you talked about. And so we have 76% of the people in the treatment schools got some treatment in the first round. And in the next round it was 72%. So that's actually nowhere near 100%. One-fifth of the students are not getting their de-worming medicine. And some students in the comparison group received treatment also. So for example, I think when you were testing the children and you found that they had worms in the comparison group, the sort of medical protocol required you to give them treatment.
So what would you do if you wanted to know the effect of medicine on the children who took the medicine? And so you can't just compare children who took the medicine with children who didn't take the medicine. That leads to all the same selection problems we had in the first few days of class where the people who decided not to come to school that day or weren't able to make it to school that day are different than the people who did. And the people in the comparison group who went out to the pharmacy and bought the de-worming medicine are going to be different than the people who didn't.
So what we do in this case is something really quite simple and it's at the foundation of the entire field of modern empirical research. But we won't go into all the details, we'll just talk about this treatment on the treated estimator, or ToT. And so what you don't do is just take the change of the people who were treated and the change of the people who were not treated and compare them. That would be just silly because people who are treated are different than the people who are not treated.
So I think conceptually, this is actually a really simple thing that we're doing. So I don't want to get bogged down or confused in the math. But in the ideal experiment, in the treatment group 100% of the people are treated. In the comparison group, 0% of the people are treated. And then the average difference is just the average treatment effect.
But in real life when we do our experiments, we very often have leakage across groups. So the treatment control difference is not 1, 100% in the treatment group treated and 0% in the control group treated. But it's smaller. The formal econometric phrase for this is instrumental variables. We instrument the probability of treatment with the original assignment and this will rescale the difference in means to give us a better estimate of what the effect of the treatment on the people who were treated is.
So this is a simple example. And it turns out it gets more complicated. We're not going to go into all the nuances. But just suppose for simplicity that children who get the treatment have a weight gain of A, irrespective of whether they're in the treatment or in the control school. And children who get no treatment have a weight gain of B. Again, in both schools. And we want to know A minus B, the difference between getting treated and not getting treated.
This is the math. And maybe it looks complicated, but it's really not. I think if we work through the Excel worksheet explaining what we're doing and then go back to the math, it'll become pretty clear.
Imagine we run this experiment with pupils in School 1 and pupils in School 2. We intended to treat everybody in School 1. We intended for everybody in School 2 to be in the control group. Unfortunately, we were only able to treat 6 out of the 10 people in this group and 2 out of the 10 people in this group managed to get treatment somehow.
The formula sort of guides us through what we need to do and then we can talk about the intuition between what it is. Is there a heroic soul who's willing to try and just talk through the math and figure out? A non-heroic soul? Cold call.
So, Will, let's start out with the easy stuff. So the formula we want is the average in the treatment group minus the average in the control group divided by the probability of treatment in the treatment group minus the probability of treatment in the control group. OK, so how do we calculate all of these things?
AUDIENCE: So first you want to look at the change in the treatment group. So you average out the observed change in weight for the treatment group.
SHAWN COLE: Great. OK, so that's three.
AUDIENCE: You do the same calculation for the control group looking at the average change in weight. You take the difference between those two numbers and that's the numerator of the proper fraction.
SHAWN COLE: OK, so that's yt minus-- OK.
AUDIENCE: And then to do the second half of the calculation you've got the denominator. Compare the rate of compliance in the treatment group to the rate of compliance in the control group. So the percentage in the treatment group that actually complied would be 0.6.
SHAWN COLE: 1, 2, 3, 4, 5, 6. Awesome. OK.
AUDIENCE: And for the control group, it would be the 2 out of 10. So the rate that received the treatment. So 0.6 minus 0.2.
SHAWN COLE: 0.6 minus 0.2. OK. And now?
AUDIENCE: Just divide the top and bottom.
SHAWN COLE: OK, so that's the math. See if we got it right. Yes, we got it right. Excellent. So what's the intuition behind what we're doing here?
AUDIENCE: You're sort of doing a weighted average.
SHAWN COLE: OK, we're certainly taking the two averages and we're taking the difference of them. And what do you mean by weighting?
AUDIENCE: You're weighting them by the degree of compliance.
SHAWN COLE: So if the difference in compliance-- keeping the top term the same, so suppose that this is still--
AUDIENCE: If compliance were really horrible you'd end up with no effect. It could swap the difference between the control and the treatment.
SHAWN COLE: OK, so let's take the mental exercise, keeping this yt minus yc the same at 2.1. If the compliance in the treatment group goes down from 0.6 to 0.3, what's going to happen to the ToT effect? Is it going to go up or down?
AUDIENCE: Up.
SHAWN COLE: Why?
AUDIENCE: [UNINTELLIGIBLE PHRASE] The people that you were targeting are not receiving the treatment. So in a sense, the effect that you are trying to describe is not what we would expect would happen because some of these people did not comply with what you were expecting from the beginning.
AUDIENCE: It could go either up or down depending on the second parameter.
SHAWN COLE: OK, so what I want to do is I want to say there are four parameters here. There's the average in the treatment group. There's the average in the control group. There's the probability of treatment in the treatment group and the probability of treatment in the control group. If this goes down, what's the ToT estimate going to do? It's going to go?
AUDIENCE: Increase.
SHAWN COLE: Why?
AUDIENCE: Because you previously underestimated the effect because not that many people received the treatment.
SHAWN COLE: So mathematically, if we're making the denominator smaller, the size of the fraction has to go up. That's just a law of mathematics. But the intuition, what I'm looking for is the intuition. So what's going on?
So suppose I first tell you that this difference is 3. Can you guys not see these numbers here?
AUDIENCE: No.
SHAWN COLE: That's excellent. OK, so let me use a blackboard, I think this'll be a little bit helpful.
AUDIENCE: So were you asking just basically that because not everybody is being treated it's diluting the overall result?
SHAWN COLE: Explain this. Give us the sort of intuition. I said that this treatment group has an average of 3 and the control group has an average of 0.1 I think. No 0.9. And first I told you that the probability of treatment conditional on being in the treatment group is 0.6. But now I tell you actually it's much lower. It's only 0.3. So we know from the math that it's going to be higher, but why? What's the intuition? Why does it have to be higher?
AUDIENCE: Because the gap in outcomes is actually caused by fewer people. Meaning that for those two people it must have been a really big gap to balance out the average to still be a lot higher when everyone else in the treated group actually got zeros.
SHAWN COLE: Yeah, absolutely. So we've got some difference in observed outcomes. And that has to be caused by the fact that there were more people in the treatment group treated than in the control group who were treated. So if there were a whole lot of people in the treatment group treated, then this rate difference could be just that the average score is two higher for each person in the treatment group, if everybody in the treatment group were treated. But if only three people in the treatment group were treated, to raise the average from 0.9 to 3, you have to have a really big effect on those three people. So that's all the treatment on the treated estimator is doing, is it's rescaling this observed effect to account for the fact that the difference is not 1 here.
If we had perfect compliance, if this were 1 and this were 0, then the denominator of the fraction would just be 1. This would look just like the intention to treat and the intention to treat and the treatment on the treated estimators would be the same. But if we have imperfect compliance, then we can scale up the effect to account for the fact that all of the change has to be due to the fact that a smaller number of people were getting the treatment.
AUDIENCE: Now you can only do this because you believe there is no systematic difference for why people were treated or not in between these two groups?
SHAWN COLE: Right. So there's some technical assumptions on how you interpret these effects. But if we agree that the effect is basically a constant effect, so our literacy program has the same effect on everybody, then this is perfectly fine. And we can do this because we randomly assigned the treatment in the control group so ex ante the two groups are the same. Will?
AUDIENCE: So why do you not have to worry that the people in the treatment group who actually comply are the more motivated batch of the treatment group?
SHAWN COLE: Right. That is a concern and it's covered in the papers, which I'll get to at the end. Basically, the sort of aggressive intuition is that this tends to measure the effect on people who are affected by the program. But in general, this is a pretty good way of just scaling up your program effects to account for the possibility of noncompliance.
So moving back to-- sure.
AUDIENCE: Did you answer his question?
SHAWN COLE: Why do we have to worry about it?
AUDIENCE: Yeah. It seemed like it's a serious problem.
AUDIENCE: It started random, but then people who actually got treated, if they were treated because they were systematically different then it's no longer-- your control group is no longer a good representation of the [UNINTELLIGIBLE].
SHAWN COLE: Right. There are technical assumptions about monotonicity and independence that I sort of would rather not go into. But if you'll at least just grant that there's a constant treatment effect, then I think we're fine and by scaling up the impact to account for the non-compliers, we'll be OK.
AUDIENCE: Is the idea-- sorry not to belabor the point. But is the idea that this method will get the correct magnitude of the treatment on those who are treated in this study, but you can't necessarily extrapolate that to say, that would have been the same treatment on the treated. Would you be able to force these other people who might be different in some way to get treated.
SHAWN COLE: Right. This will tell you the correct magnitude of the people who because they were treated, get the treatment. So that's again, a relevant parameter when you're running a program that causes people to take the treatment, you have the effect. If on the other hand you're the government and you have some ability to compel compliance, then you might not get effect.
So you may worry about this. Some people have done studies to try and sort of see how big a problem this is. And one example I can cite is some studies on educational literature. So in the US people have looked at mandatory school attendance laws that say in some states you can drop out of school at the age of 16 and in some states you can drop out of school at the age of 15. And so changes in these laws induce some people to stay in longer. But probably nobody here would have been affected by one of these mandatory schooling laws because we were all planning to go on to college anyway. And so people have compared estimates in the US with those in the UK where the mandatory schooling law were very binding and they found that the point estimates were pretty similar. So that's an example of where it can be reasonable. But this is something that you have to treat with a little bit of caution.
So there are other challenges with this. To get the ToT, we need to know the probability of treatment conditional being on the treatment and the probability of being treated condition on being in the control group. So why might these be hard to get?
AUDIENCE: I actually had a question. I don't know if it's directly related. Does this work if the probability that you're treated in both the treatment and control turn out to be equal or is there probability that--
SHAWN COLE: Great question. So you're anticipating a slide three slides from now. So let's go there and then we'll come back to my other problem. But this is the equation. So what happens if the probability of treatment and the treatment in the control group is the same?
AUDIENCE: Mathematically you have problems.
SHAWN COLE: Mathematically you're dividing something by 0 and you're going to get infinity or negative infinity. So yeah, that's not successful. I mean, another way of putting that is that your experiment failed. You randomly assigned treatment and you assumed that your program, a financial literacy education program, de-worming medicine, is going to deliver a product. But if, in fact, it doesn't change the probability of treatment at all, then your program failed.
It's sort of like if I were to sit here and working with an [? MFI, ?] send them an email and say, I'm assigning these 50 villages to treatment and these 50 villages to control. And then the email gets lost and they don't do any sort of treatment or control. And then they send me the data back a year later and I do the analysis. Well, I'm going to find that there's no difference in probability of treatment between the two groups and I won't have anything interesting to say. So that's definitely one problem. The experiment doesn't work unless the treatment induces a change in probability of treatment between the treatment in the comparison groups. So that's one problem.
There's a second problem with this estimation since we're on this slide, which is perhaps, slightly more technical. But if the difference between the treatment and the comparison group is small. So say it's 10% of the treatment group are treated and none of the comparison group is treated. Then we're estimating the mean of all of the treated people minus the mean of all the control people and dividing by 0.1, which is the same thing as multiplying by 10. So if there's a little bit of noise in these measures, then instead of-- suppose the true mean is 3, but you happened to measure 3.1. Then that noise is going to be blown up by a factor of 10 as well. So the estimate is going to be much less precise when the difference in treatment between the treatment and the comparison group is low. And as you said, if it gets to 0, you're in a pickle.
AUDIENCE: That's a pretty extreme problem. It means I had a treatment group and only 10% of the people.
SHAWN COLE: Sure. That's true, but it's not implausible that you'd want to run that type of experiment. So if you think of-- I don't know the exact numbers on the flu encouragement design papers. To try and understand how effective a flu shot is, you can send out letters to a bunch of people and some of the people-- the control group, you just send a letter saying, just for your information, the influenza season is coming. Please wash your hands a lot. The treatment group you send a letter saying that information plus we're offering free flu shots down at the clinic, why don't you come down? And you may only get a 10% response rate from that letter. But in that case, the treatment is very cheap. It's just $0.32, or whatever a stamp cost these days.
So you could do a study with 100,000 people. Then you would estimate both of these things very precisely because you'd have 50,000 people in the treatment group, 50,000 people in the control group. And you'd have these numbers and so you could come up with an estimate. It's not like we should give up on an experiment that has a low probability of treatment, it's just if we think we're going to be there we want to do our power calculations carefully. And I think you would have seen when you did your-- did your power calculations include noncompliance? OK, so if you start adjusting the power calculations for noncompliance, you see that you need larger sample sizes. And so that's an important lesson as well.
Sticking with that flu example. Why might this be hard? When we sent out these letters to all these people either encouraging them to wash their hands or encouraging them to get flu shots.
AUDIENCE: We have to observe both. Whether there's treatment in your control in particular, that could be really hard to do.
SHAWN COLE: So maybe if you're directing them to your flu clinic, you're going sort of observe everybody you send a letter who comes in for a flu shot. And maybe they're employees of Blue Cross Blue Shield or something and you hope you'll get a good estimate.
But what about this, you've got 50,000 people you've sent letters to in the control group. What can you do? Do you have to phone up all 50,000 people and try and--
AUDIENCE: And say, oh, by the way, did you happen to get a flu shot.
SHAWN COLE: Exactly. So do we need to make 50,000 telephone calls to solve this problem?
AUDIENCE: Randomly sample.
SHAWN COLE: Randomly sample? So pick 500, even 1,000, or who knows? Maybe even 200 people, 300 people will give you a pretty good estimate of what this is. You just need to make sure that it is a randomly selected sample and you're not just asking all of the people in this particular clinic.
We're getting a little bit out of order. I've gone through the math, but PowerPoint wouldn't let you see it. But at least suffice it to say Will talked us through it. It's not a lot of fancy econometrics we're doing here. This is called the Wald estimate if you've taken econometrics. But it's a very simple method for coming up.
So there's some problems or there's some areas where this could be a problem. And Will hinted at one. We can mention a couple others. So how might this treatment on the treatment design fail, let's say, in the letters example, the influenza example? So we're sending out a bunch of letters.
So suppose the treatment group is you send out a bunch of letters saying, it's flu season, come get a flu shot. And the control group is you don't send out any letters. And so what happens? Suppose you get 50% compliance. So treated and the control group, compliance means flu shot. You get 50%. Let's make it easy, in the control group you get 0%. You do a sample and just nobody gets a flu shot. Maybe this is in a developing country where people don't tend to think about flu shots. And suppose you get the flu rate to be 10% here. We could say this is in Mexico. And 15% here. Do I have my math right? Yeah.
So what would the treatment on the treated estimate here be?
AUDIENCE: 20%?
SHAWN COLE: So what's the formula?
AUDIENCE: 10 minus 15. Divided by 0.5.
SHAWN COLE: Minus 0. So it's minus 10. So giving the flu shot to a population will reduce the incidence of flu by 10 percentage points.
So what's an example of how this experiment could fail, or how this number could not be correct?
AUDIENCE: By reminding people to get a flu shot, you remind them that the flu is out there and they might do other things besides get a flu shot.
SHAWN COLE: Absolutely. So now they wash their hands, or they don't go out in public places, or they wear masks. And so you think that you're reducing the flu a lot by these flu shots. But maybe in fact, washing your hands is a lot more important than-- in fact, I think it probably is-- washing your hands off and is a lot more important than getting a flu shot. And that's what's giving you the effect. So if you're scaling up this impact in the treatment on the treated to give yourself credit for the fact that 50% of the people didn't get a flu shot. If instead they're actually washing their hands a lot, then this won't be the correct estimate of treatment on the treated. Is that reasonably clear?
AUDIENCE: I have a question. Will it be a good estimate of the impact of that specific intervention on the treated? So instead of measuring the impact of the flu shot, you're measuring the impact of reminding people about flu shots and giving them access to free ones?
SHAWN COLE: So would you want to scale that up or not? Which estimate would you want to take there?
AUDIENCE: Depends on what you're interested in.
SHAWN COLE: So suppose we're interested in, what's the impact of sending a letter to somebody and offering them a flu shot? Is the correct estimate 5 percentage points or 10 percentage points?
AUDIENCE: 5.
SHAWN COLE: Why?
AUDIENCE: Then your intent to treat is maybe more interesting because you want to take into account that people change their behavior and their compliance rates are [UNINTELLIGIBLE].
SHAWN COLE: Right. So then we're really interested in the package of effects that sending a letter causes, which includes sometimes getting a shot, more likely. But also washing your hands or being more careful. So the intent to treat-- and as we say, so if you have this situation, you should only use intent to treat. And intent to treat is very interesting and it tells us the effect of sending out a letter, but it doesn't necessarily tell us the effect of the flu shot. Anybody have any examples from their own programs or projected projects where they're concerned about this? Maybe you guys can be at least sure to work this in on your presentations tomorrow.
What about when you have spillovers or externalities? So I think Michael talked about spillovers and externalities this morning. But he might not have integrated that with intention to treat. Is that a correct characterization of his lecture? Excellent.
How could we go wrong-- let's stick to something I know well-- with the Balsakhi Program. In the Balsakhi Program, we have treatment and control schools. And sort of the compliance is 20% in the treatment schools and 0% in the control schools. And the change in test scores is, let's say, 1 standard deviation. No, that's going to be way to high. 0.2 standard deviations here and 0 standard deviations here. So quickly, as a review, how do we get the ITT estimator?
This is what we call a chip shot at HBS. But since we're not awarding points for participation there aren't a lot of golfers out there.
AUDIENCE: Intention to treat? It's just 0.2.
SHAWN COLE: OK and how did you get that? You just saw it?
AUDIENCE: You just subtract it. You don't have to do anything.
SHAWN COLE: So it's 0.2.
AUDIENCE: Minus 0.
SHAWN COLE: Minus 0. Over?
AUDIENCE: Wait, are you saying--
SHAWN COLE: Oh, sorry. Intent to treat. Yeah, exactly. Yeah, you're right. Sorry, my bad. So the intent to treat is 0.2. And what's the treatment on the treated?
AUDIENCE: 0.2 minus 0.
SHAWN COLE: Great.
AUDIENCE: Over 0.2 minus 0.
SHAWN COLE: This is confusing. This is the standard deviations in test score and this is the percentage compliance. And so what's that going to give us?
AUDIENCE: 1.
SHAWN COLE: 1. So wow, that's a spectacularly effective program. I was very proud to be associated with it. It raised test scores by 1 standard deviation. Which if you know the education literature, is a pretty big impact.
What might we be concerned about in this case? So what did the Balsakhi Program do? Refresh ourselves. It takes 20% of the students, pulls them out of the classroom during the regular class, and sends them to a tutor. And these are the kids who are often in the back of the class, the teacher's not paying attention to them because they're only teaching to the top of the class. Maybe they're making trouble, throwing things around, et cetera. What could go wrong?
AUDIENCE: You're attributing the fact to just taking this class to just those students in particular. Whereas you're taking them out of the class, so you're making the class that you left behind smaller. So there's effects--
SHAWN COLE: Right, so how does making the class that you left behind smaller matter?
AUDIENCE: Usually it's easier to learn in a smaller group.
SHAWN COLE: That's why we teach a JPAL maximum class size of 15 or 20. We hope that small classes are more effective. And there's also a tracking argument that maybe now the teacher can focus really on the homogeneous group rather than having to teach to multiple levels, et cetera. So there are all these other reasons to think that it may be the case that they're going to be some spillovers. So how would you explain in words to a policymaker why you're not sure that 1 is the right effective treatment on treated? This is particular for the IPA folks who will have to be doing this to earn their paychecks. But anybody is welcome.
AUDIENCE: There were sidebar benefits to the control group.
SHAWN COLE: Right. Well, not the control group.
AUDIENCE: I mean to the--
SHAWN COLE: Untreated students in the treatment group. So we were attributing all of this test gain to sending these kids out to class. But in fact, there could've been some test gain in the other groups. In the extreme, I suppose you could imagine that there's no performance gain for the children who go out to the Balsakhis, but getting those misbehaving children out of the class causes everybody else to learn so much more effectively that it raises the test score. So the program still has an effect, but the way it has an effect is by getting the misbehaving children out of the class.
Now it turns out that that's not the case that there are sort of some interesting ways to try and tease out how big the spillovers were. And I encourage you to read the paper if you're interested. And it turns out that there's not really any evidence of spillovers, so it really does seem like the effect happened through the Balsakhi Program. But it's definitely something we want to be aware about when we're doing our analysis.
OK, so we've already talked about this. If you have partial compliance, your power may be affected. So the intention to treat is often appropriate for program evaluations. It's simple to calculate. It's easy to explain. If you do this program, you'll get a mean change in your outcome of 0.3, 0.4, 0.5. There are a lot of advantages to it. But sometimes you may be interested as well on the program itself. And as we said, it measures the treatment effect for those who take the treatment because they're assigned to it.
If you have people who will never ever ever take the treatment. If you tried to run a randomized evaluation on Christian scientists who refuse medical treatment and you assign them free medical care, you're not going to find any effect. But we can find the effect of the treatment on people who take the treatment when they're offered it.
And so when you're doing the design of your experiment, it's important to think through these issues and think at the end of the day, at the end of the study, in two years time when we've collected all the data and analyzed it, what sort of results are we going to report? How are we going to report them? And how are we going to explain them to other folks?
So that is intention to treat and treatment on the treated. And let's move briefly to the choice of outcomes and covariates.
We always look forward to your feedback from the course. But in my view, the course might benefit from a little bit more focus on some of the practical aspects of doing an evaluation. So for example, survey design and determining what outcomes. Although maybe many of you are already familiar with that. So often when you do these randomized evaluations, you do like a household survey or you have administrative data on the individual and you have 100 or you have 150 variables about them. So you go into the field with a 20 page survey and you sit and you ask, how many cows do you have? How many goats do you have? How much land do you have? What was your monthly income? Are your children going to school, et cetera.
And so if you imagine an outcome like microfinance, which we hope causes people to be more productive and engaged and boosts household income, arguably boosts women's empowerment, et cetera. There are sort of a lot of things that could plausibly happen because you offer people microfinance. But from a statistical side, this offers some challenges. So what's the problem when you're interested in how microfinance affects 40 different outcomes. So education, consumption, feelings of empowerment, number of hours worked. You know, you can come up with a lot of plausible things that you think microfinance would affect.
AUDIENCE: At a 0.5 level too, those will come out significant having 40 even though--
SHAWN COLE: Right. So for people who haven't done hypothesis testing, hypothesis testing, which is what we use to analyze data says, how likely is it that the difference between the treatment and the control group is because of the program or simply because of random chance? And so you can look at the distribution of outcomes in the treatment group and the control group and observe their means and their variances and the number of observations, and you can come up with a rule that says it's very, very unlikely. Only 1 in 100 times would this thing have happened because of random chance. The standard that tends to be used in economics and the medical literature is this 5% p value, which is 1 out of 20 times.
But if you're looking at 40 outcomes, then on average, 2 of them are going to be statistically significant just out of random chance. So if I were to just randomly divide this group, this class into two groups, and started looking at things like US born or foreign born, or Yankees fan or Red Sox fan, or what have you, it wouldn't be too hard to eventually find something that were statistically significantly different between the two groups.
This is a challenge. There are a few ways to deal effectively with this challenge. So this isn't a particularly difficult challenge. So what the medical literature does in which, I think, we're sort of slowly moving towards in social sciences, is you have to stay in advance where you expect to find effects. So if you're going to the FDA to test the efficacy of a drug, when you apply for the phase III evaluation you have to say, I think this is going to cure Shawn's male pattern baldness. And I think that this is going to result in a more charming personality. And then you find Shawn and all of his brothers and you run the experiment. And then if the outcome turns out that the treatment group is now never get sick, simply immune from all diseases. In the past year, nobody in the treatment group got sick, you can't then go out and market that drug as something that cures diseases because that wasn't your stated hypothesis test ahead of time.
So oftentimes, we have a lot of guidance on what hypotheses to test. So if we're doing a financial literacy program, we expect that to effect financial outcomes and not employment and not divorce rates. Although you could tell a story that eventually gets you there. But when you're reporting your results to the world then, what you want to do is report the results on all the measured outcomes, even the ones for which you find no effect. So then, anybody who takes the study can look and say, OK, they're saying that their program has a great effect on income and children's schooling and health. But they tested 200 things, so I'm a little bit skeptical. Or but they only tested 6 things and half of them were large statistically significant impact, so I really believe that study.
Maybe it's a little unfortunate the last class of the course is about sort of challenges with randomized evaluations because I should just take a sidebar to emphasize that these problems we're talking about are not unique to randomized evaluations. Any evaluation you run these risks. So if it's just sort of the standard, what we like to think of as a pretty bad evaluation where you just find some treatment people and find some associated comparison people and do a survey, you're going to run into this exact same problem. So don't take many of these as a criticism as randomized evaluation, but just take them as good science. What to do for good science.
And there are other things you can do which is to adjust your standard errors. So we have a very simple way of calculating standard errors. But if you're testing multiple hypotheses, then you can actually statistically take that into account and come up with sort of corrected or bounds on your standard errors. And those are described in the literature that I'm going to refer to you at the end of the talk as well.
AUDIENCE: What about taking half your data and mining it?
SHAWN COLE: And throwing the rest away?
AUDIENCE: No, I mine half of my data. I just figure out what really matters and that's how I generate my hypothesis.
SHAWN COLE: That sounds reasonable to me.
AUDIENCE: I better have had a big enough sample.
SHAWN COLE: Yeah, you better have had a big enough sample. I think sort of that intuition is-- data mining is problematic, but it is useful when you run a study to report all of your results because if there are some surprising things there, we don't know whether they're there because of chance or because the program had that effect. But that gives us sort of a view on what to do when we test again.
So if we're going to try this microfinance program out in some other country or some other area of the country, we said it had this surprising effect on girl's empowerment. So now let's make that one of our key outcomes and let's test it again. So I think that sounds pretty reasonable. There's a pretty advanced, developed statistical literature on hypothesis testing and how you develop hypotheses. But that sounds not unreasonable to me. Other thoughts?
So another possibility is heterogeneous treatment effects, which is what we often look for. So you might think that the financial literacy program is more effective with women than men because women started out with lower levels of initial financial literacy, or you might think that the de-worming medication is more effective for children who live near the river because then they play outside more often, or something like that. And so it's very tempting to run your regressions for different subgroups. But again, there's this risk that you're data mining.
Suppose I wanted to show that I randomly assigned you this group into a treatment and control group. And I wanted to show that Yankees and Red Sox preferences were significantly different in the two groups. I could probably cut the data in different ways and eventually find some subset of you for whom the treatment and the control variables were actually different. And so you want to be aware of that possibility. And again, like the FDA drug trial way to avoid this is just to announce in advance which subgroups you expect this product to be more or less effective for and make sure you have a sufficient sample size to test statistical significance within those subgroups.
Again, as a service to all the consumers of your studies, report the results on all the subgroups. Even the subgroups for whom the program's not effective.
So there's another problem that-- did we talk about clustering in groups, data in the power calculations? OK, so that is sort of the bane of statistical analysis. Or as we liked to say when I was a graduate student, sort of people used to not really appreciate the importance of these grouped errors. It was much easier to write a paper back then because you found lots of statistically significant results. And once you start using this cluster adjustment, it's a lot harder. Now only 5% of results are statistically significant. So we whined that if only we had graduated five years earlier, it would have been much easier to get our thesis done.
But we here don't care about getting the thesis done. We here care about finding out the truth and measuring the potential for cluster in standard errors, or clustering common shocks from the group is very strong. You can often get estimates of the correlation within groups using survey data that you already have. That will inform your power calculations. But when you're doing your analysis, you need to adjust your statistical results for this clustering.
And in particular, you run into problems when your groups are very small. So if you're thinking, I want to do an evaluation where I had 15 treatment villages and 15 control villages. Well, it's very likely that outcomes are going to be correlated within that village. And then, all of a sudden, you only have 30 clusters. And even the statistical technique isn't great for sample sizes that are that small. You have to use other statistical techniques, which are sort of valid, but less powerful. So we won't go into the randomization inference. Again, it's mentioned in this paper I was talking about. You should be aware of this and I think the most important time to think about this is when you're designing your evaluation, to make sure you get enough clusters.
And if you can, it's almost always preferable to randomize by individual than group. Because randomizing by group requires typically, much larger sample sizes.
AUDIENCE: Can you just say one more thing or give us a reference on what you meant by other statistical techniques that are valid, but not as powerful?
SHAWN COLE: Sure. Let me just skip to this. This should be on your slide package. The ultimate slide is additional resources. And so there's a paper called "Using Randomization in Development Economics Research: A Toolkit, by Esther, Rachel, and Michael. And this goes through everything we've talked about this week in pretty careful detail, works out the math, and gives you references to what you need. So it's in there.
Josh Angrist, who's on the faculty here, has a very good book called "Mostly Harmless Econometrics." But it's designed for academics. It's not really a textbook. But it goes through these things in very, very, very good detail and it's fun to read.
But specifically, the technique is called randomization inference. It was developed by Fisher. And what you do is you basically-- so you have your treatment and your control group and your mean between the treatment and the mean in the control and you test the statistical significance. And then what you do is you just randomly reassign everybody to either treatment or control, regardless of what they actually did, and see if there's a difference between the treatment and control group. And if you do that a hundred times, you can sort of get a sense for how often you find statistically significant differences or not. It's related to bootstrapping. That's a reasonable method, but the problem with that is the statistical power's not very good. So you need a larger sample. And then once you have a larger sample, then you don't need to worry about it because you can cluster.
So another question that's maybe a little bit more technical is when you're doing your analysis, your regression analysis, is what covariates do you want to control for? So we're looking at the effect of financial literacy education on credit card repayment. When we do our statistical analysis, do we want to control for the age of the person, for their gender, for their initial measured level of financial literacy, et cetera.
Now the beauty of randomization is that it doesn't matter. Even if you don't have data on any of these covariates, as long as the program was initially randomly assigned and the sample size is large enough, then you'll be OK. But what the controls can do is that they can help you get a more precise estimate. So if a lot of people's credit card repayment behavior is explained by whether they ate a cookie as a child or not and you happen to have that particular data for people who have been reading The New Yorker, then you can soak up some of the variation and come up with a more precise estimate. Or you can control for age, or control for income, or other things. So it's often desirable to have additional controls.
But what you don't want to do is control for a variable that might have been affected by the treatment itself. So if you're looking at the effect of microfinance on women's empowerment, so that's the goal of your study. And then you would say, well, women who have higher levels of income report higher levels of feeling empowered. So that's an important determinant of feeling empowered. We should include that in our control when we do our analysis. But if it turns out that microfinance increased income and increased control, then we might conclude that there's no effect because we're attributing the effect to the differences in income. Is that clear? A lot of these are fairly nuanced issues and it's often worth consulting an academic or often a PhD student are eager to work on projects like this as well.
Just as a rule, it's important to report the raw differences and the regression adjusted results.
I think we advance a very strong view that randomized evaluation is a very credible method of evaluation. But even still, there are always ways to tweak or twist things a little bit to try and get the results you want. So you could have a survey with a hundred people or a hundred outcomes and only report seven of them. That might make your program look better than it is. So these rules we're proposing are ways to give people an honest and a thorough view of the effectiveness of your program.
So another rule is that when you're reporting your regression results, you should include the results with the covariates, as well as the results without the covariates.
So now let's talk about threats to external validity. So we spent a lot of time so far talking about internal validity, which is sort of was the treatment randomly assigned? Did enough people in the treatment group comply that you have a difference between the treatment and control group? But it's not sufficient to know that we've learned a lot from a randomized evaluation. So there's some threats that just doing the evaluation itself may have some impact above and beyond the program. And so these are called Hawthorne and Henry effects. And maybe we'll go back to the audience for some examples of a Hawthorne effect.
So a Hawthorne effect is when the treatment group behavior changes because of the experiment. What's an example of that? Anybody familiar with the original Hawthorne study?
AUDIENCE: Was is the lights being dimmed or different levels of lights and workers felt like they were getting attention paid to them no matter what the light level was at? It's just that there was something going on and so their productivity was higher.
SHAWN COLE: I actually don't remember the study. Can I just try and rephrase that? You can tell me whether I'm right. So the experiment was to try and figure out how the level of lighting in a factory affects productivity? I suppose. And so they said we're going to raise the level of lighting in this sort of select, maybe even randomly selected. We randomly select 50 out of 100 of our work groups and we roll in an extra light. And they're like, oh great, management really cares about us. They're including us in this survey. They're giving us extra light. We're going to work extra hard. And so you find a higher output from that group.
Alternatively, if you had said, maybe people are getting distracted by having too much light. So management picked these 50 groups, went in and unscrewed some light bulbs. They're working in sort of a dim area, they'd be like, oh, wow. Management really cares about our well being. Now we focus on the natural light and we're going to work really hard. So just the act of sort of being in the treatment causes your behavior to change. And so what's wrong with that?
I mean we're trying to measure the effect of treatment. If the effect of treatment is to increase productivity, fine.
AUDIENCE: Maybe you can not make a generalization to a population that doesn't have change that behavior because doesn't get the treatment already.
AUDIENCE: You run around changing the light levels at different factories and you don't get the effect because the real treatment was people running around and testing and observing and measuring the change in light.
SHAWN COLE: Right. And saying, we're really glad you're part of this study. It's very important.
AUDIENCE: Which was really what got people to work harder.
SHAWN COLE: Right. You might be able to generalize that if we decided to run this study in every factory in our firm, then we might get similar results in different factories. But probably, within a few months, people would sort of just catch on that this is just kind of wacky, and what's going on? It wouldn't really be the effect of the program. Any other examples from your programs of Hawthorne effects? Or things you might be worried about?
AUDIENCE: It seems like a lot of behavior situations, there's a threat to this. Especially if you have some in developing country context where you have foreigners coming in or people from the capitol coming in. Especially if it's something that-- again, with a behavior change where OK, I know I'm supposed to be washing my hands with soap. I normally don't, but I know that the white people get really happy when I do it and they're coming in to evaluate. So I'm going to go ahead--
SHAWN COLE: Right. So if you show up from abroad and put posters encouraging people to wash their hands, people may pay more attention to that. What does that validate or not invalidate? So suppose we did this and we found that the effect was it reduces reported incidence of diarrhea by 15%.
AUDIENCE: If you then don't still have white people coming into the village, then the same effect might not happen.
SHAWN COLE: Right. So I guess it's a little bit nuanced because we should distinguish between the program generalizability, which is the program could be white people come into the village, and Hawthorne effects, which is because I know I'm in the treatment group in the study, I'm going to act differently. So what's another example of really, a Hawthorne effect? I'm sympathetic to yours as a Hawthorne effect, but I want to really sort of nail it.
AUDIENCE: There's one for hand washing where every week people go into the villages and then tell them the importance of hand washing as a way to prevent malaria. And every time they ask them, did you wash hands? Do you wash hands? But that's not sustainable on a long-term basis because-- and at the same time, you're distributing free soap. So how do you separate everything?
SHAWN COLE: I would say again, that's the program. And so we would say if we scaled that program up to all of the country, we'd be fine. If we go in every week and tell people to wash their hands and distribute soap, that's fine.
AUDIENCE: So sometimes on sexual behavior studies, you'll find that a treatment group that is encouraged to adopt condoms, or something. And then in the post-measurement period, they know what the intervention is about. And so if you ask them, what has your sexual behavior been in the last week, they're much more likely to say that they've been using condoms. Or change their partnering habits or these other sorts of things that have nothing to do with--
SHAWN COLE: Right. So the effect of being in the treatment group and knowing that you're getting this treatment might change how you answer the survey questions, even if you didn't behave that way. Any other?
AUDIENCE: For the Hawthorne effect?
SHAWN COLE: So it's certainly a problem with your survey.
AUDIENCE: It might change other aspects of their behavior other than condom use that would be simply because they know that you're looking.
SHAWN COLE: So I mean I would've said something like I know that they really care about me and so because I'm part of this MIT Poverty Action Lab Study, it must be really important that I do this. But if you were to generalize the program, not as part of a study, then people would react to it differently.
The other side of the coin is the John Henry effect, which is people in the comparison group behave differently. What are examples of that?
AUDIENCE: The village in the control group is resentful to the politician who they perceived as determining who got treatment status and so they don't try as hard on whatever's being measured. Or they intentionally turn in a poor performance as a sign of protest.
SHAWN COLE: Right. They're like, why am I in the control group of this study. I wanted to be in the treatment group. I'm not going to use fertilizer because I know this study's about fertilizer or something. Other thoughts?
AUDIENCE: You could do the opposite. I could say, oh, those guys, they got the treatment. But I don't need that. I can do just as well, so I'm going to--
SHAWN COLE: I'm going to pull myself by my bootstraps.
AUDIENCE: [UNINTELLIGIBLE] going to start studying and double up and I'll show them.
SHAWN COLE: That's an interesting problem, is we don't really know which way these effects go ex ante. The Hawthorne or John Henry effects could be positive or could be negative and could be a challenge for the evaluation.
So how do you sort of try and address this to resolve these problems?
AUDIENCE: It'd be hard to do statistically.
AUDIENCE: This could be dangerous I suppose. But if you try to make the people in the control group, for instance, feel special in some other way. But in a way that you can say is not related to anything you're measuring from the treatment.
SHAWN COLE: Right. So we're doing a financial literacy evaluation where we're showing financial literacy videos to the treatment group. In the control group we're bringing them in and we're showing them films about health or something that we don't think will have any effect on financial literacy, but lots of the things are the same.
In the medical literature they do often double blind studies, where you don't even know whether you're in the treatment group or the control group. So you can't get despondent for being in the control group because you don't know you're there. Sometimes these are sort of inevitable and you can't get around them, but you should think about them carefully and try to minimize the risk.
So another problem with evaluations is sort of behavioral responses to evaluations. So we assign some schools to treatment schools and some schools to comparison schools. And the people in the comparison school say, oh. So we give textbooks to the treatment school. So lots of people say, hey, this school's got new textbooks. I'm going to go to this school. And so that increases the class size. So the textbooks may benefit the test score, but the increased class size offsets that and you find no effect of textbooks because the behavioral responses undid this.
Whereas if you were to do it throughout the country and give every school new textbooks, then there'd be no transferring around because there'd be no reason to change schools because your school would have free textbooks as well. So that's sort of another.
AUDIENCE: I just wanted to go back to your question about how to minimize the Hawthorne and John Henry effect. We're doing an impact study, impact evaluation on microfinance product in Mexico. We try not to talk about the study. The people at the top know that they're implementing this study, but the participants don't know anything about the study. And it's very kind of hush hush, basically to try to avoid changing behavior. And obviously that's not always possible. But to the extent that it is, people don't have to know that they're part of a study.
SHAWN COLE: I'm sure everybody here who's lived in the US has participated in a study sponsored by a credit card company that does randomized evaluations to figure out how to get people to sign up for their credit cards. So they randomly send some people 10 point font on the outside letter, some people 12 point font on the outside letter. Some people 16 point font on the outside letter. And they keep track of who responds and they figure out that this is the right font size. And then they say, what color should it be? This is the right color. What should the teaser interest rate be? And so lots of firms do this without you even knowing it. And then you won't get any John Henry or John Hawthorne effects because people won't even know they're in experiments.
Sometimes there are sort of consent issues that you need people's informed consent that preclude that from happening.
There's some issues that we were touching on before, sort of the generalizability of results. So can the program be replicated on a large national scale? So we're going in and giving free soap to these villages, but it would get expensive to give free soap to every village in the country.
The study sample, is it representative? So what's a problem you might run into here?
AUDIENCE: If for logistical reasons, you're only doing it in one state in a country and it's randomized within the state, but then there's just a different culture in the state, or there's a really strong history or traditions in the state that then are not generalizable up to the country as a whole.
SHAWN COLE: A problem you often run into is NGOs will, I think for reasonable reasons say, OK, let's do this study at out best bank branch or at our best district. Because doing a study is pretty hard. You have to have treatment and you have to have control and keep track of who's in which group. And so these people have been with us for five years and they can do the study really well. And so you do the study and you find some nice effect, but that is the effect of putting your best people into the program and you only have 20 of them. And now when you try to scale it up to 500 villages, you just don't have that level of human capital to implement the same quality of program elsewhere.
So sensitivity of results. This is sort of important, but may be second order important. The state of the art and the sciences, we're still looking for things that work and work well. So we're not as worried about figuring out if we give the de-worming tablet every month versus every three weeks, which one is more effective. I mean that's a useful important question and it probably deserves a study. But it's hard enough to get the big picture studies done to then move onto the sensitivity of the results. That said, sometimes there are often interesting economic questions you have. So you want to know whether microfinance has an impact on people's wealth. But you might also care about the interest rate. And so for microfinance to be sustainable, the interest rate has to be high. But for it to generate a lot of income for the borrowers, the interest rate has to be low. So you could try your program at different interest rates and see whether you find the same effect at different interest rates. That would be very interesting.
So there's often a trade-off between internal and external validity. In my experience, I think it's probably reasonable to focus on the first pass on the internal validity. Because the advantage of picking your best branch or picking your good people to get the study done and done well and have a large treatment effect is that we were sure we know what we're measuring. It's often hard to measure effects in the real world. It's not as if the hundred people in this room are the first people to think we should do something to reduce poverty. It's a difficult and thorny problem. And so if we can throw sort of our best program and show that our best program is effective, then we can sort of work on expanding and testing our second best program.
Statistical power is often stronger if you have a very homogeneous sample. So if you can randomize in a set of twins or something like that, you have very good statistical power. But twins might not be representative of the general population.
And then, of course, the study location is almost never random. In Indonesia we did manage to do a nationally representative randomized evaluation of a financial literacy program, but that was just because the world bank was doing a nationally representative survey and we persuaded them to tack the experiment on at the end. But otherwise it's almost always prohibitively expensive to travel around to hundreds of locations.
But at the end of the day, you do care a lot about external validity. You want to know that before you throw a lot of money at the program, can you get the same effect when you scale it up? And is this program effective for large populations?
So in the last 5 or 10 minutes, we'll talk a little bit about cost effectiveness. So you've done your program, you've done your evaluation, you've got the efficacy. You know how much it costs to deliver the program. Now how do you decide which program to pursue?
I guess the important thing in this is pretty obvious. It's just finding a metric that you can use to compare different programs. So in educational programs we often look at years of schooling as an output. Having an extra teacher causes people to stay in school longer, but extra teachers are expensive. You can figure out how much it costs per child year of schooling you create.
In health programs, they have something called a disability adjusted life year, which I'm sure some of you know a lot better than I do. But it's basically an unimpaired year of life with no disability counts as one. If your legs are immobile, then maybe it'd be 0.6 or something. And it sort of gets adjusted down to figure out which health interventions are more or less cost effective. Or you could do cost per death averted.
I think the interesting takeaway here is that doing these types of comparisons can sometimes lead to pretty surprising results. So we know how to get people in school by reducing the cost of education, so the PROGRESA program in Mexico made conditional cash transfers to students' parents who attended school. Providing free uniforms increases attendance. Providing school meals increases attendance. We've looked at incentives to increase learning. But we've also looked at the de-worming case.
If you'd said five years ago to educational people who specialize in education in developing countries, what do you think a very high impact intervention would be? I think very few people would have suggested de-worming. But if you do the math, you can figure out that an extra teacher will induce let's say one year of additional schooling, but costs $55 per pupil. So the cost per additional year of schooling is here for extra teacher. Iron supplements here. School meals here. Deworming here. So it's just tremendously cost effective to provide this de-worming medicine as a means of increasing years of education. Much cheaper than scholarships for girls, et cetera, or school uniforms.
And you could do this calculation not just for education, you could say, there are a lot of things that we care about. We care about health outcomes, human capital investment, externalities. And so an interesting thing that came out of the de-worming study was that if you did the old studies that didn't take into account the externalities and just sort of treated some people in a school but not other people in a school, it didn't look like a very good intervention. Because the kids would keep reinfecting each other even though they'd just been treated, and so it wasn't that cost effective. But once you did the school level randomization and took into account for the externalities, then the program turned out to look very, very cheap as a way of providing education.
It's also an incredibly effective way of improving health outcomes, de-worming. And much more effective, for example, than treating schistosomiasis.
You can do even more calculations. You can say OK, so we know that the deworming medicine is going to increase years of education by 0.2. Well, what's 0.2 years of education worth? There are economists who have done estimates of the returns to schooling in Kenya. They say if you get an extra year of schooling, you're going to get 7% more income throughout your life. And then so you've got 40 years of life at 7% higher income. You can take the present value of that stream of additional wage payments and you can see that, wow, by investing only $0.49, we're going to generate $20 more in wages at net present value, on average. And so if you have a tax rate of 10%, that's clearly a profitable intervention for the government if it's patient enough. Because it'll take in $2 in net present value in taxes at a cost of only $0.49 of delivering. Of course, there may not be any taxes on informal labor in Kenya.
So I think this is an example we like to cite first, because Michael helped prepare this particular lecture the first time around and is fond of that paper. But second, I think it's a very nice example of a program that has a really big macro effect. So basically, it's been adopted nationwide in Uganda, and they're expanding it a lot in Kenya. It's been tried in India and many other countries have realized that this is a tremendously effective program. And the ability to have this randomized evaluation, there's very credible evidence that said, we had these treatment groups, these control groups. We followed people for three years and the reason why our results are so different than the other results that you were citing as a reason not to provide de-worming is because of these externalities. And we can show you why these externalities matter. The credibility of that study really helped to transform policy and literally save thousands, tens of thousands of lives. Maybe more.
Other examples. PROGRESA, which some of you might be familiar with. It's actually the government of Mexico decided to integrate a bunch of randomized evaluations into its social welfare programs. That methodology and the results of what's been shown effective in that program have been adopted throughout Latin America and elsewhere. And Ben Olken, whom you saw earlier, did some experiments on threat of audits in Indonesia for corruption. And the government of Indonesia is increasing the probability of audits as a way of fighting corruption in the nation's fourth most populous country.
So I think the conclusion is that these evaluations, which take a lot of time and take a lot of effort, let's not kid ourselves. If you sign up for one of these, it's going to be a big affair. But it can have a tremendous impact. It's very important to know from your own perspective how effective your program is, but you can influence policy a lot. So I'm just going to conclude with two things. One is this mention of the additional resources, which should be on the JPAL website. This is a book you have to buy for $60. Only buy this if you're already familiar with econometrics. But they're both great treatments of the material we've covered this week. And I believe JPAL is in the process of developing a practitioner's guide as well. This is a much more technical guide that's full of equations. But hopefully, as you've seen throughout the last week, we've tried to explain things in ways that are accessible that you can explain to people who haven't taken econometrics and that will hopefully be coming out pretty soon.
And so if I were just to at least take two seconds to give my perspective, I'm young but I've done a few of these. It's probably helpful when you're doing one of these evaluations to engage in academics.
If you're thinking of doing a study, you just send an email to JPAL. They'll send it out to their network of 15 or 20 people and I don't know what the response rate is. But I think there's a lot of interest in doing these experiments. IPA is another organization that does this. But there's some subtle nuanced issues that require careful thinking through. Or you could just call me up and say, we're going to be doing this study. We're spending a lot of money on it, can we just talk through these issues with you? I'd be perfectly happy to do that.
And then, one thought. Just a few thoughts on keeping your evaluation costs effective. And maybe even think about this when you're proposing your program tomorrow, is that randomizing at the individual level where possible and appropriate is a tremendously useful way to keep costs down. Because their statistical power is so much higher, so to detect any given effect size you can do it with many fewer observations.
Another way to save a lot of money is to use administrative data. So if you can get people to give you access in New York to their checking accounts and credit card statements, and get those reported, at a low cost to you, the administrative data is often of very high quality and can be collected for little money.
And then the third trick is sort of using lotteries to ensure high compliance. So if you sort of announce that you've got this new program and you let everybody who's interested apply, and then you sort of randomly select 60 and have 60 as a control group, then the compliance is going to be pretty high in the treatment group. That Wald estimator, the difference between these two things, is going to be pretty high.
So we're at 2:25, which at MIT is the end of the class. And I guess the end of the course, at least from the lecturing side. So thank you very much and I will be around here to answer questions for the next 15 minutes if people would like to talk.
Free Downloads
Video
- iTunes U (MP4 - 174MB)
- Internet Archive (MP4 - 340MB)
Subtitle
- English - US (SRT)