Flash and JavaScript are required for this feature.
Download the video from iTunes U or the Internet Archive.
Topics covered: How to Randomize II
- Unit of randomization
- Cross-cutting treatments
- Stratification
- Mechanics
Instructor: Rachel Glennerster
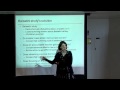
4: How to Randomize II
Related Resources
Lecture slides (PDF)
The following content is provided under a Creative Commons License. Your support will help MIT OpenCourseWare continue to offer high quality educational resources for free. To make a donation or to view additional materials from hundreds of MIT courses, visit MIT OpenCourseWare at ocw.mit.edu.
PROFESSOR: This is how to randomize two, and what--.
AUDIENCE: Are there slides?
PROFESSOR: Sorry. What we're going to talk about, it's just recap in case we missed anything this morning about the different methods of introducing an element of randomization into your project. Then I want to talk about the unit of randomization, whether you randomize individuals, or schools, or clinics, or districts. If you are very lucky and work somewhere like Indonesia, Ben Olken gets to randomize on the district level of many hundreds of thousands of people per unit of randomization, you need a very big country to do that.
Multiple treatments, and we'll go through an example of how you can design an evaluation with different treatments to get at some really underlying questions, big questions in the literature or in the development field, rather than just does this program work, but much more of the deep level questions.
Then I want to talk about stratification. And that's something where actually the theory has developed a little bit more, and as Cynthia can attest, it basically saved our project. In one case, we thought we just didn't have enough sample to do this, but we had stratified very carefully. And thank goodness we actually managed to get a result out of that project. And it was only because we did a good stratification that that was possible. So it's definitely worth thinking about how to do it correctly.
And then very briefly just talk about the mechanics of randomization. But I think that's actually best done in the groups. And we'll also be circulating and we'll put up on the website some exercises. If you actually literally-- I've learned all about randomization, but how do I literally do it? And the answer for us is normally-- the answer with me is I get an RA to do it. [LAUGHTER] You can write stata code, but you can also do it in Excel.
So this should be a recap of what you did this morning, but I just want to talk about-- I like kind of putting things in boxes and seeing pros and cons. The different kinds of ways of introducing some element of randomization into your project, to be able to evaluate it: basic lottery, just some in, some out, some get the program, some don't; a phase in. Can someone explain to me what a randomized phase in design is? Hopefully you did it this morning. Does anyone remember what a randomized phase in design is?
AUDIENCE: Is that the one where everyone gets it, but over time?
PROFESSOR: Yes. Everyone gets it in the end, but you randomize when they get it. So some people get it the first year, some people get it the second, and that's a very natural way in which projects expand over time. And so you introduce your element of randomization at that point and say, well, who gets it the first year is random.
Rotation, randomized rotation. Did Dean talk about that?
AUDIENCE: The way I remember it is it's almost like phase in, except for the service goes away from some people after a certain point.
PROFESSOR: Yeah, exactly. So with phase in, you're building up over time till everyone gets it. With rotation, you get it this year, but then you don't get it the next year. Encouragement, an encouragement design. OK, yeah?
AUDIENCE: Basically that treatment-- you use the same word. You're encouraging people to apply for a program or to get the intervention and then you're comparing all the people who have access to the program or the intervention versus people who don't.
PROFESSOR: You're comparing the people who were encouraged to go to the program, where they may not all actually get the program, but the ones who were given this extra special encouragement or information about the program, that's right. So let's just think about when those are useful. How do you decide which of those-- what are the times when you might want to use these? So basic lottery it's very natural to do when a program is oversubscribed. So when you've got a training course, and more people have applied for the training course then you've got places for.
Again, I'm sure Dean talked about the fact that that doesn't mean you have to accept everyone, whether they're qualified or not. You can throw out the people who aren't qualified and then just randomize within the people who are qualified. And that's OK when it's politically acceptable for some people to get nothing. Sometimes that's OK, sometimes it isn't OK.
A phase in is a good design when you're expanding over time. You don't have enough capacity to train everyone the first year, or get all the programs up and running the first year, so you've got to have some phase in anyway, so why not randomize the phase in? And it's also useful when politically you have to give something to everyone by the end of the treatment. Maybe you are worried that people won't cooperate with you. Maybe you just feel that unless they're going to get something at the end, maybe you feel that it's just inappropriate not to treat everyone you possibly can by the end. Whatever your reason, if you feel that you have to give everyone that you're contacting something by the end, then it's a good approach.
Rotation, again, is useful when you can't have a complete control. Just politically, it's difficult. People won't cooperate with you. In the Balsakhi example, they were very nervous that the schools just weren't going to let them come in and test the kids unless they were going to get something out of it. So they had to give them all something at some point, but you didn't have enough resources to do every one by the end. You only had enough resources to do half the people. So you can do half and then switch, and then the other half.
Now an encouragement design is very useful when you can't deny anyone access to the program. So it's been used and discussed in when you're setting up a business or former support centers that anyone can walk into and use, you don't want to say, if someone walks in your door-- you're desperately trying to drum up custom for this-- somebody walks in the door, you don't want to say you're not on our list, go away. That doesn't make sense for your program. Your program is trying to attract people. But it might make sense to spend some extra money to encourage some particular people to come and visit your center.
So it's very useful when everyone is eligible for the program, but the take up isn't very high. So you've got these centers, anybody could walk in, but most people aren't walking in. If most people are walking in and using the service anyway, you got a problem. Those are going to be very hard to evaluate, because you haven't got any margin in which to change things. But if take up is currently low, but everyone is ineligible, then that's an opportunity to do encouragement design.
It's also possible to do when you've only got two-- I was talking over lunch about trying to evaluate some agriculture interventions where they're setting up two rice mills in Sierra Leone. Two is just not enough to randomize. You don't want to randomize where you put the rice mill anyway. But you can talk about encouraging some people or informing some people that there's going to be a new place where they'll be able to sell rice or get extension services associated with the rice mill.
So advantages. A basic lottery is very familiar to people. It's easy to understand. It's very intuitive. You're pulling names out of a hat. You've got an equal chance of getting it. It's very easy to implement, and you can implement it in public. Sometimes it's useful to be able to show people that you're being fair. They see their names going into the hat, and they see people pulling them out of the hat. Sometimes that's important and useful to be able to do that.
Again, the phase in is relatively easy to understand what's going on. We're rolling it out, and we're giving everyone an equal chance of having it in the first year. Don't worry, you'll get it later on, but you'll have to wait a little bit. I understand what I'm writing here. Control comply as expect to benefit. Oh yes, so the control is going to comply with you. They're going to take the surveys because they know that they're going to get something in the end. So they're willing to keep talking to you for the three years because--
So the rotation will give you more data points than the phase in, because the problem with the phase in is over time, you're running out controls. By the end, you don't have any controls left. Whereas the rotation, you have some controls the whole time, because someone phase out. Encouragement, as I say, you can get away with the smaller sample size. You can do something even though you've only got two rice mills or two business centers in the whole of the country. And you can randomize an individual level, even when the program is at a much bigger level. But we'll talk more about the unit of randomization. You'll see what I mean by that in a bit more.
So the disadvantages-- I probably should have kept going along one line, but anyway-- so a basic lottery is easy to understand and implement. The disadvantage is that you've got a real control, and the real control doesn't have any incentive to cooperate with you. And sometimes that's a problem and sometimes it isn't. I mean, a lot of where I work in rural Sierra Leone, people are very happy to answer surveys. They don't have very much else to do. They like the attention. Oh, can you come and survey me too?
But if you're talking about urban India, there's lots of other things they should be doing. They've got to go get to their job. You've got to time the survey very carefully, otherwise they're really not going to want to talk to you. So you've got to worry a bit when you have really control in some areas about differential attrition. We'll talk about attrition later on in the week. But if you have more people unwilling to talk to you in control than you have treatment, you have a real problem.
A phrase in, again, it's very-- as they say, the advantage is it's very natural to the way that a lot of organizations work. They often expand over time. But there's a problem of anticipation of the effects. So if they know they're going to get it in two years, that may change what they're doing now. Yeah?
AUDIENCE: Can I ask a question about control groups not being willing to participate? Are there examples when an incentive has been used in order to get people to comply with the survey? There's obviously optimal ways to design that so you don't ruin the--
PROFESSOR: So sometimes we give small things, like didn't you use backpacks? Little backpacks for kids in Balsakhi?
GUEST SPEAKER: No, we used those to actually get our surveyors to comply. [LAUGHTER]
PROFESSOR: So sometimes people give things out. Normally we don't do that. Our most recent problem with it-- and there's also ethical constraints. So everything we do has to go through human subjects clearance. And you have to justify if you're going to give people an incentive to comply, and is that going to change how they respond? The one case we've had problems recently which really screwed us, as Eric can attest to because he was doing all the analysis, was people wouldn't comply to get their hemoglobin checked, which required a pin prick taking blood. And then the people in the treatment were more willing to give blood than the people in the control, and that caused us a lot of problems. But I think probably human subjects saying, we'll pay you to take your blood, we've had trouble there.
But I think if you're worrying about time and things, and kind of snacks, if it takes a long time, and you want people to come into centers to do experiments and games. Sometimes people use games as a way of getting an outcome measure, and that takes quite a lot of time to get them to play all these different games and see how they're playing them. And then providing food at the testing is kind of a very natural, and I think nobody's going to complain about that. And it helps make sure that people come in.
In the US, it's actually very common to pay people to submit surveys. So you give them a voucher, you'll get a voucher if you fill in the survey. I'm not used to doing surveys in the US, but I know my colleagues who do it in the US will pay to get people to send in surveys. So there's a bit of a cultural thing about what's the appropriate way to do this.
So as I say, with the phase in, you have to worry about anticipation of effects. And again, this really depends on what you're measuring, whether this is going to be a problem. If you're looking at accumulation of capital or savings, or buying a durable, if you know you're going to get money next year, then it'll effect whether you buy something this year. Where as if it's going to school, you're not going to wait till next year to go to school probably. So you have to think about it in the context of what you're doing.
The other real problem with a phase in is it's very difficult to get long term effects. Why is it difficult to get a long term effect in a phase in design?
AUDIENCE: Because within a short period of time, everyone has the treatment, and therefore it's hard to tell the difference between control and treatment phase.
PROFESSOR: Right. Because we are looking 10 years out, you're looking at someone who's had it for nine years versus 10 years. Whereas if you want the 10 year effective of a project, you really want somebody to have not got it for 10 years versus to have got it for 10 years. So that can often be the complete death knell to using a phase in. The one exception to that is if you've got a school program or a kind of age cohort, then you phase it in over time. Some people will just missed it because they will have moved on.
So one of our longest horizon projects is actually a phase in project, which is the deworming, because they're managing to follow up the cohorts who had left school by the time it reached their school. So it's not always impossible, but it's something you really have to think about.
Encouragement design, this you'll talk about more in the analysis session. You always have to think about what's the question that I'm answering. And with an encouragement design, you're answering the question, what's the effect of the program on people who respond to the incentive? Because some people are responding to the incentive to take up. Some people are already doing it without the incentive. Some people won't do it even with the incentive. When you're measuring the impact, you're measuring the impact on the kind of person who responds to the incentive, who's not your average person.
Now maybe that's exactly who you want to be measuring the effect on. Because if you're looking at a program that might encourage people to do more, say you're looking at savings and you've got an encouragement to come to a meeting of a 401k plan, and that encourages them to take up a 401k plan, that's kind of exactly the people you're interested in, who's a marginal 401k participant. In other cases, you're more interested in kind of the average effect of a program. So again, you have to worry about that. You need a big enough inducement to really change take up. If you change it a little bit, you're not going to have enough statistical power to measure the effect. We'll talk about statistical power tomorrow.
The other thing you have to worry about is are you measuring the effect of taking up the program, or are you measuring the effect of the incentive to take it up? If you do a really big incentive to take it up, that might have a direct effect. So there's no right answer as to which is the best design. It completely depends on what your project is. And hopefully this is what you're learning this week, is which of these is suitable to my particular problem and my situation. Yeah?
AUDIENCE: Could you explain what the control group would be in the encouragement, and how would you gather information about the control group?
PROFESSOR: So the control group in the encouragement is the people who weren't given the encouragement to attend. There's an example on our website of the 401k plan, and they're looking at what's the impact on-- they wanted to answer, if you take up a 401k plan, does it just shift your saving from one kind of asset to another kind of asset? Or does it actually totally increase your savings? So they needed some variation. They weren't going to be able to randomly persuade this company to have a 401k. Nobody is going to decide whether to have a 401k plan in their company based on the toss of the coin. It's too important of a decision. However, you will find that a lot of companies, a lot of universities-- and this was done within a university context-- a lot of the eligible people are not signed up.
So they took the list of all the people who are eligible who had not signed up and randomly sent letters to some of them saying-- I think maybe even a monetary encouragement-- to come to the meeting, where they learned about 401k plan. More of those people ended up signing up for a 401k plan than the people who had not received a letter. Some of the people who had not received a letter did sign up. But fewer of them signed up than the people who had received an encouragement to attend the meeting and sign up for a 401k plan. So all you need is a difference. More of the people in the treatment group sign up, the control group are the people who are not encouraged. And there were fewer of them who signed up. As long as there's a difference.
In our microfinance example, we have in our treatment areas where microfinance is offered, but it's not actually like we can say, you are taking microfinance, you are not. We can only offer it. It's available, and then people have to sign up for it. We have some difference-- not a huge difference, but some difference in the percentage of people who were offered microfinance who take it up versus those in areas where they were not offered it.
So all long as there's some difference there, you can statistically tease out the effect. And it's random whether you're offered. It's not random whether you take it up. It's random whether you're offered. And you'll learn in the analysis section how you actually cope with the analysis when not everyone takes it up, but some people take it up. Yeah?
AUDIENCE: How was that a nontrivial finding? More people that you market a 401k to will sign up?
PROFESSOR: No, no, that's not the finding. The finding is using the fact that more people who are marketed to sign up, you can then look at how their savings behavior changed. Did they just shift money out of their other savings and put it in the 401, or did it actually lead to an increase in total savings? And that's kind of the fundamental policy question about 401ks, does giving tax preference to savings, does it increase total savings, or is it just move your savings from one kind of instrument to another? And you look on average at the people who were offered, do they have totally more savings versus the people who were not encouraged to do 401ks? And then basically you adjust for the number of people who actually took up. Another question?
AUDIENCE: This subject has been brought up a couple times so far, but I'm still confused on. You say within the disadvantages, there's the problem that you're going to measure the impact of those who respond to the incentive. And this seems like a major disadvantage, that it puts in a lot of selection bias, because whoever is responding to the incentive. So what has been brought up so far is that you then look at the intended treatment rather than the treatment itself, but I still don't understand--.
PROFESSOR: OK, so you're going to do intend to treat versus treatment on the treated on Friday. So the actual mechanics of how you do it, we're putting it off. You're not meant to be able to do it yet, because you have a whole hour and a half on that.
AUDIENCE: But if you then do that, then does that selection disappear?
PROFESSOR: No. So you said you're worried about selection bias, the people who are going to show up. It's not that we measure the outcomes of those who sign up versus all the control. We measure, on average, the effect of all the people who are offered the treatment versus the average of all the people who were not offered. So on average treatment versus control where treatment is being offered, not taking up. So we have no selection bias.
If we see a change, we assume that all that change comes from the few people who actually changed their behavior as a result of the incentive. So say half the people take up and we see a change of 2%. If all the 2% is coming from just half the people changing their behavior, then we assume that the change in behavior there was 4%. Because it's coming from half the sample, and averaged over everyone it's 2%, so if it's only coming from half, they must have changed by 4%.
So that's what you do, very simply. It's not a selection bias because we're taking the averages of two completely equivalent groups. But we are taking it from the change in behavior of certain people. And so what we are measuring is how the program changed the behavior of those certain people. So it's not selection bias, it's just what are you measuring. Who's changing? Who are you looking at?
It's just like saying if you do the project in the mountains, you're getting the impact of doing the project in the mountains, whereas it may not tell you about what's the effect of doing it on the plains.
AUDIENCE: It's the external validity, right?
PROFESSOR: Yeah, it's external validity. But it really depends on the question. You can't say that's right or wrong. Because if your question is what happens to people who are in the mountains, then that's the right answer. If you want to know what happens to people in the plains, then you have to think about does this make sense?
In this case it's changing the effect on the marginal person who responds to incentive. If that's the very poor who respond to the incentive, then you know the effect of doing the program on the very poor. And maybe that's what you want to know. But as long as you know what the question is that you're answering, I think that's important. Then you can think about whether it makes sense, whether you've got an external validity question or whether-- you care about the poor anyway, so I'm happy.
So I've taken half an hour on one slide, so I should probably speed up. So unit and randomization. We just went over an awful lot material in that slide, so it's quite fundamental. So I'm glad you asked questions about it.
So the unit of randomization is are we going to randomize individuals to get the program, or are we going to randomize communities to get the program, or a group level? It could be a school, a community, a health center, a district. But it's a clump of people. So how do we make a decision about what unit to randomize at? So if we do it at an individual level, you get the program, you don't get the program, you get the program, we can do a really nice, detailed evaluation at a relatively small cost. That's the benefit.
But it may be politically difficult to do that. To have different treatments within one community, particularly if you're thinking-- imagine if you were in a school context, and you've got a class. And you say, well, you get a lunch. We're providing lunch to you, but I'm sorry, you don't get any lunch. It's just very hard to do that, and often inappropriate to do that. And what's more, it often doesn't work, because most kids, when they're given something and their neighbor isn't, they all share with their neighbor. At least most kids in developing countries, maybe not my kids.
And then you've just totally screwed up your evaluation. Because if they're sharing with their neighbor, who's the control, you don't know what the effect of having lunch is, because actually there isn't any difference between them. So that's not going to work. So sometimes a program can only be implemented at a certain level. There's just kind of logistical things which mean we're setting up a center in a community. We don't set it up for individuals, we set up one in the community. So we either do it or we don't. So that often gives you the answer right there as to what's the unit that you can randomize at.
Spillovers is exactly what we were talking about in terms of sharing the food. Sitting next to someone who gets the treatment may impact you. And if it does, you have to take that into account when you design what unit that you're going to do the evaluation at. So as they say, encouragement is this kind of weird thing that's halfway between the two, in the sense that the program may be implemented at a village or district level, but you can randomize encouragement to take it up at an individual level. So sometimes that's a nice way out of that.
So multiple treatments is sometimes people list as kind of an alternative method of randomization. But really, you can have any of these different approaches could be done with multiple treatments or with one treatment.
Now I'm going to take a little time to kind of work through a couple of different examples that are all based around schools. I don't know why. My last lecture was all based around schools. I guess you had Dean talking about different examples in the last lecture. So going back to the Balsakhi case and thinking about the problems that came out, and the issues around the Balsakhi case. We're going to look at two different approaches to answering some of those questions, and sort of discuss what are the pros and cons of the different ways of doing it.
So the fundamental issues, if we think about the needs assessment around Balsakhi, what were the problems in the needs assessment? You had very large class sizes. You had children at different levels of learning. You had teachers who were often absent. And you had curricula that were inappropriate for many of the kids, particularly the most marginalized kids. This was urban India, pretty similar problems in many developing countries. Most places I've worked have that problem, all of those problems.
So what are the different interventions that we could do to address those issues? We could have more teaches, and that would allow us to split the classes into smaller classes. If we're worrying about children being at different levels of learning, we could stream pupils, i.e. divide the classes such that you have people who are of more similar ability in each class. How do we cope with teachers often being absent? You could make teachers more accountable, and they may show up more.
How do we cope with the curricula being often inappropriate for the most marginalized children? Well, you might want to change the curricula and make it more basic or more focused on where the children actually are at. All too often the curricula appear to be set according to what the kids of the Minister of Education, their level rather than what's the appropriate level for kids in poor schools.
So how did the Balsakhi study approach those questions and try and answer those questions? The Balsakhi was limited in the fact that they had one treatment. So it's going to be a little more complicated to tease out all of those different questions, because this is going back to the question you discussed before, which is we've got a package of interventions. They had a package which was the Balsakhi program. And they managed to get an awful lot of information out of that. And then we'll look at an alternative that looks at multiple treatments and is able to get at these questions in a somewhat more precise way.
So in the Balsakhi study you've got this package, which is that each school in the treatment got a Balsakhi a tutor in grades three or four. The lowest achieving children in the class were sent to the Balsakhi for half the day, and all the children at the end were given a test. So that was the design of the project.
Now we're going to go through the questions that we want to try and answer. The first question is do smaller class sizes improve test scores? So as you went through in the case, even though it was one project and it was designed-- one study and it was designed to test the effectiveness of the Balsakhi program, they actually were able to answer this question to some extent by saying, the kids who were at a high level at the beginning didn't get these other elements of Balsakhi. All they got was that the low ability kids were moved out of their class.
So they actually had smaller class sizes as a result for half of the day. So that gives us a chance to look at does lower class sizes help? So you just compare the high achieving pupils in treatment and control, some of those in the treatment classes had smaller class sizes for half the day; those without did not.
Now another question that we had on our list is does having an accountable teacher get better results? So we're worried about teaches not showing up. If you make the teacher more accountable, do they show up more often, and do you get better results? Now in this case, the Balsakhi is more accountable than the regular teacher, because the Balsakhi is hired by an NGO. They can be fired if they're not doing their job. But the other teacher is a government teacher, and as we know, government teaches are very rarely fired, whether in developing countries or developed countries, whether they're doing a good job or not.
So we could look at the treatment effect for low versus high children. What do I mean by the treatment effect? What do I mean by that, comparing the treatment effect for low versus higher achieving children?
AUDIENCE: Maybe the result of the test scores.
PROFESSOR: Yeah, so we're going to use the test scores. But the treatment effect is whose test scores am I comparing to get the treatment effect for low scoring children?
AUDIENCE: The one with the government teacher versus the one with Balsakhi teacher?
PROFESSOR: So all the low scoring people are going to be-- if they're in treatment, they'll get the Balsakhi. Right? Yes, so it's-- I see what you mean. You're right. So what we're saying is what's the effect of the treatment for low scoring? That means compare the test score improvement for the low performing kids in treatment with the low performing kids in control. That's the treatment effect. What was the effect of the treatment? Compare treatment and control for low scoring kids. The difference is the treatment effect.
So what's the difference between treatment and control for low versus the treatment effect for high scoring kids? So basically all of the kids in the treatment group got smaller class sizes. Only the initially low scoring kids got the Balsakhi, got the accountable teacher. So if you look at the high scoring kids, you get just the effect of class size. If you look at just the low scoring kids, you get class size, lower class size, and accountable teacher. You also get a different curriculum. So you've got three changes.
One of the changes we've taken care of, because we've looked at that on its own. We've got smaller class sizes on its own by looking at just high scoring kids. So the two left are changing curricula and changing kind of teacher. And that's the difference between the treatment effect for low and the treatment effect for high scoring kids. So as I say, you've got two things going on for the low scoring kids. You've got three things going on, but we've controlled for one of them. The two things that are different about the low scoring kids is they get a different kind of teacher and they get a different kind of curricula. So it's going to be really hard to tease out those two things from each other.
So does streaming improve test scores? We can look at what happens to the high scoring kids, because they don't have-- we don't have to worry about the fact that they're changing the kind of teacher, because they've still got the government teacher. All they've got is the low scoring kids taken out of their class. So we could look at the high scoring kids. Now they found nothing. And that makes it easier to interpret, because if you don't find anything, and there's more streaming, they haven't got the low scoring kids in their class, and they've got smaller class sizes. So they didn't find anything. So they said, well, smaller class sizes didn't help and streaming didn't help.
But if they'd found a small effect, they wouldn't have known whether it was this or this. Because lots of things are being changed, and they've only got one treatment. That's basically what I'm trying to say. They managed to tease out a lot, but they can't nail down everything, because they're changing lots of different things about the classroom, but they've only got treatment versus control. So if they got a zero and two things going on, they can actually say, well, both of them must be zero. Unless of course, one helped and one hurt and they exactly offset each other.
Again, focusing on basic improvements, focusing the curricula, as we say, for the low scoring kids, there was an improvement, but we don't know whether it was because the teacher was more accountable or the curricula changed. We can maybe look at teacher attendance and see did that change a lot, in which case if it didn't, if the Balsakhi didn't turn up more than the regular teacher, then it's probably the curricula that's going up. Yeah?
AUDIENCE: Is it methodologically sound to compare side by side the treatment effect for low achieving students and high achieving students, even though they're starting in very different places? Can you make them comparable because they're starting at such different levels, and it might be more difficult to get from one level than from another?
PROFESSOR: Right. So again, you can't judge where low scoring kids improve by 10%, high scoring kids improve by 15%. Is one really bigger than the other, or is it just the way you measure it? Is it harder to get high scoring kids up or easier? Then you're in trouble. But in this case, the high scoring kids didn't see any improvement at all. So that's kind of easier to interpret, whereas the low scoring kids saw a huge amount of improvement. You could say, well, it's just hard to get the high scoring kids much better. But when we say high scoring kids, we're like they're on grade level. They're not massively behind grade level. It's not like they're such superstars that there's no improvement possible. They were just on grade level. That's all we mean by high scoring in this context. It wasn't that they were desperately falling behind.
But as I say, you don't want to compare an improvement of 10 versus an improvement in 15, because that depends on how you scored the test. So it's hard to interpret. But in this case, the program just didn't help the top. And in the textbook example that I talked about before, giving textbooks just didn't help the average kid. It only helped the top 20%. And then I think you can say something. Again, it's pointing you to the fact that maybe the curricula is just so over their heads. If you don't see any movement in the bottom 80% from a program, and you only see movement in the top, then you can say something.
Again, remember though my comment that you should say in advance what it is you're looking for. That you care about this, because you don't want to dice it every percentile. The 56th percentile, it really worked for them. It didn't work for anybody else, but the 56th percentile really-- well, that's not very convincing. But if you've got a story that I'm worried about the curricula being appropriate, and it's really the kids who are falling behind who are going to benefit most, and this is the intervention designed for them, then it's completely appropriate to be looking at does this intervention actually help the kids it was designed to help, which are the low achieving kids? That basic question is appropriate. Yeah?
AUDIENCE: This is a little off topic. In our group we were reviewing this, we talked about criteria for determining a low achieving student, and that that was not based on anything quantitative necessarily, that that was sort of a more discretionary choice on the part of the teacher. But in fact, the thing that you used to evaluate the efficacy of intervention at the end was a test. And it was a pretest. So I'm just curious about the test itself. Was this a specially designed test by you? Was this something that the NGO already had? Were students familiar with this kind of a test?
PROFESSOR: It was a test that was designed to pick out people who were falling behind the curricula. And the idea of the program was that we want to pull out the kids who are really falling behind the curricula. So it was a test designed to pick out the people that they thought would benefit from the program, and the things that they were targeting in the program. You're right that in a sense it would've been more effective to say, we'll decide based on the test which kids go. This test is designed to figure out who's going to benefit from the program, who's the target group for the program. We'll tell the teachers these are the kids that you should pull out.
But I guess it was just seen that in this context, the teachers have a lot of control. And it was seen that they needed to make that decision. Now in the end, they could see whether the kids that were pulled out were in fact the ones who scored lowly on the test. It wasn't a complete match, but it was a decent match. So this wouldn't make any sense if there wasn't some comparison comparative between those two, if it wasn't a pretty good predictor of who got the program.
AUDIENCE: Did you develop it, or had they already developed it?
GUEST SPEAKER: Pratham had developed it.
PROFESSOR: So it was the NGO--.
GUEST SPEAKER: Pratham hired them on the school curriculum. So they basically went through the textbook of the grades three and four-- actually on grades two and three, and included questions [UNINTELLIGIBLE].
AUDIENCE: And they did that specifically for the purposes of this study? Or was this part of their program, but it just wasn't the main criteria for the study?
GUEST SPEAKER: They were testing people independent of the study. But I think in this particular instance, this test was designed at the same time as the study.
PROFESSOR: They've since actually developed another tool which we now use in a lot of other places, which is a very, very basic tool for seeing basically can you read. And it's a very nice tool for sorting the very bottom. Because a lot of education tests are all aimed at the top, and they don't sort out the bottom half of the distribution. And Pratham's developed a very nice test, which they now introduce in-- they do a nationwide testing of kids across India. And they have a very good mapping of where a kid's falling behind. And it's a test that's really designed to get at the bottom. We now use it when I was doing a study in Bangladesh. And people in the World Bank are using it to do it in Africa. It's called a dipstick because it can be done quite quickly, but it gives you quite a good, accurate indication of where people are going.
So that was kind of complicated. We're having trouble sorting out exactly what was going on in different-- although we get a very clear idea of does the program work, and we know it works. If we want to try to get at these fundamental ideas, we got some sense from it, but it's a little hard to get at precisely. So how can we do a better job of really nailing these fundamental issues that we want to get at? Well, the alternative is that the old economics thing if you want to get four outcomes, you got to have four instruments.
So do smaller classes improve test scores? Well what's the obvious way to do that? You just do a treatment that adds teachers. Does accountable teachers get better results? You make the new teachers more accountable, and you randomize whether a kid gets a smaller class with an accountable teacher, or a smaller class with the old teacher, you randomize that. So you can test the new teachers who are accountable versus the regular teachers that were not accountable.
Does streaming improve test scores? Well, you do that separately with the different treatment. In some schools, you divide classes into ability groupings, and in other schools, you just randomly mix them. And you've got an opportunity to do that division because you've just added some more teachers. But you randomize so some schools get the division, unlike the Balsakhi, where all the classes got divided by ability. This case sometimes they will be and sometimes they won't, and then we've got more variation, and we can pick up those things more precisely.
Does focusing the basics improve results? Well, you can train some people to focus on the basics and only introduce those in some schools. This is an actual project did the first three of these. If you wanted to do this one, you'd have to add another treatment. So extra teacher provision in Kenya, at least one of those involved in Balsakhi went off and did it again with more schools and more treatments. Esther was part of both of these projects.
So they started with a target population of 330 schools. Some of them were randomized into pure control group, no extra teacher, exactly as they were doing before. Another bunch much more, and you'll realize why, because I'm about to subdivide this into many other things, got an extra teacher. And that extra teacher was a contract teacher, i.e. they could be fired if they didn't show up.
So then you split it into those who are streamed, i.e. they are grouped by ability when they're segregated into the different classes, and those who-- I shouldn't say ability, I should say achievement-- versus those who are just randomly divided between classes. There's no attempt to stream. Within that you've got your extra teachers. Some of them are contract teachers, some of them not. So you need to randomize which classes get the government teacher and which get the contract teacher.
What would happen if you didn't insist on that being randomized? Well no actually, that's fine, because these classes are the same. But when I go here, we've got some of the classes are grouped to be the low level learning when they enter school. In Kenya, this was done by whether you knew any English, because all the teaching is in English in school. So there were some kids who were turning up in school knowing some English and some kids turning up who have no words of English. So the idea is, that's kind of the fundamental thing when you start school. You're talking to a bunch of people, some of whom know English and some don't, can you adapt your teaching level to that? And this allows them to adapt their teaching to whether the kids in the class know any English or not.
So some are grouped. So these are all grouped by ability. You've got the lower level of English learning at the beginning and the higher level of English learning at the beginning. Now, if I didn't randomize, so some of these got government teachers and-- or contract teachers, and some of these got government teachers versus contact teachers. If I didn't insist on randomizing this, what would happen? What would I fear would happen, and why would that screw up my ability to do the evaluation properly? If I just sorted they by the level of scoring on English at the beginning, and then said OK, you've got contract teachers, you've got government teachers, you figure out who teaches which class. What would I worry about happening?
AUDIENCE: They would assign teachers in a systematic way, and you wouldn't be able to determine whether the effects were due to the tracking, or because we got the better teacher.
PROFESSOR: Right. My guess is all the higher level classes would be taken by the government teacher, and all the lower classes would be given to the contract teacher. And then I wouldn't know whether it was streaming works for high level but not for low level or vice versa, or whether it's something to do with the teacher. I have to take control and say, you're only doing this if I get to decide which class is taken by which teacher. But this way we know that half of the lower level kids got taken by a government teacher, half of them by a contract teacher. Which classes it was was randomized. Because otherwise I'm going to get all the low ones being-- or most of the low ones being taken by the contract teacher, most of the high ones being taken by the government teacher. And I've got two things going on, and only one difference that I can't sort out the effects.
AUDIENCE: If the interest is to determine the effect of [UNINTELLIGIBLE PHRASE].
PROFESSOR: But I'm not. What I'm-- lots of clicks involved. What were my questions at the beginning? Oh dear. I'm trying to identify things to deal with all of these. And I'm trying to identify a bunch of different things. I'm trying to identify what's the effect of smaller class sizes? I'm also worrying that children in these big classes are at different levels. So I also want to answer what's the effect of streaming kids? So that's our-- I agree. It's a whole separate question.
And I'm describing a design of an evaluation that does not answer one question. It answers three questions. This particular one didn't answer this one. So three questions that-- so one evaluation with multiple treatments is designed to answer three of, I would argue three of the most fundamental questions in education. How important a class size? How important is it to deliver-- to have coherent classes that are all at the same level so that you can deliver a message that's at the level of the children? And how important is it to make teachers accountable? Those are three completely different questions. The Balsakhi was getting some answers to these, but they only changed one thing. They only had one program, so it was hard to tease out. So we're designing this to answer those three different questions.
So you're right. If you just want to answer what's the effect of adding teaches, you don't need such a complicated design. But they also wanted to answer what's the most effective way of adding more teachers, or alternatively, just what's a better way of organizing a school? Does this improve the way the school works to do it this way? And then you see that we've got-- that's why we started with lots more schools in this group, because we're dividing it more times, and we need these different.
So how are we going to actually look at the results to be able to answer this question? So the first hypothesis we have is providing extra teachers leads to better educational outcomes. Just smaller class sizes, better educational outcomes. So to answer that question, we simply compare all the people who are in this group with all the people who are in control. That's the comparison when we do the analysis, we do that.
Our secondary thing is, is it more important to have smaller class sizes for lower performing kids? Are they the ones who benefit most? And then we can look at the low performing kids in these groups. This is a subgroup analysis saying, is the program effect different for different kinds of kids? Is this kind of approach most important for people who start at a given level? So that's relatively simple.
Our second hypothesis is students in classes grouped by ability perform better on average than those in mixed level classes. So this is exactly the second question, which is, I agree, a completely separate question. And that, we don't look at this one. Because these have a different class size, so you don't want to throw them in with this lot, because then you're changing two things at once. You take all of those who have smaller class sizes, some who are mixed ability, and some who are split by the level of attainment when they come in.
And a big question in the education literature is, maybe it's good for high performing kids to be separated out, but maybe that actually hurts low performing kids. So they were able to look at that. Actually they found that both low performing at the baseline and high performing at the baseline, both did better under streaming than under mixed classes. Their argument being that those who are in the low performing group were actually-- their teacher could teach to their level and they did better as a result.
Now the other question we have is, is it better to--?
AUDIENCE: Just a point of interest. That's a very different conclusion than has been found here.
PROFESSOR: Yes.
AUDIENCE: Because the biggest educational research study here found the Coleman effect, the benefit to the poorer kids. So it's interesting why in a developing country it would be so different.
PROFESSOR: I don't know the answer to that. It was interesting that virtually everything they found was opposite to what you find in the developed country example. So class size did not have an effect. Well, the class size evidence is somewhat mixed. But a lot of the more recent stuff, the randomized class size ones, I think, have found class size-- you probably know the-- Mike, you know the US education literature better than me. But my understanding is a lot of the recent stuff has found class size affects in the US. But now, some people argue it only helps if you bring it down below 20. And they're bringing it from 100 to 50. And a lot of educationalists will say, well, there's no point, because unless you give individual attention, and you can't give individual attention at 50. You've got to bring it way down.
So there's another question about these things could be very different in how much you would do. You can't just say class size doesn't matter. It may matter over some ranges and not over other ranges. But in terms of a practical proposal, these countries aren't going to get down to below 20. So they're arguing about should we get it down from 100 to 50, and it doesn't seem to have a very big effect in this context. What does have an effect is the streaming, and also which teacher you have; whether you have an accountable teacher.
So the accountable teacher, the contract teachers who have less experience, almost as much training actually in this context. In the Balsakhi case, the contract teachers had less training than government teachers, still performed amazingly; were the ones who saw the really big improvements. But here, the contract teachers are basically people who are trained and waiting to become a government teacher, but haven't-- they've trained lots of government teachers, but they haven't got any places for them. So they're mainly the people who are taking up these contracts did much better, much higher test scores.
You see here, we've got three different boxes with contract teachers, and three different boxes of government. And we can pool all of these together to make the comparison. Why can we do that? Because some of them have lower class sizes and some of them don't. But these have higher class sizes and these have higher class sizes. So the ones with higher class sizes are in both treatment and control, so we're fine. The average class size in all this red box is the same as the average class size in all this red box. We could pool all of them together, which helps a lot on our sample size, because you remember there are only about 55 in each one of these.
AUDIENCE: What exactly was the criteria for the contract teacher? What's their contract based on?
PROFESSOR: So their contract is with the local NGO who hires them. They have a whole other cross cutting thing which I haven't got into, which is some of the communities-- so they were hired by the community actually, with funding from an NGO. They were responsible to the community. And sometimes the communities were given extra training to help them oversee these teachers and sometimes they weren't. But they're contracted in the sense, if you get a job with the government, it's for life. And these guys can be terminated at any time.
AUDIENCE: Would they be terminated based on test stores?
PROFESSOR: No. So the main thing that's going on here is that these guys show up.
AUDIENCE: Oh I see, [UNINTELLIGIBLE].
PROFESSOR: It's just like they actually--
AUDIENCE: If you didn't show up, you would get fired?
PROFESSOR: Yes. So it's not like you've got to have an improvement of 10% in your test scores. No, it's like maybe if they like severely beat the kids regularly, or got known for raping the kids, which is actually relatively common, that might get them into trouble. But mainly the issue here is that they showed up, because they knew that if they didn't show up on a regular basis, they'd be fired. And that seems to be the big thing that's going on.
Interestingly, the cross cutting thing I talked about, we're looking at training the communities to oversee these teachers. Sometimes when you've got the contract teacher showing up, the government teacher showed up less. So sorry, I was saying about class size. We don't have lower class size, but we do have differences in these in terms of whether they're split randomly or streamed. So there are other differences going on between these different boxes, but they all have the same class size. And on average, they all have the same thing. On average, all the other characteristics are similar between these two groups.
Sometimes the government teacher saw there was an extra teacher, so they showed up even less than in this pure control. But where you had the community trained to oversee them, that actually happened less. So that was the one benefit of giving help to the community to oversee, because the contract teacher said look, the community's breathing down my neck. I'm meant to be teaching this class. I can't always be teaching your class as well.
But I'm just stunned in terms of the education literature. In all the work that we've done, this is just the first order problem. Just showing up is just one of the first order problems in education. And in health too. Health is even worse. Fewer health workers show up than teachers show up in any country we've ever studied. So that's not very complicated, it's just that they actually do their job. Yeah?
AUDIENCE: How do you parse out what [UNINTELLIGIBLE] the government teachers sometimes show up even less? So there's no counter-factual--?
PROFESSOR: Yeah, because you've got government teachers here, in the pure control. They are all government teachers. Now they have a bigger class size, but you can compare the government teachers here with the government teachers here. And indeed, that's what's giving you your-- a pure class size effect without changing accountability, you would look at this versus this.
AUDIENCE: Do you have any problems that spill over when the government teacher doesn't turn up, so the contract teacher has to teach both classes?
PROFESSOR: In a sense, that's just the program effect, right? It's not a spill over to the control, because these are all within the fact that then-- it's not like they're then showing up more to some control over here. So a spillover is when you have an effect on the control. But these aren't in the control, they're between these two. But that's the effect of having a contract teacher. When you have a contract teacher, you've got to take into account that it may change the effect. It may change what the government teacher does. So it's not going to be effective if it totally reduces what a government teaches does.
AUDIENCE: But wouldn't it limit your ability to compare across your different treatments? Because if you've got-- please go back to the last slide-- it influences your class size. Because if I've got a contract teacher who's now having to do the work of a government teacher too, what were two classrooms have now effectively become one, so it is a spillover in that that is now behaving like your control.
PROFESSOR: Well, when you look at the class size effect, you're looking at here, between these two. And what you're saying is, you think you've halved class size by having twice as many teachers. But actually you haven't, but you take into account when you're comparing that. So you're saying you're doubling the number of teachers, but the class size is improving by less than that slightly, because you've got more absenteeism.
But on the other hand, if you're looking at the program, is this a good thing to do? That's part of what it is. So in all of these cases, when you write it up and you explain what's going on, you don't just show the numbers, you explain this is the mechanism. And you're measuring all that you were talking about before in terms of the theory of change and measuring each step along the way. Then why is it we think is going on.
And then you can see, as I say, if you've got this other design of looking at which-- another treatment that actually managed to keep the two classes more separate than what's-- what do we see in those versus the ones that weren't able to keep the two separate. It wasn't that they never showed up. It was just that they did see that-- and it is important. What I would call that is a kind of unintended consequences, and that just emphasizes the fact that you really need to be thinking about what are some of the potential unintended consequences of what you're doing, so that you can measure them and make sure that you pick them up. And then if it doesn't work, maybe it's because of that. And then what can you do to offset that, and hopefully maybe you have another treatment that tries to offset that.
So then you can say, is it more effective in those ones where you've got tracking or not? Yeah?
AUDIENCE: Are government teachers assigned in the same manner within the community, where usually if you're training, you'd stay in the same community?
PROFESSOR: No.
AUDIENCE: I don't understand how large these communities are. But if you're comparing somebody who was drawn from a community, like a contractor was drawn from a community, and I understand you're mostly focusing on absenteeism, not the ability of the teacher. But if the teacher is drawn from the same community as the contract teacher, or the government teachers are randomized, is there any way you could have some sort of bias situation there? Because if you're from the same community, maybe you consistently get--.
PROFESSOR: OK, let's be really careful when we bandy around the word bias, because bias means something specific. So this is I think what you're talking about is, be careful that you're understanding what it is that's generating the difference between the contract teachers and the government teachers. I'm saying that it's the fact that they're accountable and they can be fired. But it may also be that they're more local to the area. They may live-- or not live, because they all live in the area now, but they are originally from that community, and that may make them more likely to be more responsive to the needs of the community.
So it's not just that they can be fired, it's that they know people in the community, and they're more responsive. So that's potentially part of the reason for why this is different from this. If again, if you're looking at the practical thing of what somebody would do when they do these kind of locally hired para-teachers, which is this isn't just made up for some academic point. This is happening around the world a lot, because governments can't afford to double the number of government teachers. So a lot of what they're doing in a lot of places is you have shiksha mitras in India, you have para-teachers across Africa. People who are hired, not tenured, and often have more roots in the local community.
AUDIENCE: And they're paid less?
PROFESSOR: Yes. A lot, lot, lot less. So these guys are paid a lot less than these guys. So you're right. You're changing a few things from this to this, and they're doing better. And is it because they're paid less? I doubt it's directly because they're paid less. But this is a relevant package is I guess what I'm saying. This is a relevant package that a lot of countries are exploring. And you're right, it could be some link to a local area, although a lot of people in Kenya, they will be allocated by the central government, but they will request to go to if not their exact community, to their general area.
And interesting, shiksha mitras in India are theoretically controlled by the local community, but actually the money comes from the state government at least. And they don't show up any more than regular teachers do. And they are literally from the community. So it doesn't seem to have helped that much in India.
So again, you can just split this and look at it, is the government teacher versus the contract teacher better in different situations or other situations? Are they particularly better for low performing kids or for high performing kids? And that you can only do though if you've got-- if the 55, remember we've got 55 in each of these. So when we pooled them, we have a lot more statistical power than if we try and-- where I'm just doing one box versus another box, I don't have a lot of statistical power. So you have to think at the beginning exactly how-- do you really want to tease out those real detailed questions, or am I OK with the general pooling of government versus contract teachers?
So that's an example of-- two different examples, Balsakhi, where we tried to answer all these questions with changing one treatment. So the benefits of these cross-cutting treatments is you can explicitly test these more specific questions. You can also explicitly test interactions if you do it in a cross-cutting, as opposed to one experiment here that reduces class size, one experiment here that has contract teachers. If you do it all in one place, you can see, does it work better if I pile all of them on together, or if it works better with this particular subgroup?
And often, you can economize on data collection because you're doing one survey, and changing different things. And as long as you keep a handle and keep it straight, there's a lot of piloting of the questionnaire, which you only have to do once. And maybe you only have to stick one RA and you hope that they cover 330 schools instead of 100, but you only have to pay one salary.
So the problem is that when you've got a cost-cutting design-- so a cross-cutting is this, where we're using all of the-- we're looking at government teachers versus contract teachers across these different groups, versus across these different groups. The negative is that I'm looking at a contract teacher versus a government teacher. In the background, some of those classes are streamed versus not streamed. It should all fall out in the wash, because these have streamed as well. So to the extent that streaming has an effect, it has an equal effect in these two.
And whenever you do an evaluation, some of the schools that you're working in will have some particular approach. Some of the schools you're working in will have a different approach. And you're changing across the schools, say the balance of half the schools will be Catholic schools, and half the schools will be government schools, and that's fine, because that's the world. Some schools are big, some schools are small. But you're introducing your own differences in the background, in the average score.
So if in Kenya, no one else streamed, no schools streamed, then you're testing this in an environment where over half of your schools are doing something that Kenyan schools don't normally do. So you're still getting a valid effect of the difference between contract versus government teachers, but you're testing it in an environment which may be a bit different, because you're also doing other things. So I guess what I'm saying is layering it in this way of lots of different things allows you to answer lots of questions with a smaller budget.
But you have to remember that in the end, some of these schools don't look like completely typical Kenyan schools, because they've got streaming. And most Kenyan schools don't stream. So you're testing something in a slightly atypical school. Is that important or is it not important? How important a concern is that to you? It's all about trade offs.
Stratification, 10 minutes to do stratification. The point of stratifying, you've done your little exercise on stratification in your cases? So you should understand it all. That's fine. The point of it is to give yourself extra confidence, extra chance, a higher chance than normal of making sure that your treatment and comparison groups are the same on anything you can measure. It's particularly important when you have a small sample. You did your little exercise, and so your bars go up and down, right? If you have a really big sample, it doesn't give you that much extra power, extra benefit, because they're going to be balanced anyway. Law of large numbers, if you draw enough times, it's going to be balanced anyway.
What is it? Sometimes people get really stressed out about what am I stratifying on? That's not the most important thing that you're going to learn here in what you stratify on. I would encourage you to stratify, but if you can't, again, it doesn't bias you, it doesn't give you the wrong answer, but it just helps on the margin. And sometimes that margin, as they say, we've discovered to our great relief, it saved our bacon when you're really on the edge of not having enough sample.
So all it is is dividing your sample into different buckets before you do your randomization. That's all it is. So it's a very simple concept. It's not always simple to do, but it's a very simple concept. Instead of putting everyone into one hat and pulling out of one hat, you divide it into different hats and then draw half of each hat. That's all we're talking about. So you select treatment and control from each of the subgroups, so you make sure that you're balanced on each shore.
So what happens if you don't stratify? What could go wrong if you don't stratify? What's the danger if you don't stratify?
AUDIENCE: You're taking a risk that some particular group don't get into the sample.
PROFESSOR: Right. So you're taking a risk that maybe more of your treatment ends up being richer than your control. And that was the whole point of randomizing, was to make sure that they were equal. And if you have a big enough sample, you'll be OK, they will be equal. But you risk it going wrong.
So if you do your pull, and even though you stratified it for some, or you can't stratify, you do your pull, and just by chance you get a really bad draw. And when you draw it, your treatment and comparison look very different, I would advise you to draw it again. And then phone us, and we'll tell you how to fix it in the analysis. Because you do have to do something funny with you standard errors, and that's fine.
But you're really screwed if, just by chance, you do your pull and you end up with all the rich guys in one treatment and all the poor guys in another. Then you're done. Then your evaluation isn't going to work. This is statistics, right? It's on chance, and by chance you just might get a really bad pull. This is stacking the decks to make sure it doesn't. If you do that and you see that it's just screwed, as I say, I would pull again.
Onto a different thing. So when should you stratify? You should stratify on the variables that have an important impact on your outcome variable. If you're trying to improve incomes, then it's income at the beginning. Or you're trying to improve education, and it's test scores at the beginning, those are going to be critical things. If it's something totally irrelevant, then you don't need to stratify it. So the most important thing is your outcome.
Stratify on subgroups that you're particularly interested in. Say you're really interested in what's the effect of the program on the poor, or the originally low achieving, or does this work for the Muslim minority, make sure you have enough Muslims in your treatment and control, otherwise you're not going to be able to answer that question. As I say, if you got a huge sample, you'll find we're virtually never in that situation, but it's particularly important to do it when you have a small sample set, or where your power is weak, and then it can just-- it's not going to help you enormously, but it just might push you over the threshold to getting enough power.
It starts getting very complicated to do if you try and stratify on everything. You're both income and gender, and you can imagine there may be some buckets that don't have any people in them. So it starts getting very complicated. It also makes it less transparent. It's very hard to do a complicated stratification and do a public draw. It's very easy to do a simple one, you just literally have two hats. Divide it into two hats and then you do the draw from the urban places and do a draw from the rural, and everyone can understand that. If you're trying to do urban and rural and rich and poor and whatever, then nobody is going to understand what you're doing with all these 15 different hats, and it's a mess.
So usually when we stratify, we do it on the computer. Again, if you do a public draw, you can't redraw if it turns out wrong. Increasingly we don't do public draws. You can stratify on index variables. You can put lots of things into an index and then stratify on that if you want to.
So mechanics, how do I actually do this? This is a comment from earlier courses, like we talked all about it, but how do I do a random drawing? We talk about hats, and you could do it in a hat, but we don't normally do it. The first and really important thing for designing your evaluation is you can only do a random draw from a list. You have to start with a list. And sometimes that's actually practically quite hard to do, because you don't have a list of all the people in the community. You have to go and get a list, there's really no other way to do it.
People will talk about-- and people do this when they do a random draw to interview people for doing a survey, to make sure you get a random sample-- you're surveying a random sample of people in the community, they'll talk about go to the center of the village, walk along the street and take every other person. Not a fan of that, because actually when people have done that for us, it's not a random sample, because they never get to the outlaying. And as we all know, the people who live in the outlaying parts of the community are very different from the people who live at the center of the community, so you really basically need to get a list. And that's expensive.
But you either use a census, or the list of kids in the school, or a list of schools from the Ministry of Education. And that we know can take a lot of heartache and time to persuade them to hand over those lists, or you go and do a census. Often we've just had to go and count people, list people, get the eligible people, write a list, and then draw from that.
So how can you do it? You could literally pull it out of a hat or a bucket. I keep talking about hats and buckets because it's very easy to visualize what you're doing. It's transparent, but it's time consuming and it's complicated if you have large groups, and it's hard to stratify. So what do we normally do? Well, you could use a random number generation in a spreadsheet program, and you order the observations randomly. Did you do an exercise where you actually did this? OK, so why am I talking to you about this? So I'm just going to go through it.
So you can use Excel to do this, and it's much better if you go over it in your groups and do it, or you can run a Stata program. Did you do a Stata program in your groups? No. But we can provide people with-- so I don't know, how many people here have used Stata? So this is a program, a statistical program, that economists tend to use. So the people who are familiar-- I wouldn't advise you to do it this way if you're not used to using Stata. But if you're used to using Stata, you know about it, but you just haven't done pulling a random sample from Stata, then we can just show you some code examples and you could build off those. For people who haven't done that, it's really perfectly possible to do it in Excel, as long as you don't get too complicated in your stratification.
We are also, I hope, going to be setting up a help desk in the near future for people who have attended this course. We're always available anyway for people who've attended the course to come and-- I'm doing this, you remember you talked about this, and I'm stuck because it's a bit more complicated than I thought I understood it. But we're going to actually formalize that process, and that would mean that if you need help actually doing it, you can come to us and ask us, can you just check my Stata code? Can you check that I'm doing this right in Excel? Because you don't want to screw that up.
And we'll have a team of graduate students or the kind of the people who are here TAing who will go over that and make sure that you-- because we would hate for the sake of a rusty nail that the horseshoe falls off and the kingdom is lost and stuff. If for the sake of getting your stratification or your random pull right, it's worth if you want someone to have a double check of that and make sure that you're doing it, that you're happy with it. Because if you get it wrong, then that invalidates all the rest of your work. Yeah?
AUDIENCE: When you stratify, aren't you compromising on the randomness?
PROFESSOR: No, because within groups you are randomizing. So all you're saying is I could randomly pull-- I could take all of this group and randomly draw some of you to come to the dinner tonight, and some not to. Or I could say I want to make sure that we have at least half-- equivalent numbers of men and women as we have in the course. And then I'd get all the men over on one side, and all the women over the other, and I would draw half of the men and half of the women. So it's still random. There's still equal chance of getting picked, I'm just making sure that I get proportionate.
So in the microfinance example where I said we got extra powers, we got communities, and we linked them up, we paired them as close as possible to each other. And then within pairs, one was treatment and one was control. So we always knew that there was somebody who looked almost identical to that community in the other bucket. There was always a control that was almost identical, and that meant that we just got less variation than we would have had. Otherwise, less variation gives you more statistical power.
But everyone had an equal chance of being in, we're just stacking the decks to make sure that they were as comparable as possible. Because when you draw randomly, by chance they could not be comparable. But if you literally pair them and switch one versus another, you are sure that there'll be a control that looks very much like the treatment. And you don't get the chance case that you just happened to get all the rich communities in the treatment and all the poor communities in the control.
With the stratifying, you have to take that into account when you do your analysis. So you just put in a dummy variable, those people who are actually going to run the regression at the end, you need to put in a dummy variable for each strata that you did.
AUDIENCE: What's the cost of stratification?
PROFESSOR: So that is the one cost. You have to put in a dummy in every case. So as I say, it gets quite complicated to do it when you have lots. The main--
AUDIENCE: And you cut a degree of freedom?
PROFESSOR: Yeah, you lose a degree of freedom.
AUDIENCE: You lose power?
PROFESSOR: Yes. So there's been a long esoteric debate in the econometrics, amongst the serious econometricians over the last year about whether you can decide not to put the dummy in the end. I think the conclusion is that you have to decide in advance whether you're going to put your dummy. So basically, you just have to put your dummy variables in. So you do lose a degree of freedom. The argument was, maybe then you could be worse off. Someone did a whole bunch of simulations. They proved that you could potentially be worse off. Nobody has been able, even in a simulation, to come up with an example whether you were actually worse off.
But you lost more power than you gained. But if you remember my advice to you was to do it on the ones that are likely to be important, and that is because potentially there is a theoretical possibility you could over-stratify, because you could lose some degrees of freedom at the end, because you have to put in a dummy for something that didn't actually help you reduce variance.
But as I say, even someone who is trying to prove this case did a bunch of simulations and couldn't find a single example where they actually ended up with less power through stratify. So my gut from this, and especially after having done the microfinance one was on the whole, I would-- now the main constraint is you normally don't have a lot of information before you start.
If you've done your baseline before you randomize, you have a ton of information. Then you can stratify on anything you have in the baseline. If you randomize before your baseline, you probably don't know very much about these communities, and it's pretty hard. But if you're randomizing after you've done your baseline, and it entered and cleaned, basically in order to do that, you have to delay your implementation for several months after you've done your baseline. If you want to do you implementation immediately you've done your baseline, you're probably not going to have that many variables entered. And then you just have to stratify on what variables you have entered or cleaned or whatever.
So that's usually the constraint, that you don't have much information on which to stratify. That's really what it comes down to usually. OK, I went over a bit, but any questions? Yeah?
AUDIENCE: I just have one question-- more of a clarification-- but it goes back to the questions-- the different levels of questions that you asked. So were those questions that the client came to you with? Or did the client come to you and say, we have a program, we want to know if it works? And then as you were talking with them, the different layers came out because you realized you're doing all this stuff?
PROFESSOR: So if you remember where I talked about in terms of when do you do an evaluation, I talked about different possibilities. And one was you have to program, that you want to test the program, and that was the Balsakhi case. And they had this program, they wanted to test it, and the researchers used the fact that the program did some things, and that some of the things affected some kids and not other kids to tease out some interesting questions.
In the extra teacher provision case, it was more like where I said, you want to know some questions? Set up a field site and just go experiment the hell out of it so that you can find out what the hell's going on, and you understand these deep parameters that then you could go off and design. So the extra teacher program was really that. It was saying there are these fundamental questions in education that we don't know the answer to. And we're going off the literature in the US where they've done lots of experiments, but we don't know if that's relevant to these countries.
So those are important questions to lots of people around the world. Let's find out what the answers to-- it wasn't really a very-- it was an NGO program, but it was mainly done for these research purposes, and these more general policy purposes. And it was paid for not by the NGO, the evaluation wasn't paid for by the NGO. But it was, I think, relevant. As I say, it is a relevant policy package, because the reason they did it was not just of academic interest to see does class size matter, but governments around the world are worrying about whether to hire more teachers, and should they hire them as government teachers or contract teachers?
There isn't actually much discussion in the policy world about the streaming. But given our other findings about how the curricula is so over the head of other kids, and the tentative findings from Balsakhi that it really looked like focusing-- the lower performing kids seemed to do better being pulled out, that was the motivation for saying, well, actually maybe this is something that's relevant in other cases. And I think it's more that that then raises the question, and then people are starting to think about it, rather than that streaming really was a big question in the policy context of Kenya at the time.
And the ETP one, I should say, that's probably one of our most complicated. Don't look at that complicated diagram of all the different things that were being tested and multiple treatments. That's kind of one of the most complicated designs we've ever done, so that's not like we're expecting everyone to go out of here, or go off and do something like that. But it was just kind of the extreme of this is the potential that you could potentially get at. OK, thanks.
Free Downloads
Video
- iTunes U (MP4 - 222MB)
- Internet Archive (MP4 - 427MB)
Subtitle
- English - US (SRT)