Flash and JavaScript are required for this feature.
Download the video from iTunes U or the Internet Archive.
Topics covered: Sample Size and Power Calculations
- Estimation
- Hypothesis testing
- Power: significance level, variance of outcome, effect size
- Clustered design
Instructor: Ben Olken
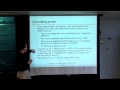
6: Sample Size and Power Ca...
Related Resources
Lecture slides (PDF)
FEMALE SPEAKER: The following content is provided under a Creative Commons license. Your support will help MIT OpenCourseWare continue to offer high-quality educational resources for free. To make a donation or to view additional materials from hundreds of MIT courses, visit MIT OpenCourseWare at ocw.mit.edu.
PROFESSOR: OK, so my name is Ben Olken and we're going to be talking about how to think about sample size for randomized evaluations. And more generally that the point of this lecture is not just about sample size but we've spent a lot of time, like in last lecture, for example, thinking about the data we're going to collect. Then the question is, well, what are we going to do with that data? And so it's about sample size but also more generally, we're going to talk about how do we analyze data in the context of an experiment. OK.
So as I said, where we're going to end up at the end of this lecture is, how big a sample do we need? But in order to think about how big a sample we need, we need to understand a little more about how do we actually analyze this data. When we say, how large does a sample need to be to credibly detect a given treatment effect, we're going to need to be a little more precise about what we mean by credibly and particularly think a little bit about the statistics that are involved in thinking through-- evaluate-- understanding these experiments.
And particularly, when we say something that's credibly different, what we mean is that we can be reasonably sure, and I'll be a little bit more precise about what we mean by that, that the difference between the two different groups-- the treatment and control group-- didn't just occur by random chance, right? That there's really something that we'll call statistically significantly different between these two groups, OK? And when we think about randomizing, right?
So we've talked about which groups get the treatment and which get the control, that's going to mean that we expect the two groups to be similar if there was no treatment effect because the only difference between them is that they were randomized. But there's going to be some variation in the outcomes between the two different groups, OK?
And so randomization is going to remove the bias. It's going to mean that the groups-- we expect the two different groups to be the same, but there still could be noise. So in some sense, another way of thinking about this lecture is that this lecture is all about the noise. And how big a sample do we need for the noise to be sufficiently small for us to actually credibly detect the differences between the two different groups, OK?
So that's what we're going to talk about is basically, how large is large so we can get rid of the noise? And let me say, by the way, that we've got an hour and a half, but you should feel free to interrupt with questions or whatever if I say something that's not clear because there's a lot of material that we're going to be going through pretty quickly. OK.
So when we think about how big our sample means to be-- remember, the whole point is how big does our sample have to be remove the noise that's going to be in our data? And when we think about that, we think essentially about how noisy our data is, right? So how big a sample we need is going to be determined by how noisy is the data and also how big an effect we're looking for, right?
So if the data is really noisy but the effect is enormous, then we don't need as big of a sample. But if the effect we're looking for is really small relative to the noise in the data, we're going to need a bigger sample. So actually, sometimes it's the comparison between the effect size and how noisy the data is. It's the ratio between these things that's really important.
Other factors that we're going to talk about are, did we do a baseline survey before we started? Because a baseline can essentially help us reduce the noise in some sense. We're going to talk about whether individual responses are correlated with each other. So for example, if we were to randomize a whole group of people into a given treatment, that group might be similar in lots of other respects. So you can't really count that whole group as if they were all independent observations because they might be correlated. For example, you all just took my lecture. So if you all were put in the same treatment group, you all were exposed to the treatment but you also all were exposed to my lecture and so you're not necessarily independent events.
And there are some other issues in terms of the design of the experiment that we'll talk about that can help affect samples as well, like stratification, control variables, baseline data, et cetera, which we're going to talk about, OK?
So the way we're going to go in this lecture is, I'm going to start off with some basics about, what does it mean to test a hypothesis statistically? And then when we get into hypothesis testing, there are two different types of errors that we're going to talk about. They're helpfully named type I and type II errors. And you have to be careful not to make a type III error, which is to confuse a type I and a type II error. So we'll talk about what those are.
Then we'll talk about standard errors and significance, which is, how do we think about more formally what these different types of errors are? We'll talk about power. We'll talk about the effect size. And then, finally, the factors that influence power, OK? So this is all the stuff we're going to go through, all right?
So in order to understand the basic concepts of-- when we're talking about hypothesis testing, we need to think a little about probabilities, OK? Because all this comes down, essentially, to some basic analysis about probability. So for example, suppose you had a professional-- and the intuition here is that the more observations we get, the more we can understand the true probability that something occurred-- whether the true probability that something occurred was due to a real difference in the underlying process or whether it was just random chance.
So for example, consider the following example. So suppose you're faced with a professional gambler who told you that she could get heads most of the time. OK, so you might think this is a reasonable claim or an unreasonable claim, but this is what they're claiming and you want to see if this is true. So they toss the coin and they get heads, right? So can we learn anything from that? Well, probably not because anyone, even with a fair coin, 50% of the time, they would get heads if they tossed it. So we're really can't infer anything from this one.
What you saw that they did five times and they got heads, heads, tails, heads, heads. Well, can you infer anything about that? Well, maybe. You can start to say, well, this seems less likely to have occurred just by random chance. But you know there's only five tosses. What's the chance that someone with an even coin can get four heads? Well, we could calculate that if we knew the probabilities. And it's certainly not impossible that this could occur, right?
And now, what if they got 20 tosses, right? Well, now you're starting to get information, although in this particular example, it was closer to 50-50. So now you have 12 versus eight. Could that have occurred by random chance? Well, maybe it could have, right? Because it's pretty close to 50-50. And now, suppose you had 100 tosses or suppose you had 1,000 tosses with 609 heads and 391 tails, right?
So as you're getting more and more data, right, you're much more likely to say something is meaningful. So if you saw this data, for example, the odds that could occur by random chance are pretty high. But if you saw this data with 609 heads and 391 tails out of 1,000 tosses, it's actually pretty unlikely that this would occur just by random chance, OK?
And so this shows you, as you get more data you can actually say, how likely was this outcome to have occurred by random chance? And the more data you have, the more likely you're going to be able to conclude that actually, this difference you observed was actually due to something that the person was doing and not just due to what would happen randomly.
And in some sense, all of statistics is basically this intuition, which is, you take the data you observe and you calculate what is the chance that the data I observe could have occurred just by random chance. And if the chance that the data I observed could have happened just by random chance is really unlikely, then you say, well then it must've been that your program actually had an effect, OK? Does that make sense? That's the basic idea, essentially, of all of statistics is, what's the probability that this thing could have happened randomly? And if it's unlikely, then probably there was something else going on.
Here's another example. So what this example shows is, now suppose you have a second gambler who had 1,000 tosses and they had 530 heads and 470 tails. What this shows is that-- and that's really a lot of data. But in some sense, what we can learn about this data depends on what hypothesis we're interested in. So if the gambler claimed they obtained heads 70% of the time, we could probably say, no, I don't think so, right? This is enough data that the odds that you would get this data pattern if you had heads 70% of the time are really, really small, right? So we could say, I can reject this claim.
But suppose they said that they claim they could get heads 54% of the time, OK? And you observe they got heads 53% of the time. Well, you probably couldn't reject this claim, right? Because this is similar enough to this that if this was the truth, this could have occurred by random chance. So in some sense, what we can say based on the data depends on how far the data is from our hypothesis and how much data we have. Does that make sense as some basic intuition? OK.
So how do we apply this to an experiment? Well, at the end of the experiment, what we're going to do is we're going to compare the two different groups. We're going to compare the treatment and the control group. And we're going to say-- we're going to take a look at the average, just like we were doing in the gambling example. We'll compare the average in the treatment group and the average in the control, OK? And the difference is the effect size.
So for example, in this particular case, in the Panchayat case, you'd look at, for example, the mean number of wells you've got in the village with the female leaders versus the mean number of wells in the villages with the male leaders, OK? So that's in some sense our estimate of how big the difference is. And the question is going to be, how likely would we have been to observe this difference between the treatment and the control group if it was just due to random chance, OK? And that's what we need the statistics to figure out.
Now one of the reasons-- so where does the noise come from? In some sense, we're not going to observe an infinite number of villages. Or we're not going to observe all possible villages. In fact, even if we observe all the villages that exist, we're not going to observe, in some sense, all of the possible villages that could've hypothetically existed if the villages were replicated millions and millions of times. We're just going to observe some finite number of villages.
And so we're going to estimate this mean by computing the mean in the villages that we observed, OK? And if there are very few villages, that mean that we're going to calculate is going to be imprecise because if you took a different sample of villages, you would get a slightly different mean, OK? If you sample an infinite number of villages, you get the same thing every time.
But suppose you only sampled one village. Or suppose there was a million villages out there and you sampled two, right? And you took the average, OK? If you sampled a different two villages, just by random chance, you would get a different average. And sometimes that's where the part of the noise in our data is coming from.
So for example-- sorry. So in some sense, what we need to know is, we need to know if these two groups-- it sort of goes back to the same as before, if these two groups were the same and I sampled them, what are the chances I would get the difference that I observed by random chance? So for example, suppose you observed these two distributions, OK? So this is your control group and this is your treatment group. Now you can see there is some noise in the data, right? This one is a mean of 50 and this one is a mean of 60. And there's some-- these are histograms, right? So this is the distribution of the number of villages that you observed for each possible outcome.
So you can see here that there's some noise, right? It's not that everyone here was exactly 50 and everyone here was exactly 60. Some people were 45. Some were 55 or whatever. But if you look at these two distributions, you could say it's pretty unlikely that if these were actually drawn from the same distribution of villages, all of the blue ones would be over here and all the yellow ones would be over here. It's very unlikely that if these were actually the same and you draw randomly, you get this real bifurcation of these villages, OK?
And where are we basing that idea, that conclusion on? We're basing the conclusion on the fact that there's not a lot of overlap, in some sense, between these two groups. But now, what if you saw this picture, right? What would you be able to conclude? Well, it's a little less clear. The mean is still the same. All the yellows still have an average of 60 and all the blues have an average of 50. But there's a lot more overlap between them. Now if we look at this, we can sort of eyeball it and say, well, there's really a pretty big difference even relative to the distributions there. So maybe we could conclude that they were really different. Maybe not.
And what if we saw this, right? This is still the same means. The yellows have a mean of 60 and the blues have a mean of 50. But now they're so interspersed that is harder to know-- it's possible, if you saw pictures like this, you would say, well, yes, the yellows are higher, but maybe this was just due to random chance, OK?
So what the purpose of these graphs are, is to show you is that in order-- so in both cases, we the same difference in the mean outcomes. It was 60 versus 50 in all three cases, right? But when you saw this graph, it was quite clear that these two groups were really different. When you saw this graph, is was much harder to figure out if these two were really different or if this was just due to random chance, OK? Does that make sense of where we're going?
And so, just to come back to the same theme, all the statistics are going to do in our case is going to help us figure out, are these differences big enough, given the distribution of data we have, how likely is it that the difference we observed could have happened by random chance. And so intuitively, we can look at this one and say, definitely different. And this one, maybe not sure. But if we want to be a little more precise about that, that's where we need the added statistics.
AUDIENCE: Is the sample size the same in both examples?
PROFESSOR: Yeah, the sample size is the same. Yeah, sample size is exactly the same. So you can see that the numbers go down because it's more spread out. All right.
So in some sense, what are the ingredients that we've talked about in terms of thinking about whether you have a statistically significant difference? If you think back to the gambler example, we talked about the sample size matters, right? So if we saw 1,000 tosses, we had much more precision about our estimates than if we had 10 tosses or five tosses. The hypothesis you're testing matters, right? Because the smaller an effect size we're trying to detect, the more tosses we need in the gambler example. If you're trying to detect a really small difference, you need a ton of data, whereas if you're trying to detect really extreme differences, you can do it with less data, OK?
And the third thing we saw is the variability of the outcome matters, right? So the more noisy the outcome is, the harder it is to know whether the differences that we observe are due just to random chance or if they're really due some difference in the treatment versus the control group. OK, so does this makes sense? Before I go on, these are the three ingredients that we're going to be playing with. Do these make sense? Do you have questions on this? OK.
So you may have heard of a confidence interval. How many of you guys have heard of a confidence interval? OK. How many of you can state the definition of a confidence interval? Thanks, Dan. I'm glad that you can. So what do we mean when we say confidence interval? What we mean by a confidence interval-- so let's just go through what's on the slide and then we can talk about it a little more.
So we're going to measure, say, 100 people and we're going to come up with an average length of 53 centimeters. So we want to be able to say something about how precise our estimate is. So we say the average is 53 centimeters. How confident are we or how precise are we that it's 53%? And that's what a conference interval is trying to say.
And a confidence interval, essentially, tells us that with 95% probability-- so we have a confidence interval of 50-56 says that with 95% probability, the true average length lies between 50 and 56. And so the precise definition is that if you had a hypothesis that the true average length was in this range with-- no, I'm going to get it wrong. It says that if you had a hypothesis that the true average was in here, it's within 95% probability that you would get the data that you observe, OK?
A converse way of saying it is that the truth is somewhere in this range, right? You can be 95% certain that the truth is somewhere within this range, So if you did 20 of these tests, only one out of 20 times would the truth be outside your confidence interval, OK?
And so an approximate interpretation of a confidence interval is-- so we know that the point estimate of 43, we have some uncertainty about that estimate. We think the average is 53, but there's some uncertainty. And the confidence interval says, well, it's 95% likely that the true answer is between 50 and 56, if that was the confidence interval, OK?
So why is that useful for us? Well, our goal is to figure out-- we don't care, actually, what our estimate of the program's effect is. We care what the true effect of a program is, right? So we did some intervention. Like, for example, we had a female Panchayat leader instead of a male Panchayat leader and we want to figure out what the actual difference that that intervention made is in the world. We're going to observe some sample of Panchayats and we'll look at the difference in that sample. And we want to know how much can we learn about the true program effect from what we estimated. And the confidence interval basically tells us that with 95% probability, the true program effect is somewhere in the confidence interval, OK? Does that makes sense?
How many of you guys have heard of the standard error? OK. So a standard error is related to the confidence interval in that a standard error says that if we have some estimate, you could imagine that if we did the experiment again, essentially, with a new sample of people that looked like the original sample of people, we might get a slightly different point estimate because it's a different sample. The standard error basically says, what's the distribution of those possible estimates that you could get, OK? So it says that basically, if I did this experiment again, maybe i wouldn't get 53, I'd get 54. If I did it again, maybe I'd get 52. If I did it again, I might get 53. The standard error is essentially the standard deviation of those possible estimates that you could get.
What that means in practice is that-- well, in practice, the standard error is very related to the confidence interval. And basically, a good rule of thumb is that a 95% confidence interval is about two standard errors. So if you ever see an estimate of the standard error, you can calculate the confidence interval, essentially, by going up or down two standard errors from the point estimate, OK?
And the confidence interval and standard error, essentially, are capturing the same thing. They're both capturing-- when I said we need statistics to basically compute, how likely is it that we would get these differences by random chance, those are all coming out in the standard error and the confidence interval, right? They're computed by both looking at how noisy our data is, which is the variability of the outcome, and how big our sample is, right? Because from these two things, you can basically calculate how uncertain your estimate would be. This is a lot of terminology very quickly, but does this all make sense? Any questions on this? OK.
So for example. So suppose we saw the sampled women Pradhans had 7.13 years of education and the men had 9.92 years of education, OK? And you want to know, is the truth that men have more education than women or is this just a random artifact of our sample? So suppose you calculated that the difference was 2.59. That's easy to calculate. And the standard error was 0.54 and the standard error was going to be calculated based on both how much data you had and how noisy the data was. You would compute that the 95% confidence interval is between 1.53 and 3.64, OK?
So this means that with 95% probability, the true difference in education rates between men and women is between 1.53 and 3.64. So if you were interested in testing the hypothesis that, in fact, men and women are the same in education, you could say that I can reject that hypothesis. With 95% probability, the true difference is between 1.53 and 3.64-- so zero is not in this confidence interval, right? So we can reject the hypothesis that there's no difference between these two groups. Does that makes sense?
So doing another example. So in this example, suppose that we saw that control children had an average test score of 2.45 and the treatment had an average test score of 2.5. So we saw a difference of 0.05 and the standard error was 0.26, OK? So in this case, you would say well, the 95% confidence interval is minus 0.55. This is approximately two. It's not exactly two. Minus 0.55-- oh, no, it is exactly two in this example. Minus 0.55 to 0.46, OK?
And here, you would say that if we were introducing the hypothesis that the null hypothesis is that the treatment had no effect on test scores, you could not reject that null hypothesis, right? Because an effect of zero is within the confidence interval, OK? So that's basically how we use confidence intervals. Yeah.
AUDIENCE: Shouldn't the two points of that confidence interval be equidistant from 2.59?
PROFESSOR: From 0.05 you mean? Yeah.
AUDIENCE: [INAUDIBLE]
PROFESSOR: Yeah, I think-- oh, over here?
AUDIENCE: Yeah.
PROFESSOR: So they actually don't always have-- so you raise a good point. So there may be some math errors here. I think a more reasonable estimate, by the way, is that this would have to be minus 0.05 for you to get something like this.
AUDIENCE: But in the first example, if 2.59 is the mean, is the difference--
PROFESSOR: So it's approximately the same, isn't it?
AUDIENCE: I think it's a little skewed--
PROFESSOR: Yeah.
AUDIENCE: On that side, it is 2.64.
PROFESSOR: OK. Yeah, so you raise a good point. So when I said that a rule of thumb is two times the standard error, that's a rule of thumb. And in particular cases, you can sometimes get asymmetric confidence intervals. So you're right that usually they should be symmetric and probably, for simplicity, we should have put up symmetric ones, but it can occur that confidence intervals are asymmetric.
For example, if you had a-- yeah, depending on the estimation, if you have truncation at zero-- if you know for sure that there can never be an outcome below zero, for example, then you can get asymmetric confidence intervals.
AUDIENCE: When the distribution is not normal?
PROFESSOR: Yeah. Exactly. But for most things that you'll be investigating, usually they're going to be--
AUDIENCE: Normal.
PROFESSOR: Yeah, for outcomes that are zero. One [UNINTELLIGIBLE] get non-normal, but yes, in general, they are pretty symmetric. But they might not be exactly symmetric. OK.
So as I sort of was suggesting as we were going through these examples, we're often interested in testing the hypothesis that the effect size is equal to zero, right? The classic hypothesis the you typically want to know is, did my program do anything, right? And so, how do you test the hypothesis that my program-- so we want to know, did my program have any effect at all? And so what we technically want to do is we want to test what's called the null hypothesis, that the program had an effect of nothing, against an alternative hypothesis that the program had some effect. So this is the typical test that we want to do.
Now you could say, actually, I don't care about zero. I want to say that I know-- for example, this is the standard thing that we would do in most policy evaluations that we're going to be doing. It doesn't have to be zero. Suppose you were doing a drug trial and you knew that the best existing treatment out there already have an effect of one. And so instead of comparing to zero, you might be comparing to one. Is it actually better than the best existing treatment? In most cases, we're usually comparing to zero, OK?
And usually, we have the alternative hypothesis that the effect is just not zero. We're interested in anything other than zero. Sometimes you can specify other alternative hypotheses, that the effect is always positive or always negative, but usually this is the classic case, which is we're saying, we think this thing had-- the null is no effect. We want to say, did this program have an effect and we're interested in any possible effect, OK? And hypothesis testing says, when can I reject this null hypothesis in favor of this alternative, OK?
And as we saw, essentially, the confidence interval is giving you a way to do that. It's saying, if the null is outside the confidence interval, then I can reject the null. Yeah.
AUDIENCE: Surely, if we're trying to assess the impact of an intervention, we're always going to think it's positive. Or in general, because-- I gave someone some money to increase their income or not. We've got a pretty good idea it's going to be positive. The probability it's negative is pretty--
PROFESSOR: Why do you--
AUDIENCE: Yeah, why do we change our significance level-- [INTERPOSING VOICES]
PROFESSOR: You ask a great question. And I have to say this is a bit of a source of frustration of mine. Let me give you a couple different answers to that. Here's the thing. If you did that-- if I said I can commit, before I look at the data, that I only think it could be positive, that would mean that if it's negative, no matter how negative, you're going to say that was random chance, OK? So it would require a fair amount of commitment on you, on your part, as the experimenter to say, if I get a negative result, no matter how crazy that negative result is, I'm going to say that's random chance, OK? And typically, what often happens ex post is that people can't commit to actually doing that.
So suppose you did your program and you-- so I actually have a program right now that I'm working on in Indonesia that's supposed to improve health and education. And it seems to be making education worse. Now, we have no theory for why this program should be making education worse, OK? But it certainly seems to be there in the data.
Now, if we had adopted your approach, we wouldn't be entertaining the hypothesis that it made education worse. We would say, even though it's looking like this program is making education worse, that must be random noise in the data. We're not going to treat that as something potentially real. Ex post, though, you see this in the data and you're likely to say, gee, man, that's a really negative effect. Maybe the program was doing something that I didn't think about. And in our case, actually, we're starting to investigate and maybe it's because it was health and education and we're sort of sucking resources away from education into health.
So it requires a lot of commitment on your part, as the researcher, that if you get these negative effects, to treat them as random noise. And I think that, because most researchers, even though they would like to say they're going to do that, if it happens that they get a really negative effect, they're going to want to say, gee, that looks like a negative effect. We're going to want to investigate that, take that seriously. Because most people do that ex post, the convention is that in most cases, to say we're going to test against either hypothesis in either direction.
AUDIENCE: Except that the approach--
PROFESSOR: Does that makes sense?
AUDIENCE: Your issue is do I do this program or not. So it doesn't matter whether the impact of the program is zero or negative. Even if it's zero, you're saying that it's--
PROFESSOR: You're absolutely right. So if you were strict about it and said, I'm going to do it if it's positive and not if it's zero, then I think you were correct that, strictly speaking, a one-sided hypothesis test will be correct and it would give you some more power.
AUDIENCE: So it would give you power.
PROFESSOR: Yeah, it would give you more power.
AUDIENCE: [UNINTELLIGIBLE]
PROFESSOR: Right. And the reason it gives you power is, remember, how does hypothesis testing work? It says, well, what is the chance this outcome could have occurred 95-- what would have occurred by chance 95% of the time? When you do a two-sided test, you say, OK-- where's my chalkboard? Here. You imagine a normal distribution of outcomes. You're going to say, well, the 95% is in the middle and anything in the tails is the stuff that I'm going to [UNINTELLIGIBLE] by non-random chance.
Well, what you're doing with a one-sided test is you're going to say, I'm going to take that negative stuff-- way out there negative stuff-- and I'm going to say that's also random chance. So I'm going to pick my 95% all the way to the left. And that means that the 5% that's not random chance is a little more to the right. Do you see what I'm saying? But it requires that if-- you're committing to, even if you get really negative outcomes, asserting that they're random chance, which is really, often, kind of unbelievable.
The other thing is that, although this is technically the way hypothesis testing is set up, the norms and conventions are that we all use two-sided tests for these reasons I talked about. And so I can just tell you that, practically speaking, I think if you do a one-sided test, people are going to be skeptical because it may be that you, actually, would do that, but I think most of the time, people can't commit to do that. And so the standard has become two-sided tests. But I certainly agree with you. It's very frustrating because one should be able to articulate one-sided hypotheses. That's sort of a long answer, but does that make sense?
It's OK. OK, now, for those of you on this side of the board, you won't be able to see, but maybe if I need to write something on the board it will be better. OK.
So now we're going to talk about type I and type II errors, which, as I mentioned, are not helpfully named. OK. A type I error-- so this is all about probability, so nothing we can ever say for sure. We can always say that this is more or less likely. And there's two different types of errors we can make when we're doing these probabilities or doing these assessments.
The first error, and it's called type I error, is we can conclude that there was an effect when, in fact, there was no effect, OK? So when I said the 95% confidence interval, that 95% is coming from our choice about type 1 errors. So for example-- a significance level is the probability that you're going to falsely conclude the program had an effect when, in fact, there was no effect, OK? And that's related to when you say a 95% confidence interval, the remaining 5% is what we're talking about here. That's the probability of making a type I error, OK?
And why is that? Well, we said there's a 95% chance that it's going to be within this range. That means that just by random chance, there's some chance it could be outside that range, right? So if your confidence interval was over here and zero was over here, you would say, well, with 95% conference, I'm going to assume the program had an effect because zero is not within my confidence interval. However, 5% of the time, the true effect could be over here outside your confidence interval. That's what a 95% confidence interval means. So in some sense, that's what we mean by a-- so that's in some sense what a type I error is. A type I error is the probability that you're going to detect an effect when, in fact, there's not.
And so the typical levels that you may see are 5%, 1% or 10% significance levels. And the way to think about those significance levels is, if you see something that's significant at the 10% level, that means it 10% of the time, an effect of that size could've been just due to random chance. Might not actually be a true effect. And if you've heard of a p-value, a p-value is exactly this number. A p-value basically says, what is the probability that an effect this size or larger could have occurred just by random chance, OK?
So that's what's called a type I error. And typically, there's no deep reason why 5% is the normal level of type I errors that we use, but it's kind of the convention. It's what everyone else uses. If you use something different, people are going to look at you a little funny. So the conventions are we have 5%, 10%, and 1%, as these significance levels. And you might say, gee, 5% or 10% seems pretty low. Maybe I would want a bigger one. But on the other hand, if you start thinking about it, that means that if you use 10% significance, that means that one out of every 10 studies is going to be wrong. Or if you had 10 different outcomes in your data set, one out of every 10 would be significant even just by random chance.
So the other type of error is what's called, as I said, helpfully, a type II error. And a type II error says that you fail to reject that the program had no effect when, in fact, there was an effect, OK? So this is, the program did something, but I can't pick it up in the data, OK? And we talk about the power of a test. The power is basically the opposite of a type II error. A power just says, what's the probability that I will be able to find an effect given that the actual effect is there, OK?
So when we talk about how big a sample size we need, what we're basically talking about is, how much power are we going to have to detect an effect? Or what's the probability that given that a true effect is there, we're going to pick it up in the data, OK? So here's an example of how to think about power. If I ran the experiment 100 times-- not 100 samples, but if I ran the whole thing 100 times-- what percentage of the time and in how many these cases would I be able to say, reject the hypothesis that men and women have the same education at the 5% level if, in fact, they're different, OK?
So this is a helpful graph which basically plots the truth and what you're going to conclude based on your data, OK? So suppose the truth is that you had no effect and you conclude your no effect, OK? Then you're happy. If there was an effect and you conclude there was an effect, you're happy. So you want to be in one of these two boxes. And the two types of errors you can make-- so one type of error is over here, right? So if the truth is there was no effect, but you concluded there was an effect, that would be making a type I error, OK? And this is what we talked about size. So this one, we normally fix this one at 5%. So it's only 5% of the time-- if there's no effect, 5% of the time you're going to end up here and 95% of the time you're going to end up here. That's what a 95% confidence interval is telling you.
And the other thing is, suppose that the thing had an effect but you couldn't find it in the data, OK? That's what's called a type II error. And that's, when we design our experiments, we want to make sure that our samples are sufficiently large that the probability you end up in this box is not too big, OK? So that's a sense of what we mean by the different types of mistakes or errors you could make. Yeah.
AUDIENCE: It's kind of a stupid question. So power is the probability that you are not making a type II error?
PROFESSOR: Yes.
AUDIENCE: So then power is the probability that you're in the smiley face box, that you are-- [INTERPOSING VOICES]
PROFESSOR: Yes. Power is the probability you're over here. Yeah, we say power is related to type II errors. Power is over here. This is the power. Power is conditional on there being an effect. What's the probability you're in this box, not this box? Probably should say one minus power to be clearer. OK? Does that makes sense? All right.
So when we're designing experiments, we typically fix this at conventional levels. And we choose our sample size so that we get this, the power, or the probability that you're in the happy face box over here to a reasonable level given the effect size that we think we're likely to get, OK? OK.
Now, in some sense, the next two things, standard errors, are about this box, size. And power is about this box, or these boxes. Yeah.
AUDIENCE: Why is power not also the probability that you end up in the bottom right box as opposed to the bottom left?
PROFESSOR: Because that's size.
AUDIENCE: Isn't size also linked to-- or power also linked to--
PROFESSOR: No, they're all related, but we typically-- they're related in the following way. We assert a size because when we calculate our standard error-- our confidence intervals, we pick how big or small we want the confidence intervals to be. When we say a 95% confidence interval, we're picking the size, OK? So this one, we get to choose.
AUDIENCE: So it's not sample size, it's size of the confidence interval?
PROFESSOR: No. Yeah, this is size is a-- yeah, it's the size of the confidence interval. That's right. Sorry, it's not the sample size. That's right. It's called the size of the test in yet more confusing terminology. That's right. This is the size of the confidence interval, essentially. And this one you pick, and this one is determined by your data. OK? All right.
OK, so now let's talk about this part, which is standard errors and significance. It's all kind of related. All right, so we're going to estimate the effect of our program. And we typically call that beta, or beta hat. So the convention is that things that are estimated, we put a little hat over them, OK? So beta hat is going to be our estimate of the program's effectiveness. This is our best guess as to the difference between these two groups.
So for example, this is the average treatment test score minus the average control test score. And then we're also going to calculate our estimate of the standard error of beta hat, right? And remember that the confidence interval is about two times the standard error. So the standard error is going to say how precise our estimate of beta hat is, which is, remember, if we ran the experiment 100 times, what will be the distributions of beta hats that we would get, OK?
And this depends on the sample size and the noise in the data, right? And remember we went through this already that here, in this case, the standard error of how confident we would be-- so the beta hat, in this case, is going to be 10, and in this case, it's also going to be 10, right? But here, these two things are really precisely estimated, so our standard error of beta hat is going to be very small because we're going to say we have a very precise estimate of the difference between them. And so the confidence interval is also going to be very small. And here, there's lots of noise in the data, so our estimate of the standard error is going to be larger.
So in both cases, beta hat is the same. It's 10 in both cases. But the standard error is very big here and very small here, OK? Now, when we calculate the statistical significance, we use something called a t-ratio. And the t-ratio-- it's actually often called the student's t-ratio, which I thought was because students used it. But it's actually named after Mr. Student. It's the ratio of beta hat to the standard error of beta hat, OK? And the reason that we happen to use this ratio is that, if there is no effect, if beta hat is actually zero, we know that this thing has a normal distribution, so we can calculate the probabilities that this thing is really or really small, OK?
So we calculate this ratio of beta hat over the standard error of beta hat. It turns out that if t is greater than, in absolute value-- sorry, if the absolute value of t, I should say, is greater than 1.96-- so essentially, if it's bigger than 2 or less than minus 2, we're going to reject the hypothesis of a quality at a 5% significance level. And why is that? It's because it turns out, from statistics, that if the truth is zero, OK? So if we're in the no effect box and the truth is zero, this ratio, it turns out, will have a normal distribution. And it just turns out from a normal distribution that the probability that the 5% confidence interval is 1.96 away from zero if you have a normal distribution. That's just a fact about normal distributions, OK?
So if we calculate this ratio and we say it's greater in absolute value than 1.96, we're going to reject the hypothesis of a quality at the 5% level, OK? So we can reject zero. Zero is going to be outside of our confidence interval. And if it's less than 1.96, we're going to fail to reject it because zero is going to be inside our confidence interval, OK? So in this case, for example, the difference was 2.59. The standard error was 0.54. The t-ratio is about seven. No, it's about five. So we're definitely going to be able to reject in this case. So we have a t-ratio of about five, OK? So you may see this terminology and this is where it's coming from.
Now, there's an important point to note here, which will come up later when we talk about power calculations, which is, in some sense, that the power that we have is determined by this ratio of the point estimate to our standard error. And so this says, for example, that if we kind of look at this a little more, that if you have bigger betas, you can still detect effects for a given standard-- so if you fix the standard error but you made beta bigger, you're more likely to conclude there was a difference, right? So what's going to increase your being able to conclude there was a difference? Either your effect size is bigger or your standard error is smaller, mechanically. OK.
So that's how we are going to calculate being in this box. So how do we think about power, which is the probability that we're in this box? We had an effect and we're able to detect that-- sorry, power's in this box-- that we had an effect, OK?
So when we're planning an experiment, we can do some calculations to help us figure out what that power is. What's the probability, if the truth is a certain level, that we're going to be able to pick it up in the data? And what do we need to do that? We're going to have to specify a null hypothesis, which is usually zero. We're going to be testing that something's different than zero, the two groups are the same, for example. We're going to have to pick our significance level, our size. And that, we almost always pick at 5%. We're going to have to pick an effect size. And we'll talk about what exactly this means in a couple more slides. But when we calculate a power, a power is for a given effect size, OK? And then we'll calculate the power.
So for example, suppose that we did this and a power was 80%. That would mean that if we did this experiment 100 times-- not 100 times, but actually repeated the whole experiment 100 times, 80% of the times we did this experiment, if the hypothesis is, in fact, false, and instead, the truth is this, we would be able to reject the null and conclude there was a true effect 80% of the time, OK?
That's a little bit complicated, but does that make sense, what we're going to be trying to do with power? So we're going to fix the effect size. So remember, we fix the bottom box. When we calculate power, we have to speculate not just effect versus no effect. We have to postulate just how effective the program is. So we're going to say, suppose that the effect size is 5%. The truth is 0.2, right? How big a sample would we need to be in this box 80% of the time, OK? So when we say power, that's what we mean.
And when we calculate the size of the experiments, you have to make a judgment call of how big a power do you want. The typical powers that we use when we do power calculations, are either 80% or 90%. So what does this mean? This means-- suppose you did 80%. Or [UNINTELLIGIBLE] this. If you did 80%, that would mean that if you ran your experiment 100 times and the true effect was 0.2 in this case, you would be able to pick up an effect, statistically 80 out of those 100 times. 20 out of 100 times, you wouldn't. And the bigger your sample size, the larger your power is going to be, OK? Does that make sense so far? OK.
Suppose you wanted to calculate what our power is going to be. What are the things you would need to know? You would need to know your significance level of your size. And as I said, this, we just assume, OK? This is that bottom box. We're just going to assume that it's 5%. And the lower it is, the larger sample you're going to need. But this one is sort of picked for you. We almost always use 5% because that's the convention. That's what everyone uses, essentially.
The second thing you need to know is the mean and the variance of the outcome in the comparison group. So you need to know-- so remember, all this power calculation is going to depend on whether your sample looks like this, really tight, or looks like this and is very noisy. Because you obviously need a much bigger sample here than here. So in order to do a power calculation, you need to know, well, just what does the outcome look like, right? Does the outcome really have very narrow variance? Is everyone almost exactly the same, in which case it's going to be very easy to detect effects? Or is there are huge range of people, in which case you're going to need bigger effects.
Now, how do we get this? So this one, we just conventionally set. This one, we have to get somewhere. And we usually have to get it from some other survey. So we have to find someone that collected data in a similar population. Or sometimes we'll go and collect data ourselves in that same population. Just a very small survey just to get a sense of what this variable looks like, OK? And if the variability is big, we're going to need a really big sample. And if the variability is really small, we're going to need a small sample. And it's really important to do this because you don't want to spend all your time and money running an experiment only to turn out that there was no hope of ever finding an effect because the power was too small, right? Yeah.
AUDIENCE: And this is in the entire population, not just the comparison group, right? It says-- --
PROFESSOR: Yeah, but before you do your treatment, the comparison and the treatment are the same.
AUDIENCE: They are the same.
PROFESSOR: Doesn't matter.
AUDIENCE: So it's a baseline population.
PROFESSOR: Baseline would be fine. Yeah. Before you do your treatment, they're the same. So it doesn't matter, OK?
And the first thing you need is, you need to make an assumption about what effect size you want to detect. And this one-- sometimes you also have to supply this. And the best way to think about what effect size you want to put in here is you want to say, what's the smallest effect that would prompt a policy response, OK?
So one could think about this, for example, by doing a cost-benefit calculation, right? You could say that we do a cost-benefit calculation. This thing costs $100. If we don't get an effective 0.1, it's just not worth $100, right? So that would be a good way of coming up with how big an effect size you want here.
And the idea, then, is if the effect is any smaller than this, it's just not interesting to distinguish it from zero, right? Suppose that the thing had a true effect of 0.001, right? But if it was that small of an effect, it could be completely cost effective. So say the thing happens at an effect of 0.001. Who cares, right?
So you want to be thinking about, from a policy perspective is, what's the smallest effect size you want to know, from a policy perspective, in order to set your power calculations? Yeah.
AUDIENCE: I have a question back at the mean and variance thing.
PROFESSOR: Oh, here. Yeah.
AUDIENCE: Yeah. So in terms of the baseline thing that you would collect-- so I'm on the implementation side of this, right? So we do projects. We collect baseline data. Now, the case that I'm thinking of, the baseline data that we would collect might not be exactly the same kind of data that we are looking for in terms of our study. What kind of base-- how--
PROFESSOR: Right, OK. So when we say baseline, there's two different things we mean by baseline. For this case, this is not strictly a baseline. This is just something about what's your variable going to look like. Let me come back to that in a sec. We also sometimes talk about baselines that we are going to use of actually collecting the actual outcome variable before we start the intervention, right? Those are also useful, and we'll talk about those in a couple slides. And those, one wants them to be more similar, probably, to the actual variable you're going to use.
Now, for your case, we often don't-- the accuracy of your power calculation depends pretty critically on how close this mean and variance are to what you're going to actually get in your data. And when you start in the example that you guys are going to work on or that maybe you've already started working on, you're going to find that it's actually pretty sensitive. Turns out it's pretty sensitive. So getting these wrong is going to mean your power calculation is going to be wrong. So that's sort of an argument for saying you want this to be as good as possible.
Now the flip side of that, though, is you're going to find that these power calculations are fairly sensitive to what effect size you choose as well. So you're going to find that if you go from a effect size of 0.2 to an effect size of 0.1, you're going to need four times the sample. That's just the way the math works out. By which I'm going to mean that I think that these power calculations are useful for making sure you're in the right ballpark, but not necessarily going to nail an exact number for you.
All that's by way of saying that you want to get-- because these things are so sensitive, you want to get as close as possible to what's actually going to be there. On the other hand you're going to find the results are also so sensitive to the effect size you want to detect that if this was a little bit off, that might be a tradeoff you would be willing to live with in practice.
AUDIENCE: So, from my--
PROFESSOR: Does that make sense?
AUDIENCE: Yeah, but it seems like the effect size-- your estimate of your effect size is this kind of-- we've got all this science for the calculation and yet your estimate of your effect size is based on--
PROFESSOR: You're absolutely right.
AUDIENCE: --getting that--
PROFESSOR: Hold on, though. Let me back up a little bit, though. You're right, except the-- in some sense, the best way to get estimates for your effect size is to look at similar programs, OK? So now there are lots of programs in education, for example. And they tend to find effect-- I've now seen a bazillion things that work on improving test scores. And I can tell you that they tend to get-- standardized effect size is the effect size divided by the standard deviation. And they tend to get effect sizes in the 0.1, 0.15, 0.2 range, right?
So you can look at those and say, well, I think that most other comparable interventions are getting 0.1, so I'm going to use 0.1 as my effect size. So you're right if you're just trying to sit here introspectful-- what my effect size is going to be, it's very hard. But if you use comparable studies to get a sense, then you can get a sense.
And the other thing I mentioned is, you can do cost-benefit analysis and say, well, look-- which is sort of another way of saying it, If there are other things out there which cost $100 per kid and get 0.1, then my thing, presumably, has got to do at least as well as 0.1 for $100-- suppose the other thing also costs $100 a kid, I've got to do at last as well as 0.1. Otherwise, I'd rather do this other thing. So it's another way of getting at the effect size.
AUDIENCE: Could you, then, also look at existing data in the literature for the mean and variance thing, or do you have to--
PROFESSOR: You could, but this one is going to be more sensitive to your population.
AUDIENCE: So it would just have to be very well-matched to be able to use it.
PROFESSOR: Right. I mean, look, if you don't have it, you could do it to get a sense, but this is one where the different populations are going to be very different in terms of their mean and variance. In order to get an estimate of this, you need a much, much, much smaller sample size than you need to get an estimate of the overall treatment effect of the program. So you can often do a small survey-- much, much, smaller than your big survey, but a small survey just to get a sense of what these things look like. And that can often be a very worthwhile thing to do.
AUDIENCE: I have a related question. How often do you see--
PROFESSOR: Oh, sorry. I just wanted to do one other thing on this. I've had this come up in my own experience, where I've done this small survey, and found that the baseline situation was such that the whole experiment didn't make any sense. And we just canceled the experiment. And it can be really useful. If you say, if I do this and my power is 0.01, for reasonable effect sizes, this is pointless. So it can be worth it. Sorry. Go ahead.
AUDIENCE: So to estimate the effect size, have you seen people run small pilots in different populations than they're eventually going to do their impact evaluation to get a sense of what effect size are they seeing with that same intervention?
PROFESSOR: Not usually, because you can't do a small pilot to get the effect size, right?
AUDIENCE: You're going to see something--
PROFESSOR: You've got to do the whole thing.
AUDIENCE: Yeah, yeah.
PROFESSOR: Right? That's the whole point of the power calculations is, in order to detect an effect of that size, you need to do the whole sample. So a small pilot won't really do it.
AUDIENCE: OK.
PROFESSOR: So it's not really going to-- you could get a-- no, I guess you really can't get a sense because you would need the whole experiment to detect the effect size.
AUDIENCE: Don't you think that there should be a lot more conversation about effect size before things start? Because if you've got a treatment, if you've got a program, and you can't have a very-- and you've struggled to have a good conversation about what is actually going to happen to the kids or what's going to happen to the health or what's going to happen to the income as a result of this, it really may be quite telling that you really don't know what you're doing. That there isn't enough of a theory behind your-- or practice or science or anything behind what your program is. If people are not pretty sure, what--
PROFESSOR: I mean, yes and no--
AUDIENCE: And then, also, on the resource allocation. Resource allocation, it just seems to me, most of the time, if your ultimate client is really probably the government, right? Because the government is the one that's going to make the big resource allocations--
PROFESSOR: It depends on who you're working with. It could be an NGO, whoever. But yes.
AUDIENCE: No, but an NGO is doing something, usually, as a demonstration that, in fact, if it works, then the government should do it.
PROFESSOR: Not always, but there's someone who, presumably, is going to scale up.
AUDIENCE: Right. And yes, businesses, maybe, right? But I would say, 90% of the time, it's going to be, ultimately, the government needs to--
PROFESSOR: Often, it's the government. In India, for example, there are NGOs who are-- I don't know who's worked on the Pratham reading thing. They're trying to teach-- NGOs trying to teach millions of kids to read, as an NGO. So sometimes NGOs scale up too. But anyway, you're right that there's an ultimate client who's interested in this.
AUDIENCE: So then, having a conversation very early on about--
PROFESSOR: Yeah. Could be very useful. That's absolutely right. That's absolutely right.
AUDIENCE: Because--
PROFESSOR: Now, in terms of your point about theory, though, yes and no. So I can design an experiment that's supposed to teach kids how to read. I know the theory says it should affect reading but I have no idea how much. And so--
AUDIENCE: Wouldn't you say that a significant percentage of the time, if it's a good theory about reading, it actually should tell you?
PROFESSOR: Not always. I mean--
AUDIENCE: Well, then I'd say it's not such a great theory, right? Wouldn't you--
PROFESSOR: It's a little bit semantic, but I think that a lot of times, I can-- say I'm going to teach kids to read a paragraph or whatever. But what percentage of the kids is it going to work for? What percentage of the kids are going to be affected? I think that using theory to calculate how-- I think theory can tell you a lot what variables should be affected. And that's what we talked about in the last lecture. I think theory can tell you what the sign of those effects likely to be. I think it's often putting a lot of demands on your theory to have them tell you the magnitude. And that's why you want to do the experiment.
AUDIENCE: And you just told me that even beyond the theory, you say, well, but we did this in one school and we saw it had this great thing, but you're saying-- [INTERPOSING VOICES]
PROFESSOR: But your confidence interval is going to be-- well, it's not nothing. It's going to tell you something, but your confidence interval is going to be enormous.
AUDIENCE: Right, nothing that you could rely on to set a good-- [INTERPOSING VOICES]
PROFESSOR: Right, it gives you a data point, but it's going to have a huge conference interval.
AUDIENCE: I don't want to belabor this, but if you think about it in business terms, right? I want to go out and raise some money.
PROFESSOR: Yes, absolutely. [INTERPOSING VOICES]
AUDIENCE: --something. And so, in order to raise that money, I have to tell you that, in fact, you're going to make this much money.
PROFESSOR: Right.
AUDIENCE: And, of course, it could turn out to be wrong. But I have to tell you you're going to get a 25% return on your money. And that means I have to explain to you why this business is going to successful, how many people are going to buy it, how I'm going to manage my costs down. So it's always curious to me that, when you're talking about social interventions, that I'm not having to make that same argument with that same level of specificity, which means I've talked about the effect size. Because I can't raise money if I tell you, look, I might only make you 5% or we might shoot the moon and make 100%. You'll say, thank you very much. This person doesn't know what their business is. I'm not going to give them my money.
PROFESSOR: Right. So you actually hit on exactly what's on the next slide. Which is exactly what I was going to say, which is, what you want to think about with your effect size is exactly this thing. What's the cost of this program versus the benefit it brings? And sometimes, what's the cost vis-a-vis alternative uses of the money, right? And that's going to be a conversation you're going to have with your client, which is going to say, if the effect size was 0.1, I would do it. And then you say, OK, I'm going to design an experiment to see if it's 0.1 or bigger, right? So I'm totally on board with that. Because, as I was saying, if the effect size is smaller than that, it still could be positive, but if your client doesn't care, if it's not worth the money at that level, then why do we need to design a big experiment to pick that up?
It's also worth noting this is not your expected effect size, right? I could expect this thing to have an effect of 0.2 but even if it was as low as 0.1, it would still be worth doing, OK? And in that case, I might want to design an experiment of 0.1, right?
Conversely, you guys can all imagine the opposite, which is you could say, I expect this thing to be 0.1, but maybe it's 0.2. Maybe it's actually-- I'm not sure how good it is. I think it's OK. But maybe it could be really great. And if it was really great, I would want to adopt it, so I would design an experiment to 0.2. So it's not the expected effect size, it's what you would use to adopt the program.
When we talk about effect sizes, we often talk about them-- we talk about what we call standardized effect size, OK? As I mentioned, how large an effect you can detect depends on how variable your sample is. So if everyone's the same, it's very easy to pick up effects. And we often talk about standardized effects are the effect size divided by the standard deviation of the outcome, OK?
So standard deviation of outcome is the measure of how variable your outcome is. So we often express our effect sizes relative to the standard deviation of the outcome, OK? And so when I was talking about test scores, for example, test scores are usually normalized to have a standard deviation of one. So this is actually how we normally express things in terms of test scores, but we could do it for anything.
And so effect sizes of 0.1, 0.2 are small. 0.4 are medium. 0.5 are large. Now what do we mean by that? This is actually a very helpful way of thinking about what a standardized effect size is telling you. So a standardized effect size of 0.2, which is what we were saying was a modest one, means that the average person in the treatment group, the median or the mean person of the treatment group, had a better outcome than 58% of the people in the control group.
So remember, if it was zero, it would be 50-50. It would be 50%, right? If there was no effect, the distributions would line up and this person's in the treatment group-- the median person in the treatment group would be better than 50% of the people in the control group. So this is saying, instead of lining up at exactly 50-50, it's lining up 58%-50%, OK? If you get an effect size of 0.5, which we were saying was a large effect, that means that 69% of the people in the treatment group are going to be bigger than the median person in the control group. Sorry, it's the other way around. The average member of the intervention group is better than 69% of people in the control group.
So the distributions are still overlapping. But now there's-- the middle of the treatment distribution is at the 69th percentile of the control. And a large effect of 0.8 would mean that the median person in the treatment group is at the 79th percentile of the control. That just gives you a sense of when we're talking about standardized effect sizes, how big we're talking about. And so you can see that 0.2, is actually-- you can imagine is going to be pretty hard to detect, right? If the median person in the treatment group looks like the 58th percentile of the control group, that's going to be a case where those distributions have a lot of overlap, right? And so this is going to be much harder to detect than this case when the overlap is much smaller. Yeah.
AUDIENCE: So in your experience, what do most people think their effect size is? Where do they settle? They probably wouldn't settle at 0.2?
PROFESSOR: Actually, a lot of people in a lot of educational interventions--
AUDIENCE: That's enough for them?
PROFESSOR: Yeah. I would say the typical intervention that people study that I've seen in education, the effect size is in the 0.15, 0.2 range. It turns out it's really hard to move test scores.
AUDIENCE: Yeah.
PROFESSOR: So yeah, I would say a lot of-- but you'll see when you do the power calculations, that to detect 0.2, you often need a pretty big sample. Look, it depends a lot on what your intervention is, but I've seen a lot in that range. And I'm just trying to think of an experiment I did. I can't think of it off hand. But yeah, I would say a lot in this range.
AUDIENCE: So would the converse be true, that in fact, you don't see too many that have a real large effect size?
PROFESSOR: I would say it's pretty rare that I see interventions that are 0.8. Yeah.
AUDIENCE: Do you think it's valuable that just because you're setting a low effect size in designing your experiment, you're being conservative. You can still pick up a [UNINTELLIGIBLE] effect size--
PROFESSOR: Of course.
AUDIENCE: It's just in the design process-- [INTERPOSING VOICES]
PROFESSOR: Right. This is the minimum thing you could pick up. That's absolutely right. That's right. So right, if you design for 0.2 but, in fact, your thing is amazing and does 0.8, well, there's no problem at all. You'll have a p-value of 0.00 something. You'll have a very strong [INAUDIBLE]. It's a good point. OK.
So how do we actually calculate our power? So there's actually a very nice software package, which, have you guys started using this yet? Yeah? OK.
AUDIENCE: I have a question. Can you just clarify something before you go on?
PROFESSOR: Yeah.
AUDIENCE: So by rejecting a null hypothesis, you won't be able to say what the expected effect is, so you won't be able to necessarily quantify the impact.
PROFESSOR: No, that's not quite right.
AUDIENCE: OK.
PROFESSOR: So you're going to estimate your-- you run your experiment, you're going to get a beta, which is your estimate, And you're going to get a standard error. You reject the null, which means you say with 95% probability, I'm in my confidence interval. So you know you're somewhere in the confidence interval.
And then beyond that, you have an estimate of where in the confidence interval you are. And your best estimate for where you are on the confidence interval is your point estimate. Does that make sense?
So in terms of thinking through the cost-benefit or whatever, your best guess of the effect of the program is your point estimate, is your beta. If you wanted to be a little more precise about it, you could say-- so this is your estimate, this is your beta hat, this is your confidence interval, right? Zero is over here, so you can reject zero in this case. But, in fact, there's a distribution of where your estimates are likely to be.
And when we said it was 95% confidence interval, that's because the probability of being over here is 95%. But this says you're most likely to be right here, but there's some probability over here. You're more likely to be near beta then you are to be very-- it's not that you're equally likely to be anywhere in your confidence interval. You're most likely to be right near your point estimate. So, in fact, if you actually cared about the range, you could say, well, what's the probability I'm over here? And calculate that. What's the probability I'm over here? And you could average them to calculate the average benefit of your program.
Usually, though, we don't bother to do this and usually what we do is we say our best estimate is that you're right at beta hat. That is our best estimate and we calculate our estimate based on that. But in theory, you could use the whole distribution [INAUDIBLE]. OK.
OK, so suppose we want-- so how do we actually calculate some of these? So using the software helps get a sense, intuitively, of what these tradeoffs are going to look like. And I don't know that I'll have time to go through all this, but we'll go through most of it, OK?
So for example, so if you run the software and look at power versus number of clusters-- hold on. So how would you set this up in the software? So we'll talk about clustered effects in a sec. As we discussed, you have to pick a significance level. You have to pick a standardized effect size. That's what delta is in the software.
So we use 0.2, OK? In the software, it's always a standardized effect size. You just divide by your standard deviation of your outcome. That's why you know your actual outcome variable because you know-- but I think the actual effect is whatever-- people get one centimeter longer in order to get a standardized effect size, I need to know the standard deviation of my outcome variable. And the program is going to give you the power as a function of your sample size, OK?
And one of the things that you can see is that this is not necessarily a linear relationship, right? So for example, here, we've plotted a delta of-- effect size of 0.2 and here's an effect size of 0.4. So this says that with about 200 clusters, you're going to get to a power of 0.8 with the effect size of 0.4, but you're still going to be at a power of 0.2 with an effect size of 0.2. So the formulas are complicated. They're not necessarily a linear function of your power.
When we think about power, we've talked about a couple of things that influence our power in terms of the variance of our outcome, right? The variance of our outcome, how big our effect size is. And those are the basic things that are going to affect our power. But there are things that we can do in our experiment-- in the way we design our experiment that are also going to make our experiment more or less powerful. And here are some of the things that we can do.
One thing that we can do is we can think about having a cluster-- so whether we whether randomize at the individual level or in clusters, whether we have a baseline, whether we use control variables or stratification, and the type hypothesis being tested. All four of these are things that we're going to do that for a given outcome variable and a given effect size, in some sense, are going to affect how powerful our experiment is. In some sense-- given that I may not have time to finish everything, the one that I want to focus on is the clustering issue. This is the one that is the biggest for designing experiments, and it often makes a big difference.
So the intuition for clustering is that-- so what is clustering? Clustering is, instead of randomizing-- suppose I want to do an experiment on whether the J-PAL executive ed class improves your ability to-- whether you took this lecture improves your understanding of power calculation, OK? Suppose I randomly sampled this half of the room and gave you my lecture and this half was the control group. And I flipped a coin so I split you in halves down the middle and I said, OK, I'm going to flip a coin, which is control, which is treatment.
You guys, presumably you all sat with your friends, OK? So people on this side of the room are going to be more like each other then people on that side of the room, OK? So I didn't get an independent sample, right? This group, their outcomes are going to be correlated because some of you are friends and have similar backgrounds and skills. And this group is going to be correlated.
On the other hand, suppose I had gone through everyone and randomly flipped a coin for every person and said, treatment or control, treatment or control, treatment or control? In that case, I would've flipped the coin 60 times and there would be no correlation between who is in the control group and who is in the treatment group because I wouldn't have been randomizing you into the same groups together, OK? By doing the cluster design, splitting you in half and then randomizing treatment versus control or splitting you into groups of 10-- you five, you 10, you 10. You 10, you 10, you 10, and then flipping the coin. I have less variation, in some sense, than if I had flipped the coin in individual-- person by person-- because those groups are going to be correlated. They're going to have similar outcomes.
So the basic point is that your power is going to be-- the more times you flip the coin to randomize treatment and control, essentially, the more power you're going to have because the more your different groups are going to be independent, OK?
So to go through this again, suppose you wanted to know-- this is, in general, about clustering. Suppose you wanted to know how the outcome of the national elections are going to be. So you could either randomly sample 50 people from the entire Indian population, or you randomly pick five families and you ask 10 people per family what their opinions are. Clearly, this is going to give you more information than this is because those family members are going to be correlated, right? I have views like my wife and like my father, et cetera. So we're not getting independent views, whereas here, you're getting, really, 50 independent data points. And that's the same as what we were talking about with the class. So this approach is going to have more power than this approach because of the way you did the sample. Yeah.
AUDIENCE: So is the only reason that you would cluster, then, just because you had to because you had no choice--
PROFESSOR: Yes.
AUDIENCE: --for political reasons or just feasibility.
PROFESSOR: And cost.
AUDIENCE: And cost.
PROFESSOR: Yeah.
AUDIENCE: Well, and the level of intervention.
PROFESSOR: Exactly. And we'll talk about that. There are lots of reasons people-- given this issue, people have lots of good reasons for clustering, but the point is that there are negative tradeoffs for sample size.
AUDIENCE: About the clusters. If you flip the coin for all of the class and then after, you decide that you will select among the people that you have assigned, you will select those seated-- you will select half of those seated on the left. Will that solve the problem--
PROFESSOR: You select half of the ones seated on the left?
AUDIENCE: Yeah.
PROFESSOR: Well, it's a different issue. Suppose I first select the left and now I go one by one, flip a coin of the people on the left. I don't have the clustering issue because I flipped the coin per person. But I have a different issue, which is that the people I selected are not necessarily representative of the whole population because I didn't pick a representative sample. I picked the ones who happened to sit over here.
AUDIENCE: My question--
PROFESSOR: So there's two different issues. One is, essentially, how many times you flip a coin is how much power you have, how independent you're thing is. The other issue is, is this group here representative of the entire population? You might think that people who sit near the window like to look at the river are daydreamers and they're not as good at math as people who don't sit near the window. And so I would get the effect of my treatment on people who like to sit near the window and aren't as good at math. And that might be a different treatment effect than if I had done it over the whole room. So it's a different issue.
AUDIENCE: Yeah, but my question was you first draw a random number of people that you assign to the treatment or to the control. And after that, within that people, you now say, I will take half of those people-- I will take half that are seated on the left and half that are seated on the right.
PROFESSOR: I'm not sure-- let me come back to your question. I'm not sure I fully understand what you're saying. Maybe we can talk about it afterwards. I think what you're saying may be about stratification. Why don't we talk about it later? Because we're running a little short on time. In fact, can I borrow somone's handouts? Because I want to make sure I cover the most important stuff in the lecture. Let me just see where we are. OK.
AUDIENCE: And if you need to, you can take ten extra minutes.
PROFESSOR: I may do that. I was going to ask you, Mark, for permission. I just wanted to see what I had left. OK. So where were we? Right. OK. Right.
So as I was saying, when possible, it's better to run clustered design. And so a cluster randomized trial is one in which the units that are randomized are clusters of units rather than the individual units. So I randomized a whole cluster at a time rather than individual person by person. And there are lots of common examples of this. So the PROGRESA program, for example, in Mexico was a conditional cash transfer program. They randomized village. Some villages were in, some villages were out. If a village was in, everybody was in. In the panchayat case we talked about, it was basically a village. It was a panchayat. So the whole panchayat was in or the whole panchayat was not in.
In a lot of education experiments, we randomize at the level of a school. Either the whole school is in or the whole school is out. Sometimes you do it as a class. A whole class in a school is in [UNINTELLIGIBLE] is out. In this iron supplementation example, it was by the family. So there's lots of cases where you would do this kind of clustering.
And there are lots of good reasons, as I've mentioned, for doing clustering. So one reason is you're worried about contamination, right? So for example, when they're interested in deworming, worms are very easily-- there's a lot of cross-contamination. If one kid has worms, the next kid who's also in school with him is likely to get worms. So if I just deworm half the kids in the school, that's going to have very little effect because my control- they're going to get recontaminated by the kids who weren't dewormed, right?
Or it could be the other way around. It could be that if I deworm half the kids, that's enough to knock worms out of the population. The control group is also affected. So you need to choose a level of randomization where your treatment is going to affect the treatment group and not affect the control group. So that's a very important reason for cluster randomizing.
Another reason is this feasibility consideration. So it's often just for a variety of reasons not feasible to give some people the treatment and not others. Sometimes within a village, it's hard to make some people eligible for a program and others not. It's just sometimes hard to treat people in the same place differently. And so that's often a reason why we do cluster randomization. And some experiments naturally just occur at a cluster level.
So for example, if I want to do something that affects an entire classroom, like give out-- suppose I want to train a teacher, right? That obviously affects all the kids in the teacher's class. There's no way to have that only affect half the kids in the teacher's class. It's just a fact of life. So there are lots of good reasons why we do cluster randomized designs even though they have negative impacts on our power.
So as I mentioned, the reason the cluster has a negative impact on your power is because the groups are correlated. The outcomes for the individuals are correlated. So, for example, if all of the villagers are exposed to the same weather, right? All villagers are exposed to the same weather. So it could be that the weather was really bad in this village. So all those people are going to have a lower outcome, for example, than if the weather was good. And so, in some sense, even if there are 1,000 people in that village, they all got this common shock, which is the negative weather, you don't actually have 1,000 independent observations in that village because they have this common correlated component, OK?
And this common correlated component we denote by the Greek letter rho, which is the correlation of the units within the same cluster. So rho measures the correlation between units in the same cluster. If rho is zero, then people in the same cluster are just as if they were independent. There's no correlation. Just as if they had been not in the same cluster. If rho is one, they're perfectly correlated and it means it they all have exactly the same outcome, OK? So it's somewhere between zero and one. And the lower the rho is, the better you are if you're doing a cluster randomized design.
And why is that? It's because the problem within a clustered randomized design is, as I was saying, if people were all exposed to the same weather, it's not as if you had 1,000 independent people in that village. You effectively had fewer than 1,000 because they were correlated. And rho captures that effect-- how much smaller, effectively, is your sample, OK? And the bigger rho is, the smaller your effective sample size is, OK?
And once again, when you do the power calculations, you can play with this and you'll note that small differences in rho make very big differences in your power. And I'll show you the formula in a sec. So often it's low, but it can be substantial. So in some of these test score cases, for example, it's between 0.2 and 0.6, which, 0.6 means that most of the differences are coming between groups, not within groups. So the groups, really, are much closer to one object. Yeah.
AUDIENCE: What does the 0.5 mean? Are you saying that in Madagascar, the scores on math and language--
PROFESSOR: It's the correlation coefficient, which is the-- technically, I believe it's the between variation divided by the total variation. I think that's the formula. Dan's shaking his head. Good. Excellent. A for me. It's what share of the variation is coming between groups divided by the total share of variation. So 0.5 means that, in some sense, half of the variation in your sample is coming between groups.
AUDIENCE: Okay.
PROFESSOR: What?
AUDIENCE: Isn't it within [INAUDIBLE]? If a rho is--
PROFESSOR: No, it's between. Because if rho is one, then each group is one. Yeah, it's between. If it was zero, then they're independent and it's saying that it's all coming from within. Yeah.
AUDIENCE: But here it's between math and language scores of one kid or between math plus language scores of two kids in the same group.
AUDIENCE: Or is it math and language scores in Madagascar are explained by--
PROFESSOR: This says the following. This was in Madagascar, they sampled math and language schools by-- they took math and language scores for each kid by classroom-- or by school. I think it was by school in this particular case. Then they said, looking over the whole sample that they looked at in Madagascar, what percentage of the variation in test scores came between schools relative to within schools. And they're saying that half of the variation was between schools. OK.
So how much does this hurt us, essentially? So we need to adjust our standard errors, given the fact that these things are correlated. And this is the formula, which is that for a given total sample size, if we have clusters of size m-- so say we have 100 kids per school-- and intercorrelation coefficient should be a rho, the size of the smallest effect we can detect increases by this formula compared to a non-clustered design. So this shows you what this looks like, OK?
So suppose you had 100 kids per school, OK? Suppose you had 100 kids per school and you randomized at the school level rather the individual level. If your correlation coefficient was zero, it would be the same as if we randomized at the individual level because they're totally uncorrelated. Suppose your correlation coefficient was 0.1-- rho was 0.1. Then the smallest effect size you could have would be 3.3 times larger than if you had done an individual design. So does that make sense how to interpret this?
And so this illustrates that, even with very mild correlation coefficients-- and we saw examples of those math test scores that were like 0.5. This is only 0.1, but it already means, in some sense, that your experiment can detect things-- if you had been able to individually randomize, you would be able to detect things that were three times as small, right? Now that's a combination of the fact that you have the correlation coefficient and the number of people per cluster.
AUDIENCE: Then in the previous slide, 0.5 does not mean half?
PROFESSOR: No, 0.5 is the correlation-- it's rho.
AUDIENCE: No, in the
PROFESSOR: It's rho.
AUDIENCE: Then it does not mean half of the difference--
PROFESSOR: No, it's half of the variance. Let me move on. We can talk about the formula for that. OK.
So what this means is, if the experimental design is clustered, we now not only need to consider all the other factors we talked about before, we also need to consider this factor rho when doing our power calculations. And rho is yet another thing we can try to estimate based on our little survey of our population to get a sense of what this rho is likely to be. And given this clustering issue, it's very important not just that you have a big enough number of people involved in your experiment, but that you randomize across a big enough number of groups, right?
And the way I like to think about is, how many times did you flip the coin as to who should be treatment and who should be control? And, in fact, it's usually the case that the number of groups you have is often more important than the total number of individuals that you have because the individuals are correlated within a group, OK? So moving on. So I'm going to flip through this. This is mostly going over some of this if you were doing the exercise quickly. OK.
And so this chart-- in your exercise shows you some of the tradeoffs that you should think about when you're trying to decide how you should trade off the number of groups you have versus the number of people within a group, OK? So in this particular case, a group was a gram panchayat, and within a group there were villages, OK? And there were different costs involved in doing these different things, right?
So going to the place involved transportation costs to get to the gram panchayat. That, say, was a couple of days. And then it took, like, half a day, say, for every village you interviewed. So that said, there's some cost of adding a new gram panchayat, but also some marginal cost of adding additional village per gram panchayat, OK? So you could calculate, based on all your parameters and power of 80% and whatever the intercluster correlation is in this particular case, you could say, well, if we had this many villages per gram panchayat, how many gram panchayats would we need and how many villages would we need, OK?
So you can do this set of exercises and you can say that-- and you'll note, for example, that as we reduce the number of gram panchayats we go to-- another way, as we add more villages per gram panchayat, the total number of villages we need to survey goes up. And in this particular case, it doesn't go up by that much because the intercluster correlation is not that high. And you could actually do this type of calculation and you could say, well I know my costs are, right? I know what my costs of going this place are. And I can calculate which of these designs is the cheapest design given what I want to achieve.
The other thing is, in this case, the experiment was happening everywhere and they were just trying to design the survey. But often when we're doing this, we also need to pay for the intervention itself. And, at least in a lot of the cases that I've worked with, the cost of actually doing the intervention is much bigger than the cost of doing the survey. And so, in that case, if you always have to treat every village in the gram panchayat, you can actually save a ton of money by going down in the number of gram panchayats and surveying a lot more villages.
But the whole point is there are these tradeoffs and you need to, in deciding how you're going to structure your experiment and how you're going to structure your survey, you need to think through what these tradeoffs are, make sure you have enough power, given your estimates of your intercluster correlation and sort of do the cost minimizing thing.
OK, so in the last five minutes or so, let me just highlight a couple of the other issues that come up in thinking about power calculations. So as I mentioned, the cluster design is one of the most important ones. And the key thing is making sure you have enough independent groups, where you flip the coin to randomize betwee treatment and control enough times. Some other things that matter are baselines, control variables, and the hypothesis being tested. So one minute on each of those.
A baseline has two uses-- main uses. One use of a baseline is that it lets you check whether the treatment and control group look the same before you started. And if you randomized properly, we know they should look similar. But you want to make sure that your randomization was actually carried out the way it was supposed to be and that it wasn't the case that people were pulling out of the hat until they got a treatment or something, that they were actually randomizing the way they were supposed to. And having a baseline conducted before you start can allow you to test that your randomization is actually truly random and your groups look balanced.
The other thing is, the baseline can actually help reduce your survey size needed because, but it requires you to a survey before you start the intervention, right? And the reason it can reduce your sample size is that now, instead of just looking at, say, test scores across kids, I can look at the change in test scores from before versus after the experiment started. And if people are really persistent, like if the people who did really well on the test this year are likely to do really well on the test next year, that can essentially reduce the variance of your outcome. It can be that the variance of difference in test scores can be a lot lower than the variance in test scores. And having a baseline can help you for that reason.
And as this slide points out, your evaluation costs basically double because you have to do two surveys, not one survey, but the costs of the intervention go down because you can have a slightly smaller sample. So if your intervention is really expensive relative to your survey, this can make a lot of sense. If your survey is really expensive relative to your intervention, you might not want to do this.
And to figure out how this is going to affect your power, you need to know yet another fact, which is how correlated are people's outcomes over time, right? What's the correlation between how well I do on a test today and how well I do on a test tomorrow? And some things are really correlated and some things are not that correlated. And a baseline really helps you on things that are really correlated.
Another thing that can help you is stratification. So what stratification can do is, stratification says, suppose I-- in some ways, it's conceptually a little bit like a baseline, which is, suppose I know that all of the people who live in this village tend to have similar outcomes and all of the people who live in this village tend to have similar outcomes. And all of the people who live in this village tend to have similar outcomes. If I can then randomize by village, I can compare the people in each village to each other, OK? So if I'm looking within village, if people in villages tend to be similar and I can randomize within village, if I look within villages, the difference between the treatment and the control group is going to be less noisy.
So stratifying is basically a way of saying I'm going to make sure my sample is balanced across the treatment and control groups within certain subgroups of the population. And then I'm going to compare within those subgroups of the population when I do my analysis. And once again, we can think this as a way of reducing noise. That if people in similar villages tend to be similar, if I only compare treatmet and control within the same village, the noise there is going to be smaller. So in some sense, it's similar.
So some things we tend to stratify by, if we know the baseline value of the outcome, we can sometimes stratify by that because we know that the effects are going to be similar for people who have very similar baseline values. Or often, I think, geographically we often tend to do that. So basically, we think that people in certain areas tend to be similar so we're going to make sure our treatments and controls are balanced in those areas as a way of reducing noise.
And the final thing we want to mention is the hypothesis being tested, which is, the more things you want to test, the bigger your sample is going to need to be. So for example, are we interested in the difference between two treatments as well as the treatment versus control? If so, we need a much bigger sample because we not only need to be able to tell the treatment versus the control but we also need to be able to tell the two treatments from each other, right?
So suppose you have two different treatments. Are you interested in just the overall effect of each of the two treatments? Or are you interested in whether the treatments interact produces a different effect if they happen together, right? The more things you're interested in, the bigger your sample needs to be because you need to design your sample to be big enough to answer each of these different questions.
Another thing, for example, you were interested in testing whether the effect is different in different subpopulations. Do you just want to know the average effect of your program or do you want to know if it was different in rural areas versus urban areas? If you want to know if it's different in rural versus urban areas, you're going to need a big enough sample in rural areas and a big enough sample in urban areas that you can compare the difference between them.
So the more different things that you want to test, obviously, the bigger your experiment's going to need to be. And a lot of times, in actually designing the experiment, this is something that comes up all the time, that you will very quickly figure out that the number of questions you would like to answer is far bigger than the sample size you can afford. And one of the really important conversations you need to have as you're starting to design an experiment are, which are the really critical questions that I really need to know the answer to?
So for example, in a project I was recently doing in Indonesia, it turned out that the government really wanted to know whether this program would work differently in urban versus rural areas because they had a view that urban areas are really different. And they were willing to do different programs in urban versus rural areas. So we designed our whole sample to make sure we had enough sampled in urban areas and in rural areas that we could test those two things apart. That almost doubled the size of the experiment, but the government thought that was important enough that they really wanted to do that.
The point here is that-- that was the one they wanted to focus on. There was a million other things we could have done instead. And so it's really important to think about, before you design the experiment, what the few key things you want to test are because, as I said, net you're never going to have enough money to test all things you want. That's sort of a universal truth.
So just to conclude, we've talk about in this lecture-- going back to the basic statistics of how you're going to analyze the experiment, thinking about how noisy your outcome is going to be and how you're going to compute your confidence intervals, how big your effect size is going to be. That's all what goes into doing a power calculation. You also need to do some guess work, right? The power calculation is going to require you to estimate how big your sample is going to be-- sorry, how much variance there's going to be, what your effect size is going to be. You have to make some assumptions. And a little bit of pilot testing before the experiment begins can be really useful, I think, mostly for thinking about-- just collecting some data can be useful to estimate these variances.
The power calculations can help you think about this question of how many treatments you can afford to have, and can I afford to do three different versions of the program or do I really need to just pick one or two? How do I make this tradeoff of more clusters versus more observations per cluster? The power calculation can be very helpful here.
And the other thing, and in some sense, the place I find power calculations the most useful, especially because there is a bit of guesswork in power calculations and you get rough rules of thumb. You don't get precise answers because it depends on the assumptions. But what I find this is really useful for is whether this is feasible or not, right? Is this something where I'm kind of in the right range where I think I can get estimates, or where there's no chance, no matter how successful this program is, that I'm going to be able to pick it up in my data because the variable is just way too noisy.
And it's really important that you do the power calculation, particularly-- both for structuring how to design the experiment, but particularly to make sure you're not going to waste a lot of time and money doing something where you're going to have no hope of picking it up. Because a study which is underpowered is going to waste a lot of everyone's time and you're very frustrated. Very frustrating for everyone involved. So you want to make sure you do this right before you start because otherwise, you're going to end up spending a lot of time, money, and effort on an experiment and ending up not being able to conclude much of anything. OK. Thanks very much.
Free Downloads
Video
- iTunes U (MP4 - 225MB)
- Internet Archive (MP4 - 448MB)
Subtitle
- English - US (SRT)