Flash and JavaScript are required for this feature.
Download the video from iTunes U or the Internet Archive.
Description: This lecture by Prof. Jeff Gore is about the mechanisms of biological pattern formation. One mechanism discussed is diffusion.
Instructor: Prof. Jeff Gore
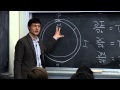
Robustness in Development a...
The following content is provided under a Creative Commons license. Your support will help MIT OpenCourseWare continue to offer high quality educational resources for free. To make a donation or view additional materials from hundreds of MIT courses, visit MIT OpenCourseWare at ocw.mit.edu
PROFESSOR: Today what we're going to do is we're going to talk about some of the big ideas in class. Today what we're going to do is talk about pattern formation in biology, some of the different mechanisms that are employed in development and other contexts in order for organisms to figure out where to put things.
Now before I get into that too much, I wanted to make couple administrative type announcements. So first, we have graded the exams and we'll hand them back at the end of class today. If I try to forget then please somebody remind me.
What we're going to do is we're going to start by just talking about diffusion a bit more. I think that there's a fair range of different maybe experiences thinking about diffusion and what it can do for you. So we'll work on our intuition a little bit. And then I'll say something about the reading that you did from [INAUDIBLE] book about robust mechanisms for pattern formation in the context of development.
So simple diffusion with degradation leads to these exponential profiles that are not robust to changes in the concentration or production rate of the morphogen. Whereas if you have self enhanced degradation then this leads to power law fall off it's more robust to the concentration of this morphogen.
Then what we're going to do is transition to these turning patterns, reaction to fusion systems where you can have a really surprising effect, whereby in a well mixed situation these chemicals or proteins or reactants, they might just reach a stable state.
However, once you add diffusion, then you can start getting pattern formation, which is a very funny thing because diffusion is normally something that's smooths out profiles. Normally we think about diffusion as being something that removes patterns and indeed that's generally what happens.
But following from this class of work from Alan Turing at the end of his life, he showed that it's actually in principle possible to have the emergence of patterns from diffusion. And we'll talk about some of the ways that could possibly happen.
So Turing patterns are already a surprising case where diffusion leads to patterns. There's another interesting phenomenon where if you add another source of noise that-- again, you think noise, demographic noise, the random creation destruction of individuals or chemicals or proteins.
Normally, we think this should also kind of be a force for removing patterns. But there's been a number of pieces of work over the last decade showing that in some cases, demographic noise can actually lead to patterns either in space or in time. We're going to talk more about this in the context of these so-called noise induced predator-prey oscillations.
But since we're talking about patterns here I will tell you something about how such noise can enhance the formations of patterns in the context of the Turing mechanism. And then we'll end by talking some about recent work of how E. coli find the center of their cell when they want to divide.
So you can imagine this is not an obvious thing to figure out how you might do. And we'll talk about the so-called Min system that E. coli use to find the center of the cells so they know where to septate, where to cut off and make two cells.
So I just want to start by making sure that when we talk about diffusion, we're all talking about the same thing. Now, hopefully for those of you that have not been thinking about diffusion so much recently, you did read the notes that Alexander Van Oudenaarden put together for Systems Biology a few years ago. I think it's useful, but in addition to the math, as maybe many of you know I'm a huge fan of graphical representations of ideas.
So I just want to make sure that we all agree on some of the basic ideas of how, for example, we get flux in the context of diffusion. So imagine that we have a one dimension system where there's some chemical with concentration c. We can imagine, for example, it starts out looking like this. This is a concentration profile as a function of position x where we have maybe a box of length l. Concentration of some chemicals of function of position x in some space.
Now I just want to make sure that we're all thinking about the same thing. So it starts out some concentration of c1, over might be some c2. Linearly here. I just want to make sure we all agree on the magnitude of the flux at some different points.
So, in particular, we can think about A, B, this is capital C. So where is the magnitude of the flux largest? A, B, C. D corresponds to them being the same. E as don't know. Are there any questions about the question? Yes.
AUDIENCE: On the y-axis, you're plotting concentration?
PROFESSOR: Yes, this y-axis is the concentration is the function of position x. All right, I'll give another five seconds to think about it. All right, do you need more time? Let's vote. Ready three, two, one. OK, great. So I'd say a vast majority here are agreeing that it's going to D. The flux is going to the same everywhere.
Now the flux-- can somebody remind us? We'll say the flux of J. And what is it going to be? So there's a minus D times the change in c with respect to x. This was derived in the notes.
So this is highlighting that what leads to a flux is the change in a concentration with respect to position. And so it doesn't matter that the concentration is higher here than here, we have the same flux. And the flux is in which direction? Left, right, up, down? Three, two, one. Left, so the flux is here.
Minus sign-- like always, it's hard to remember from equations whether they're plus minus signs but you should be able to just remember you can draw something like this and say OK, this is a positive DCDX, so flux is going to be in the negative direction, right?
Now, what will be the change in the concentration with respect to time at this point right now? So this is change in the conservation at point b with respect to time. Is it greater than 0, equal to 0, less than 0, or can't determine? Does everybody understand the question I'm trying to ask?
Change in the concentration at this point with respect to time, at this time that's wrong. I'll give you 5 seconds. Ready? Three, two, one. OK. And so this, again, it's pretty good but not everyone. So it is going to be b. Can somebody explain why it's b here? Yes.
AUDIENCE: There's as much flux coming in as coming out on the other side?
PROFESSOR: That's right. So it's true that there's a net flux here coming to the left. But from the sample, if we want to know whether there's a change in the concentration at that point, we need to know what's the net number of particles moving to the left verses the net particle number there that are coming in from the right.
Now of course, on this left face-- if I draw a little box here-- are all the particles crossing this position? Are they all moving to the left? No. So the idea is that all the particle motion is random.
And indeed, there's only a slight access of particles moving to the left as compared to the right. In the sense that, if you look at the concentration there, the concentration is rather large, it's only a little bit larger to right than to the left of this plane. Which means that there's only a slight excess of particles defusing to the left as coming to the right, but that leads to a net flux of particles crossing this plane to the left.
So where will the concentration be changing? At this point here will the concentration be changing with respect to time? Ready three, two, one. No. So well does it change at all ever anywhere in this example? Is this a steady state profile, yes or no? Ready three, two, one.
AUDIENCE: No.
PROFESSOR: No. What should be the steady state profile?
AUDIENCE: Flat.
PROFESSOR: Flat, OK. So how does that come about if the concentration is not changing any? Yeah.
AUDIENCE: It'll only change at the edges.
PROFESSOR: It's only changing the edges initially. Now, it's important to note that it's not that the concentration profile is not going to start looking like this. So that will not actually be how it-- eventually it's going to be flat. But it's going to smooth out on the edges.
Do you guys understand what I'm trying to say? It's not that it's a line that kind of goes like this, but instead we do end up getting curvature. One more of these, and then we'll consider ourselves to be expert diffusionists.
All right, so let's say the concentration as a function of position looks something like this. What I want to know is, where is DCDT maximal? We have some different points. We have A, B, C, D. Five seconds. Ready three, two, one.
All right, so we finally got a lot of disagreement. I like it. Turn to your neighbor, you should be able to find somebody that disagrees with you. What is it that determines?
AUDIENCE: [ALL DISCUSSING]
PROFESSOR: All right, did you guys all agree? You all agree on it? OK let's go ahead and reconvene. And let me see, maybe they were forming the domains of some sort. All right, ready. Wait, he says, no, no. All right, OK, one question.
AUDIENCE: Is this instantaneous in some sense?
PROFESSOR: Yeah.
AUDIENCE: Exactly at the inset.
PROFESSOR: All right, so I guess what I would say is that this is concentration profile at some time as a function of position in time. If you want to know the concentration profile some time delta t later, right? t plus delta t. Well then, it's going to be the concentration we had before then a little bit. Plus delta t times the derivative with respect to time.
Right, so this is the concentrate file at this time. And I want to how much is it going to change in the next delta t? And I'm assuming, of course, that I'm not adding any particles or taking any particles away. So just do the diffusion.
All right, let's see where we are. Ready three, two, one. OK. All right so it's we got domain, definitely some domains. All right. Well, it's going to end up being A, as I think the majority of the group is now saying. And why is it a?
AUDIENCE: Because of the second derivative.
PROFESSOR: Second derivative, right? So just what we said before is that, if we wanted to know the change of concentration with respect of time, at this point that was zero. Because the flux particle was leading that point and the flux coming in were the same. And we can also think about the second derivative.
So this indeed just from fix, like I said, was first law/ we got a D, so this gives second derivative c with respect to x squared. So that's a 2. What this is saying is that, to determine how rapidly this concentration is going to change at a particular point with respect of time, we need to know the curvature of the concentration with respect to position.
So many people were saying c here. And actually, this is where the concentration will remain exactly constant over this next delta t. And of course, if we want to know about what the concentration is going to do for a long time, then we have to do something more subtle.
But if we want to how the concentration is changing right now, then we just look at the curvature. And indeed, the curvature here is maximal. So concentration DCDT at this point, is it going to be greater or less than 0? Ready three, two, one.
PROFESSOR: Less than 0, right? Now, it's true that only the curvature at a particular point and matter is to know how the concentrations going to change over this next delta t. But if you want to know about what's going to happen over longer periods of time, than you have to worry about more of the global structure.
And what's true is that this local maximum may quickly go away. So you don't have to wait very long before this thing gets smooth and then DCDT will-- the magnitude will go to 0. And sometimes this big hump will stick around longer, but because of the curvature, this has the maximal magnitude of DCDT.
And it makes sense that the concentration is going to go down because the net flux here is to the right and to the left. And so there's a net flux, particles leaving, concentration is going to come down. Now are there any questions about what we've said?
If you find this discussion confusing, I would strongly encourage you to get together with a friend and just draw some random lines, curves, and just make sure you understand where diffusion is going to pull things and so forth. No questions?
All right, I just want to say a few things about the so-called French flag model in development. This is perhaps the most famous. Does everybody know what the French flag looks like? What's that? All right. There are three stripes and some orientation.
No, but the idea here is just that a simple way to specify a structure across one axis is to have a defusing chemical or generally a protein. And then based on the concentration of that protein or morphogen, you can just have the tissue read out what it's supposed to be, or what it's supposed to do.
So the idea here is that, you have some morphogen that starts maybe on one end of an embryo and then diffuses. So we end up with some curve that tells us about the morphogen concentration as a function of position. This is a functional position. So this is along the embryo.
Now the general challenge in the context of development is asking-- we started this embryo with many different cells, they're all genetically identical, and they all start out maybe without any positional information. They don't know where they are. But what that means is they don't know they need to develop into a head or a tail or something in between.
Now what often happens is that you can have a deposition often by the mother so you have a maternal deposition of, say, RNA that leads to expression of some morphogen on one end and then it diffuses. And based on the concentration you can say what part of the body you should be developing into.
So the idea is you just have these thresholds that might be some M1, M2. All right, now all the cells over here know that they should maybe develop into a head. Over here, they're going to develop into the mid body. And over down here it could be the low body, for example.
So just from the concentration that a cell feels at a particular location. So if you had a cell right here, you just say OK the concentration of morphogens between M1 and M2 and then from that you know what l you're supposed be.
Now if you have no degradation within the body. Let's just imagine you say, that at one end is 0. We're told morphogen concentration is M0. And let's say that the other end, we know the morphogen concentration is going to be 0. Let's say there's a lot of degradation at an end. If there's no degradation in between, what should the profile look like given these?
So, no. All right. So just diffusion with these boundary conditions. I'm going to draw some options and hopefully you can close your eyes and imagine what it should be. All right, we know that starts out M0 over L is equal to 0. Is it closer to A, B, C, or D? Do you understand what I'm asking? Yes.
AUDIENCE: That is a steady state.
PROFESSOR: This is the steady state concentration profile of the morphogen given there's no degradation in the interior. Ready three, two, one. All right. So at least a majority are agreeing it's going to be B. Although, there's a significant minority that's saying A.
This is why I'm bring this up because, we're so used to seeing exponential profiles and think that you should always be getting them. And I want to be clear why it's an exponential profile. That's the thing we start with, and for example this model in [INAUDIBLE] book.
Noori's book, you get exponential profiles as a result of first order degradation. Whereas if you don't have any degradation in the interior, then we say, OK, DCD-- well, do we want to use C or M now? We'll use M, just because now we're thinking very particularly for morphogen.
All so DMDT. We want to know the steady state profile. We set this thing equal to 0. Integrate twice. Right, so we're going to get-- morphogen profile should just be some Ax plus B, OK? My line's kind of crappy, I'm sorry. However, when we have first order degradation, then we get these exponential profiles. Are there any questions about--?
AUDIENCE: Is it possible to have [INAUDIBLE]?
PROFESSOR: Yeah, right. What does this require?
AUDIENCE: The morphogen is generated into halves. X equals 0 and then--
PROFESSOR: Degraded it at--
AUDIENCE: Disappearing.
PROFESSOR: Yeah. Right. That's what I'm saying, is that when I said that at the end it was a 0, this means that we have degradation at this point. Any time that the morphogen reaches the end of the embryos, it gets degraded. So it's not degradation inside if it's only degradation here.
And this of course a situation where you have constant flux throughout the embryo, constant rate of production of the morphogen, constant rate of degradation over here. But when you have this first order degradation-- if we're told that instead-- well, maybe.
So, if in addition to having diffusion, you also have this first order degradation. Now remember M is a function of both x and t, right? And this is the situation where we get exponential profiles.
Now in Murray's book, he often discusses this where the boundary condition is that you have some concentration M0 at one end. And then we get an exponential profile here. What was the characteristic length scale? This is something we should be able to figure out. You should, of course, be able to solve the equations, but you should be able to figure it out from our favorite approach of dimensional analysis. What's the units of things here? So units of D is what? Length squared over time. Units of alpha? So this is a morphogen concentration over a time. This is a morphogen concentration. So this thing has to be one over time.
So the length scale is then going to have to go as the square root of D over L. And indeed, if you solve it, it's exactly equal to that, but this dimensional analysis just told us that it had to scale like that. So this is telling us about a characteristic length of which this morphogen profile is going to fall off. This is the characteristic length L.
Incidentally, you'll see many cases of things that look like this. So if there's first order rate that's something is either degraded or is uptaken or whatnot, then together with diffusion, you'll get an exponential profile with a [INAUDIBLE] length scale that's given by this ratio. And you'll see this in many different contexts of, for example, nutrients going into a biofilm. If you have cells picking up nutrients then it's not that it's being degraded, but it's being imported. Then you'll have a similar process.
This is a nice, fine situation except for what was the problem that [INAUDIBLE] pointed out? It's not robust. It's not robust against what? Initial condition. Yes, but I think we have to be a little more specific on that. Right, it's not robust to changes in M0.
Incidentally, in most context, I think for mathematical simplicity, it's useful to just have a banner condition where M0 is constant. But in general, is that what the mother is going to be fixing necessarily? What would the mother typically be fixing? What was that?
AUDIENCE: Production rate.
PROFESSOR: Production rate. How do we figure out what the production rate is in this situation? That's right, the flux ware. That's right. The production rate is the flux right at or just to the right of 0.
Incidentally, where's the flux maximal here? Is it maximal at A, B, or C? Ready three, two, one. You can do it verbally. Fun. A. Right. And that's because the profile steepest here and then shallows out. So flux is decreasing here. And that's because some of the morphogen is being degraded.
So indeed, we can figure out the production rate. Right. This is equal to the flux, which is equal to minus DCDX at that location. Right, so the morphogen profile M is a function of x is some M0e to the minus x over L, where L is given by this character of lengthscale, right?
So if we ask what's DMDX? Well, it's going to be-- we get a minus M0 over L. Evaluated at x equal to 0 just makes that go away, so we end up with this. So you figure out what flux is, right?
Yeah, so, it's just an extra couple terms, it's fine. But that would be what would typically be constant because there might be some RNA deposit there or if it's actually transcription off of the genes at in the cells in this location and then it's based on whether there's one or two copies and so forth.
So this is all fine, except that this thing is not robust. It changes in M0 or this production rate. Whereas in many cases, what we seek experimentally is that if we, for example, have the production rate, then the profile that we see out here is somehow remarkably similar. Yes?
AUDIENCE: [INAUDIBLE].
PROFESSOR: Right. Yeah, OK. So I'd say that in many cases, it's not that it's directly this M squared business. But there are a number of cases in Drosophila, where they actually do see this. In many cases, it was a self enhanced degradation but not that it was directly, it was via binding to a receptor.
So this was seen in patch lists together. I want to remember which were the models that-- I'm worried I'm always doing it get so it's like patchless and frizzled, or-- are there any Drosophila people here that came up with these crazy names?
All right, yeah, so I always got confused which are the morphogens and which are the receptors in these situations. But I'd say that it is something that's observed in number of different contexts, at different stages of development, both in Drosophila and-- they see this in the wing as well as in the body.
And so in different stages I think it is signature. But I'm very much not a developmental biologist. All right so the proposal that [INAUDIBLE] suggests as a way to make this thing more robust is to somehow change this term.
Now, the goal in all this was to have some degradation rate. And he writes it as F is a function concentration M. In principle, this thing could be a function of the position x, but he doesn't want that. And why was it that he didn't want that? Yeah.
AUDIENCE: Because we already know [INAUDIBLE].
PROFESSOR: Yes, right. So the whole goal is that we want to start with some situation where we don't know where we are as a function of position x. And then from that get some spatial information. So we really want something that's not a function of x in there. Because then we've already solved the problem that we're trying to address.
And so one proposal is indeed just to have something that-- and this is very much a phenomenological approach-- which is to have this thing be self enhanced degradation. So the idea is that here if we have the morphogen profile that looks something like this.
There are multiple ways you can have something that looks like this. So if along the way to degradation, if the morphogen actually binds the receptor, but then activates the degradation. And this is seen, for example, in Drosophila. This is Hedgehog and Patched. So R is-- in this case, you have the morphogen is diffusing outside the cells. It binds to the receptor, and then the receptor activates imported degradation of the morphogen.
So it's not that the morphogen is directly leading to its own degradation, but it's via some network here. And there was another one that they talk about, which is that, when the degradation is mediated by 2. These look like this, and this is in context. And this is again Drosophila, but you could probably guess what this thing does. And so it is a wing development as well.
If you write down an actual model of one of these things, and you could play with it and see where you're going to get something that's going to look like an M squared type term. But the nice thing about this phenomenological approach is it kind of gives you a sense of the kinds of effects that you should be looking for that will enhance the robustness of the pattern.
Now the key thing in this whole discussion to remember is that if you have a profile here, if you change, it's just somehow translationally invarion in the sense that since there's no information about where you are here, these patterns can kind of slide over. So if you change the boundary condition M0, that's just equivalent to sliding this over some distance and you could figure out where everything has to go.
And that's true not just for the exponential profile, but for any of the profiles. And that's just because there's no information about what's going on once you're inside the embryo. Can somebody give the intuitive explanation for why some sort of self enhanced degradation like this might make the pattern more robust?
AUDIENCE: Decrease faster [INAUDIBLE].
PROFESSOR: Decrease faster when M is big. That's right. And so the idea is that if you change the M0 here, for example, you double it, then with a self enhanced degradation you kind of quickly come back to where you were. So there's some sense that if the pattern quickly comes down, then you can get it being more robust.
In particular, this profile for large x in the proper regime, this thing falls off as some A a over x squared. And indeed, one of the problems in Murray's book is that if this is just degrading as M to some power n, then you can calculate what happens here. But this is some sort of power law fall off.
So of course, it's falling off rapidly here, but not as rapidly as if it were an exponential. And this is just like in the case of the power law distributions of networks that we were talking about before, that for the exponential fall off, then it's just very, very unlikely that you'll find some node with many edges. Similarly here, you quickly fall to zero here as compared to a power law distribution. So that means that you can specify patterns out further in some sense.
Are there any questions about this basic idea and where it comes from? I think the discussion in [INAUDIBLE] book is actually reasonable. So I don't really want to spend too much time on it. In the context of the eldar model that was from the last section of that chapter.
One thing I just want to highlight is that it's useful to be able to look at these models, and from the equations that you see-- for example, the supplemental material of a paper-- make sure you are very clear about what assumptions they've made in getting to this model. And so maybe we'll just spend a few minutes talking about this.
So this is again a Drosphil embryo two, two and a half hours after fertilization where at that stage, you really have the embryo that looks like a-- we're trying to understand the patterning around the front back. So we think about diffusion along in one dimension, but it's really around this radial direction. And in particular, where this is trying to understand patterning in the dorsal region.
And what we have is a situation where we have this protease inhibitor. And so the protease distributed uniformly. Inhibitor starts out out there, and then we have the morphogen that somehow starts out in here. And we want to end up with a situation where the morphogen profile in here is kind of well defined. So where you just have the morphogen at the center of this dorsal region.
And then we have diffusion of these inhibitors coming in and then the morphogen coming out. And the question is, how can we make sense of this? And if you look at the equations that they write down-- So we have diffusion of the inhibitor. This is the complex of the inhibitor and the morphogen-- complex c.
And so this is a case where they wrote down what they basically knew about the biologists system. And then they asked, well, what range of parameters would lead to a robust pattern of the morphogen in here, if you vary things such as the total concentration inhibitor, the morphogen or the protease?
So we have diffusion of each of these things, the inhibitor, the morphogen complex. We're not going to worry about the protease, because this thing is uniformly distributed. You can argue about whether that's the right thing to do, but that's based on the model. So we have diffusion of each of these guys. Can somebody say what's going on here? What are these things trying to capture? John?
AUDIENCE: [INAUDIBLE].
PROFESSOR: So this is just saying, OK, the complex is formed as a result of binding of the inhibitor and the morphogen. It's proportional to concentration those two things, at that particular position at the particular time. And just remember that any time that you create something, if you have binding at two things together, then you have to consider these things going away. And it's not they're being degraded, it's just that they're forming this complex. So in these equations you just have to be very careful about keeping track of which things are really going away and which things are just changing form. Yeah?
AUDIENCE: [INAUDIBLE].
PROFESSOR: Right. You're saying why that there's not a minus term? So this is an assumption. And I think this is a kind of thing that you have to be clear about in any of these, because that's always going to occur at some rate. And the assumption is that this is dominant. And then just be clear in words, what is this thing?
AUDIENCE: [INAUDIBLE].
PROFESSOR: Right. The protease. And you're saying cleaving inhibitor.
AUDIENCE: Yeah, cleaving the compacts by degrading the [INAUDIBLE].
PROFESSOR: Right. So at a rate proportional to the Proteus concentration-- the complex concentration-- this complex goes away. Now the question is we have to figure out where it went or what happened, right?
Well we have a minus alpha c here but then we have a plus alpha c here. But this isn't the morphogen right. This is at the rate that the complex somehow is being disappeared by the protease, the morphogen is appearing. But then, we don't have the same term here. And that's how you know that what the protease is doing is it's degrading the inhibitor as part of the complex.
So by looking at these equations, you can actually figure out what are the assumptions that have been made of what the biology is that's being captured here. But in many cases, there are assumptions of what's big and what's small. And then what's this thing here? Somebody else that has not yet-- do these equations look familiar? Yes, please.
AUDIENCE: It's the protease [INAUDIBLE].
PROFESSOR: That's right. It's the protease degrading the inhibitor when the inhibitor is not down to the morphogen. Now of course, what the authors did next is they searched numerically across parameter space over [INAUDIBLE] magnitude, and they found that for some parameter regime there was a robust pattern of the morphogen developed in here. There's no reason for you to necessarily remember which thing it was.
But what they found is that if this term went away and this term went away, then you end up getting a robust profile of the morphogen against changes in the overall morphogen inhibitor concentration.
Now this is pretty weird, I would say. I don't know, do you think-- is this one weird? Weirdness is in the eye of the beholder. But I would say it's not so shocking. Can somebody say verbally what this would correspond to?
AUDIENCE: The protease doesn't degrade the inhibitor on its own.
PROFESSOR: That's right, the protease doesn't degrade the inhibitor on its own, only when it's part of the complex with the morphogen. And independently there was experimental data already indicating that was true. So you could have if you want to just written the model without that, because there was already evidence for that.
But as I said, this is a pretty weird thing. And so, this would be saying that, for some reason, diffusion of the morphogen on its own is very small. In particular, small compared diffusion of the morphogen when it's a complex. Now can somebody say why I might think that's weird? You don't have to believe it's weird.
AUDIENCE: You'd think bigger is better.
PROFESSOR: That's right. Exactly. This is saying, well, when it's complex, it's bigger, it's somehow diffusing faster. So this would not happen do the simple Stokes type drag. This would have to be biology. Somebody's biology is allowed to do anything that doesn't violate the laws of physics.
Indeed, later there was experimental evidence of something like this was actually happening. And you could imagine having either from sort of active transport type dynamics for the complex, or from binding type dynamics of the morphogen.
I think the key thing to take from this example is just that, in general, we would like biological function-- biology would like biological function to be robust to things that are often fluctuating or varying. And that's one way to try to make guesses about what might be happening in the system.
This sort of computational exercise is not all a proof that this has to be what's happening. They wrote down a particular model-- it could have been that there are other terms they're not aware of and so forth. But it's at least a way of generating hypotheses that you can go and test.
And quite generally, I'd also say that its essential for all of us as consumers of models to be able to look at some of these equations and figure out what it is that they've assumed.
I want to move on to this idea of pattern formation via the reaction diffusion or Turing-type patterns. And I think it's really important to start by just acknowledging that this is really a surprising finding, that it's even possible for this to happen.
Because this is a situation where you have a couple chemicals-- or proteins, or reacting elements-- and if you just mix them, if you have them in some well mixed tube, they reach some steady state. Whereas somehow if you allow diffusion, then you can get these patterns. And I would say based on my intuition at least, I would not have thought that this would be possible.
And this really just is because if you can just imagine, you start with some profile. Now there's some structure here. But diffusion's going to actually cause this to come down, and this to come up. So diffusion acts to remove these spatial patterns. But somehow in some prime regimes, if you have coupled reactions that are activating and inhibiting each other, then you can actually get these spatial patterns.
And as you read about, there's some idea that you need to have a local activation and a global inhibition. But ultimately the mathematical-- you always have to go and think about more carefully about the math, and would be indicated just by those words. This is at least a way to guide your thinking a little bit.
But ultimately what matters are around this stable what would be the stable fixed point, you have to actually look at these derivatives and so forth. And the derivatives can be subtle I would say. Just because you think of something as activator inhibitor doesn't mean that it's going to have that role around the fix point that you're studying. So this is just a caution.
What I want to do is just give you, for example, one example of a simple model that does experience these Turing patterns. And in an appropriate regime. And this Levin-Segel model of pattern formation.
And this was published in 1976, and it was actually meant as a model of predator-prey interactions in ecology. We'll talk significantly more about predator-prey interactions in a few weeks. But I just want to write down what they said.
And this is a simplified model of their stuff. So it's actually trying to think about some plankton herbivore before interactions. And this is derivative with respect to time. We're going to follow the nomenclature of a paper by Butler and Goldenfeld a few years ago, because they're the ones who thought about the demographic noise enhancing the patterns.
PROFESSOR: All right, so we have [? phi ?] and [? phi. ?] First of all, who's eating whom? All right, we're going to say it verbally. Who is the predator? Ready three, two, one.
AUDIENCE: Herbivore.
PROFESSOR: Herbivore. Herbivores are not what they used to be. So indeed, the herbivore benefits from the presence of the plankton. So nobody cares about plankton, I guess.
So this corresponds to the predator-prey type interaction. So there's some death rate. So let's see, the herbivore or the predator. And then what you see is there's these two growth terms for the plankton. So there's this term, which would be kind of simple, exponential growth. And then this term, which is actually some sort of super exponential growth.
There's some sense in which the plankton benefit each other. And this was originally introduced because of something called predator satiation. But it's just a general reflection of the fact that in many cases, individuals benefit from the presence of other individuals. And in particular, this is known as the ally effect, and we'll spend time talking about this in a few weeks as well in the context of populations and ecology. For now, I just want to use this as an example of a model that gives you these Turing patterns.
So if you want to, you can go ahead and ask, well, what happens if we just have a well mixed situation? If everything is not a function of x, but it's still can to be a function of time. You can solve these equations, and you can find what the steady states are equal to. So there is indeed a steady state stable coexistence of the predator and the prey in a well mixed situation.
However, if you then go and you analyze the stability of different spatial modes, what you'll find is that in some situations, particular wavelength or wave vectors become unstable. And it's just over some range of wavelengths, and that corresponds to the wave length of the Turing patterns that you'll see.
If you're curious about these things in more depth, I encourage you to attend Mehran Kardar's class Statistical Physics in Biology, he is an expert on these topics and I think is a wonderful clear lecturer.
Now there's going to be some condition for these things leading to Turing patterns. Now from your reading, do you think it's going to be mu--? So this is Turing patterns require-- I'll give you 30 seconds to think about what. Do you need more time? I'm not sure where we are. Maybe another 15 seconds just to--
Let's see where we are. Ready three, two, one. OK, we're all over the place. I think we're uniformly distributed between A, B, and C. So there's an idea that you're supposed to have so-called local activation and global inhibition. Why don't we turn to a neighbor and see if you can figure out what's going on.
OK, why don't we reconvene, I'm curious where you're thinking is. Let's go ahead and vote. Ready three, two, one. OK, so we have many, many C's. The idea that some mu has to be much less the nu. Yes. I want to make sure. All right, mu is telling us about the diffusion coefficient of the prey. Nu is the diffusion coefficient of the predator.
So what you want is to have local activation. And the thing that's activating itself is the plankton. So you can see that that's like, for example, psi squared term. And from the stand point of a spatial situation, you can say, all right, well, let's say we have some region with a lot of this prey. Well, it's able to activate itself, so it kind of comes up. And as it does that, it is creating more of the predator, phi.
But because the predator has a larger diffusion coefficient-- well, it's obviously going to grow here. But it will also diffuse away. And that's this global inhibition of neighboring regions. Now of course, these are just words, you have to take it a little bit of a grain of salt.
But if you can actually do this calculation analytically, and derive in this model the condition for Turing patterns to emerge as a function of everything. And for example, if you have b 1/2, p 1. So for unity type parameters, then the requirement to get Turing patterns is that nu over mu is the greater than 27.8.
The key thing though is that this thing is much larger than 1. So a general thing that emerges in these Turing patterns is that you need this so-called inhibiting type partner to have a much larger effective diffusion coefficient than the activating partner.
Now the problem with this is that this thing is indeed much larger than 1, which limits the overall usefulness of this is a mechanism. Because if it really is simple diffusion, how much bigger would the-- let's say, low Reynolds number regime, these are just molecules experience diffusion. How much bigger does the-- this is so confusing.
So this is big and this is little, right? So how much bigger does the activator-- they're too many things to keep track of here. It's because I don't have anything written down. So that's the activator. So this one's the activator, and this one's the inhibitor. And the inhibitors has to diffuse much more than the activator. So the inhibitor has to be much smaller than the activator.
OK good, so how much bigger does the activator have to be than the inhibitor for this to be true? Does it scale as this 28 or 28 squared or 28 cubed? In terms of radius, how much? Scales linearly, remember? Einstein equation, you guys had fun thinking about on the exam. KT over gamma.
This would require, for example, the activator to be 30 times bigger in terms of radius. And the inhibitor, in order to have this emerge just as a result of diffusion, simple diffusion. And that's just not a typical thing for just a range of protein sizes. But you could imagine putting up various ways to make this happen.
But there is a very nice development that I mentioned, which is that there's another surprising aspect in these problems, which is that in many cases, demographic noise can lead to patterns-- -- or maybe you might call them quasi-patterns. But things that, for all intents and purposes, look like some of this pseudo-periodic. So there's a pseudo-Turing pattern, maybe.
And indeed, if you actually do this simulation with those parameters where you do the explicit demographic fluctuations-- i.e. you take into account that this corresponds to a prey giving birth. This corresponds to a predator eating a prey, random events just like what we've done in the context of a Gillespie simulation.
Then what you find is that with demographic fluctuations or demographic noise, then the condition here nu over mu has to be only 2.48. And indeed in Butler and Goldenfeld, they derive these things using field theoretic approaches. There was a 2011 paper and also 2008 or 2009.
The nice thing here is that what you see is that in the presence of these demographic noise that will be there, the difference in diffusivity that are required is not nearly as large as when you're thinking about the mean field equations. Are there any questions about this before we talk about center fighting in E. coli? Yeah.
AUDIENCE: [INAUDIBLE].
PROFESSOR: OK, now you're thinking about the actual plankton-herbivore. I think there are many things to say. One of them would just be that maybe the patterns that you see between actual plankton and actual herbivores is not due to the Turing mechanism.
For the Turing mechanism to be at play to generate spatial patterns, it requires that this inhibitor do something equivalent to diffusion more rapidly. It has to have to move away more rapidly.
AUDIENCE: [INAUDIBLE].
PROFESSOR: Right. It could be on the plankton only move around on the currents, whereas the herbivore actually has direct motions. I have to say, I don't actually know enough about plankton to know whether this is at all-- That base statement I thin is true, but I don't know how the numbers work out. Yeah.
AUDIENCE: [INAUDIBLE].
PROFESSOR: Yes. Both in this case and the simple predator-prey oscillations. One thing we're going to see the predator-prey populations later is that you can also get this sort of oscillations in time. So this could be in time or this could be a function of space. But in both cases, it's really that you have a resonant enhancement in some areas. So that the demographic fluctuation somehow excite all frequencies or all wavelengths.
But then what happens is that, in this case, if you look at the eigenvalues-- OK, I want to be close to 0, though. If you look at how the modes decay-- so the eigenvalue's function of a wave number-- what you see is that you end up getting things that look like this.
So whereas a Turing pattern is when particular wave length or wave modes actually becomes unstable, and then you get mean field type patterns. And in this situation what happens is that you're just close here, so then you excite all the wave numbers or wavelengths. And then some of them take a long time to go away, so then they build up and then that's the resulting patterns that you see.
So what I want to do is just for the last 10 minutes to talk about this Min system. Because it's I think it's a beautiful example of a combination of Systems Biology questions together with reaction diffusion systems, and also some beautiful in vitro experiments that I've quite appreciated.
So the question is-- imagine yourself, you've gone to all this work right, so now you're nice and long. So you'd like to divide into two cells. And the question is, how do you know where to divide? So you imagine that you're some E. coli cell. You're maybe six microns long, everything is great. And the question is, you want to divide? Where would you like to divide if you're an E. coli cell? Middle.
OK, so what you'd like to do is go here. And indeed, what happens is that there's the so-called z-ring. There's a a pseudo-polymer protein that forms a ring around here, then it constricts itself and pinches off the membrane. And that's how you get two cells.
So there's this formation of this z-ring that constricts and that's this cell division event. The question is how do you know where to put it? And that's what this Min system is for. And it's, of course, like everything in bacterial genetics, it was identify by mutations.
So the Min system it's called that because these mutants formed so-called mini cells. If you, instead of dividing the center where you might have two copies of the genome here. If instead you divide over here, then what your going to end up with is a very long cell with two copies of the genome. You're going to end up with this mini cell without any DNA.
And those mini cells, are they going to do well for the long term?
AUDIENCE: No.
PROFESSOR: Right. They actually can survive for a little bi. And people have argued that maybe that could be useful for synthetic biology because they still make proteins, but they're not going to go take over the world because they don't have any DNA. But these are the so-called mini cells that happen if you have mutations in The min system.
Now, I think the three players are MinC, MinD, and MinE. So I think that MinA and MinB ended up not actually existing or something, in the sense that they identified the mutants, but then they were wrong about something. So the three that actually ended up being involved in the actual Min system are C, D and E.
So this MinC prevents formation of the z-ring. Now MinD and MinE are kind of the amazing guys. So this guy binds to the membrane and recruits MinC. Whereas MinE-- what it does is it pulls MinD off the membrane. So it binds to MinD and ejects from the membrane.
And what has been seen by doing imaging in live E. coli cells with fluorescently labeled MinD and MinE is that there are remarkable oscillations from pole to pole. And indeed, that doesn't require MinC, so MinC is somehow following the others.
What happens is that MinD binds over here, then MinE binds and pushes it off. And then the MinD comes over here, and then MinE pulls it off, and it goes back and forth. The period is minutes, maybe. So it's a really remarkable thing that happens in vivo.
And the idea of what's happening is that if MinD comes here and then it comes here, on each of the edges, then it doesn't hang out in the middle. And that means that it's only in the middle where MinC can then bind-- oh, sorry. Because MinC is following MinD, then only in the middle can you form the z-ring, because that's where MinC is not. Right.
It's wonderful to do things in live cells because that's the native context and so forth. But I think that in some cases, it's also wonderful if you can take purified components and recapitulate interesting behaviors in vitro. Because then at least you know what is sufficient to generate a particular kind of dynamical behavior.
And because this system had been proposes as a model system for reaction diffusion mechanism to get this behavior. And so there's this paper by Martin Loose, in Petra Schwille's lab in-- where was it? In Dresden.
And what they did is they took a supported lipid bilayer-- so a membrane on glass-- and then they added purified components of MinD and MinE that were fluorescently labeled. And then they saw amazing patterns, these amazing reaction diffusion waves traveling along.
Now on the first day of lecture, I actually showed you what some of those things look like. I didn't want to use up the projector again, but maybe I can pull up this movie because it is kind of fabulous. So maybe you can't see these things very well. But this is a MinD and MinE and an overlay of the two, imaged on a two dimensional membrane.
And the movies are just amazing. When you see this, you think that it's a simulation. It's so incredible watching these things go. After class, you can come up, I can show you the paper, and you can look at the movie in more depth. But they're really amazing patterns, and it's patterns that you would predict from some sort of Turing type reaction diffusion mechanism.
So there are few things that are may be worth saying in this business. So these are fluorescently labeled MinD, MinE. They started out with MinD that was uniform. And then they added MinE, and over the time scale of about an hour they started seeing these sorts of patterns.
And as maybe you expected from the in vivo behavior, what you see are these things where MinD looks like this, whereas MinE looks like that. And then the wave travels here to the left. Because the MinE is ejecting the MinD from the membrane, causing this whole thing to move.
So this is a situation where you have both of these proteins in the liquid. All right, so in buffer, as well as on the membrane and they're coming on and off and so forth. And in this situation, it's wonderful because they can do all sorts of things like control the concentration of MinE, and see that the velocity of these waves changes as you change concentration in MinE.
So this is a really experimentally trackable system. And then you can ask what kind of model would lead to that sort of behavior? Such waves, do you think that it requires ATP? Just for fun, we can vote yes or no, it's all right. Ready three, two, one.
AUDIENCE: Yes.
PROFESSOR: Yeah. So indeed it does require ATP. And that's a general feature of these Turing type patterns is that there is a non-equilibrium structure formation.
Now one nice thing you can do in this sort of system is you can ask, well, what happens if I photo bleach a particular area? So I come in and I photo bleach. So now the profile looks like-- I locally deplete the fluorescence here.
Question is, will this move together with the traveling wave, or does it stay fixed? And in these mechanisms, do you think should this locally depleted area, should it move or should it stay where it is? Do you understand the question? Move, yes/no. Ready three, two, one.
AUDIENCE: [INAUDIBLE].
PROFESSOR: It actually doesn't move. Depleted area doesn't move. And this is just a reflection. These waves are not the result of individual molecules moving together with the wave. A wave like what you see in the ocean, or on a stream, or what not.
So the motion is a result of the individual molecules coming and going and communicating with each other, but it's not a reflection of actual molecules having directed motion. Because indeed, there's no mechanism in these models for directed motion. What you see is that in all these models, the only thing that is a function of position is diffusion terms. So it's all random diffusion, but then you get global motion.
Free Downloads
Video
- iTunes U (MP4 - 181MB)
- Internet Archive (MP4 - 181MB)
Subtitle
- English - US (SRT)