Flash and JavaScript are required for this feature.
Download the video from iTunes U or the Internet Archive.
Description: In this lecture, Prof. Jeff Gore begins with a review problem on rugged landscapes. He then moved on to the main subject: evolutionary game theory. This includes the Nash equilibrium, the prisoner's dilemma, and the hawk-dove game.
Instructor: Prof. Jeff Gore
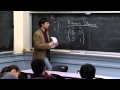
Evolutionary Games
The following content is provided under a Creative Commons license. Your support will help MIT OpenCourseWare continue to offer high-quality educational resources for free. To make a donation or view additional materials from hundreds of MIT courses, visit MIT OpenCourseWare at ocw.mit.edu.
PROFESSOR: All right, so today what we're going to do is we're just start with a short review problem on rugged landscapes, just so that you get some sense of the kind of thing that I would expect you to be able to do a week from today. And then we'll get into the core topic of the class, which is evolutionary game theory. And we'll discuss why it is that you don't need to invoke any notion of rationality, which is kind of the traditional thing we do when we're talking about game theory applied to human decision making.
Then we'll try to understand this difference to know what a Nash equilibrium is in the context of game theory versus an evolutionary stable strategy in this context. And we'll say something about the evolution of cooperation and experiments that one can do with microbial populations in the laboratory. Are there any questions before I get started?
All right, so just on this question of evolutionary paths, on Tuesday we discussed the Weinreich paper where he talked about sort of different models that you might use to try to make estimates of the path that evolution might take on that fitness landscape that he measured. So he measured this MIC, the minimum inhibitory concentration, on all 2 to the 5 or 32 different states, and then tried to say something about the probability that different paths will be taken. So I just want to explore this question about paths in a simpler landscape, where by construction here, I'm to going to give you some fitness values just so that we can be clear about why it is that there might be different paths, or what determines the probability that different paths are taken.
So what we want to do is assume that we are in a population that is experiencing this Moran process or Moran model, constant population size N equal to, in this case, we'll say 1,000. And let's say that the mutation rate is 10 to the minus 6. So each time that an individual divides, it has a 1 in a million probability of mutating. And that's a per base pair mutation rate. And I'll show you what I mean by that.
And in particular, we're going to have genotypes. Originally when we discussed this, we were talking about just mutations, maybe A's and B's. But now, what we're going to have is just a short genome that's string length 2. So we might have 0, 0, which has relative fitness 1, 0, 1.
So we're assuming that this is relative fitness as compared to the 0, 0 state. We're going to start in the 0, 0 state with 1,000 isogenic individuals, all 0, 0. And the question is, what's going to happen eventually?
And in particular, what path will be taken on this landscape here? In particular, what we want to know is the probability of taking this path.
You can start thinking about it while I write out some possibilities that we can vote for, and I'll give you a minute to think about it. So don't--
Are there any questions about what I'm trying to ask here?
AUDIENCE: So this is the long time? So we assume that in the long time, it will go from 0, 0 to 1, 1?
PROFESSOR: That's right, yes, so if we wait long enough, the population will get there, and the 1, 1 genotype will fix in the population. We can talk a bit later about how long it's going to take to get there, and so forth.
AUDIENCE: And we're assuming that from 0, 1, it can't go back to 0, 0?
PROFESSOR: Right. Yeah, so we'll discuss the situations when we have to worry about that, and when we don't, and so forth. But for now, if you'd like, we can say that this is even just mu sub b, the beneficial mutation rate per base pair, assuming that the 0's can only turn into 1s. Then after we think about this, we could figure out if that's important, or when it's important, and so forth. Yes.
AUDIENCE: [INAUDIBLE]?
PROFESSOR: No. All right, so we're starting with all 1,000 individuals being in the 0, 0 state, because now we're allowing some mutation rate.
AUDIENCE: [INAUDIBLE].
PROFESSOR: And you also have to think about this first mutation-- will it fix or not?
AUDIENCE: But it's not [INAUDIBLE] first mutation, the mutation of one element of that population will go to 0, 1 [INAUDIBLE]?
PROFESSOR: I'm not sure if I understand your question.
AUDIENCE: So if we start out with all 0, 0, and then one mutation [INAUDIBLE]? And if that mutation-- are we assuming that that mutation is 0, 1 and then figuring out [INAUDIBLE]?
PROFESSOR: Well, OK, you're asking kind of what I mean by path here.
AUDIENCE: Yeah, I guess.
PROFESSOR: Yeah, all right, so I'll say path means that that this was the dominant probability trajectory of the population through there. We'll also discuss whether it somehow is very likely is going to kind of have to go through one or the other of them. The probability of getting both mutations in one generation is going to be 10 to the minus 12.
So that's going to be a very rare thing, at least given these parameters, and so forth. And then there's another question, which is, will 0, 1 actually fix in the population before you later fix this population? And actually, I think the answers to all these questions are in principal already on the board. Because there's a question of do we have to worry about clonal interference? Are these things neutral or not?
And really, this is in some ways a very simple problem. But in another way, you have to keep track of lots of different things, and which regime we're in and so forth. So that's what makes it such a wonderful exam problem.
If you understand what's going on, you can answer it in a minute. But if you don't understand what's going on, it'll take you an hour. Yes? No? Maybe?
Well, I'll give you another 20 seconds. Hopefully, you've been thinking about it while we've been talking.
All right, do you need more time?
Why don't we go ahead and vote? I think it's very likely that we will not be at the kind of 100% mark, in which case you'll have a chance to talk about it and think about it some more. Ready? Three, two, one.
OK, all right, so we do have a fair range of answers. I'd say it might be kind of something like 50-50. And that's great. It means that there should be something to talk about.
So turn to a neighbor. You should be able to find somebody that disagrees with you. And if everyone around you agrees, you can maybe-- all right, so there's a group of D's and a group of B's here, which means that everybody--
AUDIENCE: Let's fight.
PROFESSOR: All right, so everybody thinks that everybody agrees with them, but you just need to look a little bit more long distance. So turn to a pseudo-neighbor. You should be able to find somebody there. It's roughly even here, so you should be able to find someone.
[INTERPOSING VOICES]
So I don't see much in the way of vibrant discussion and argument. You guys should be passionately defending your choice here.
[INTERPOSING VOICES]
Yeah, that's a higher order point. I wouldn't worry about that.
[INTERPOSING VOICES]
All right, it looks like people are having a nice discussion. But I might still go ahead and cut it short, just so that we can get on to evolutionary game theory. But I would like to see where people are. And we'll discuss it as a group, so don't be too disappointed if you don't get finished there.
But I do want to see kind of where we are. Ready? Three, two, one. OK, so it still is, maybe, split roughly equally between D and maybe a B-ish and some C's. All right, does somebody want to volunteer their explanation? Yes.
AUDIENCE: I'm not sure how good it is, but I was thinking about what's the probability of going to 0, 1 instead of 1, 0. And I just took it as the ratio of the extra benefit of 0, 1 over the benefit of 1, 0.
PROFESSOR: Sure, OK, and just to start out, which answer are you arguing for?
AUDIENCE: D.
PROFESSOR: D, OK, all right. So you're saying D. And you're saying, all right, maybe because of the extra, that 1, 0 is somehow more fit than 0, 1. And you've taken some relative rates or ratios for which reason?
AUDIENCE: Well, I took 0.02 and then 0.1, which is 1/5. And then I decided that that should be around what it is, but slightly less, because there's also a chance that [INAUDIBLE].
PROFESSOR: OK, yes. I think the arguments there-- there's a lot of truth to the arguments that you're saying. Yeah, it's a little-- right and another question is exactly why might it be 1/6 instead of 1/5 is, I think, a little bit hazy in this here. It's OK, but it's close.
Does somebody want to offer an explanation? So here, that was an argument of roughly maybe why it's D-ish. Because D is very different from B-- order of magnitude different. So can somebody offer why their neighbor thought it was B? Yeah.
AUDIENCE: So I knew that it was B, because I considered the two paths, both from 0, 0 to 0, 1. I first checked S, N and it's non-neutral. So probably [INAUDIBLE] S. So the probability for that first path would be the S for 0, 1, so it's 0.02, which is 1/50, multiplied by the probability that the other [INAUDIBLE] 1, 0 would die out [INAUDIBLE].
PROFESSOR: Right, so there are two related questions. And I think that this explanation here is answering a slightly different question. OK, so let me try to explain what the two questions are here.
So the question that you're answering is, if you have kind of 998 individuals that are 0, 0 individuals, and you have one that's 0, 1, and you have one individual that is 1, 0. So this is like these problems that we did a couple weeks ago, where we said, you imagine in the population you have a couple different kinds of mutants that are present maybe in one copy. And then we were asking, well, what's the probability that this individual is going to fix? And what's the probability this individual is going to fix? And what's the probability that these guys are going to go extinct, and this one will therefore fix?
And I think that's the calculation that you're describing, where you say, OK, well, in order for this individual to fix, he has to survive stochastic extinction, which happens with the probability of 2%. And the 1, 0 individual has to go extinct, which happens 90% of the time. And so this is, indeed, answering the question that if you had one copy of each of these two mutant individuals in the population, that's the answer to what is the probability that this 0, 1 mutant would fix in the population. Right?
But that's a slightly different question than if we ask, we're going to start with an entire population at 0, 0, and now these mutations will be occurring randomly at some rate. And then something's going to happen. Somehow the population is going to climb up this fitness landscape.
And we're trying to figure out the relative probability that it's going to take kind of one path or another. Do you see the difference between these two questions? So indeed, this is the correct answer to a different question.
And so it's going to end up being D. And now we want to try to figure out how to get there. Because I think it is a bit tricky.
And in order in order to figure out to get there, we have to make sure we keep track of which parameter regime we're in. So there are a couple of questions we have to ask. First of all, we have to remember that we start out with everybody, all 1,000 individuals in the 0, 0 state.
So there are initially no mutants in the population. But they're just replicating at some rate. And every now and then, mutation's going to occur.
Now one thing we have to answer, we have to think about, is whether these are nearly neutral mutations. Verbally yes or no? Ready? Three, two, one.
AUDIENCE: No.
PROFESSOR: No, right. And that's because we want to ask for, if it's nearly neutral, we want to ask, is the magnitude of S times N much greater or much less than 1? In particular, if they're much greater than 1, as is the case here, then we're in a nice, simple regime.
And it's easy to get paralyzed in this situation, because there's more than one S. But in both cases, S times N is much larger than 1. We can take the smaller S, which is 2 over 100, and S times N is 20, right? So S for the 0, 1 state times N is 20, which is much greater than 1.
What this tells us is that if we do get this mutant appearing in the population, then he or she will have a probability S of surviving stochastic extinction. So probability of surviving stochastic extinction if the individual appears is equal to S 0, 1, which is equal to 2%. Whereas for the 1, 0 state, that's going to be 0.1.
Now, this is assuming that the mutation appears in the population, that's the probability it will survive stochastic extinction. Now, just as a reminder, surviving stochastic extinction roughly corresponds to this becoming an established idea. And becoming established was what again?
AUDIENCE: [INAUDIBLE].
PROFESSOR: What's that?
AUDIENCE: S1 is [INAUDIBLE].
PROFESSOR: It's when S1--
AUDIENCE: [INAUDIBLE].
PROFESSOR: Yeah, that's right. All right, so established-- when we say established, what we mean is that this corresponds to saying that this probability that we talked about before this X sub i is approximately equal to 1. So this question is, how many individuals do you have to get to in the population before you're very likely to fix?
And what we found is that that number established went as 1 over the selection coefficient. So in this case, you would need to have 50 individuals before you were kind of more likely to fix than not. So if you want to be much more likely, you might need twice that or so. Do you guys remember that? This is not important for this question, necessarily, but it might be important at a later date.
AUDIENCE: And so for nearly neutral mutations, the whole point is that the number needed to become established is equal to the population.
PROFESSOR: Yeah, so everything kind of works, right? OK, so the way that we can think about this is, now we have this population, 1,000 individuals. They're dividing at some rate.
Mutations are going to appear. Now we know if they did appear, the probability they would fix. This is assuming there's no clonal interference, right?
Because if there's clonal interference, then surviving stochastic extinction is not the same thing as fixing. If they both appear in the population, and they both survive stochastic extinction, then this mutant loses to this mutant. That's the clonal interference. Do we have to worry about clonal interference in this situation?
So remember, this was comparing the two time scales. This was comparing the time between successive establishment events, which went as 1 over mu N S. And the other one is the time between the time to fix, which went as 1 over S log of NS, right?
So we can ignore clonal interference if this is much larger than that. So no clonal interference corresponds to mu N log NS much less than 1. No clonal interference, same as this statement. Is that right? Did I do it right? OK.
So and once again, there are multiple S's, and it's easy to get kind of upset about this. But you can just use whichever S would be-- which S would you want to use to be kind of--
AUDIENCE: Small or large [INAUDIBLE]?
PROFESSOR: To be on the safe or conservative side, we want to take this to be as big as possible. So we take S actually as big as we can, right? It's in the log. So details, right?
But we can see we have 10 to the minus 6, 10 to the 3, and then this is the log of maybe 100, which is, like, 4 or 5. Is it closer to 4 or 5? I don't know, but it doesn't matter. We'll say 5. This is indeed much less than 1.
So indeed, we don't have to worry about clonal interference. This is a wonderful simplification. What it's saying is that the population is dividing. Every now and then, a mutation occurs in the population.
It could be either the 0, 1 or the 1, 0. But in either case, the fate of that mutation is resolved before the next mutation occurs. So you don't need to worry about them competing in the population.
Instead, just at some constant rate they're appearing. And given that they appear, there's some probability that they're going to fix. So that leads to effective rates going to each of those two steps-- going to 0, 1 or 1, 0.
And in particular, this is like a chemical reaction, where we have some chemical state here. We have two rates. There's the k going to 0, 1, the k going to 1, 0.
And what we know is we know the ratio of those rates. And that's everything we need to know to calculate the relative probabilities of taking those states, because the probability of going through to 0, 1-- we want to go that direction-- 0, 1, this is going to be given by k 0, 1 divided by k 0, 1 plus k 1, 0.
So this is how we get 1/6 instead of 1/5. Because this thing is 1/5 of that. So it's like 1, and then 1, 5.
So this is actually, in principle, not quite answering the question that I asked, because this is talking about the relative probability of the first state, the first mutant to fix. In principle, it is possible that from there, there's some rate of coming back. Or they might not necessarily move forward on up that hill.
Do you guys understand what I'm talking about? Because it goes from here to there. Because we really want to know about this next step, going to the 1, 1 state.
But in this case do we have to worry about going backwards? No. And why not?
It's very unlikely. And in particular, you could think now that you're here you can talk about the rate of going to the 1, 1 state as compared to the rate of going to 0, 1. And those are going to be exponentially different.
Because just as this was a non-neutral beneficial mutation, that means that going from 0, 1 back is going to be a non-neutral deleterious mutation. So the probability of fixing it in the back direction is not 0, but it's exponentially suppressed. I think it's very important to understand all the different pieces of this kind of puzzle, because it incorporates many different ideas that we've talked about over the last few weeks. If there are questions, please ask now. Yes.
AUDIENCE: What about the [INAUDIBLE]? [INAUDIBLE] 0, 0 to 1, 0 to 1, 1? Then it seems like the benefit of 1, 0 versus [INAUDIBLE].
PROFESSOR: All right, so you're wondering about-- so the fitness of the 1, 1 state was 1.2. So you're pointing out that it's actually easier to go from the 0, 1 state to the 1, 1 as compared to the 1, 0 to the 1, 1.
AUDIENCE: Right, which seems like a reason for why we wouldn't care about [INAUDIBLE].
PROFESSOR: Yeah, OK, right. So if anything, in some ways, this actually provides a bias going towards the 0, 1 state, because it's saying that if we do get to 0, 1, it's actually easier to move forward as compared to this other path. In practice, it doesn't actually matter, because this acts as a ratchet.
Because all these mutations are non-neutral, once you fix this state or this one, you can't go back. So the population will move forward once it gets to one of those two states. Now I mean, it would be a very interesting question to ask if we instead did a different arrangement. What would the rate of evolution be, and so forth?
Yeah, but what you're saying is certainly true, that if this took up all of the benefit going here, then it may not actually be somehow an optimal path in terms of the rate of evolution or something like that. I'll think about that when designing. Problems.
AUDIENCE: In this system, 0, 0 eventually becomes 1, 1.
PROFESSOR: That's right.
AUDIENCE: So the probability is 1.
PROFESSOR: That's right, so we are guaranteed that we will eventually evolve to this peak in the fitness landscape. And so what we're asking here is which of these two paths is going to be taken.
AUDIENCE: Yeah, so how to mathematically prove that the system will go from 0, 0 to 1, 1?
PROFESSOR: I mean, I feel like I kind of proved it, although I understand that nothing I said was rigorous. And of course, there are non-zero probabilities of going backwards. It's just that they are reduced.
And actually, you can prove, for those of you who are interested in such things, that over long time scales, there's going to be an equilibrium that distribution over all these states, where the probability of being in a particular state will-- it goes as the fitness. It scales as the relative fitness to the Nth power.
So we talk about these fitness landscapes as energy landscapes. And indeed, in this regime where you have small mutation rates, then it's going to be a detailed balance. And it's actually a thermodynamic system.
So then in that case, you can make a correspondence between everything that we normally talk about, where fitness is like energy and population size is like temperature. So the relative amplitude of being in this peak as compared to the other states is going to be, in this case, the ratios of those things is, indeed, described by the ratios of the fitnesses. And it's going to go as kind of like 1.1 to the 1,000th power, which is big. Which means that the population has really cohered at this peak in the finished landscape. Yeah.
AUDIENCE: So if you want to calculate a problem going from 0, 1 to 0, 0, then [INAUDIBLE] that would just be-- I guess I'm not sure.
PROFESSOR: OK, you want to know the rate that that's going to happen.
AUDIENCE: Yeah.
PROFESSOR: No, that's fine. Let's do that. So for example, let's imagine that we don't have-- so let's imagine that we just have the 0, 0 and the 0, 1 states, just so we don't have to worry about going up the landscape. And so what we have is we have r is relative fitness 1 and 1.02.
Now what we want to do is we want to ask, well, what is the rate of going back and forth? Well, so the rate of going forward, well, we sample mutations at a rate mu. And this is mu only for this one state, because pretend that we're not going to mutate this other one. So rate mu N, you have mutations appearing. And times this s, 0.02, is the probability that it'll actually fix in the forward direction.
And now what we want to know is the rate of coming back. Well, the beginning part's the same, because we have mu N is the rate that you get this deleterious mutant in the population. But then we need to multiply it by the probability of fixation.
And the probability of fixation is-- there was this thing X1, which was 1 minus-- now this is r, but it's r in the other direction, so be careful. Because the general equation was 1 over. But now r, instead of being 1.02, is 1/1.02.
So which of these terms is going to be dominant? This thing gets up to be some really big number is our problem. So we should be able to figure this out, though. Because this new r is 1/1.02. So we for example, have 1 minus 1.02, 1 minus 1.02 to the 1,000. All right, so this is a negative number, but this is a negative number, too. So we end up with 0.02--
AUDIENCE: 200.
PROFESSOR: Is it 200? Yeah, you're keeping only the first, which, since it's much larger than 1, it's bigger than 200. Right? I mean do you guys understand what I'm saying? You can't keep just the first term in a series. If the terms grow with number.
AUDIENCE: [INAUDIBLE] squared, 3.98 or something.
PROFESSOR: Wait, which one?
AUDIENCE: 1.02 to the 1,000.
PROFESSOR: It's 4? OK, all right. OK, so it's 1 minus 4. So it's 2/300. OK, so this is teamwork, right?
OK, so there's less than a 1% probability of it fixing. Is this believable?
AUDIENCE: It's about right.
PROFESSOR: 2, 1,000, 50-- I think that you did it 1.02 to the 100 rather than 1.02 to the 1,000.
AUDIENCE: OK.
PROFESSOR: No? Do you not have it in front of you?
AUDIENCE: No, it's 3-- [INAUDIBLE].
AUDIENCE: 4 times 10 to the 8.
AUDIENCE: I never thought that my calculator would become so controversial.
AUDIENCE: Oh, 4 times 10 to the 8.
PROFESSOR: Yes, sorry, I was just saying this doesn't-- so this is why I'm saying you always check to make sure that your calculation makes any sense at all. So it's not this. But it's tiny, right?
AUDIENCE: Yes, [INAUDIBLE].
PROFESSOR: Yeah, because this didn't make sense, because this was of the same order as-- well, this would be larger than 1 over N, so it's totally nonsensical. Because 1 over N would be the probability of fixation of a neutral mutation. This is a deleterious mutation. It's not even nearly neutral.
So it has to be much less than 1 over N, right? So this whole thing is 10 to the minus 10, or something like that? OK, 4 times 10 to the minus 8. Well, OK, whatever. It's 10 to the minus 9. It's something small.
So this times the probability of fixation, which is 10 minus 9-- this is how you would calculate the rate of going backwards. There's some rate that the mutation appears, and you multiply by the probability that it would fix. And it's tiny. OK? All right, any other questions about how to think about these sorts of evolutionary dynamics with presence of mutation, fixation, everything? Yeah.
AUDIENCE: Can we handle a situation where [INAUDIBLE] interference is important at this point?
PROFESSOR: Yeah, so this is what you do in your problem set with simulations. Yeah.
AUDIENCE: [INAUDIBLE] numerical.
PROFESSOR: You know, I think that it gets really messy with clonal interference, I'll say.
AUDIENCE: But, like, with basic-- I guess I was thinking about it and you could probably imagine that [INAUDIBLE] calculate the probability that 1, 0 doesn't arise first.
PROFESSOR: Right, yeah, OK, this is an important statement. In the limit, as you get more and more mutations, when clonal interference is really significant, then you're pretty much just guaranteed to take the 1, 0 path. Because if you have many mutants, the definition of clonal interference is you have multiple mutations that have established. And once you have multiple mutations that have established, then it's likely that one of them is going to be this.
And if it's established, it's going to win. But the other thing is that as you go up in the mutation rate, you don't even do successive fixations. So it may be that neither state ever actually fixes, because it could be that the 1, 0 state is growing exponentially, but is a minority of the population. And it gets another mutation that allows it to go to 1, 1.
So as you increase the mutation rate, you don't have to actually take single steps. You can kind of move through states. And there's a whole literature of the rate at which you cross fitness valleys. So this is like tunneling in quantum mechanics or so.
And it has a lot of the same behaviors, in the sense of exponential suppression of probabilities as a function of the depth and the width of the valley you're trying to traverse. And there's some very nice papers, if you're interested in looking at this stuff. And one of them is actually in the syllabus that I mentioned. I'm trying to remember who. It was Journal of Theoretical Biology, but I put it on as optional reading for those of you who are interested.
All right, OK. So what I want to do now is I want to switch gears, so we can think about this evolutionary game theory business. And I think the most important thing to stress when thinking about evolutionary game theory is just that this point that we don't need to assume anything about rationality. Because the puzzles that we like to give each other in your dorm rooms Friday night, you give these logic puzzles to each other. Is that-- I don't know.
[LAUGHTER]
OK, let me just say, back when I was in college, that was, like, all the cool kids were doing it. But in these puzzles, you assume that there's hyper-rationality. You assume that if this guy knows that I did this, and that, and if I did that, he would do that. And then you end up, and then you have the villagers that are jumping off cliffs on the seventh day. Have you guys done these puzzles? No? OK. All right. Well.
[LAUGHTER]
So the point was that people assume that when we're talking about game theory, you have to invoke this hyper-rationality even humans don't engage in. And I think that it's just very important to remember that we're talking about evolutionary game theory in the case of, well, biological evolution. You don't assume anything about rationality.
Instead, you simply have mutations that sample different strategies. And then you have differences in fitness that just lead to evolution towards the same solutions of the game. So it's evolution to the game solutions, so the Nash equilibrium, for example.
So it's not that we think that the cells are engaging in any sort of weird puzzle solving. Instead, they're just mutations. And the more fit individuals spread in the population. And somehow, you evolve to the same or similar solutions, to these Nash equilibria in the context of game theory. And we'll see how this plays out in a few concrete examples.
Now, there are always different ways of looking at these games. One thing I want to stress, though, is that all the selection that we've been talking about in the last few weeks, that all is consistent with game theory in the sense that the idea of the game theory is that we allow for the possibility that the fitness of individuals depends upon the rest of the population. Whereas in all these calculations we've been doing, I told you, all right, I just gave you some fitnesses.
So I said, here we have a 0, 0 state that has some fitness. 0, 1 has a higher fitness, and so forth. But in general, these fitness values may depend upon what the population composition is. And in that situation, then you want to use evolutionary game theory. In many cases, people just assume that you can do something like this-- that you can describe it as some fitness landscape. But you can't do that if there's this frequency-dependent selection-- if there's any sort of evolutionary game interactions going on.
So it's just important. If the fitnesses depend on composition-- this is the population composition-- then you cannot even define a fitness landscape. Then no fitness landscape. For example, you can have situations where the population evolves to lower fitness.
So you can have a situation where, if I tell you individual 0, you measure its growth rate, whatnot, its fitness might be 1. So this is genome, and this is fitness. Now, if I go and I measure the fitness of some other individual, different genome-- so another strain of bacteria or yeast or whatever-- and you say, oh, well, its fitness is 1.2.
So this strain has higher fitness than this strain. Now, it would be very natural to assume that this strain will out-compete this strain. And indeed, that's been the assumption in everything we've been talking about.
But it's not necessarily true. And that's the basic insight of evolutionary game theory, is that just knowing the fitness of a pure population is not actually enough information to know that it's going to be selected for. Because it's still possible that in a mixed population, the genome 0 may actually have higher fitness than the genome 1.
And once you kind of study these things, it's kind of clear that it can happen. But then it's easy to then go back to the lab and forget that it's true. And so we'll see how this plays out.
AUDIENCE: On this game theory, [INAUDIBLE]?
PROFESSOR: No. That's the other thing, is that I like to just draw these things as graphs, because I think it's much easier to see what's happening. And it's clear that things can be non-linear. But the basic insights are all intact.
From my standpoint as kind of an experimentalist-- don't forget about the exam-- I think that the more formal evolutionary game theory thing-- these two-player games that you guys just read about-- I think they're important because they tell you what are the possible outcomes of measurements or of systems, even in the most simple situation where everything's linear. Now, when things are not linear, of course you can get even richer dynamics. But in practice, you basically get the categories of outcomes that we saw there.
So maybe what I'll do is-- so what we're going to do is think about competition between two individuals A and B. And often, we talk about these things in the context of the two-player games, where we have A, B, C, D. And because this is really importing the kind of approach, or the nomenclature, from conventional game theory, and then immediately applying it to populations where you just assume that all the individuals have equal probability of interacting with everybody in the population. So it's what you would get if you just had some two-player game like they study in game theory, but in a population of 1,000 or whatnot.
You just made a bunch of random pairwise interactions. You had them play the game. And then you had them do that again over time. And then this is the payouts that you read about in Chapter Four are kind of what would happen in that sort of situation, where everybody's interacting with everybody else with equal probability.
Now, remember the way that you read this is that, depending upon the strategy that these guys are following, you get different payouts. And normally what we say is that if this could be, for example, strategy one and two, strategy one and two. And this is telling us about the payout that the A individual gets depending on what he does, and depending upon what his opponent does.
Now, we're not explicitly saying what the payout to the B individual is, but we're assuming that this is a symmetric game, so you could figure it out by looking at the opposite entry. So if A follows strategy one, B follows strategy one, then individual A gets little a fitness, whereas B also gets little a fitness, because it's a symmetric game. So the case it's different is when we're in the diagonals.
And from this framework, you can see that there are going to be already a bunch of kind of non-trivial things that can happen, even in this regime where everything's linear. And the probably best well-known of these is this Prisoner's Dilemma, which is the standard model of cooperation in the field of game theory.
So there's a story that goes along with it. It's this idea that-- I'm sure you guys watch these cop shows, where you have the cops bring in the two accomplices. And then they put them in separate rooms. And they tell them that they have to confess to committing the crime, because the guy in the other room is confessing, and if he doesn't confess, then he's going to be in trouble, et cetera. You've seen these cop shows?
And incidentally, in these questions, when cops are questioning witnesses, they're actually allowed to lie to the person being questioned, which feels a little bit weird, actually. Doesn't it? I know, I know, this is not relevant.
So the idea of the prisoner's dilemma is that if you set up these jail sentences in the right way, then it could be the case that each individual has the incentive to confess, even though both individuals would be better off if they cooperated. And you can come up with some reasonable payout structure that has that property. And we'll call this-- so this is for individual one, say and individual two.
So there are different strategies you can follow. And do you guys remember from the reading slash my explanation how to read these charts? All right, now the question is, just to remind ourselves, what is the Nash equilibrium of this game?
And I know that you read about it last night. Well, use your cards. Is it C or is it D? Or is there no Nash equilibrium, you can flash something else.
AUDIENCE: Are those negative or positive?
PROFESSOR: These are positive fitness. I kind of don't like the Prisoner's Dilemma as a story, because it's not very intuitive, because you have to actually specify the jail terms, and you have to remember that jail terms are bad, not good. So these are good things, OK? These are years off that you get as a result of doing one thing or another. You want to get big numbers. Ready? Three, two, one.
So at least we have a majority that are D, but it's not all of them. And I think this is basically a reflection-- and D is indeed the Nash equilibrium. It's to do this strategy D that we're saying here. All right, now the question is why? And part of the challenge here is just understanding how to read these charts.
Now, first of all, the payout that everybody gets if everyone follows strategy D is what? Verbally, three, two, one.
AUDIENCE: One.
PROFESSOR: So everybody gets payout one. Now, if you look at this chart, you say, well, gee, that is a shame. Because 1 is just not the biggest number you see here.
And indeed, the important point to note here is that if both players had followed this strategy C for cooperate, D for defect, then both individuals would be getting fitness 3, or payout 3. So the idea here is that both individuals would do better if they both played strategy C. But the problem is that that's not evolutionarily stable. Or in the context of game theory, that is cheatable in some ways.
And so the reason that this is a Nash equilibrium is that you ask-- so a Nash equilibrium, what it means, if you recall, is that if everyone's playing that strategy, then nobody has the incentive to change strategy. So no incentive to change strategy. So now you just imagine, let's say, that you're playing against somebody else, or in the context of biology, it's a population of individuals following the D strategy.
The question is whether you as an individual would have the incentive to switch to the other strategy? And the answer is no, because what you have control over is this rows. The column is specified by the rest of the population.
So if you're in this state, what you have a choice of is to switch to the cooperate strategy, which would be to go up here. So you have a choice to move up to this 0 payout, but that's not to your advantage.
Now, it's true that your opponent would get payout 5. So you opponent would actually do wonderfully. But you would do poorly.
So you'd be selected against, if you imagine this being in the context of biology-- that you have a genotype that are playing D. If you're a mutant that starts following this strategy C, you have lower fitness, so you're selected against. So that's saying that the strategy D is noninvadable.
We can also think about what happens if we're a population of cooperators. Now everybody has high fitness-- fitness 3. Question is, what happens if there's a mutation that leads to one individual following the D strategy? Is he selected four or not?
AUDIENCE: Yes.
PROFESSOR: Yes, so the point here is that you always will have higher fitness, regardless of what your opponent does in the context of a game theory situation, or regardless of the distribution of cooperation and defection in the population. It's always better to be a defector. So the problem here is, it's always better to play D.
Now, I really like drawing the graphs of these things, because I think it's just much more clear. And you can either draw the fitness of the two types minus each other, or just the raw fitness. Yes.
AUDIENCE: So what if instead of 5, you have 7? Because then the population as a whole's fitness decreases when you [INAUDIBLE]. So how does that--
PROFESSOR: So you're saying if this 5 were a 7 instead?
AUDIENCE: Yeah.
PROFESSOR: Right, then what you're saying is that-- so it doesn't change. The Nash equilibrium is still defect. The subtle thing here is that, in general, in terms of game theory, we like it when the mean of these two is smaller than this one.
That's why you're asking, right? Exactly, because that's right. Exactly, right. So yeah, so that's a slightly more complicated situation, because in that situation, then, if you had two rational agents, say, playing this game, then you could alternate cooperation and defection.
And that would actually be the ultimate form of cooperation in such a game, because you could actually get a higher payout by alternating. Right, so we've chosen the numbers as they are so that this is subtlety is not an issue. Does everybody understand the issue there?
So in the context of evolutionary game theory, what we can do is we can plot as a function of the fraction of the population that's cooperator between 0 and 1, say. And we can plot the payout for the cooperator and for the defector. For example, I'm going to draw a solid line for the cooperator, dashed line for the defector.
Now the question is, what should be the y-axes on either ends and so forth? Do you understand? So what should these things look like?
I'd like to encourage you to-- I'll give you 30 seconds to try to draw what this should look like. So this is the payout or the expected payout. So we're assuming that you're going to interact randomly with the other members of the population as a function of the fraction cooperator. So then 1 minus that will be the fraction defector. Do you understand what I'm trying to ask you to do?
AUDIENCE: So the scale on the right-hand side supposed to be for the defectors? [INAUDIBLE]?
PROFESSOR: This is just a legend, or key, or something. So I want you to draw something over here that's a solid line and a dashed line.
AUDIENCE: All right, so it's just one scale. And you don't--
PROFESSOR: It's one scale.
AUDIENCE: [INAUDIBLE].
PROFESSOR: Oh, yeah, sorry. I'm just telling you what's going to be a solid line and what's going to be a dashed line. And I'll give you a hint, that up here is number 5.
This is going to be the expected payout for a lone individual given the rest of the population is following some fraction of cooperator.
Do you guys understand what I'm asking you to do? Because I'm a little bit concerned that there are very few plots in front.
AUDIENCE: What is fc?
PROFESSOR: So this is the fraction of the population that's cooperator.
Well, I was giving you a chance to think about it. But from looking around, I think that maybe you're not quite sure what I'm trying to ask you to do. So I'm trying to plot the expected payout for an individual that is either cooperating or defecting, based on the fact that the rest of the population has some composition between all cooperate or all defect.
So it's the evolution game theory extension of this simple model. So first, we can ask, well, if the entire population is cooperating, we want to know the fitness for a cooperator or defector. Well, this is really just saying that we're all the way over on here, and we just choose between the two. And the defector is the 5 one.
So this is going to be a dashed line that's going to start from here. And then this is 2 and 1/2, 3. So this is cooperator starts here, and defector starts here. Do you understand what I'm?
Now, OK, let's see. So now this is the one where if everybody else is defecting, well, now, the cooperator line goes to what? Verbally, three, two, one.
AUDIENCE: 0.
PROFESSOR: 0. The defector line goes to 1.
OK, that line, I started going the wrong direction, but that's supposed to be a line. So this is an example of what this looks like for the Prisoner's Dilemma. And what you see is the defector fitness is always above the cooperator fitness. So for any population composition, defectors have higher fitness than cooperators. So evolution brings you to the pure defecting state, where you have fitness 1.
And if you want, you could calculate what the mean fitness of the population is, for example. And the mean fitness starts out over here, and ends up over here. So the mean fitness decreases over time.
Now, you can imagine that in the simple, two-player models, all these are lines. But you can imagine that the only thing that's important are how these lines cross each other. So for example, there are only a few different things that can happen.
You can have one strategy that dominates, which is what occurred here. And surprisingly, that does not mean that that strategy is higher fitness, in the sense that you may evolve to a state of low fitness. That's what's weird. You can have coexistence, or you can have bi-stability.
So I'll give you another example of this. So now we're just going to have two strategies. The strategies-- we'll just call them A and B.
And the question is, what is the Nash equilibrium? Is it A, B, or C should be neither, D is both. Do you understand?
I'm going to ask, because if this is the game and this is the interaction, is the Nash equilibrium A, Nash equilibrium B? If you vote C, it means neither, D means both. Do you understand the question? I'll give you 30 seconds to think about it.
All right, are we ready to vote? Ready, three, two, one. All right, so we have a fair distribution. I may not have us vote, but yeah, in this case, they're actually both Nash equilibria.
So let's see this. If both individuals, or an entire population, say, is playing A, they're getting fitness 5. Question is, as a lone individual, you can choose to switch over and get fitness 3. Do you want to do that? No.
So that means that A is going to be a Nash equilibrium. Incidentally, the difference between the so-called regular Nash equilibrium and the strict Nash equilibrium is that Nash equilibrium means that no individual has the incentive to change strategy. A strict Nash equilibrium means that any change in strategy leads to an actual decrease in fitness. So it's a question of whether you can make neutral changes in strategy or not. Do you understand?
So A is a Nash equilibrium. What about B? Well, in that case, everybody's getting to fitness 1.
Now, as a lone individual, what can you do? All you can do is switch. As an individual, you can only choose rows. So you go up to 0. That's a decrease of fitness. So that means that strategy B is also a Nash equilibrium.
So there are two Nash equilibria in this game. And what does that mean about which regime you're in here, if you convert this into an evolutionary game theory scenario?
Ready, three, two, one. OK, so a majority is saying yes. This is indeed a situation in which you have bi-stability. So what does that mean in terms of these lines if we draw them?
So this is payout as a function of the fraction that is playing the A strategy. Should the lines cross? Yes or no? Ready, three, two, one.
AUDIENCE: Yes.
PROFESSOR: Yes, and indeed, in principle, the math that we do in all these situations is kind of super simple. Yet it's easy to get confused about what's going on in all these situations. So the idea here is that if the population is A, that means that the A here is at 5. But then it goes down to 0. Whereas over here, B here is 3. And then it goes to 1.
Because these two lines cross, does that mean that you have bi-stability? Ready, yes or no, three, two, one,
AUDIENCE: No.
PROFESSOR: No, and why not?
AUDIENCE: [INAUDIBLE].
PROFESSOR: That's right, because you can also do the other thing, and then that leads to coexistence. Now, in some ways coexistence is the most subtle of the situations. And that's for an interesting reason.
AUDIENCE: Sorry, sir, you said you can also do the other thing. What is the other thing here?
PROFESSOR: I'm saying that these things can cross in the other orientation. Let me put a matrix out there, and then-- so this is something that, for example, is what's known as a Hawk/Dove game. Or it has many other names.
And we can maybe figure out what would be the Hawk strategy, and what's the Dove strategy. Now, we want to ask the same question-- is A a Nash equilibrium? Is B a Nash equilibrium? Is it neither? Or is it both?
And maybe I shouldn't have covered this up, so you're not influenced, in case you actually did do the reading. Then I don't want to you to be influenced by this. So think about it for 30 seconds.
Do you need more time? Let's go see where we are. Ready, three, two, one. All right, so most of the group is agreeing in this case, neither are the Nash equilibrium. So neither are a Nash equilibrium.
Does that mean that this game has no Nash equilibrium? Yes or no, verbally-- ready, three, two, one.
AUDIENCE: No.
PROFESSOR: No, it does not mean that. This game has a Nash equilibrium. And indeed, all games like this have Nash equilibria.
And this is what Nash won the Nobel Prize for, so this is the famous one-page paper published in PNAS. If you look at it, I have no idea what it says. I mean, he basically just pointed out that this theorem implies this, implies that-- done. And so it's good that somebody knew what he was saying, otherwise we'd be in trouble, all of us.
So what he proved is that such games, even with more players, more options, and so forth, they always have such a solution in this sense. There exists some strategy such as if everybody were playing in, nobody would have the incentive to change strategy. But you have to include so-called probabilistic or mixed strategies.
And we can draw what this thing is. So just like always, so everyone else is following A, then A starts here at 3. And then it goes to 1. Whereas the B individuals start at 5, and they go to 0.
So this looks very similar to that, but they're rather different, in the sense that in this situation, we had bi-stability. So if you look at the direction of evolution, depending upon where you start, you go to either all B or all A. Whereas in this situation over here, we have coexistence. Does not matter where you start. So long as you have some members of both A and B in the population, you'll always evolve to the same equilibrium.
Now, the important thing here that's, I think, interesting is that in a population, if you have genetic A's and genetic B's that are each giving birth to their own type, then you evolve to some coexistence of genotypes. So here, this is some fraction. f a star is the equilibrium fraction, fraction of A in the population. So this is a case where you have genetic diversity that leads to phenotypic diversity in the population.
Whereas the mixed Nash equilibrium-- this is a situation where you have, in principle, genetic homogeneity. So this is a single genotype that is implementing phenotypic heterogeneity. And indeed, one of the things that we've been excited about exploring in my group is this distinction here, where it's known that in many cases, isogenic populations of microbes can exhibit a diversity of phenotypes as a result of, for example, stochastic gene expression and bi-stability.
So that's a molecular mechanism for how you might get heterogeneity. Another question is, what is the evolution explanation for why that behavior might have evolved? Now in general, we cannot prove why something evolved, but we can make educated guesses that make experimentally testable hypotheses.
And for example, in the experiment that we've been doing, we've been looking at bi-modality in expression of the galactose genes in yeast. And that was still a problem set in early-- oh, no, we removed that one this year. Well, so experimentally yeast, in some environments, bimodally or stochastically activate the genes required to break down the sugar galactose.
And what we've demonstrated is that if you make the mutants that always turn on or always don't turn on these genes, then they're actually playing game where you actually get this exact thing-- where you get evolution towards coexistence of those two strategies. So that's saying that maybe the wild type that follows this stochastic, mixed strategy-- it may be implementing the solution of some game that is a result of such frequency dependence. There are other possible explanations to this.
In the coming weeks, we'll talk about this idea of bet hedging-- that given uncertain or fluctuating environments, it may be advantageous for clonal populations to have a variety of different strategies to cope with that uncertainty. So we'll talk about those models later. But since we're talking about mixed strategies now, I wanted to mention that. Yeah.
AUDIENCE: So f a star, just to be sure, is going to converge to this probability [INAUDIBLE]?
PROFESSOR: Exactly, so the Nash equilibrium mixed strategy plays A with probability-- so it's p should be equal to f a star. Exactly, so indeed, the heterogeneity there can be implemented either way. It's either coexistence of genotypes following different strategies, or it could be one genotype implementing both, or it could be a mixture of those, actually. Indeed, a characteristic of these situations is that, let's say that you have a genotype-- a population has this genotype that is implementing the mixed Nash equilibrium choosing strategy A with probability p. That's that equilibrium fraction.
What's interesting is that any individual in the population following any strategy has the same fitness. And of course, that's kind of why this was in equilibrium. This equilibrium is when the two strategies have equal fitness.
But the funny thing is, what that means is, it doesn't matter what you do at the equilibrium. Depending on how you look at it, it's either super deep or super trivial. But it's a weird thing that if your at the equilibrium, or if the population or the opponent is playing this Nash equilibrium in these games, then it just does not matter what you do.
You can do A. You can do B, actually, in any fraction. So since A and B have the same fitness, you can choose between them at any frequency you want, and you have the same fitness, if the rest of the population is playing this mixed Nash equilibrium.
And indeed, in this there are nice conditions for what makes it this Nash equilibrium. And I'm going to just highlight that you should make sense of why it means what it is. So if the payout, the expected fitness or payout-- if you're following the Nash equilibrium against Nash equilibrium-- is equal to this guy.
So that's what I just said-- that it doesn't matter what you do. If everyone else is doing p star, you have the same fitness. So that's saying it's a Nash equilibrium.
Whereas there's another interesting kind of statement here, that--
AUDIENCE: [INAUDIBLE]? That you can't unilaterally increase your fitness by switching.
PROFESSOR: Right, it's an equality, which means it is a Nash equilibrium. Because it's saying that you don't have the incentive to change strategy. It's true that you're not dis-incentivized. So it's a Nash equilibrium. It's not a strict Nash equilibrium.
AUDIENCE: [INAUDIBLE].
PROFESSOR: Well, it has to be greater than/equal to, and it's actually equal to. The condition it has to be greater than or equal to, but it's equal to, which means it is a Nash equilibrium.
AUDIENCE: [INAUDIBLE] Nash equilibrium for that situation, [INAUDIBLE] strategy.
PROFESSOR: That's right. So this is not the definition of that. But this thing is true, which means that it's a Nash equilibrium.
And this other thing that's interesting is that-- so this tells us that it's actually one of these ESS's. And if you have questions about this, I'm happy to answer it. It's explained in the book as well.
We are out of time, so I should let you go. But good luck on the exam next Thursday. If you have questions and you want to meet with me, I'm available on Tuesday. So please let me know.
Free Downloads
Video
- iTunes U (MP4 - 180MB)
- Internet Archive (MP4 - 180MB)
Subtitle
- English - US (SRT)