Flash and JavaScript are required for this feature.
Download the video from iTunes U or the Internet Archive.
Description: In this lecture, Prof. Jeff Gore discusses bacteria. He asks, and answers, questions like how do bacteria find food? How do they know which direction to swim, and how do they swim? All of these questions relate to the low Reynold's number regime in which bacteria live.
Instructor: Prof. Jeff Gore
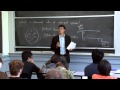
Life at Low Reynold’s Number
The following content is provided under a Creative Commons license. Your support will help MIT OpenCourseWare continue to offer high quality educational resources for free. To make a donation or view additional materials from hundreds of MIT courses, visit MIT OpenCourseWare at ocw.mit.edu.
PROFESSOR: So today, the general theme is going to be to try to understand how bacteria find food. And in particular, how do they know which direction to swim? How do they swim given that they're operating in this low Reynolds number regime that you read about maybe last night? What did you guys think of the life in low Reynolds number?
Yeah, it's kind of fun. You very much get the impression that you are kind of there in that lecture hall with him. They do say that some essential arm waving was not reproduced or so, right? But you kind of imagine his arms waving as he talks, even though you don't get to hear him talk.
So that's fun. And it's a surprising number of typos given that-- every time I read that, I'm like, somehow this is still the best version that I've been able find of that talk. But I think it's just a neat example of how clearly a talented physicist can kind of from a naive perspective say interesting things about the way that life has to work.
Now today, so we will be talking indeed about how bacteria are swimming. But we want to start by just making sure we understand something about how diffusion works, what life is like in this low Reynolds number regime. And for these sorts of problems, it's really very, very valuable if you can just apply some simple dimensional analysis ideas. And so we're going to practice that in a few different cases, that dimension analysis can often tell you a great deal.
In many cases, you can discover new physical laws. You can figure out how things have to scale. And in some cases, the scalings are rather surprising. And given some of the constraints that microscopic life is facing in this low Reynolds number regime where diffusion is very important, viscous forces are dominating over these inertial forces, it really constrains what bacteria, for example, are able to do. And it really constrains how they have to go about solving the challenges of life.
So what I want to do is start by thinking about just a very kind of simple situation, which is we have in, we'll say water, a non-permeable membrane that is separating two compartments. Whereas over here, we have maybe salt or something on the right. So this is a salty solution. And this is pure water.
Now, this is a non-permeable membrane. So it doesn't allow the salt to cross. But the question is, what happens if I puncture, and I put a little hole in the membrane of some radius a, let's say? Now, the question is, what will be the flow rate, the net flow, of salt, in this case, from the right to the left?
All right, so first of all, can somebody kind of answer at least for a couple-- what are possible things that might be relevant if we're going to try to figure this out? What quantities will we need to know or measure?
AUDIENCE: Concentration.
PROFESSOR: Concentration seems relevant, yeah. And in this case, there's only one concentration. Because well, this conservation is 0 here, and some concentration c over here. What else might we need to know?
AUDIENCE: Pore size.
PROFESSOR: The pore size, perfect. We'll use a as the radius of the pore. And is that going to do it?
AUDIENCE: You need a time for something.
PROFESSOR: We need a time. Because what we want is some number of molecules per unit time that is going to be crossing from the right to the left. So we need something that has a time. Yes.
AUDIENCE: The diffusivity.
PROFESSOR: All right, the diffusivity. So D, so we'll say D, which is the diffusion coefficient. How many S's? All right, now other things that might be relevant?
AUDIENCE: Temperature
PROFESSOR: Temperature, OK.
AUDIENCE: I guess it's probably going to be incorporated into the [INAUDIBLE]
PROFESSOR: Exactly, right, so indeed the flow rate will be dependent upon the temperature. But the way that that manifests is by doing what to D? In general, if we go up to higher temperature, how will D change?
AUDIENCE: Should get bigger.
PROFESSOR: Should get bigger, yeah, so indeed, D will be typically given by this Einstein relation of kT over gamma. We'll talk more about this in a little bit. But for now, let's just say that D is something that we've measured. Now, other things that may enter into the expression?
All right, so this is pretty simple. We have three things. Now, the question is, if we double the size of the pore, i.e. if we double a, how does the flow rate change? So we'll go ahead and do a vote, since we like to do this. All right, so we double a. The flow goes up by what factor? All right, maybe not all. All right, and if you think it's something else, you can splay your cards out.
Now, the idea here is that using dimensional analysis, we should be able to figure this out. Now, I'll give you just a minute to try to think through this.
AUDIENCE: [INAUDIBLE]
PROFESSOR: All right, so you don't even need to know this in principle. I'm going to give you something else that may help.
AUDIENCE: Will it be by x?
PROFESSOR: Yeah, so this is the mean squared distance that something will diffuse. So I wasn't explaining, because I was sort of-- a hint in the sense that in case you might remember something like an equation like-- Yes.
AUDIENCE: Are we doing this at the very beginning of--
PROFESSOR: Yes, right, OK, so there's going to be some question of timescales here. OK, so it's really that after you puncture the hole, very rapidly it's going to assume some kind of steady state. And then the flow rate will be constant for some rather long time.
But then eventually the concentration on this half will decrease. So we're thinking about the flow rate after you've reached that steady state, but before you've equilibrated across the two sides of the tube. All right, do you need more time? All right, let's go ahead and see where we are. Ready-- three, two, one.
All right, so we have a pretty good agreement. It seems that it's D. But didn't I tell you how to do the problem already? OK, so it's not--
AUDIENCE: So the naive thing, I guess, would be C. Because-- oh, sorry, never mind. Except for-- [INAUDIBLE]
PROFESSOR: All right, so I'm going to give you another-- collaborate with a neighbor. And I'll tell you that it's not D to nudge you in the right direction. So spend a minute.
[INTERPOSING VOICES]
PROFESSOR: All right, let's go ahead and reconvene. All right, I just want to see where we are now. So if you still believe it's D despite my telling you that I don't think it's D, you can still vote D, and then we can argue about it. And that's fine, too.
All right, ready-- three, two, one. OK, so now there's maybe a disagreement between A and C now. All right, well, nobody liked my root 2? Maybe you saw me hesitate a little bit before I wrote it. I was like, oh, let me throw in a root.
So indeed the flow rate does not scale as the area. It only scales with a linear dimension of this hole. This is weird, which is why everybody says D. And even after you've done this problem, you still think it's D in your intuition. But you have to like pound that intuition away from you. We'll try to understand in multiple different ways why this might be. But what I want to do first is just make sure that we understand from dimensional analysis why this has to be the case.
Now, the first thing you need to know for dimensional analysis is the units of your answer. We're looking for something that is going to be units, some number of molecules over time. Number-- there's no units attached to that. So the answer is supposed to be just 1 over time.
Indeed, this is why we needed something that had some unit of time somewhere in order to get an answer that could possibly do this. And indeed, we know that the diffusion coefficient has units of, for example, microns squared per second. So it's a length squared over time.
Now, the one barrier that people have to use dimensional analysis is because we have trouble remembering what the units of things are. So if you don't remember something like the units of a diffusion coefficient, then what you have to do is try to find some equation in your brain where that symbol is used. And of course it should be the correct use of the symbol.
So here this is why I'm saying it. This is essentially the definition of this linear diffusion coefficient. And from that, you get the units. This is just very, very common. Something like viscosity, impossible to remember what the units of that thing are. But then you just have equations in your brain where the viscosity enters, and you can figure it out.
All right, so then if you want to use this dimensional analysis study, just be very clear. Write down what all the dimensions are. And then the answer just comes screaming out at you in general. Now, we see that we want something 1 over time. There's only one of these symbols that has a time in there. So we know exactly how the diffusion coefficient has to enter.
We know that the flow is going to have to be something where we know that D has to enter in there linearly. Because if it was any other way, then the time would have some weird units. It would be time squared or square root of time or something.
Now, it's true that we could in principle dream up something crazy between concentration and the pore size in the sense that if we wanted to, we could do c times-- we could always add a c times a cubed or something like that. But of course this is diffusion.
What that means is that these are non-interacting diffusing molecules. That means that if we double the number of the molecules over on the right hand side, we have to double the flow rate in this diffusion equation. And for diffusion, these molecules are not interacting at all. They don't see each other. And that means if you just double the concentration on the right, you have to double the flow rate.
So that means we know that it has to show up as a single c. And all we're left with is going to be one unit of length that we have to get rid of. Now, this is not, of course, a rigorous definition. And does this have to be actually an equal sign? No, so what we can actually say is that it has to be proportional to this. But actually just from dimensional analysis and this idea of, say, superposition of the concentrations, you can get something that's really kind of a deep statement about how diffusion is going to operate. Yes.
AUDIENCE: It seems to me that we can break that. There's this degeneracy. We can't quite sort out the powers of c and a, right?
PROFESSOR: Right, so that's what I was saying, that just from the units alone, we could always add a c times a cubed, or to some power. And that's why I'm saying that you have to be able to invoke this idea that if you double the concentration of the salt on the right, you'll double the flow rate. Because these are non-interacting molecules. Because they're just diffusing around in solution.
AUDIENCE: I was going to say that if we simply chose the measure of flow in 90 units of number of atoms, but in units of mass per unit time, and concentration in units of mass per unit time, then we would clearly see what power of c it would be. And it would have to be 1.
PROFESSOR: It's an interesting-- I think I'm going to disagree with you. But I want to make sure that I-- so you want to say, OK, you're entering. Yeah, the diffusion coefficient is about what the molecule is doing. So I'm not sure how we're going to-- if we measure concentration in units of, you're saying, mgs per mil of some protein, or so, then you're really saying that there's some mass density.
And I guess you can always define diffusion coefficient, even from the standpoint of-- because we don't even need numbers. We can measure some fluorescence or something of how it diffuses across. So that doesn't require numbers. Yeah, I'm trying to think if this is going to end up being different from the argument. I'd have to--
AUDIENCE: If we put in the number of molecules, then it's going to have to be number of molecules per time. And then c has to be linear. Because c is number of molecules per something, right?
PROFESSOR: OK, yeah, you could, say, treat molecules as a unit. And then that also ends up requiring that it's-- yeah, no, I think that's fair. But I'd have to think about this, how it would play out if you try to measure things in terms of mass per unit volume.
Of course, you should be able to calculate this explicitly, and then also to figure out what the proportionality constant is. But indeed, what you find is that it scales linearly with the radius instead of as the area, which is, I think, very surprising. Yes.
AUDIENCE: We can also say D scales with a squared times flow.
PROFESSOR: Oh, you're saying that maybe the concentration is not relevant.
AUDIENCE: If we look at it that way, then the answer is D.
PROFESSOR: OK, what you're saying is that maybe somehow the flow is just equal to or proportional. It goes as D times a squared in the sense that you would say that--
AUDIENCE: Over. Over.
PROFESSOR: Right, I think that this non-interacting particle business really does tell you that the flow has to be proportional to the concentration. And yeah, I think that is sufficient already. I agree that just from units, you could do this. But I think, well, it's not true, and it's nonsensical. And it's not proportional to c.
Let's move on. But I think it's important that it's possible to say something that's a deep kind of physical principle just based on analysis of the units. And then, of course, can somebody offer the intuitive explanation for why this might be? Why is it that it goes as a instead of a squared?
And this is a little bit weird given the fact that we all check our intuition, we all say D. But the thing is, once you know an answer, you should still try to figure out what the intuition might have been that you missed when you originally said D. Yes.
AUDIENCE: OK, this might not make any sense. So say you would first think it goes like a squared, because the area goes like a squared. But then maybe the fact that the perimeter grows like a, and the perimeter adds drag, maybe that would subtract from or cancel out any-- not necessarily make sense?
PROFESSOR: Yeah, well, OK, so I don't think that's the intuition that I would use. But then we have to figure out, OK, what might be wrong? Well first, I don't think you can subtract an a. But also we haven't invoked any actual drag in the sense that there's no sense that the perimeter should be-- right?
All right, so this is so mysterious that we are-- that's fine. So the way that I like to think about this is that it's not that we're shooting bullets at this membrane. If we were shooting bullets, or if these were raindrops coming, then indeed it would scale as the area.
But because it's diffusion, diffusion operates on gradients of concentration. And what's happening here is that you get a local depletion of the concentration at the pore. So it's not the case that you have concentration c all the way until you get to the pore, and then all of a sudden you have a linear gradient of c across that little pore, but rather that you have a depletion of the concentration as you approach the pore. And so as you grow the pore, you're somehow competing with yourself.
Another way to think about this is to think about what happens if we have two pores. For example, let's imagine that we have this poor here of size a. But now I'm going to add a second pore over here far away, same size. What should this do to the total flow rate, A, B, C, D, E? I'll give you 10 seconds to come up with an opinion. So I'm adding a second pore far away. Ready? Three, two, one-- all right, so we're all agreeing. And this should be 2x, again.
This is for two pores now. We also get 2x. This is because if the two pores are far away from each other, as relative to the size of the pores, then these are really non-interacting in the sense that there's none of this depletion effect that I was telling you about.
Whereas as we bring these pores together, then they start interfering with each other. Because they each involve some local depletion of the concentration. And once that kind of depletion area starts to overlap, then the point is that there is some molecule that was diffusing. And if you have the pores right next to each other, you're not going to get twice the net flow. Because that molecule, it might have diffused through the other pore even if it-- if one pore were not there, then it might have diffused through the other pore.
AUDIENCE: Can you not think of it as having to do with the fact that under a random walk, the displacement goes like square root of the time? So if you increase the number of molecules going through by the square of the perimeter, then actually be like total molecule displacement through [INAUDIBLE] will only go linearly in that perimeter, which would account for the fact that molecule is coming back across.
PROFESSOR: Right, so it's true that the molecules coming back across you always have to worry about. But I don't see this argument actually necessarily in my work.
AUDIENCE: Maybe in the case of, like [INAUDIBLE] which doubled in size.
PROFESSOR: Oh, I see. Yeah, I think that it may be connected, although I'm not sure if I'm sold on the argument. Yeah, I think it's a little bit dangerous. Yeah.
AUDIENCE: So you mentioned you appeal to some sort of depletion with that. But if you just punctured a hole in the--
PROFESSOR: OK, and this is why there is this question of timescale. Immediately after you puncture the hole, then the flow rate will actually be higher than it will be at that steady state.
AUDIENCE: So it's going to be a squared.
PROFESSOR: Yeah, right, I think that the flow right after you puncture it indeed will scale as a squared. Because what you're doing is you're taking the number of molecules across this area. And half of them are going to come through.
And there's just more molecules in that area when you first puncture it. But that's going to equilibrate in, whatever, a microsecond. That'll be really fast. Whereas in the steady state flow rate, that could go in principle forever if it's a large reservoir.
OK, so I want to move on. But I want to make sure that we understand how this basic principle allows us to say some interesting things. For example, there's a related problem, which is, how much food could a cell possibly eat? And can somebody say why there might be an upper bound on this?
AUDIENCE: There's a point at which you get too many nutrients inside of the cell.
PROFESSOR: I'm sorry?
AUDIENCE: There's a point at which you get too many nutrients inside of the cell.
PROFESSOR: Oh no, this is a super hungry cell. It can in principle be able to use every glucose molecule that came in.
AUDIENCE: No, but I'm saying that the osmosis of the water coming in would pop the membrane.
PROFESSOR: Oh, OK, we're talking about osmotic effects. OK, yeah, but we can just say that it's got a super tough cell wall. So it can take, we'll say, infinite. I guess what I'm saying is there's something. Even if I invoke such a thing, there still is a limit. That's really what I'm trying to say.
AUDIENCE: The concentration of the glucose inside the cell is a bit high.
PROFESSOR: Well, the glucose is going to get imported. And then it's going to be immediately converted into useful things. But then still there's a limit. There's a maximum. This is the maximum kind of possible nutrient uptake. Yes.
AUDIENCE: If the diffusion is somewhat slow, or maybe there's [INAUDIBLE] around the cell.
PROFESSOR: OK, right, so maybe it could be somehow diffusion limited. And what's the best that a cell could possibly do in this regard? So here's a cell. And now maybe we will, again, have it be radius a. So this is the cell of radius a. The best they can be, how would you describe that relative to diffusion?
AUDIENCE: Can you be more specific?
AUDIENCE: Everything that [INAUDIBLE] membrane could be absorbed.
PROFESSOR: That's right. So that's what I was-- so the best that it could possibly be is a perfect absorber. What we mean by that is something where the moment that a molecule touches it, it gets imported. Now of course, a cell is not a perfect absorber. But what's fascinating is that even if it were, then it does not mean that it's going to be consuming, say, glucose at an infinite rate.
And particularly, we can ask, well, if we have a situation like this where we have a cell, a sphere of size a, it's a perfect absorber, how will its uptake rate scale with its size? In particular, if we double a, what is it going to do for the flow? All right, we're going to have 10 seconds to think about this.
If we imagine a cell as a perfect absorber, the moment that this nutrient touches it, it gets imported and chewed up. The question is, how does that maximum uptake rate change if I double the size of the cell, double the radius? Are we ready? OK, let's see where we are. Ready? Three, two, one.
OK, so now it's a bit more of a mixture of A's, C's, D's, although it is a majority. We're getting a majority C. And again, this is, cell doubles size.
And indeed, from the standpoint of dimensional analysis, this is the same problem as that pore that we just did. Because again, it's diffusion. We're interested in uptake. Now, it's not flow across a membrane. But this is uptake rate by the cell. It's going to be numbers of molecules per unit time.
Again, there's diffusion. The concentration is still relevant. And so all the same arguments apply. And indeed, this is, again, proportional to the radius a. And remember, we're following the convention of Purcell and calling this a. Because Reynolds numbers is an r, and then problems arise. Are there any questions about why the argument is the same here as it was there?
OK, now, there's something interesting about this though, which is that you can imagine the fact that this only scales as the radius of the cell tells you that there's some sense in which we're wasting an awful lot of that surface area. Because we have this large surface area.
But it's not as good as you would have thought it would have been. And what that means is in principle, you could have a smaller fraction of the area covered by a bunch of little pores or transporters that are kind of analogous to this situation that we had before with the salt crossing the membrane.
And indeed, if you just imagine that these are each little absorbing patches that are perhaps one nanometer in size, small, there's an amusing calculation you can do, which is you can ask, well, how is it that the-- this is now uptake rate. We want to know, how is it that that is going to change as a function of the fraction of the surface area that we have covered with these transporters, with these little perfectly absorbing patches?
So we imagine the cell is 1 to 10 microns in size. Now we have a bunch of these little pores that we're going to go on. And of course, this is going to saturate somewhere. And it's going to saturate. And as it turns out, this goes as 4 pi D, I think. Yeah, so the uptake rate is actually equal to 4 pi cDa for a sphere.
So this is the part that we could not have figured out from dimensional analysis. So indeed, the uptake rate, once we get up to-- this is a fraction that's covered. Once that's 1, indeed it's going to be 4 pi cDa. Now, the question is, how does it behave over here?
Now, you might have thought that it would just be some line like that. It's just the more of these transporters that you have, you just get a linear increase in your total uptake rate. But it turns out that that's not true. Because even at just having, say, 1%, roughly, of the surface covered by this transporter, you can get something like 50% of the total maximal uptake rate. And that's because these guys are not competing with each other so much, because they're far away. So this curve really looks something like-- well, OK?
Now, this is, I think, especially interesting. Because a cell is not trying to import just one thing. In principle, it's trying to import many different things. So you need carbon sources. You need all sorts of trace metals. You need nitrogen.
So in principle, there are many, many, many things that a cell is trying to get from the environment. And by simply having different transporters specific to different things-- all right, so here are some squares that uptake something else, and little, I don't know, triangles, whatever. So you can have many, many, many of these different transporters on the cell surface. And they're each uptaking their own thing.
And kind of surprisingly, you can get near optimal uptake rates of many, many different things. I feel that I've lost you. Somebody ask a question. Yes.
AUDIENCE: So this [INAUDIBLE], is it really proportional to the actual surface area covered?
PROFESSOR: Right, so this initial thing, this increases linearly for small areas. Because just like what we said before, if you had these two little holes in the membrane that are far away from each other, then they don't compete. What that means is that you do get an initial linear increase here.
AUDIENCE: But I guess my question is sort of, how can we get that 50% of 1%? Is there a central calculation that you can do to get that, or [INAUDIBLE]?
PROFESSOR: Oh no, we can do it. Because actually, I think that the most straightforward way to do this is to ask, what is the absorbing rate of a little patch, let's say a circular patch? And we already figured out that when-- so these are very related problems, as you can kind of see. I just don't remember whether it's 2 pi or if it's 4 pi for that particular problem.
Because this is some uptake of a patch, we'll say. It's going to be either 1, 2 or 4 pi Dca. But there's some uptake rate from each patch. But then that means just that the uptake total for small numbers is indeed just going to be equal to the number of patches or transporters times the uptake rate of each one. Because they're just not competing against each other.
And the striking thing is that because of this difference in scaling between the radius and the area, this can give you a lot of uptake rate while only occupying a small fraction of the area. This is a fraction of area covered. Do you see why that ends up being the case? Yes.
AUDIENCE: So that's also saying that [INAUDIBLE] pretty soon.
PROFESSOR: Well, it depends on what you mean by soon. I mean, it's soon on the scale of the amount of area. But it could be that you can have 1,000 of them or so, right? So the way I would think about it is that you don't have to cover up very much of the cell surface in order to get really good uptake kinetics.
Now, these are some, say, fundamental limits to the way that bacteria, for example, and other cells can uptake food and other nutrients. But from the standpoint of trying to find where the nutrients are better, what you need to be able to do is you need to be able to measure the concentration.
Now, the basic way that you could, for example, measure a concentration of something, the best that you could do, is if you're a perfect absorber, you uptake molecules at this rate. And then you count the number of molecules that you uptake over some time t. Indeed, the number that you uptake over some time t will just be given by this.
What will be the distribution of these numbers? I should just have somewhere up on the side always a bunch of probability distributions that we can vote on. But think about it for a moment, and then we'll vote. All right, ready? Three, two, one.
What is going to be the probability distribution of the number of these things absorbed over some time? Right, it's going to be distributed Poisson. What is going to be the distribution of times between successive absorption events? Ready? Three, two, one. All right, yeah, that is going to end up being exponential.
And this is all confusing. So this is a Poisson process. These things are occurring at random times, at some rate. And if you have such a process that's random, and you ask, how many events over some time t, that is the definition of a Poisson.
If this is a large number, then the probability distribution is going to look like something else. What is it going to look like? Ready, three, two, one. Most people are trying to find the D, the central limit theorem there.
What is going to determine the resolution of that measurement that we make? So in some ways, what you would like to know is, well, what's the standard deviation in your measurement of the concentration divided by the concentration? So this is maybe a fractional error in your measurement of the concentration.
Do you guys understand why this is the error in the measurement? Well, this actually will go as the standard deviation in our measurement of the number divided by the number that we measure. What's going to be the standard deviation of this number?
AUDIENCE: The scale--
PROFESSOR: What's that?
AUDIENCE: [INAUDIBLE]
PROFESSOR: The mean, yeah, so it's close to--
AUDIENCE: [INAUDIBLE]
PROFESSOR: Yeah, it's the square root of the mean number. What that means is that this thing is going to be the square root of a 4 pi cDaT. And this is just 4 pi cDaT.
The number that you measure here, the number that you uptake over some time period t, that's going to be distributed as a Poisson. And in a Poisson, the variance is equal to the mean, which means the standard deviation, which is the square root of the variance. It's going to be this. Yes.
AUDIENCE: I'm not understanding the first step where you changed the c [INAUDIBLE].
PROFESSOR: Right, so from the standpoint of the cell, what the cell is doing is it's counting the number of these things that have come. And it's true that from that number of, say, molecules that are absorbed, to get a concentration of units of number per cubic micron and whatnot, the cell would need to know kind of how big it is, what the diffusion coefficient is, and so forth. But in terms of the fractional error, those all end up disappearing.
Because the fractional error is telling us, well, if this time I measure it, and I get 10, and next time I measure it I get 20, then this is telling us about how much error. This is indeed just the fractional error in our measurement of the concentration, and irrespective of whether the cell is able to estimate the concentration units of a physical quantity. Because that sort of error would scale together. I'm worried that I'm not helping you, though.
AUDIENCE: So you're kind of saying because c and n are linearly related, any proportionality factors would cancel out.
PROFESSOR: Yeah.
AUDIENCE: Would I be right to think that since n is distributed as a Poisson, so is c?
PROFESSOR: Yeah, our estimate of c would be-- because ultimately, what the cell would do is it would measure some number. And then it would say, all right, well, I multiply by all these things. And then that results in an error in c that is distributed in the same way as the error in n. But they're kind of proportional to each other.
AUDIENCE: What has me confused is I'm thinking that c is Poisson distributed, and then our original ratio that we want, sigma of c over c, should be 1 over square root of c, because c is Poisson itself. But then that's different from what we got.
PROFESSOR: Sure, OK, I think we have to be careful. So it's not that the c is actually Poisson distributed. Because it's really that you take a Poisson distribution and multiply it by something to get an estimate of c. But if you take a Poisson distribution and you multiply the numbers by 10, then it's no longer a Poisson distribution.
Because the mean goes up by 10, but the variance goes up by 100. So then the variance no longer equals the mean, so it's not a Poisson anymore. So you can't just multiply a number times a Poisson to get--
AUDIENCE: All right, thanks.
PROFESSOR: And this expression kind of makes sense in that the longer that the cell measures, the less error there is. If there's more diffusion or higher concentration-- less error. And this is really in some ways some estimate of the best the cell could do. And surprisingly, cells can get close to this.
Now, this is a measurement of the concentration for a perfectly absorbing sphere as a cell. Now, you might ask, well, how would our error change if instead of absorbing the molecules we simply had a detector? So they just asked, OK, each time that a molecule bounces against my cell, I count one? Do you understand the difference?
So this is for a perfect absorber. So what about a perfect monitor? And the question there is, does a perfect monitor do better or worse than a perfect detector? So we'll say, better, worse, or no change.
And it's of course not possible for you to actually do this calculation. But it's useful to imagine the situation and make your best guess-- better, worse, and same. I'll give you 5, 10 seconds to think about it. Because it's interesting to imagine the situation. All right, let's see where we are-- ready, three, two, one. Oh, we have a fair range of answers.
All right, I think everybody agrees it's going to do something. Because it would be kind of a coincidence if it didn't change things. Can I get somebody to volunteer what their neighbor is thinking? I know you didn't actually talk to your neighbor yet. But you can still invoke that. Yes.
AUDIENCE: So I don't know about this so much. But the first thing I was thinking is that maybe this monitor might be encountered on at a different rate proportional to its area. It seemed like it wouldn't change the concentration gradient, because it wouldn't be subtracting any. And so then things would bounce off of it, and that would be proportional to its area.
PROFESSOR: OK, that's interesting. So you're arguing for a different scaling. And then it would actually be either-- depending on the area, then it would be different.
AUDIENCE: Yeah, so that seemed weird to me, which is why I wanted to just check where other people were on that.
PROFESSOR: Yeah, no, that's fair. That's fair. In the end, the scaling is the same. Although this is very complicated, confusing. Can I hear somebody else argue for one or the other? Because he actually argued for both.
AUDIENCE: I just thought if it's not taking up the molecules, then it could bounce against the same molecule several times.
PROFESSOR: Yeah, and therefore, so which one are you arguing for?
AUDIENCE: For worse.
PROFESSOR: Yeah, for worse, OK. Yeah, and indeed, it does end up being worse. And it's actually significantly worse. It's something like 10 times worse. And I think the intuitive explanation is indeed what you just said, that a perfect monitor, that's great.
Except for the fact that it's counting every time that something hits. But then it doesn't know if it already counted that molecule or not. So then there's extra uncertainty that results from that. Of course, ultimately, you have to go and do the calculation if you want to be convinced of something like this. But indeed, the perfect absorber is the best that you can possibly do.
AUDIENCE: Unless it tagged the molecule [INAUDIBLE].
PROFESSOR: That's right. Right, so that's equivalent to being a perfect absorber, right?
AUDIENCE: Oh, OK. Well, no, because then-- oh.
PROFESSOR: Yeah, because these are non-interacting molecules. So if you tag it green, then all that you do is when the green molecule comes back, you say, I'm going to ignore that. But then that's equivalent to if you had just absorbed it.
AUDIENCE: But there's no local depletion.
PROFESSOR: Yeah, there's no local depletion. But because these are all non-interacting particles, then it can't make a difference. And I agree that this is confusing. But I'm pretty confident what I said is true. Because since they're all non-interacting, there's just no more information there. Yeah.
AUDIENCE: So what exactly is the error?
PROFESSOR: Right, OK, this is asking, over some period of time, I count the number of molecules that hit me or that I absorb, and then I say, OK, well, I think that I counted 20. And the question is really, well, if I did this again, how close to 20 would I get? Would I get, again, 20 or 21, or would I next time get 10 or 50? And that's the real question. How repeatable are my measurements?
So if I plot a histogram of a bunch of numbers that I get over this time-- this is the frequency that I observe a particular number of molecules over some period of time t. I get some histogram. And this is telling us about the width of that histogram relative to the mean.
Now let's imagine that you're the cell, and you calculated the concentration in a really wonderful way. Now, the question is, do you know where you should go to get more food? No, right? So you know the concentration at your location. But what you need to know for that purpose is a gradient.
And I'm trying to remember, in this paper, they maybe are not very explicit. Why is it that a bacterial cell might do this biased random walk that's described in the paper instead of just measure the gradient? Yeah.
AUDIENCE: Because cell size is so small.
PROFESSOR: Yeah, so bacteria are just small, I think this is the argument that we often give. And that's just saying that if you want to measure a gradient, then what you would do is, if you have a sphere like this, you might say, OK, I'm going to count the number of the molecules that hit me over here, and I'm going to compare that to the number of molecules that hit me over there.
So you get two measurements of concentration. But then in order to get a gradient, what's relevant is you have to look at this distance. And of course if you have a bigger cell, then a given gradient shows up as a larger difference in concentration, or a large difference in the number of molecules that are going to hit the cell. And does that scale linearly or quadratically with a?
AUDIENCE: Linearly?
PROFESSOR: Linearly-- difference in concentration is going to be equal to the size of the cell, maybe 2a, times dc/dx. So there's some gradient that's out there. And then so we get a linear amplification of our signal based on the size of the cell. So if you look at many eukaryotic cells, for example Dicty and others, they indeed often do this direct gradient measurement. And then from that, they decide where to go.
Bacteria, on the other hand, they do this other thing that's described in the paper where they have a biased random walk. We're going to be talking much more about this and the gene network that allows cells to do it on Tuesday. But the basic idea is that the bacteria, they're swimming in some random direction.
And then instead of directly measuring the concentration as dc/dx, which you might do as a eukaryotic cell, instead what they measure is the change in concentration with respect to time. Because they are moving. So they ask, are things getting better? Are they getting worse?
If things are getting better, you keep on going. If things are getting worse, then you randomize your direction, and you try again. And this implements a biased random walk. We'll talk about the decision making circuit in the cell that allows it to do that on Tuesday. But that's the basic notion there. And can someone remind us, how long is a typical run for E. coli?
AUDIENCE: [INAUDIBLE]
PROFESSOR: Yeah, it's maybe 30 microns. So there's this idea that a cell might go like this for 30 microns. And it takes about a second for it to do that. But then that's a run. But then it'll engage in some random tumble that leads it to go some other direction, and then another tumble, and then it kind of goes a different direction, and so forth. So these are runs and tumbles.
Now, the nice thing here is that you can see that to measure this concentration over here, the change of concentration across the cell went as a, which might only be 1 or 2 microns, whereas here now the E. coli can measure a change in a concentration over tens of microns. So it's in some ways like a longer antenna that allows it to pick up the signal better.
There's a comment about what happens here in the sense that if you imagine you have a cell, it's a few microns in size, flagella is pushing it, it's going at 30 microns per second. The question is, if at some time it stops spinning its flagellum, how far will it coast? And this is after stopping swimming.
OK, so we'll do an approximate, so 10 micron maybe. How many microns does a cell coast after it stops swimming?
AUDIENCE: This is a bacterial cell?
PROFESSOR: This is a bacterial cell. This is E. coli, and this is, we'll say, 3 microns in size. Ready-- three, two, one. All right, OK, so everybody says-- and is this actually the right answer?
AUDIENCE: No.
PROFESSOR: No, it's not. So this is the closest, it's true. But none of these things are true. So how far does it coast?
[INTERPOSING VOICES]
PROFESSOR: Right, yeah, so it's some ridiculous-- I mean, and this is really, really weird. This guy-- 30 microns a second. So this is 10 times its body length every second. It's really shooting along. But the moment that it stops swimming, it stops moving. So it's none of these things. It was 1 angstrom or less than an angstrom?
AUDIENCE: 0.1.
PROFESSOR: OK, 0.1 angstroms, which this is 10 to the minus five microns. OK, so it's orders of magnitude smaller than you would ever imagine. And that's because our intuition comes from kind of our length scales where if I were swimming this fast, I would keep on drifting after I stopped swimming. But that's not the world that bacteria live in. And that's because of the low Reynolds number.
The Reynolds number is much, much less than 1. So this thing is the dimensionless number that quantifies the relative importance of inertial forces to viscous forces. Now there was some discussion of the Reynolds number in your reading. I maybe won't get into too much detail about it. But what I'll say is that if anybody wants to talk about a way of thinking about the Reynolds number, maybe you can ask me after class, and I can explain to you why this weird expression can be thought of as the ratio of the inertial to viscous forces.
But I want to think a little bit more about the strategy that these cells are following, which is, why is it that a run is of the length scale that it is? In particular, why not go further? We already said that its ability to measure concentration is somehow-- if you did a direct measurement, it would scale with the size of the cell. In this case, it sort of scales like the length of the run. So why not run for 10 times longer, 100 times longer, so you can get a really good measurement of a gradient?
AUDIENCE: Because if you're going the wrong way, then you'd go 10 times longer.
PROFESSOR: OK, so it could be just if you're going the wrong way. Although then we could maybe even be just more sensitive in that. Because what's striking is that even when it's going in the right direction, it still tumbles. The difference between the run lengths and the correct and incorrect directions are kind of modest, I guess.
So maybe we could come up with a different strategy where we say, all right, well, if I feel like things are getting worse, I'll tumble quickly. But if I feel like things are going great, maybe then I'll go in the same direction, and I'll measure that gradient really finely so I can get precisely the right direction. So I guess the question is, is there anything that's going to limit how useful it is to go swimming, even in a good direction, in principle? Yeah.
AUDIENCE: So it's so small that collision with actual molecules can randomize its direction as it goes along, and it sort of loses memory.
PROFESSOR: And this is a weird thing, that not only does diffusion act kind of in a linear sense on the center of mass position of an object, but it also acts rotationally, which means that the cell and other objects in liquid lose their orientational order. What that means is that if this cell starts swimming in one direction, it's actually the case that after a few seconds, it forgets where it was going. So you can't actually collect information about the gradient in that direction, because you're actually going in a different direction from what you were a few seconds ago.
This is actually a pretty tough limitation that these cells are facing. On the one hand, they're small, so it's really hard to measure gradients. On the other hand, every few seconds, they get turned around. And if you imagine trying to navigate in that kind of situation, it would be pretty hard.
Now, I want to make sure that we understand how to figure out what the timescale is that this orientational order is lost in. And again, we're going to use dimensional analysis, because I love it. And I can tell that you guys are big fans as well.
OK, so I will tell you something that hopefully-- how much do I want to tell you? What I'll tell you is this, that the diffusion coefficient for angular fluctuations is defined just the way it is for diffusion coefficient for the center of mass. Given that, the question is, if I have a sphere-- again, size a-- now I want to know, how does the typical correlation time, the time to randomize, randomize orientation, how does it scale with the radius?
So it could be that this timescale tau could go a to the 0. And you should be starting to think. All right, do you need more time?
All right, why don't we go and see where the group is. And it's OK if you've not figured it out. Make your best guess. Ready-- three, two, one. OK, so I'd say it might be a slight majority B. But then actually there are fair numbers spread around.
Yeah, there's A, B, C's, D's, and E's. All right, maybe I'll give you a minute to talk to your neighbor. And I'm just going to give you an extra hint, which is it's good to look at the units of the diffusion coefficient, which is why I wrote this equation.
Yes, no? You did that. You're like, that's what we did. Well, I'll give you another minute. Because I think that it's important to work through this at least some way mentally.
[INTERPOSING VOICES]
PROFESSOR: All right, why don't we go ahead and reconvene. I know that you may not have had time to fully converge on this. But it turns out that this actually goes as a cubed.
AUDIENCE: Oh, no one would have guessed that. Come on.
PROFESSOR: He's so upset. It's the first sphere, yeah. It's a sphere.
AUDIENCE: So it's not a ball.
[LAUGHING]
PROFESSOR: I don't know what to say.
AUDIENCE: Where's the mass? Is the mass on the surface, or is the mass in the middle? Does it make a difference?
PROFESSOR: No, no, no. We're in the low Reynolds number regime. So what that means is that this is all dominated by the interactions between the object and the fluid. So it actually doesn't enter into-- yeah, mass is irrelevant. So it doesn't scale. That would've been a fine question as well.
So a cubed, all right, this is weird. Now, of course you can think about things in many different ways. But the thing that you can see maybe is that before, for the sort of linear diffusion, so D linear with respect to time, you can see that the difference in units between these two diffusion coefficients is a factor of-- there's a length squared difference between those two. And it's that length squared that turns this linear dependence of a for normal linear diffusion up to an a cubed.
Now, of course there are other things we can write down that may be useful. So remember this D linear, Einstein told us this is kT over gamma linear. So this is if we try to move it. This tells us how hard we have to push to get something to move. So this is if you apply force, then gamma linear tells you how fast it will move in this low Reynolds number regime.
Now, there's a similar set of things that we can say here. I'm going to erase this. So we can still say it's actually Einstein is just always right. So the D rotational again is going to be kT. Now this is a gamma rotational.
But instead of thinking about forces, now this is torques. So what's relevant is that if you apply-- oh, I already used a tau. OK, I'll just say, torque. So if you apply torque, on, say, a sphere, or this object, then this will cause an angular velocity, so gamma rotational times some omega around it.
Now, for concreteness, if you have a sphere, Stokes' drag tells us this is 6 pi eta a times v. So this thing tells us how hard we have to push an object in order to make it move some velocity if it's a sphere. Whereas the gamma rotational here, this ends up being 8 pi eta a cubed omega. So the gamma rotational ends up being that.
So you can see that in terms of rotational diffusion, rotational order, objects scale-- what do I want to say? The scaling is slower than you would expect somehow. Well, the scaling here is larger than you would expect, whereas the scaling here is smaller than you would expect. Yes.
AUDIENCE: Would this be any different for a bar-shaped bacteria?
PROFESSOR: Yes, it's always different, but not by very much. So for example, this thing, the Stokes' drag, is only true for a sphere. But this linear dependence on a is true for any of these objects. So it's really that a is some typical length scale. And in particular, it's actually the larger of the two length scales.
So for example, if you take a long kind of cylinder, pipe, now you can ask, well, now it's not isotropic anymore. So there's going to actually be two different gammas. There's going to be a gamma that we might call parallel if you want to push it in this direction, and a gamma perpendicular if you want to push it in this direction.
What's fascinating is that if this is something that's, say, a nanometer in size here but a micron here, so an aspect ratio of 1,000 to 1. Still the difference between gamma parallel and gamma perpendicular is still just a factor of two or so. Because in both cases, it's dominated by the longest linear dimension. So what's relevant is this big thing, length. So this is, again, an aspect of the surprising nature of the low Reynolds number regime.
This is not true if you were a skydiver or something, right? But the reason is that this longest dimension kind of tells you how much water you're going to be pulling with you. What's relevant is not the cross sectional area that you're kind of pushing. But rather it's telling you how much fluid are you kind of disturbing along the whole length. Are there any questions about what I mean by this? Yes.
AUDIENCE: I was wondering if [INAUDIBLE] because the flow is one different time. So you're asking about the time--
PROFESSOR: OK, so you're wondering how I actually get to a time from this. Yeah, OK, sorry, I should have finished this. So let me just show you what I meant. So one way to think about this is we know that this is where we start. And what you could say is, well, the typical time to randomize, that's when we've gone to enough time so that change of theta squared is, say, of order radian squared.
So it's enough that that's sort of a characteristic reorientation. So you just say, OK, well, we're going to set this equal to around 1. So 2Dr times this reorientation time tau will be around 1. So that gives us that the reorientation time is going to be around-- so we have 1/2, and then D rotation. Now we have 8 pi eta a cubed over kT. Is that kT, yeah?
And actually in units-- so I grew up in the single molecule world where everything was in units of piconewtons, nanometers, and seconds. And in those units, we could actually write, OK, we have a 4 pi. kT is actually 4.1 piconewton nanometers. So it's around 4 in these units.
And then eta is actually 10 to the minus 9 in those units. a, for a bacteria, is around a micron. So it's 1,000. But this is piconewton nanometer second units. So we have to do 10 to the 3 cubed. Because a micron is 10 to the 3 nanometers. And conveniently, 10 to the 3 to the third, 10 to the minus 9, we can just cross these out. We can cross the 4's out. All right, so we get pi seconds.
Should we take the pi seriously? No. But the point is that it's of order 1 to 10 seconds. This is what you get from such a back of the envelope calculation. But this is saying that from the standpoint of the bacterial cell, it starts going. And it forgets which direction it started going over the timescale of a few seconds.
So it's not worth it for the cell to actually have a run that's longer than that. Because it will have lost its way anyways. All right, what we're going to do on Tuesday is we're going to try to understand something about how bacteria use this combination of runs and tumbles in order to perform these exquisitely sensitive kind of travels to find food sources. If you have any questions about anything that we talked about, please come on up.
Free Downloads
Video
- iTunes U (MP4 - 177MB)
- Internet Archive (MP4 - 177MB)
Subtitle
- English - US (SRT)