Flash and JavaScript are required for this feature.
Download the video from iTunes U or the Internet Archive.
Description: This recitation continues to look at versions of the document distance code, and briefly discusses insertion and merge sort.
Instructor: Victor Costan
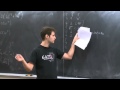
Recitation 3: Document Dist...
The following content is provided under a Creative Commons license. Your support will help MIT OpenCourseWare continue to offer high-quality educational resources for free. To make a donation or view additional materials from hundreds of MIT courses, visit MIT OpenCourseWare at ocw.mit.edu.
PROFESSOR: So did everyone turn in PSET1? Yes? Good. OK, so there is a PSET1 critique due in a few days. My advice? You already did PSET1. You remember what you wrote out on the proof. Look at the solution. Write one paragraph today, and you're done with it. Then you go focus on PSET2. If you leave it off until Tuesday, you're going to have to read your proof again, remember what you're thinking. It's a lot more work. Just do it now, get it out of the way, and put PSET1 behind you.
AUDIENCE: Is the critique only for the proof? Or is this for all of them?
PROFESSOR: Nope. Just the proof. So you have to compare your proof with our proof?
AUDIENCE: Is there an assignment for that? Or do we just know to do it?
PROFESSOR: Uh. PSET1 be Stellar. Oh, no, sorry. It's not in Stellar. It's on our new grading site that just went out. So you have to go to our new grading site, and you have to type in your critique there. And it's one paragraph. You should aim for one paragraph. If you're doing more than that, then you're doing something wrong. And it's LATEX Plus math mode. So you can use math mode, and that's about it.
OK, any more questions about the critique? It's a new thing. We care about it because it will make our grading life easier. And because it'll force you to look at the solutions and see what you understood and what you didn't. So we care about it. Don't ignore it. Yes?
AUDIENCE: Like, how much is it weighted? How much does it count toward the grade?
PROFESSOR: If you don't have a critique, we will most likely give you a 0 for proof.
AUDIENCE: [INAUDIBLE].
PROFESSOR: If your proof is bad and your critique of the proof is good, then you might get something. If your proof is bad and you have no critique-- actually if your proof is whatever it is and you have no critique, you get a 0.
AUDIENCE: Yeah. [CHUCKLE]
PROFESSOR: Any more questions about that? OK. Who needs help remembering what the document distance problem is? OK. Everyone who went to lecture or to [INAUDIBLE] remembers. That's good. Who went to lecture last time? Cool. That's good.
So we did insertion sort and merge sort from a theoretical standpoint. Today we're going to look at the code for the insertion sort and, if we have time, look at the code for merge-sort, and use the same strategy as we did last time to analyze them, look at the running time, make sure the running time matches the theory, and see how pseudocode turns into Python.
So you all have your listings. Last time in document distance, we covered Main, and we covered most of the functions except for count frequency. Can anyone remind me what the call graph looked like? So the call graph is the tree that I had up on the left, and it started at Main. Thank you. So Main calls word frequencies for file, which in turn calls?
AUDIENCE: Well, it's probably line list.
PROFESSOR: OK.
AUDIENCE: And count frequency.
PROFESSOR: So we pretend we don't see the read file called. We assume that the data is already in memory or that the call takes time that's proportional to the running-- to the length of the file. And we only look at get-word from line list and count frequency. OK. Who else does Main call?
AUDIENCE: Vector angle?
PROFESSOR: And the vector angle?
AUDIENCE: Inner product.
PROFESSOR: OK. Let's put up the constants for-- for the document distance problem that we used last time. So we said that the document has W words. And then when you take that list of words and you turn it into a distance vector, you will get assigned to a document vector. You will get L elements, which basically means L unique words. So L is the document vector length.
And we assume we're using a natural language like English, so all the words are bounded in size. Like 5 to 10 characters, for example. And to make our life easier, we say all the words have the same size W. So w is the word length.
Using these numbers, can anyone remind me what we said the costs for these methods are? And we didn't analyze count frequency, so it's OK to not take my word for it and not tell me what I said last time. But I would like numbers for word frequencies from file, get word from line list, vector angle, and inner product.
AUDIENCE: Was that mu squared? That was last time.
PROFESSOR: OK. Which-- which one? Does anyone-- does anyone else want to try? Let's not do guessing. I'll pull them up if nobody remembers. I spent an entire hour on that, and you guys did too. It was painful.
AUDIENCE: I know. But we had to, like, you know, add them up, and then--
PROFESSOR: Yeah. So we did a lot of work for those numbers. So get word from line list was order of W squared. Does anyone remember why? What made it take so much time?
AUDIENCE: 'Cause you append it to the word list. For, like, you add it to the end to go through.
PROFESSOR: OK. So you add it. So what?
AUDIENCE: So, like, every time you need it, you, like, do word list equals word list plus words in line.
PROFESSOR: OK. Excellent. So get words from line list, line five. There's a plus there. And that plus sign messes up the performance. So that's why it's w squared. Count frequency, we didn't cover it, you had to take my word for it. So we will cover it now. And, inner product.
So suppose you have two vectors of line-- length L1 and L2. How much time does it take to compute the inner product?
AUDIENCE: L1, L2 time.
PROFESSOR: OK. So how much time does vector angle take?
AUDIENCE: L1 L2 time.
PROFESSOR: L1 L2. So it makes three calls, right? You get the two things. First, it computes the inner product of the two lists. And then it has to compute the inner product of each vector-- of each document vector with itself. Because that's what's on the bottom of the fraction. So what's the running time for that?
AUDIENCE: Plus L1 squared, plus L2 squared.
PROFESSOR: Plus L1 squared, plus L2 squared. So someone was really helpful last time and asked me, can you make this simpler with some math? And I said, I don't know so I don't think I will. But I looked at-- my, I looked at my high school math afterwards, and it turns out that these-- so L1 squared plus L2 squared are guaranteed to be greater than L1 L2 as long as these numbers are positive. And we're working with document list, so they're always positive. So this will go away.
So let's assume count frequency is w squared or smaller. So the total running time for word frequencies from file is w squared. What's the running time for everything?
AUDIENCE: Would just be, like, w squared plus L1 squared plus L2 squared?
PROFESSOR: Almost.
AUDIENCE: Actually, it depends on which one's greater, right?
PROFESSOR: Well, almost. So here W works, assuming you get one document with W words. But word frequencies from files is called twice, once for each document. First document has W1 words, second document has W2 words. So what's the running time?
AUDIENCE: W1 squared plus W2 squared.
PROFESSOR: W1 squared plus W2 squared. So I take this, and I add this. Right? Except when I add this, if I want to add L1 squared, I know L1 is the number of unique documents-- of unique words in the document. And W1 is the total number of words in the document. W1 guaranteed to be greater or equal than L1, so it's going to dominate. I don't need to add it. Same for L2. This is it.
OK. You guys don't seem to remember the numbers for these. So that means I didn't torture you enough last time. So let's do more. Let's look at count frequency. And let's compute the cost for that.
So what we did last time was, we went through each line of code. We thought, how much time does it take to execute the line once? And how many times does the line run? And then we compute the product of that, add everything up, and that's the cost for the function. First off, before I put numbers here, what does the method to do?
AUDIENCE: It takes a list of words--
PROFESSOR: OK.
AUDIENCE: For each item in that list, checks to see if it's-- you know, list of words that it's-- counted, right?
PROFESSOR: OK. So you're telling me what the code does.
AUDIENCE: Yeah.
PROFESSOR: Try to look at Main or try to look at word frequencies for files. So look at it top-down, and tell me what the purpose of it is. What's the goal?
AUDIENCE: Making a list of-- and each object is a list with a word and a number.
PROFESSOR: OK. Excellent. So big picture, I have the first document. I read it in. I break it up into words. And I have a list of words. That's what word frequencies for file gives me-- sorry, that's what you get words from line list gives me. List of words. The fox is in the hat. And this gets passed to count frequency, and count frequency gives me, you said, an object, which is a list. Of lists, where each of them has the word and how many times it shows up. So I would have "the" shows up twice, "fox" shows up once, "is" shows up once, "in" shows up once, and-- I need a shorter example-- "hat" shows up once.
So it takes this and turns it into that. So on line 2, I have a list L that's initialized. And then, at the end, it's returned. So I'm going to guess that L is going to look like this. Line 3, for new word in word list, iterates over the input. So iterates over this. And then, line 4 checks to see, for each new word, it looks at the list that I have under construction.
So exam-- for example, if I ran through all the words and then I'm trying to put in hat right now, I wouldn't have it here. What line 4 does is, it looks at all the entries. And it says, if I can find the words-- so if I can find the word hat somewhere here-- then increment the number. If I can't, then make a new entry and say that the word shows up once, because it's the first I see it.
So this is what the code does. Now let's see how fast it does that. So line 2 initialize the output to an empty list. What's the cost for that?
AUDIENCE: [INAUDIBLE].
PROFESSOR: Very good. How many times? For new word in word lists. Cost?
AUDIENCE: [INAUDIBLE]. I know the cost is 1.
PROFESSOR: OK, so we are-- here, it's a bit confusing, right? We're saying that, oh, there's does iteration over a list. And each step of the iteration is constant time, but the iteration happens L times. I'm sorry, not L times. The length of the list times. How many-- how many elements are in word lists?
I heard a very low W, so I will pretend I heard it. Or I hope I heard W. So word list, the words I got from the document, W. How about the if. So, it looks at the word that I have-- oh. This code is confusing because I forgot a line, right? Pretend that between line-- oh, no, sorry, I didn't. New word is-- new word is assigned in line 3. So new word in line 3 is compared to the first element of the entry that's assigned in line 4. So hat is compared with the, fox, so on, so forth. And if the comparison is true, it runs line 6 and 7. And if not, it keeps looping.
So line 5, the if how, many times does it run? Just the comparison.
AUDIENCE: W.
PROFESSOR: W.
AUDIENCE: Oh, no, no, no. No. I'm thinking of line 4.
PROFESSOR: Oh. Yeah. I'm getting confused too. So let's start with line 4. Sorry. Shouldn't do line 5.
AUDIENCE: --new word, then you're not-- like, you're not going to run through that again.
PROFESSOR: Yeah. Let's worry about that right afterwards. Let's do line 4 first. Sorry, I jumped over line 4. So, line 4 definitely runs W times because it's inside the for loop from line 3 to line 9. So everything here will definitely run W times. But how many times does it run overall? So, line 4 iterates over all the entries here. How many times does that happen?
AUDIENCE: 1 plus W over-- times 10/2, because it's just worst case, L-- the length of L increases by 1 every time. [INAUDIBLE].
PROFESSOR: OK, so I like that you started with worst case. Normally I would say exactly that. Worst case or W. But we had a different constant for the number of words that you have in the end. So let's say something a little bit better than W. Let's say, let's put the lower bound on it. So yeah, worst case, all the words are different. But what if they're not all different? And what if in the end I know I have L words?
CLASS: [INAUDIBLE].
PROFESSOR: Worst case. Almost. So, I know I have a W from the outer loop. For each word in the outer loop, how many times does the inner loop execute? How many times do I have to go through something in the inner list? So I know here I have W words, suppose here I have L elements in the vector. For each one of these, how many times do I have to go through-- So how many elements do I have to go through here?
AUDIENCE: Depends on where you are, though. For the first word, you only have to go through one.
PROFESSOR: Yep. But--
AUDIENCE: For the second word, you have to through--
PROFESSOR: Yep. But I heard the worst case. And I like that, because it's easier to reason about the worst case. And most of the time it's sort of like the average case.
AUDIENCE: So then, length of list-- L.
PROFESSOR: The length of the list, and that's L. Worst case, the first words that I see will be L different words. And then all the words that I see will be the same as the words that I saw before. So worst case, the list will grow to L very fast, and then I'll keep seeing L L L. And I'll ignore what was there in the beginning, and I'll say L times. So I know the second list is bounded by L in length, the first list is bounded by W in length. So worst case this runs L times W times. And what's the cost of iterating?
AUDIENCE: What is the difference between L and W? L is the document vector length, and W is the number of words. But isn't the number of elements in the document vector the number of words?
PROFESSOR: How about this case? What's W?
AUDIENCE: 6, yeah.
PROFESSOR: So L is the number of unique words in a document. And I heard a really cool argument that I liked last time. Does anyone remember? About L? If we're really dealing with a natural language like English, how many words do I have in English?
AUDIENCE: Well, I think, at the max, there's actually around 250,000, but a lot of them are not used anymore.
PROFESSOR: OK. So 250,000, right? Max. So that's a constant. If I have a document that contains all the writings of all the authors that were ever done, and say that's a billion words, L is still going to be 250,000. Right? So L can be very different from W. That's why we're keeping track of them separately.
One W L times W. What's the cost of iterating? So we know how many times line 4 runs, but what's the cost of one step of iterating in the list? 1. Very good. Line 5. How many times does it run?
AUDIENCE: W times L?
PROFESSOR: Yep. Same as line 4, right? The if is run all the time. And lines 5 and 6 only run sometimes, but-- sorry, line 6 and 7 only run sometimes, but line 5 runs all the time. What is the cost?
AUDIENCE: We can say it's constant.
PROFESSOR: We can say it's constant. I like we can say it's constant, but why is it that we can say it's constant? Why don't I just say 1, if-- this is an empty list. This is a number.
AUDIENCE: Depends on the word length.
PROFESSOR: OK. It depends on the word lis-- length, very good.
AUDIENCE: And we're assuming that words are all the same length.
PROFESSOR: OK.
AUDIENCE: And? So, 1 times L W. Little w.
PROFESSOR: OK, very good. So we do assume that all the words are the same length. But unless I tell you that the length is really small, which I did, you can't say 1. So, when you said we can say it's constant, it's right. We can say, but we also have to say why, or at least think why, that's the case.
So it's W-- we're going to use W here and when we copy it here, we're going to forget about it.
AUDIENCE: Can you put a top bar on the W, just so I can tell that it's not the other W.
PROFESSOR: OK But you have to be responsible for reminding me to put that.
AUDIENCE: OK.
PROFESSOR: OK. So string comparison, not constant. If I have two very long strings that only differ in the last character, I have to go through them character by character by character until I find the last character that's different. Because until I look at that, the strings might be equal. So comparing two long strings takes time that's proportional to the length of the strings.
OK, so line 5 costs w, tricky part, runs L times W part-- L times W times. How about line 6 and 7? I didn't ask line 6, I asked line 6 and 7 together, because there is a trick there.
AUDIENCE: I think it's constant for line 6, right?
PROFESSOR: Why?
AUDIENCE: 'Cause it's a number. And one place in the entry. We've already grabbed the entry.
PROFESSOR: So the cost of running it once is 1. Good. I can tell you that that's the same case for 7. How many times do they run? This is the hard part.
AUDIENCE: Wait, does break line break out of one loop?
PROFESSOR: Yep. Break breaks out of the loop between lines 4 and 7. I have a question.
AUDIENCE: Is L supposed to be not in line with if? Yes.
PROFESSOR: It is supposed to be where it is. I will talk-- yes. So what happens is, that else is in line with a for. And if the for loop runs to completion, then it does get executed. If there's a break somewhere inside the for, then it doesn't get executed. So the idea behind that is, usually use this for finding stuff. So you iterate over a list, and when you find something, break out of the loop. You did something, you break out of the loop. If you didn't find it, you can put an else and then say what code happens. And you don't have to write code on your own to check if you broke out of the loop.
So if break executes, then it's going to take us out of the loop. It's going to ignore the else. And it's going to run the loop on line 3 again. So it's going to do another iteration. So line 6 and 7, how many times?
AUDIENCE: W minus L?
PROFESSOR: W minus L.
AUDIENCE: Like, if it's the difference in number of words and number of-- unique words.
PROFESSOR: Smart. You gave the precise answer right the first time. W minus L. Very good. And what happens once-- what happens once this runs? Why do I know-- why do I know that the if won't be-- Oh. Sorry, I'm getting myself confused. So it's going to run W minus L times total, right? Total times overall without this W thing here, so I should put an arrow and say--
Now suppose I didn't notice this. Is there another way I can get the decent bounds? So this is the right, perfect answer. You have this, you're done. You don't need to think further. Suppose you don't have this. What else can you do?
AUDIENCE: But we don't have what?
PROFESSOR: If I didn't notice that, hey, there are L words-- There are L new words, W words total, so W minus L words repeat themselves. So this is how many times the if is going to be true. If I didn't have that, then I could see that line 7 breaks out of the loop. So if that if runs once, then we're done. We're out of the loop. It's not going to run again. So a bound that's not as precise as the one you gave me is 1 times W, because it runs, at most, once per loop.
Does people see this? So this is an easy way to cop out of thinking. And I don't like to think more than necessary, because you have finite time on a test or in life, and you don't want to spend too much time on one thing. We covered the loop. Let's look at else and append.
I already got a helpful question, so I explained when the else would run. The running time for else. Else is a flow-- control flow statement. It's like break, so Python will keep track of whether a loop completed or not in constant time. I'll give you that. That's in the cost model. How many times does this else run, at most? OK, L. Good. Perfect. How about line 9? Loop stops here. How about line 9? How many times does it run? That's easy.
AUDIENCE: [INAUDIBLE].
PROFESSOR: Yep. And what does it do? It's an append. What's the cost for append?
AUDIENCE: Constant.
PROFESSOR: OK Line 10 runs how many times? What's the cost?
AUDIENCE: 1?
PROFESSOR: You guys didn't listen to me last time. So I was saying you have to look at the notes and you have to practice this. Because you have to have this model in your mind. So that when you're writing code, this has to happen automatically. You shouldn't have to think explicitly about it. Because if you do, you're not going to do it.
AUDIENCE: For the else, shouldn't it be W tim-- I mean, it would be called W times not L times? Because you want to look at the outer loop and not the inner loop? So it can-- you call all-- once at the end of the total-- the inner for. Right? So, so it could be-- happen W times, maximum, not L times. 'Cause the L is the for loop that the else coincides with. And the else would only happen once for every total iteration of that.
PROFESSOR: OK so you're proposing W as the bound for the else, right? Here.
AUDIENCE: Yeah.
PROFESSOR: So I could say, hey, it runs, at most, once for outer loop. So it's, at most, W times. This is a nice, easy argument. We have a bound. L is a tighter bound. And when I got L, what happened here was the same kind of thinking that you did earlier to get this. So this bound is good, this bound is tighter. This bound is good, this bound is tighter. And the argument behind this one is that, hey, this else only happens for new words. If there's no new-- if the word that I looked at is old, then break is going to execute. And else is not going to execute. So that's why I can say L.
The beauty of asymptotics is that I can use either of the bounds and I'll still get the correct running time. So I'm not going to fuss over it too much. I like the tighter ones, because it means you guys are thinking. And you're looking at the algorithm, and you're understanding it. But if you don't have them, you'll still get the correct running times. So I think that's nice.
PROFESSOR: OK, let's get the running time for everything. Can someone do it in one step? Then let's do it step by step. So let's compute partial products. What are they?
AUDIENCE: 1. W. LW. L, W, little w-- with the bar.
AUDIENCE: (LAUGHING) With the bar.
PROFESSOR: Awesome.
AUDIENCE: W minus L. W. L, L, 1.
PROFESSOR: OK, so if I add them up, this is all asymptotic. So the biggest one will dominate. In general, I can just take a max instead of doing actual addition. So who dominates here?
AUDIENCE: The fourth one down?
PROFESSOR: Fourth-- OK. Yep. So line 5 is the biggest time consumer in this algorithm. And I know it's W times w-bar. So now I'm going to copy it here. What did I say I'll do when I'm copying it here?
AUDIENCE: [INAUDIBLE].
PROFESSOR: Yep. So I'm assuming English five- to ten-character words. W L. And W L is smaller than w squared, so the assumption that I had before is correct. I don't have to change anything here. That is good.
So we noticed last time, and already forgot by now, that the biggest problem in this whole implementation was the plus in get words from line list. Suppose we forgot about it and we have this big pile of code, how do I go about making it faster? Method one, go through every method. Do this. Compute the running times, and see which one's the slowest. Does this scale to 1,000 lines of code? Not so much. We're going to be giving you roughly 1,000 lines of code for PSET2, and we're going to ask it to make it faster. Do want to understand everything? No.
Instead what you want to do is run the code through a profiler. So we have a computer. The computer can tell you which line takes the most time to run. So you don't have to do it on pen and paper. Whenever we can automate, do so. So we'll teach you how to run a profiler. It's in the notes. And if you look in the code outputs right after [INAUDIBLE], you'll see a profiler output. So what that tells you is for each function, how much time does it take-- that's the total time? And there's the cumulative time, which is how much does it take together with its children?
In this case for word frequencies from file, order of W-- this is how much time it takes including the functions it's called. So this is the cumulative time. Cumulative time is useful if I'm during runtime analysis. Is not so useful if I'm looking at where's the slowness in my program? Because if you look at cumulative time, you might see the slowness in one of the functions that get-- that word frequencies from file called. So the cumulative time for this is really big, but the total time-- the time that's spent inside it-- is not that bad.
Instead if you look at total time, you'll see that the worst function is-- surprise, surprise-- get words from line list. So 5 lines to look at-- hey, there's a plus there. I remember from lecture that plus copies over lists, and it's kind of slow, so maybe I should use something else.
Does d remember what else we should use? We talked about that last recitation. Extend. Document distance 2 . The only difference between it an document distance 1 is get words from line list, line 5. That plus turned into an extend. One character turned to six, so about eight keystrokes, 30% runtime improvement. Very good return on investment. Everything else is going to be harder.
So let's look at that line, because that line dominated the running time of get words from line list. And think what's, then, your running time for it now that I have extend?
Does anyone remember what get words from line list does?
AUDIENCE: It gets the words out of the document.
PROFESSOR: OK . It gets the word out of the document. So it reads a document that looks like a regular text file, and it gets this out of it. The way it does that is it goes through each line, reads the line as a string, breaks up the string into a list of words, and then combines all those lists of words together. Get words from string, line 4, returns the number of words in a line. Sorry, the list of words in the line, and then extend combines the lists together. Let's add some constants that we had last time.
I think we had that K is the number of words per line. And Z is the number of total lines. So this K is actually-- W over Z. No, I don't like that. That's work. So Z is K W over K. And we argued that we're not going to talk about K too much, because a document needs to fit on a screen or needs to fit on a piece of paper. So the line length has to be finite, right? Otherwise, if I have a document that has 10,000 character lines, I can't even write it on this board. Even though it's really long. So K, the number of words in a line, is going to be finite. But we'll need it for this analysis.
So line 4 returns a list with the words on a line. How many elements in that list? This returns a list with how many elements?
AUDIENCE: Could K [INAUDIBLE] words on line.
PROFESSOR: So even if I ask easy questions, you guys have to answer. Because otherwise I'll stall until you do. So 4 gives me a list with K elements, and 5 appends that small list to the big list of words in the entire document. Before I used plus and that did something bad. Now I'm using extend. What's the cost of one extend called?
AUDIENCE: Constant? Order of K?
PROFESSOR: I want to know your Python implementation.
AUDIENCE: If Python did it like a linked list , like doubly linked lists, could be order constant?
PROFESSOR: I like your question. So if Python lists were actually linked lists-- so if the name wasn't confusing-- then yes, merging two lists would be constant. But then accessing one element in the middle of a list would not be constant anymore. Say I want to access element number 200 in a list of 10,000 elements. I have to go through 200 elements. We didn't do linked lists when we ran it, so don't worry about it.
So they decided that it's less confusing to have lists actually be arrays. So Python lists, array in CLRS.
AUDIENCE: That can use their storage contiguously?
PROFESSOR: Yep.
AUDIENCE: So when you copy, why can't you copy a block? Why do you have to access each other? Does that make sense?
PROFESSOR: So, you can copy a block, but in order to copy a block, you still have to move everything. So if your block is 10,000 elements, you still have to move 10,000 bytes times element size. And the CPU works on 4 bytes at a time or 8 bytes at a time. OK. But this is the right kind of thing to be thinking about when you're doing the cost model. And this is what you want to have in your head when you're writing Python. So, good. I like your question.
I wanted to say that at some point, but I didn't get the occasion yet. Lists in Python are not lists in CLRS.
OK. So with that long explanation, an extend is a list-- is a sequence of appends, right? If you have two lists and you want to extend the first list to the second list, extend basically goes through each element in the second list and calls append on the first list. The list is length K, the second list is length K, so K appends are going to happen. And append is constant time. Total cost, K.
Now many times does line 5 run?
AUDIENCE: [INAUDIBLE].
PROFESSOR: Very good. So what is the total running cost of the algorithm if this is the line that dominates? I don't want to do every other line, so I'll promise that this is the line that dominates. What is the total running time?
AUDIENCE: [INAUDIBLE].
PROFESSOR: OK. Very good. K times L. And that is? Oh, K. So I shouldn't have said K times L, sorry. L is not the number of lines. L is the number here, so Z is the number of lines. So it's K times Z. Which means I'm using bad letters, so please bear with me. We'll forget about them in a minute. So K times Z equals?
AUDIENCE: W.
PROFESSOR: W. So what do I write here?
AUDIENCE: [INAUDIBLE].
PROFESSOR: OK. Good. Very good. What do I write here? Word frequencies from file.
AUDIENCE: W L.
PROFESSOR: OK. What do I write here?
AUDIENCE: W 1.
AUDIENCE: We have to-- put L 1 squared and L 2 squared back in.
PROFESSOR: OK, do we? Well, the-- No. W, you want to always be bigger than L. I hope.
PROFESSOR: Yep. So if I put it in, L 1 squared is L 1 L 1, which is smaller than W 1 L 1. But I have to think about it before doing that. I can't ignore this completely. So this is document distance 2. The asymptotic complexity improved, the running time improved.
Next thing that happens to make this faster is-- I'm not giving you the profiler output, but you have to take my word for it that the longest methods are count frequency and inner product. So what I'm going to do first is I'm going to make inner product faster. But in order to do that, I have to make word frequencies for file slower. And that is because I happen to know an algorithm for inner product that is a lot faster, if only you can promise me that in this list, the words are ordered. The words are sorted.
So what happens in that list 1 is, the moment I see a word, if it's not in the list I add it at the end. So the words here show up pretty much in the order that they show up in the file. Well, if instead I could have something that looks like-- what is it? Fox. In. His. Hat is somewhere here. So if these words would be in this order, together with the word that I'm missing, then I can combine two lists. I can do an inner product a lot faster.
Let's see how that would work. And I'm already getting confused my words, so let's do a trick. Let's say that instead of words, we'll use numbers. So instead of saying "the," I'm going to say the is the 50th word in the dictionary. So I'm going to use number 50. Because I want to write numbers. The numbers are easier to deal with.
So say the first document that I have-- the fox is in the hat-- as words number 3, 4, 6, 8, and 9. And they show up twice, once, once, once. 9, once. And say I'm trying to compute the inner product of this with a document that has word number 2 showing up once, word number 3 showing up once, word number 6 showing up once, word number 7 showing up once, word number 8 showing up once.
OK, the algorithm for inner product that we talked about last time was, go through each element in one of the vectors, find an element with the same word in the second vector, and if you can find it, then take the number of times the words show up and multiply them. So here I have a 3, 2. I would find this element here that has 3, 1. I have these everywhere. And I take the 2 and the 1 and I multiply them. So the 3s have to be the same, then I think the 2 and the 1, and I multiply them. And for all the elements where that's case, I add up the results of the multiplication.
So step one, go through each element in a vector. That's not going to get faster if this other vector is sorted, right? But the step of looking up the second element can be sped up. The first and easiest way I can speed this up is, hey, this is sorted. If I'm looking up three, why not do a binary search? What would be the cost if I do that?
So here I have L1 element, here I have L2 elements. What's the cost of doing one binary search here?
AUDIENCE: Log of L2?
PROFESSOR: OK. And how many times do I do a binary search?
AUDIENCE: [INAUDIBLE].
PROFESSOR: So if I go through each element here and I do a binary search, which is a nice and easy algorithm that I can explain in 10 seconds, I'm already faster than L1 L2. So it's worth sorting. Now, the algorithm that we use in class takes time proportional to L1 plus L2. So that's even trickier, and it's going to take a bit more time to explain. Does-- did anyone understand the algorithm for class and wants to help me explain it? Didn't think so.
OK. So idea is that both of these vectors are sorted. So if I have a 3 here and I found my 3 here, next time when I get to 4, I know for sure that 4 is not going to be anywhere here, because this vector is sorted. Say I couldn't find 4, then I go to 6. If 6 is here, when I have to look for 8, I know for sure that 8 is not going to be anywhere up here.
So what I do is, I have a pointer here that remembers, where's the last element that I have seen? Does this make sense to people? So when I start here and I look at 3, I have a pointer here that says, I didn't see anything here. I look at 2, it's not 3. It's smaller. I look at 3. I found it, good. I do my product. Then I go look for the next element here, 4. I look at 6. 6 is bigger than 4. So I know for sure that nothing below it is going to be 4. So I can stop right here and keep my pointer here.
Then I go to 6 here. And I look here. Where did I stop? I stop here. This element matches this. I do my product. Keep it in. Now I go to 8 here. I was at 6. 6 is smaller than 8. 7 is smaller than 8. 8 is equal to 8. I found something. Sweet. I do a product. And then I stop. And I look at the next element. I know for sure that nothing here is going to be 9, so I can keep looking down. I hit the end of my list. OK. Whatever I have down here-- it's going 9, 10, 11, 12-- is not going to be in this list, because it was sorted. So I can stop.
AUDIENCE: How do you keep going-- What algorithm so you use to keep looking down on L2?
PROFESSOR: Plus 1.
AUDIENCE: So what if--
PROFESSOR: I keep going down.
AUDIENCE: What if the left side was 3, 4, 6, 9243?
PROFESSOR: 9,000, what?
AUDIENCE: Just a big number.
AUDIENCE: Right. Then you have to increment by 1 each time--
PROFESSOR: So I'm not incrementing the number I'm looking at. Here, I looked at 6. Then I'm looking for 8. And after I found 8, I'm looking for 19,000. So I'm going to go down, either until I find 90,000 or until I stop.
AUDIENCE: So are you going to go down one at a time,
PROFESSOR: Yep.
AUDIENCE: --why not do a binary search?
PROFESSOR: Because if I do a binary search, the analysis that says it's fast is not going to work. It turns out that this gives me the optimal running time. If I do a binary search, suppose I have a list that's like this. 1, 2, 3, 4, 5, all the way down to 10,000. Ugh, I can't write. And I have another list that's like this- 1, 2, 3, 4, all the way down to 10,000. 1, 1. I do a binary search, takes log N. I look at 2. I do a binary search, takes almost log N. I look at 3, do a binary search, takes almost log N. So on, so forth. So this is--
AUDIENCE: But if your left list had been
PROFESSOR: Log N plus log N.
AUDIENCE: --10,000.
PROFESSOR: Yeah.
AUDIENCE: You'd have taken N time.
PROFESSOR: Yes. Well, this algorithm takes N time, even if I have to list like this. It takes 10,000 plus 10,000 time. Whereas this algorithm will take time that's actually proportional to 10,000 log 10,000. You believe me. So, a way to look at this is to do bounds and say, for the elements 1 through 5,000, it's going to do a binary search for more than 5,000 elements. So, the time-- the running time is definitely bigger than N over 2 log N over 2. Constant. This becomes log N minus 1 and log N.
That is a good question. I wondered about that the first time I saw merge-sort too. And I was thinking, hey, I'm going to do a binary search here because it's faster, and I'm going to make a faster algorithm than anyone has ever seen. Well, if you do the analysis, not so much. But you need to think about it, and you need to know why that's true or that's not true. So I like your question. Thank you.
So now let's get down so the plain old merge-sort that everyone-- sorry, merge that everyone knows. So if we go through these one by one, how many times am I going to be advancing this pointer? In total?
AUDIENCE: L2?
PROFESSOR: L2 times. So this pointer can only go down, right? So worst case is going to go down L2 times. And then I'm done with the list, return. How many elements am I going to look through? So how many times does this pointer going to advance?
AUDIENCE: L1?
PROFESSOR: This one.
AUDIENCE: But I thought, like--
AUDIENCE: Then you get extra ones in between.
PROFESSOR: What if L2 is bigger than L1? What if--
AUDIENCE: Oh, right. OK, never mind. The reason I said L2 was because--
PROFESSOR: You're thinking after I'm going through this list, I'm out, right?
AUDIENCE: Right, that's what I thought.
PROFESSOR: So, your answer works if this list, say, has 10 elements and this has 10,000. And I go through this one really quickly. But if this list has 10 elements and this list has 10,000, and they both start with 1 through 10, I have to say a 1 because that's a better bound. So I have to say this.
AUDIENCE: Could you say the opposite value of the difference between the two of them? Because if you're going to stop at 1 has 10 and the other one has 10,000, and let's say that only the first ten are actually equal, then you're going to go through that list, find all 10, and you stop. That would be 9,000--
PROFESSOR: I could say about that if I'm looking at one case, but the magic trick is-- let's-- we're looking at the worst case. So worst case, if I have 10 elements, they'll be all the way down in the other list. Or they won't be there at all. And I have to go down through all the list.
AUDIENCE: OK.
PROFESSOR: So worst case, L1 plus L2. Let me see if I have any time left. Nope. So, what I would like you to do is go through insertion sort. Insertion sort matches the textbook. Look at the definition in the textbook, look at the code, convince yourself it's the same. Look at the running time, convince yourself it's N squared. Then look at inner product and convince yourself that this is what it does.
Go through this line by line, see where they match, put the cost on. Make sure that the cost is L1 plus L2. And last, go through merge-sort and notice that merge in [INAUDIBLE] 6 is exactly the same as inner product. So this pointer magic that I did here is exactly what's happening inside merge. And understand merge-sort. Look at the textbook, look at the notes, and see how they match. OK. Thanks, guys.
Free Downloads
Video
- iTunes U (MP4 - 117MB)
- Internet Archive (MP4 - 117MB)
Subtitle
- English - US (SRT)