Flash and JavaScript are required for this feature.
Download the video from iTunes U or the Internet Archive.
Description: This recitation reviews numerics and graphs in preparation for Quiz 2.
Instructor: Victor Costan
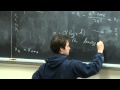
Recitation 18: Quiz 2 Review
The following content is provided under a Creative Commons license. Your support will help MIT OpenCourseWare continue to offer high quality educational resources for free. To make a donation or view additional materials from hundreds of MIT courses, visit MIT OpenCourseWare at ocw.mit.edu.
PROFESSOR: So you guys might have heard, we have a quiz tomorrow. So we're going to do a review for that. Did everyone turn in P set six? Yes? Good. All right, so what's on this quiz? Numerics and graph stuff.
So are there any specific pain points? I have problems prepared. Now, and then at 8:00 PM is going to be a review session for concepts. So now problems, 8:00 PM concepts, tomorrow [? Yakim ?] has office hours at 5:00 PM, conveniently placed before the quiz. Poor guy. So take advantage of that. So yeah.
AUDIENCE: Numerics, on P set we were mostly asked-- you said five, four, whichever came before. You mostly asked for the running times and things like that. But the numeric lecture notes are kind of detailed and go into Newtonian method and all that. How much detail do we need to know?
PROFESSOR: So on the P set we make your life easier by giving you the pseudocode of the code that you needed to implement. And we ask you one algorithm design question, the problem of finding the k-th root.
And that required you to understand almost everything that was going on. We'll want you to understand things that are going on to come up with something fairly similar to what you've seen. So yeah, I know that I told you guys before that ignore numerics. Just close your eyes and pretend it didn't happen.
Actually it turns out there's a significant amount of numerics on the quiz. So we're going to start with a numerics problem, and go through it. I'm really sorry about that. Clearly I didn't have my way for this, right?
OK so we started two big things in numerics. Karatsuba, which is really easy compared to Newton which requires a lot of understanding. So we're going to spend our time on Newton today.
OK, so anything aside from that? Yes.
AUDIENCE: The thing we were just talking about before you were here was what kind of edges are produced by depth first search versus breadth first search?
AUDIENCE: And why do they matter?
PROFESSOR: And why do they matter. OK.
AUDIENCE: Because it's probably going to be a question on the--
PROFESSOR: So I'm going to go on this very quickly now, because I want to cover problems, but tonight-- this is on the outline for the review. So if you guys can make it tonight--
AUDIENCE: It's late, so I can't make it.
PROFESSOR: Sorry. Well, they are also in the lecture notes. But I'll go through them very quickly now. OK, anything else?
AUDIENCE: Making trees with DFS and BFS, this might be tonight also.
PROFESSOR: Making trees--
AUDIENCE: Or your search trees. So instead of-- do you know what I mean by that?
PROFESSOR: Do you mean graph transformation?
AUDIENCE: So you've got a tree and you search through it. So then when you're searching through it, the result of searching it, you can put that into almost a binary tree.
PROFESSOR: OK so how BFS and DFS work and how they produce trees, I'm assuming, right? Because if you start out with a tree, everything's going to be really nice and simple.
AUDIENCE: Right.
PROFESSOR: Yes?
AUDIENCE: Bidirectional search.
PROFESSOR: OK.
AUDIENCE: Is that going to be included?
PROFESSOR: At a very conceptual level because we didn't give you a P set on it.
AUDIENCE: Was that what rubrics was?
AUDIENCE: Yeah.
AUDIENCE: It was bidirectional?
AUDIENCE: Well it's not going to be on it so, we're happy.
PROFESSOR: Well I mean we didn't give you-- you kind of had to solve a problem just by-- we have to tell you this is bidirectional BFS, go code it up. This is bi-directional Dijkstra, go code it up. So we're not going to ask you anything too fancy on them. So nothing past the P set.
AUDIENCE: Will you have a question like when you were studying BFS, saying how there will be levels of graphs?
PROFESSOR: Yep.
AUDIENCE: Could there be anything about that?
PROFESSOR: So levels of graphs as in the levels in BFS? Or the levels that we're using when we're building graphs?
AUDIENCE: Copies of graphs and then--
AUDIENCE: Transformations.
PROFESSOR: Yep. OK, transformations and layers. Yeah, I think this is important.
AUDIENCE: Any particular tips on what kind of transformations we might encounter?
PROFESSOR: Sure. Problem one has this transformation, problem two--
[AUDIENCE LAUGHTER]
AUDIENCE: Basically, when do we use these? Is it when we are given more than one parameter for a cause.
PROFESSOR: So I have some problems on this but we have to have a deal. You guys have to help me go through the problems fast enough so that we get here. OK? So if you know the answer, say the answer. Don't let me wait. OK.
AUDIENCE: Also, when you run Dijkstra and Bellman-Ford on a graph, with positive edges, they produce the same shortest path weight but might have different trees?
PROFESSOR: Yes, that's conceivable. Because they--
AUDIENCE: It's one of the questions on spring 2011.
PROFESSOR: OK. So first off, they both have positive edges, so they both work, right? If you had to choose, which one would you choose? If you had to choose which are going to run, which one would you run?
AUDIENCE: Dijkstra.
PROFESSOR: OK, why Dijkstra?
AUDIENCE: It's faster.
PROFESSOR: Faster. OK so positive edges, Dijkstra is faster. Now if you're going to run both of them, they relax edges in different orders. Right? So let's say we have this.
I know you guys remember the sequence of diamonds, right? My nice example that shows that the number of paths in a graph is exponential in the number of vertices. So let's have one diamond. 1, 1, 1, 1. S, A, B, T. So say we're looking for shortest path from S. Right?
So the distance from S to S is zero, and everything else starts out as infinity. SA is infinity. SB is infinity. ST is infinity.
Suppose I relax edges in this order. One, two, three, four. What am I going to get? If I relax SA, then the distance from S to A is going to become 1, right? Because it's 0 plus 1 1. And then the parent of A is going to be S.
Now say I relax AT. The distance from S to T is going to be 2. Sorry. Parent of T is going to be A. Right? OK now I'm relaxing this one. This is going to become one and the parent of B is going to become S. And as I'm relaxing this one, nothing happens because I already had a distance of 2.
Now if I relax them in this order instead, so the bottom one first and the top one is afterwards, I'm going to get the different path. Right? OK so what path do I have now from S to T?
AUDIENCE: Right now you have SAT.
PROFESSOR: OK, why SAT? How do you compute the path? Parent pointers, right? Start at T, look at the parent pointer. Look at the parent pointer.
So because I relax them in this order, this path was considered before this path. So the parent pointer of T is A but if I relax them in the other order, the parent pointer could be B.
So by the way, this could happen if you're running Dijkstra or Bellman-Ford. Or if you're running Dijkstra, it's just about how you're going to have the ties separated.
So your priority queue implementation might give you different paths. Does it make sense now? So if the order in which edges are relaxed. That's what defines with path you're going to end up with.
AUDIENCE: OK.
PROFESSOR: OK. Hopefully useful. All right. I saw four hands before.
AUDIENCE: Oh, just overall, how similar is this test compared to previous tests?
PROFESSOR: Less algorithm design, more concepts.
AUDIENCE: Nice.
AUDIENCE: Yes.
PROFESSOR: Well OK, not less algorithm-- so it's not going to be as much out-of-the-box thinking, it's going to be a lot more of what we drilled already. So as a hint, what kind of problem did we practice over, and over, and over?
AUDIENCE: States
PROFESSOR: OK. Graph transformation, right? So chances are you'll see that.
AUDIENCE: Wait, what's graph transformation?
[LAUGHTER]
PROFESSOR: So we start with a complicated problem and reduce it to a shortest path problem or to a shortest length path problem. OK. Cool. So that being said, let's go.
Numerics, everyone's favorite topic. So suppose we're trying to compute this. Cube root of 6,006. And the problem statement says this, you start out with an initial approximation of one, and it wants two approximations after that.
The problem also tells you which function we're going to use. But why don't we make this fun and try to guess what the function will be?
AUDIENCE: x cubed minus 6,006?
PROFESSOR: OK. x cubed minus 6,006 why did I choose this function? Well, not me. Why did you choose this function?
AUDIENCE: It's the root.
PROFESSOR: OK so reason number one-- right? Important. Has to be zero at the answer, because that's what Newton's method gives us. And what else?
AUDIENCE: Multiplication is easy.
PROFESSOR: OK. So it's easy to compute whatever Newton's method wants us to compute. What does Newton's method want us to compute? So given an approximation, xi, how do we get to the next approximation, xi plus 1. Formula. By the way, this formula should be on your cheat sheets. If it's not, no sympathy for you.
AUDIENCE: I don't remember. xi plus f over f prime.
AUDIENCE: Where's the prime?
AUDIENCE: Prime's on the bottom.
PROFESSOR: So yeah, where's the prime? And is this right?
AUDIENCE: Isn't there a constant?
AUDIENCE: Isn't it minus?
PROFESSOR: Isn't it minus?
AUDIENCE: Maybe.
AUDIENCE: There you go.
PROFESSOR: So yeah, if you guys start out with the wrong formula you'd get the wrong answer. Again, no sympathy. So get that on your sheets.
OK so this is what we need to compute, right? You start with an approximation and you have to subtract this. So this has to be easy to compute.
What if I chose instead a very nice function. x-- sorry, x minus. This is clearly zero where I want it to be, right?
AUDIENCE: But we don't know what six--
PROFESSOR: But if I'm trying to compute the successive approximations, very good, I'm getting what? f of x over f prime of x is x minus square root 3 6,006 over-- what's the derivative of this?
AUDIENCE: One.
PROFESSOR: One. So I haven't done anything, right? In order to compute the successive approximation, I have to know this number. So this doesn't get me anywhere, that's why it's a bad function.
By the way, it's not enough that this is easy to compute. What you want is this guy has to be easy to compute. In the case of division, we had a function that looked pretty wacky. But then when we divided that by the derivative, we got something reasonably nice. So this has to be easy to compute.
AUDIENCE: So we changed it from that one to this one just because this one is easier or--
PROFESSOR: So we're using this one because this one-- let's see how easy this one is to compute. Computing this one requires that you know the answer to your original problem, which is not cool. Right.
So you're trying to compute this number. But if you use this, Newton's approximation requires that you compute this. So you have to know this here. Which is what you're trying to do in the first place.
AUDIENCE: Wait, I'm confused. We went from x cubed minus 6 over 6 to x minus cube root of 6 over 6. So what's your--
PROFESSOR: So this is a good function. We can use this. This is a bad function and I was asking why is this a bad function.
AUDIENCE: Oh, OK.
PROFESSOR: OK. So lets see, if we try to compute it using this, what do we get? xi minus
AUDIENCE: x cubed minus 6,006.
PROFESSOR: X cubed minus 6,006. Divided by--
AUDIENCE: 3x cubed.
PROFESSOR: OK. So someone simplify this for me.
AUDIENCE: 6i minus x over 3 plus 6,006 over 3 squared. Oh, well. It's 2,002.
PROFESSOR: I'll choose the easy step. OK. So what's x1?
AUDIENCE: You plug in x0.
PROFESSOR: OK. I heard a 2,002. I don't think it's-- it's very close.
AUDIENCE: 2,002 and two thirds. 0.67.
PROFESSOR: OK, cool. So 0.67, let's get to that later. I don't like fractional numbers. I mean this is the right answer math-wise, but we'll get to that because if we want to code this up, we probably don't want fractional numbers. So very good point there.
What if I want to compute x2? I take this guy and I plug it into where? This thing over here, right? So this way I can compute approximations that get closer and closer to my original value. OK. Does this ring a bell? Yes, everyone is happy with this? Cool.
So how about the initial guess? Here we started with a known initial guess. Is this a good guess?
AUDIENCE: It's a really bad guess. 2,002?
PROFESSOR: No, I mean 1.
AUDIENCE: 1? That's also a really bad guess.
AUDIENCE: Why would you even ask?
AUDIENCE: 11 and a half.
PROFESSOR: All right. So you're saying 11 and a half would be a nice guess. So, first off, let's see, what do we need to-- what does Newton's algorithm guarantee? What-- if the guess is really, really bad, would we get an answer all the time?
Maybe takes forever but we'll get an answer. True or false?
AUDIENCE: True.
PROFESSOR: Not true. Sorry.
AUDIENCE: But it has to be right for your answer to be--
PROFESSOR: OK, so what does Newton's method guarantee?
[INTERPOSING VOICES]
PROFESSOR: Yep, yep. So what is the fancy math name for that?
AUDIENCE: Quadratic convergence or something.
PROFESSOR: Quadratic convergence, all right. So this is what Newton guarantees. Quadratic-- so what does this mean? For all your approximations, they're all going to be xi.
If we write them as true answer times 1 plus error, i. So this error is the relative error of the approximation. Newtons method guarantees that the error at each step is squared.
So if this guy-- if E0 is greater than 1, would we get an answer?
AUDIENCE: No.
PROFESSOR: OK so what we need? What's the minimum that we need? E0 has to be between what and what?
AUDIENCE: Negative 1?
AUDIENCE: Is it negative 1, 1 or 0 and 1?
AUDIENCE: It would be 0 and 1, right? Because won't it be less than one?
AUDIENCE: Negative 1.
AUDIENCE: Oh, absolute value.
PROFESSOR: I think if you score something smaller than negative 1 you get a positive and then-- so, let's say this works. So then x0 has to be between what and what in relation to the real answer?
AUDIENCE: The real answer minus x0 over [INAUDIBLE].
AUDIENCE: 2 times the actual answer is 0.
PROFESSOR: OK so this is a sucky guess. We can do a lot better, but at least it will make the thing converge. Fair enough?
OK now what if I want a good guess? First off, let's backtrack to a more general problem because this is easy, right? To plug it into our calculator and you have the answer.
So what if I'm trying to compute cube root of r. Can everyone see, by the way?
AUDIENCE: Yes.
AUDIENCE: Mhmm.
PROFESSOR: OK. So I'm trying to compute cube root of r. r is a number. Suppose I'm trying to compute, for example, cube root of 2. What's the first thing I need to do?
I'm going to code this up, by the way. Well, not me but suppose we want to code this up. What's the first thing we want to do?
AUDIENCE: I think we want an initial guess at a function.
PROFESSOR: OK so we're going to need an initial guess. That's good. And what else do we want?
AUDIENCE: We need to decide how much precision we want.
PROFESSOR: Yep. And why is that?
AUDIENCE: Because this cube root of 2 is 1. If you round down. That doesn't tell us very much. I don't know, we don't like the fractions.
PROFESSOR: We don't like fractions. OK, why don't we like fractions? It's a lot easier to code.
AUDIENCE: Decimal point there.
PROFESSOR: Yep, you have to deal with the decimal point and we don't like that. So we want to take this problem, and we want to move it into integer land. So what we did here has fractions. We don't like that because that's a pain to code.
We didn't make you code fractions on the P set, right? So then we better know how to avoid fractions if you want to solve real life problems.
So we're going to say that we're happy with some amount of precision in the answer. And then we're going to do something that lets us do all our computations in integer land. Because that's a happy place.
So how do I-- suppose I'm working in base B and I want d digits of precision. What do I want to compute? How do I transform the problem in that way? Yes?
AUDIENCE: You multiply 2 root 3 times B to the d.
PROFESSOR: OK so we want the answer to be 2 times B to the d. And if we're integer land, what are we actually going to get?
So suppose B is 10-- let's work with familiar numbers-- d is 2. So we're going to want to compute square root of 3 2 times 100. Does anyone have a calculator and can tell me what square root of 3 is? First two digits?
AUDIENCE: Cube root of 2.
PROFESSOR: Sorry, cube root of 2.
AUDIENCE: 1.2.
PROFESSOR: So let's say that square root of 3 is 1.2345. Hopefully someone will fix this up for us. But let's say it's this. If I multiply it by 100 what do I get? 123 times 45.
So multiply by 100 means you move the decimal point to the right. Right? So it's still not an integer. If we work in integer land, our algorithm will return an integer. What's the integer that we're hoping our algorithm will return?
AUDIENCE: 1.23, right?
PROFESSOR: We're hoping so, right? Why? What can you do with 123? Shift right. So if you divide this by 100, you get 1.23. And this is our answer with two digits of precision.
AUDIENCE: Do you want the actual-- it's 1.2599.
PROFESSOR: You guys are good. OK. So our algorithm will give us this, right? So it's not going to give us exactly cube root of 2 times 100. It's not going to give us exactly this value. What's it going to give us?
AUDIENCE: Two digits-- close to that.
PROFESSOR: So it's going to give us an integer, right? So what kind of integer? Math.
AUDIENCE: Truncated?
PROFESSOR: OK, what's the math for that? Truncated. Floor. Very good. So this is what we're actually going to compute. Actually, because we're in integer land, we're going to have floors at every step when we're doing that approximation. And there's a fancy proof for Newton that says that even though you're taking floors all the time, it will still converge to the right thing.
So in order to compute this, how are we going to transform the problem?
AUDIENCE: Slide the Bd into that.
PROFESSOR: OK.
AUDIENCE: You're going to have to put a 3 in there. B to the 3d.
PROFESSOR: So we're actually going to compute cube root of 2 times B to the 3d. Fair enough? And if we slide this right by the digits afterwards we're going to get our two digits of precision. OK.
So now I figured out my precision. I want an initial guess-- and actually I lied, there's one more problem. Running time. Do you guys want to start with the running time or initial guess?
AUDIENCE: Initial guess.
PROFESSOR: OK so what I want a good initial guess?
AUDIENCE: Between 0 and 2x.
PROFESSOR: OK so if I don't have that the algorithm will crash and burn. When we did Newton in recitation, I said that I would like two things. And I backed up, I coded that into P set five when I did the guess. So what are the two things that I want from a good initial guess?
AUDIENCE: Order of magnitude?
PROFESSOR: OK. Perfect.
AUDIENCE: Oh, god. [LAUGHS]
PROFESSOR: OK, so order of magnitude is a fancy CSE way to say, right number of digits. Suppose we're computing this. Or suppose we're computing the cube root of some number in base B. So supposed r is somewhere between 0 and B. It's one digit in base B. How many digits is this result is going to have?
AUDIENCE: The amount of digits divided by 3. The amount of digits in 2 times B to the 3d. Which is whatever B to the 3d is.
AUDIENCE: D plus 1?
PROFESSOR: OK so roughly either d or d plus 1. See, d plus 1. So a decent approximation would be a 1 followed by these zeros. An even better approximation is what if we can get the first digit right or almost right? How would we do that?
AUDIENCE: Pick up the first digit of our r
PROFESSOR: OK and I promise that r is one digit. Just So let's say we pick up r. And?
AUDIENCE: We do Newtons method on that. And we just guess one? Is that a thing?
PROFESSOR: Uhh--
AUDIENCE: Take the cube root of it.
AUDIENCE: It should be some kind of inefficient--
PROFESSOR: Yeah what's some kind of inefficient way that we can think of quickly?
AUDIENCE: Can you--
AUDIENCE: Binary?
PROFESSOR: Did you say divide and--
AUDIENCE: --divide it by 2r?
PROFESSOR: Oh, sorry. I thought You were>you were going to say divide and conquer. So I heard binary search, so binary search wins. So we're going to the binary search. Binary search from 0 to r. Right? In order to the binary search, suppose I have my guess, g, I want to see how g compares to cube root of r. How do I do this?
So this is equivalent to g cubed compared to r. g cubed is to multiplication, so I know how to do that. OK? Make sense for everyone? Sorry, r. My bad.
So this is going to be one digit in base, B, so hopefully not too much work. OK. But remember this, you can;t do the comparison directly because you can't compute this directly. So you need to do it this way.
AUDIENCE: So r is just the first digit of the number?
PROFESSOR: Yep. So we're going for a slightly easier problem. We're trying to compute the cube root of a single digit number to an arbitrary precision. You can make it harder, but there's no point right now. So we'll stick to this.
So how much time will this take? How many approximations will I need to do? By the way, so suppose I compute my approximations. Right? So I compute x0, x1, x2, x3, so on and so forth. When do I stop?
AUDIENCE: When both digits are the same?
PROFESSOR: All the digits are the same. So when one approximation is the same with the next one. That's what you're? Right.
So these are all integers. The moment I get two identical integers, I know there's no point to continue because I'm going to get the same value forever. Also this means that Newton's method has converged on this integer. So hopefully I will get the answer, which I can convert into the right answer.
OK so how fast does this work? What is n? When am I going to stop?
AUDIENCE: Log base 2 of d?
PROFESSOR: OK. So n is-- so the number of digits in my approximation-- the number of correct digits-- doubles every time. So after log these steps, I have the right answer.
Now what if instead of doing this I did binary search? So instead of doing Newton's method I do binary search on 0 to r? I can do that, right? Because I can get a guess and then I can use this to see if my guess is right or wrong.
So in theory I could do it in binary search. How fast would that be?
AUDIENCE: And what is the difference between big r and little r?
PROFESSOR: Big R is-- oh, I didn't write the big r. Big r is this guy, here.
AUDIENCE: Oh, OK, gotcha. OK.
PROFESSOR: And this is little r. Sorry. Good question, I apologize for that. So R is little r times B to the 3d.
AUDIENCE: D times log 2 of B--
AUDIENCE: To the d. Log r to the d.
AUDIENCE: Oh, it's the same thing. Right.
AUDIENCE: Yeah. On the y.
PROFESSOR: OK so the number of guesses is
AUDIENCE: Log big r.
PROFESSOR: Log big r. And someone was one step ahead and said it's order of B times log little r. Or I think it's d plus little r. So this is order of d. Does this make sense for everyone?
AUDIENCE: I didn't quite follow what happened there.
PROFESSOR: So I'm doing a binary search on the range from 0 to r. How many steps? How many guesses? Log r. Right? This is binary search. R is little r times b to the 3d.
So if we log this, log R is log little r plus 3d log B. B is order one, so we get order of log B.
OK so Newton's method is exponentially faster in terms of the number of digits compared to binary search. So if you can pull it off with Newton's method and you have big numbers, you probably want to use Newton's method. Because binary search will give you a slower algorithm.
OK, how are we doing with these concepts? I see everyone's unhappy. Is it because of Newton or something I said?
AUDIENCE: Can you summarize the difference between binary search and Newton's, again? So you said binary search takes kind of long versus Newton's method.
PROFESSOR: OK. So how do you do binary search?
AUDIENCE: Divide and conquer, which is--
PROFESSOR: Divide and conquer, right? You start out with an interval, you guess somewhere in half, and then you see which half of the interval you recurse onto. And your interval halves in size every time. So given the initial size of the interval, the number of guesses you have is log of that interval size.
In this case we're guessing a number between 0 and r. So the big goal is to compute cube root of R. And we're guessing that hey, it has to be somewhere between 0 and R. So we're going to do binary search on this.
And in order to see how our guess compares, we're going to use this trick, here. So the number of guesses we make is log R. Now R is that number, over there. It's little r times B to the 3d.
So how many digits does it have? Roughly d digits. So log of R is going to be order d.
OK so if you do binary search to guess a d digit number, the running time will be roughly order d. Order of the number of digits. If you use Newton's method and your function is good and everything converges, then you have quadratic convergence. Which guarantees that you'll have log of d guesses. Log of d approximations.
So Newton's method is a lot faster for big numbers, because you have a log there instead of d. So this is the big difference between them. But for Newton, you need a lot of things. Right? You need that function, you need an initial guess, you need a lot of things to make it work. Yes?
AUDIENCE: So is that just like the number of times it runs? Because don't you have a multiplication and a division?
PROFESSOR: Yep. So this is the number of guesses. The total running time is quite different. You need to jump through a few more hoops to analyze that. But this is the number of guesses or approximations that each method makes. So if your math would be order one, this would be the running time. If the math isn't order one, then it gets more complicated.
AUDIENCE: So what's the running time of a division?
PROFESSOR: What is running time of the division using Newton's method? It's the same as the running time of the multiplication that you're using to compute your approximations. And it's like two pages of notes to prove that. So let's not go over that now.
But the idea is that every time you're multiplying, you start multiplying small numbers and the numbers double in size when you're multiplying them. So only the last multiplication counts. All the others are tiny compared to that.
OK. Anything else about Newton? Sorry guys, we have Newton on the exam so we have to go through this. Let's go through some fun graph problems.
OK so we kicked off these two. Graphs the BFS edges and graph transformations.
OK I'm going to do a graph transformation first because I think that's more useful than BFS edges, but hopefully I'm going to get to both.
So suppose I have a graph. Each path is either red or blue. By the way, no political connection whatsoever. And you have weights on each path. So we want a path from S to T with the following constraint.
So these weights are the costs of, maybe, how much gas you're spending to go on that road. So in order to switch from a red edge to a blue edge, you also have to pay some money. You have to pay a cost of 5. So if you're going to go-- if you're going to go from S to A-- let's call this guy A-- if you're going to go this way, then your total cost is 3, 1, 4.
If you're going to go from S to B, then if you go this way, your total cost is going to be 3 plus 1 plus 5 for switching from a red to a blue edge. So the cost for switching from red to blue is 5. The cost for switching from blue to red is 5.
And I'll let you guys think of it for a bit while I erase the board.
AUDIENCE: What's your goal in playing the best path from--
PROFESSOR: Best path from S to T. Smallest total cost.
AUDIENCE: OK.
PROFESSOR: So any thoughts? Yes.
AUDIENCE: It's kind of on the run, but create a supernode connect all the red paths. And create another one connecting all the blue paths. And then try to find the shortest length to T just using those two graphs, I guess.
PROFESSOR: So you're going to create a supernode connecting the paths. So how does this work?
AUDIENCE: You create the path from S to T just using the reds.
PROFESSOR: OK so I'm going to copy everything and use the reds. So S, C, D, T, and I'm only going to use the reds. All right, red, red, red. OK.
AUDIENCE: And then-- wait, is that all the right paths?
PROFESSOR: I hope so.
AUDIENCE: All right, and then you're going to try doing that with the blue paths as well.
PROFESSOR: So am I creating another copy of this?
AUDIENCE: Yeah.
PROFESSOR: OK. Let's see, what else, what else? OK
AUDIENCE: From S to T, right?
PROFESSOR: A path from S to T?
AUDIENCE: Yeah, so you just compare the path of S to the path of T. So you completely take that out, the additional cost [INAUDIBLE] just by comparing those two and seeing which ones are the shortest path.
AUDIENCE: It's correct for this graph, but it's not correct in general.
[INTERPOSING VOICES]
AUDIENCE: And the last option would be combining--
PROFESSOR: OK so if I do this so far. So I've taken the original graph and I've created two copies. One that has all of the red paths, one that has all the blue paths.
AUDIENCE: Right.
PROFESSOR: By the way, let's label this. The nodes are--
AUDIENCE: So it would work in this specific case because you [INAUDIBLE].
PROFESSOR: So if you compute the two shortest paths here, you'll get the path using only reds, path using only blues. But you're not expressing the fact that you're allowed to alternate between reds and blues. How do you express that?
AUDIENCE: Make a lot of corrections.
AUDIENCE: Put two layers in--
PROFESSOR: Two layers. OK so these are two layers. This is the red layer, and this is the blue layer. And how do I connect them?
AUDIENCE: If there's an edge connecting them on the upper graph, we're just connecting them.
AUDIENCE: Or connect the respective nodes and make it a 5.
PROFESSOR: Both answers work, they have the same number of edges. The last answer is easier to visualize, so I'm going to go with that. So I can go from the red world to the blue world, and back, if I'm willing to pay 5. So this is what--
AUDIENCE: And you just use those with the new edges. Or not DFS, but Dijkstra's.
PROFESSOR: All right so all the edges here are positive, right? So I'm going to use Dijkstra.
AUDIENCE: Is there any case in a positive graph that Dijkstra's slower than Bellman-Ford? Because one is like b log b, and the other one is like b e. Right? So if you have a lot of--
PROFESSOR: OK so what is Dijkstra?
AUDIENCE: B log b-
PROFESSOR: Almost.
AUDIENCE: T log b, I think, right? That's the theoretical best case ever,
PROFESSOR: So actually, the theoretical is E plus--
AUDIENCE: Yeah, that's what I meant.
AUDIENCE: Oh. Oh--
PROFESSOR: This is what you get with heaps, so this is what we got in the P set.
AUDIENCE: What was the first one?
AUDIENCE: The first one is like Fibonacci heaps. It's got a really high constant factor, so you never actually use it.
PROFESSOR: So this is a nice theoretical thing, and this is what you get in practice. If you use the regular binary heaps. And so you compare this to Bellman-Ford which is-- whoops-- V times E. I'm going to guess that this is faster no matter what.
AUDIENCE: Yes, OK. So the theoretical one could be slower, then, possible. The Fibonacci one? Because if you have a ton of vertices without many edges.
PROFESSOR: OK If you have a ton of vertices but not too many edges.
AUDIENCE: Yeah.
PROFESSOR: Then you're saying that this is going to be bigger than-- so what do you do in that case?
AUDIENCE: I mean you could just ignore the vertices, I guess.
PROFESSOR: OK so if you have a ton of vertices and not that many edges. So if you have edges smaller-- sorry-- yeah, it's just smaller than vertices, then some vertices have to be unconnected. So what to do?
AUDIENCE: You just ignore them.
AUDIENCE: You're not going to reach them anyway.
PROFESSOR: How do you ignore them? How do you ignore them in--
AUDIENCE: Oh, I see your point. So you're never going to get to them anyways. So--
AUDIENCE: Well no, but once you've started at one, you're going to have to touch them all. They're all going to be in the heap. Yeah.
AUDIENCE: No, don't put them in the heap until you look at them.
AUDIENCE: Well, there's a starting point, right? If you can't go anywhere in the starting point, you're done.
PROFESSOR: OK so you take the starting point and you do--
[INTERPOSING VOICES]
PROFESSOR: Ok so I would take the starting point and do a BFS and ignore everything else. And that is clearly order of how many vertices and edges that are reachable from that. So that will give you a nice graph. Either that, or if you initialization your Dijkstra carefully enough, this actually only reflects the reachable edges and vertices. So you never have this. So either one works.
In theory mode, it might be better to say I'll do a BFS and reduce the graph. Because that's easier to argue. Yes.
AUDIENCE: So, for this, when you make all that-- I guess my question is are we going to be asked to talk about running times for different transformation? Or the algorithms?
PROFESSOR: Well so at the very least, last time we said, this is the problem. Give us an algorithm with this running time.
AUDIENCE: Right.
PROFESSOR: So you have to be able to know your transformations. So not the running for computing the transformation, but you have to be able to know how much bigger the graph gets and what that implies for the running time.
Turns out that usually for these transformations you can compute them in linear time. So the time for computing a transformation is much smaller than the time for running Dijkstra or Bellman-Ford. So that's why we don't really go into that. This one is definitely linear time.
So what happens to the graph in this case? Number of edges and number of vertices.
AUDIENCE: Number of edges increases by V and number of vertices. So increase by V.
PROFESSOR: That's V, and times is 2. So you plug this into Bellman-Ford or Dijkstra, you find out the new running time. So every time you see what is the size of your new graph, and you plug that into the algorithm.
OK so what is the intuition behind this? Did everyone get this problem? So it's the old problem that we're going through again and again, where you have a graph that's 2D, and we want to compute something that Dijkstra can't compute on it's own. Or that Bellman-Ford can't compute on it's own.
So in order to be able to compute those things, we need to add additional states to the graph. And the way we do that is we make copies of the graph that we call layers. Because we're thinking that if you take that 2D map, and you create copies of it, you basically have a 3D graph where there is the original graph.
That's the science part of the problem, the art part of solving the problem is figuring out what those layers are and how you connect them. Because by doing that you can solve a ton of problems, as we have seen in this class.
AUDIENCE: Is there a possibility that you have a very large number of layers needed to be permutations of-- different choice to make instead of just a red or a blue?
PROFESSOR: Yep. So is there a problem where we had a ton of layers?
AUDIENCE: Mhmm.
PROFESSOR: Yep. OK so two problems, right?
AUDIENCE: One for every second. [LAUGHS]
PROFESSOR: And so these are the layers, presumably. We had two problems that had a ton of layers. One of them was the highway problem where you had timetables. And there, the number of layers was the number of minutes in the day you're considering. So roughly, 1440.
And the other one was StarCraft where the number of layers was something ginormous, right? So as long as that fits the theory, that's fine. As long as the number of layers fits whatever the problem wants you to compute, its OK.
AUDIENCE: So transforming another layer, is that like in V time because copying the number of vertices?
PROFESSOR: Yeah, so you should be able to implement this in order of V plus E, which is what you need to output the new graph description. I claim that for this you can.
AUDIENCE: So for E prime, I guess, how'd you get that?
PROFESSOR: So for each vertex you are going to create a red copy and a blue copy. 2V, this is easy. Now, the red edges stay in here, the blue edges stay in here. So nothing changed so far. But each red vertex needs to be connected to the blue vertex by an edge of weight 5.
So that means that we're going to copy over the original edges and we're going to add the edges that connect the vertices.
AUDIENCE: [INAUDIBLE].
PROFESSOR: So we're not going to ask about the running time of the transformations in general. Because we assume that they can be done in linear time. But you need to at least have a sense of whether your graph is going to double, whether it's going to increase exponentially, or what's going to happen to it.
So let's make a small tweak to this problem. Suppose that the instead of having this, I can go from red to blue, but once I've gone from red to blue, I can't go back. So I can start out either red or blue, I can go red to blue, but once I'm in blue I can't go back to red.
AUDIENCE: Make those directed edges.
PROFESSOR: From where to where?
AUDIENCE: Red to blue.
PROFESSOR: OK so this is how I express constraints, in constraints among layers. Before, we had two layers, red and blue. And we connected them by an edge of weight 5, which says you can go from red world to blue world, and back, all you have to do is pay 5.
If we have directed edges, then this is a constraint. If the constraint said there's no cost, but you can only go from red to blue, you still have to do two layers. And keep track of which layer you're in. But then your edge of the weight 0.
Since we have to pay, the edge is weight 5. So we can use layers to express additional costs, or just to express constraints. Does this make sense?
AUDIENCE: Does this include back edges, or forward edges, as well?
PROFESSOR: Let's get to that. OK, so does this make sense so far? One more question, what shortest path do I want to compute here? To make sure that you guys got it.
AUDIENCE: Oh, you have to do two of them maybe, four--
PROFESSOR: OK, I like four of them. So I have to do SR, TR, SR, TB, SB, TR, SB, TB. Fortunately, our algorithms give us the shortest path from one source to all of the other vertices. So I'd only have to run Dijkstra or Bellman-Ford twice.
What if I want to run the algorithm once? What do I do?
AUDIENCE: You put vertices on the edges.
PROFESSOR: OK so supernode as a source, supernode as a destination. And I connect them to what?
AUDIENCE: Zero weight.
PROFESSOR: To?
AUDIENCE: The two.
AUDIENCE: Final V.
PROFESSOR: OK, very good. And?
AUDIENCE: Same.
PROFESSOR: So what happened here? This says you can go anywhere. And this says you can come back from anywhere.
AUDIENCE: Bridge between the worlds.
PROFESSOR: Yep.
[AUDIENCE LAUGHING]
PROFESSOR: So do we want this to be directed or undirected, by the way, does it matter?
AUDIENCE: Directed. You can only go one way--
AUDIENCE: Oh, depends on which problem you're talking about.
PROFESSOR: I think if they're undirected, it shouldn't matter too much, because there shouldn't be a path where you go back to the source and then you switch. But this would be if you're on a quiz and you don't want to think about that, I'd make them directed just to be on the safe side.
AUDIENCE: What were the four shortest paths you said we needed to calculate for the original problem, before you added the supernodes?
PROFESSOR: So we don't know whether we start out with a red edge--
AUDIENCE: Right.
PROFESSOR: --with a blue edge. We don't know whether we end up with a red edge or a blue edge.
AUDIENCE: Stay in red world, stay in blue world, red to blue and blue to red.
PROFESSOR: Yeah OK, cool. So let's talk about BFS and DFS very briefly, I guess. So BFS and DFS. What does BFS give us? Why is it useful?
AUDIENCE: Shortest paths.
PROFESSOR: Shortest paths in terms of number of edges, right? No weight on the engines. So shortest paths using the number of edges. What does DFS give us?
AUDIENCE: Topological start.
PROFESSOR: Hey, man, you had this on the P set, I wouldn't call it nothing. OK, how does BFS look at the graph? How does it partition the graph? Nodes are grouped into--
AUDIENCE: Levels.
PROFESSOR: Levels.
AUDIENCE: The light distance and the source.
PROFESSOR: So you start with a source, and then all the nodes that are one edge away are at level one, all the nodes that are two edges away are level two, so on and so forth. What does DFS give us?
A mess? A mess, right? So it gives us edge types. And it gives us exit times. Exit times are useful for topological sort, right? Yes.
AUDIENCE: It also gives us a tree.
PROFESSOR: OK, does BFS give us a tree?
AUDIENCE: Yes.
AUDIENCE: Yes, they both give us trees.
PROFESSOR: So both of them will give us trees. So what are those trees? For each node, BFS or DFS discovered that node by going from some parent node across an edge. So that edge belongs to the BFS or to the DFS tree.
How do we compute those trees?
AUDIENCE: Just do it?
PROFESSOR: So how do you, in BFS and DFS, what do you compute to--
AUDIENCE: Parent pointers?
PROFESSOR: Parent pointers. So that's what you use to keep track of them. Sorry the question is bad but that's what I want you to get out of it. Parent pointers.
So DFS and BFS will both compute trees of the graph. Are they the same trees or different trees?
AUDIENCE: Probably different.
PROFESSOR: Different trees, right? Let's take this. S, A, B, right? BFS will give us this, DFS will give us this.
OK, what edge types do we have in DFS?
AUDIENCE: Forward edges.
PROFESSOR: OK, forward. Cool. What are they?
AUDIENCE: Those are just from parent to children that are being presented.
PROFESSOR: OK.
AUDIENCE: So, I don't know how else to put it.
PROFESSOR: OK so you should have this on your cheat sheet, right? If nobody knows the answer, you should have it on your cheat sheet. So tree edges, the ones that show up in the DFS tree. Backwards edges,
AUDIENCE: Cross edges.
PROFESSOR: Cross edges.
AUDIENCE: Forward edges.
PROFESSOR: And forward edges. If your graph is undirected, what types of edges do you not have?
AUDIENCE: Forward edges. Forward edges and backward edges. Or no, forward edges.
PROFESSOR: OK. So this is a lecture notes, and we're going to go over them tonight. But if you can't make it, they're in lecture notes. So you should have this on your cheat sheet if you don't know them. Right?
Nobody answered today, so if you guys don't have them on your cheat sheet I will be upset. OK. Cool. More questions?
AUDIENCE: Edges do not exist in BFS, edge types?
PROFESSOR: DFS uses-- so out of BFS we get levels, and out DFS we get those edge types.
AUDIENCE: Is there-- about the edge types?
PROFESSOR: Some algorithms use them in their proofs. So the proof for topological sort uses the fact that in directed acyclic graphs, you won't have some types of edges. For the other types of edges it argues that the order that you get from topological sort is the right order.
So it's mostly theoretical but since we taught you about edge types, we might ask you about them. Yes.
AUDIENCE: So does DFS actually-- is it able to distinguish between back-crossing over, or is it only-- does it only see that the node has already been visited by something?
PROFESSOR: So how would you make it distinguish between them? You have to, right? Because otherwise, why are we studying them? There has to be a way to distinguish between them.
AUDIENCE: Because the DFS does recursive calls and you know where the back is.
PROFESSOR: So let's do a DFS tree quickly. So suppose we went like this. And then like this, this, and then this. Sorry, I'm trying really hard to make up an example on the spot such that I won't discount myself. How do I get that, do you know?
AUDIENCE: Maybe an edge from the second one to the last one on the right.
PROFESSOR: Here?
AUDIENCE: Yeah and this will be the arrowhead, and you can start from the second one.
PROFESSOR: This one?
AUDIENCE: Yeah. Wouldn't that be a forward edge?
PROFESSOR: OK
AUDIENCE: Because it's downward--
PROFESSOR: Yeah, I like that. OK, I like that. OK so S, A, B, C, D, E. So assume this is the order that they listed in adjacency list. So let's label all of the edges. SA and SB are what?
AUDIENCE: Forward edges.
PROFESSOR: OK AC?
AUDIENCE: Forward.
PROFESSOR: CD?
AUDIENCE: Forward.
PROFESSOR: CE?
AUDIENCE: Forward.
PROFESSOR: OK, AE?
AUDIENCE: Aren't those tree edges?
AUDIENCE: That's a forward edge.
PROFESSOR: That took a while, guys. That took a while.
AUDIENCE: It goes forward.
AUDIENCE: Well no, forward means like you're skipping a generation.
PROFESSOR: OK so the three edges.
AUDIENCE: [INAUDIBLE]
PROFESSOR: So the three edges are the ones that DFS uses to go forward. So they're the ones that map the DFS calls. So how do we express that? So suppose you're at some node u, and you have an edge, uv.
AUDIENCE: The parent of v is u.
PROFESSOR: So you're at here, right now. OK, so v.parent has to be u. And? v is? Is it visited or not?
AUDIENCE: It's a new vertex.
AUDIENCE: It's not.
PROFESSOR: So v is not visited yet. So this is a tree edge. Now let's compare this to a forward edge. So what happens to a forward edge?
AUDIENCE: Parents of children--
AUDIENCE: Parent ancestors--
PROFESSOR: So you're at here right now. And you're looking at the edge uv. Right? so we're looking at the edge uv. uv would point downward in the tree. What's true about--
AUDIENCE: v.parent is u.
AUDIENCE: No--
AUDIENCE: Not necessarily.
PROFESSOR: OK. So v.parent is u. So it can be the parent, or the grandparent, or-- so u has to be somewhere up the tree. Right? So I can have a ton of dot parents here.
AUDIENCE: There has to be more than one dot parent, right? Because otherwise we get tree edge. It can't just be one parent.
PROFESSOR: OK.
AUDIENCE: So you'd have to recurse up more than once.
PROFESSOR: Yep. So for a node you go up until you find u, and if you found u, then it's a forward edge, otherwise, if you find the root of the tree then you give up.
AUDIENCE: Well then it's a cross edge. If you go up and you get to the root but-- oh--
PROFESSOR: Yeah, so if you got to the root, it is not the forward edge. Right now we're looking at what does it mean. So you have to be somewhere around the ancestor chain of u, so if you keep following v's parents, you have to see u. If not, it's not the forward edge.
OK. And when you see it, did you visit it or did you not visit it?
AUDIENCE: Already visited.
PROFESSOR: OK. So now we have two more edge types, we have back edges, and we have cross edges. Right? Show me a back edge here.
AUDIENCE: DA.
PROFESSOR: DA is a back edge. OK back edges are also reasonably easy. So let's do those. What's a back edge. So I am at u now. I'm looking at the edge uv.
AUDIENCE: It's a cycle.
AUDIENCE: Node to ancestor.
PROFESSOR: Sorry?
AUDIENCE: It's a node to an ancestor.
PROFESSOR: OK so who is who's ancestor?
AUDIENCE: A is D's ancestor.
AUDIENCE: V is u's ancestor.
PROFESSOR: OK. So if I keep going, u.parent many times over, I should eventually see v. Uhh-- yeah. So the difference between this and this is who is who's parent?
AUDIENCE: Wait, wouldn't u be a parent of v? Because we didn't go from A to C to D and then at D I realize that I can go to A, but I won't go because it's already visited?
PROFESSOR: Wait.
AUDIENCE: But assuming that--
AUDIENCE: A is an ancestor of D.
PROFESSOR: So A is an ancestor of D because by the time I got to D, I've already set the parent pointers for C and D.
AUDIENCE: Yes. But-- wait. u.parent is v. Then u is A here and v is D here--
AUDIENCE: No u is D here.
PROFESSOR: I'm at u right now, so I'm at D. And I'm looking at the edge uv.
AUDIENCE: Do we have a cross edge in here?
PROFESSOR: Do we have a cross edge in here. Good question. What is the cross edge?
AUDIENCE: C?
PROFESSOR: OK so this guy is a cross edge. So what is the difference between a cross edge and a forward edge?
[CLASS MURMURS]
AUDIENCE: It's not an ancestor.
AUDIENCE: Ancester goes up to S.
PROFESSOR: So a cross edge is very close to a forward edge except u is not an ancestor of v. v in the DFS tree. OK?
AUDIENCE: [INAUDIBLE]
PROFESSOR: Yep. Yep. So the only common ancestor between B and C is the source. OK so please get these conditions in the notes.
So as a summary, if it's an edge that DFS uses, it's a tree edge. If it's going against the way of the DFS, it's a back edge. If it fast forwards in DFS, so instead of going one level down, it does multiple levels down, then it's a forward edge.
And otherwise, it's weird edge so it's a cross edge. One way to look at it. Yeah.
AUDIENCE: Is this only true because we were going alphabetically?
PROFESSOR: Yeah, this depends on the order in which the nodes are listed in the adjacency list.
AUDIENCE: So it could've been pretty different if we went from A to E?
PROFESSOR: Oh Yeah, yeah. Yep.
AUDIENCE: I'm trying to solve this another way. The graph information--
PROFESSOR: Yeah
AUDIENCE: --would you say that's moderate or an easy example? The transformation.
PROFESSOR: This is on the easy side. So look at the problems that we had so far. There are more problems in this review packet. There are like six, seven, eight problems. It's going to be online. It's the notes for this recitation. Sorry, bad name. So the notes for this recitation.
This is on the easy side. The StarCraft problem, last time is on the insanely difficult side, so that's not going to happen.
AUDIENCE: I so don't even get that.
PROFESSOR: That's on the hard side.
Free Downloads
Video
- iTunes U (MP4 - 141MB)
- Internet Archive (MP4 - 141MB)
Subtitle
- English - US (SRT)