Flash and JavaScript are required for this feature.
Download the video from iTunes U or the Internet Archive.
Description: Students learned all the steps in the Life Cycle Analysis to quantify environmental impacts and products, and how to apply it to make sustainable decisions when facing uncertainties.
Instructor: Prof. Anthony Craig
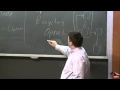
Lecture 5: Life Cycle Analysis
The following content is provided under a Creative Commons license. Your support will help MIT OpenCourseWare continue to offer high quality educational resources for free. To make a donation or view additional materials from hundreds of MIT courses, visit MIT OpenCourseWare at ocw.mit.edu.
PROFESSOR: OK, let's go ahead and get started. We're actually not going to be joined by our friends in Zaragoza today, but we're still going to be recorded. So still have to stay on our best behavior, I guess. Today we're going to go through life cycle assessment, and talk about that and how we use it to quantify environmental impacts of products.
The goal today is pretty simple. First is to lay understand the steps in the LCA, exactly what is the methodology, how it's used, the specific steps in it and what they mean, what goes into each of them.
But really, what we're also going to spend time on is understanding the decisions that go into that, using the hand dryer study as an example to go through and understand how you link all these different steps together, what it means in understanding the decisions that you have to make as a person doing this or as a company, in terms of setting the boundary, understanding what your goal is, and all the uncertainty that goes along with all the decisions and assumptions that you have to make along the way.
Last time when we were talking about carbon footprinting, we were talking really about things that go on at the corporate level. Within the boundary is essentially drawn around your organization.
We talked about how you could use those internally, doing things like trying to evaluate different departments in your organization, tracking your performance, setting up some kind of internal metrics. All the standard sort of things you would do for financial cost, quality, sustainability, really just one extension of that. But also talking about its relation to external, you're publishing a corporate CSR report, participating in programs like the Carbon Disclosure Project, reporting to regulators, investors, et cetera.
And one of the things we've talked about was the difficulty in comparing between organizations that might be doing different activities. Even though they operate in the same business space, they might produce some of the same products, it's very difficult to compare two organizations based on their organizational level reporting, because they might control different aspects of the supply chain. They may do different things, even if they're producing the same products.
Today we're going to talk about the lower section here, which is how we do a product or a supply chain level comparison. And some of the same ideas still apply, that there might be reasons to do this both internally and externally.
Internally, right, trying to understand where in the supply chain your environmental impact is. What are the things you might have control over that you might be willing to-- that you might be looking to change. Exploring, especially differences in the design or changes that you might make to reduce those impacts. But also it has an external components as well, namely around things like product labeling or making environmental claims about your product. Trying to convince consumers or your customers that your product is better than ones that are on the market. And again, there's going to be some slight differences that go on between what you're trying to do and how you might go about it.
So with that, we'll start off talking about this hand dryer study, and then go through the bits and pieces of it individually. This really is getting into-- it's a pretty good example of something that gets at the fundamental questions of how you compare different products and different things. In this case, we don't really care much about who owns which piece of each of these products. We're looking at the product as a whole from beginning to end and trying to come up with a way of comparing them. This is the result from some of the material that came out of the publication of this study. But it requires a different type of analysis and sort of a different level of work.
Has anybody in here done a life cycle assessment before? What kind of product, or what did you look at?
AUDIENCE: I used to work at a company that made seafood products, and we looked at three of our SKUs that we made for Walmart. They asked us to specifically do a life cycle assessment on three different products of theirs.
PROFESSOR: Just to understand what the--
AUDIENCE: Yeah, not of a larger, they were working with us and numerous other companies, but they were trying to get a sense of what the larger impact of individual items that they carried was in different departments over the course of the weeks.
PROFESSOR: Did you actually do the-- were you responsible for sort of--
AUDIENCE: I did a large portion of the data collection for the stuff we were most directly responsible for. It was kind of broken into chunks, so a major part was packaging, then there was the food that went in the packaging. And so I was working with all the different groups who knew the most about those respective portions to then draw the data from them and put it together cohesively.
PROFESSOR: How about anybody else? Anybody read previous studies like this before, or seen anything like this before?
AUDIENCE: I actually read this one for when we were working on the [? S-Lab ?] project. And I really wanted some guidance on the choice of materials. So they said LCA, but when we got down to it, it was actually just the materials, so it was not the whole assessment.
PROFESSOR: What were some of your impressions about it after reading through this?
AUDIENCE: I thought it was strange of them in the beginning to say that they were going to do a full life cycle assessment including transportation costs, and then two sentences later they said they were going to ignore the entire supply chain and just focus on doing an apples to apples comparison of the performance. And they sort of black box all of the dryers into the same sort of process in China. And I thought we weren't necessarily getting who, maybe, was the real champion between Dyson and Excel. Because if perhaps one of them happens to have manufacturing in the United States, then they're getting a large transportation cost lumped into the cycle that maybe doesn't exist.
So I don't really know why they decided to go that route. It seems like they went through all the machinations for the paper towels, so it wouldn't have been maybe that difficult to get it for the other companies as well, so that they actually did the real full life cycle. Because otherwise, it seems like they should have collapsed the scope if they only wanted to do performance, and leave the upstream part of the supply chain out of it.
PROFESSOR: So right, they didn't really ignore the supply chain, they more just standardized it, in a sense. Because they did include all of those things that go on, but they didn't model the exact supply chain that each specific product might have taken. So what was the reason for that? They mentioned why they did it in there.
AUDIENCE: They said they wanted to put them all on equal footing as part of the supply chain, but it seems like not putting them on equal footing is sort of the purpose of the life cycle, to see who actually has the better process.
AUDIENCE: It actually didn't matter. They had a figure that explained if all these different devices were manufactured in Malaysia, US, or China, there's very little difference across them.
PROFESSOR: Right. We'll talk about that, because as we read through there, there's a lot of assumptions that they had to make going on. So what might have been one of the reasons they had to make that assumption about the supply chain, about taking this.
AUDIENCE: They just might not have had the data available and they determined that they could spend a year trying to find the data, but in the end, it wouldn't have made a difference and they wanted to go through the study and finish it anyways.
PROFESSOR: Right. In essence, that if you didn't have all the information for all of the products, then you really had two choices which was to perhaps go spend the time trying to identify everything, the exact path that all of those products took in their supply chain. The other would be to try to ignore that piece and put them on a sort of standardized setting to do the comparison with, and then come back later and test some of those assumptions. And that was sort of the approach that they took with this one. What were some of the other areas that stood out?
AUDIENCE: The sensitivity analyses were the majority of the paper.
PROFESSOR: The paper itself was like 25 pages, and then there was like 80 pages of sensitivity analysis and whatnot at the end.
AUDIENCE: Yeah, and there were a lot of assumptions in understanding which of the assumptions had more risk. What I was really struck by, too, was the fact that so much of the data was coming from either the companies themselves or from other studies. And there was a point at the end of the paper that said they didn't have access to the writers, authors, or practitioners who prepared those studies. So I think that the gap, they made assumptions made on gaps of information, but even the information that they do have I called into question. I [? didn't believe ?] the accuracy of it.
PROFESSOR: So access to data was something that came up a number of times. You saw there are a number of previous studies that have been done, and you kept probably noticing the references throughout the document of every time that there's some assumption or some play made. And most of them were being traced back to previous studies that had been done before.
AUDIENCE: Even if you had access to data, the thing also is that data changes and you get updates, and you get more data. So I thought that the LSA was true at some point, but it's also made very quickly.
PROFESSOR: I'm going to say it's static. It's a snapshot of a point in time of a specific configuration of the supply chain analysis.
AUDIENCE: My frustration with it was the single most important variable when it's how long people take to dry their hands or how many paper towels they use, and they had no real data on that. It was just completely made up, because you can't really get that kind of data, because you can't put cameras in a bathroom, and you can't put someone in there without affecting their behaviors.
PROFESSOR: The joke that they had-- the study authors were here at MIT in the material systems labs-- is that they thought about going out to the airport and just sort of standing in the bathroom with a notepad tracking people, but figured that might get them thrown out by security pretty quickly. In some sense, this comes back to the idea of access to data. You're making these assumptions because you lack the data that, if you had gone through and done some of the studies and things like that-- actually observed people-- you might understand how exactly it is, what is the average hand drying time, things like that.
AUDIENCE: They talked about the lifetime of the dryer, which they had as five years, and thought that seemed kind of low. It seems like five years would be pretty frequent for a building manager to change out dryers. Is that time until it breaks or is that the time until they recommended [INAUDIBLE].
PROFESSOR: Anybody know why they chose five years?
AUDIENCE: Warranty.
PROFESSOR: That's what the warranty is.
AUDIENCE: But how many people--
PROFESSOR: Throw it out if it's working fine. I'm going to Juan, this goes back to your first point about what they chose. The configuration of the supply chain was important, right. And this goes back to, if we're taking a static snapshot of something that all the different permutations and all the different combinations might be difficult to do, so you have to start making some judgments about the configuration of the supply chain. And so they did that, in this case, by assuming they all came from China. Except for the towels, which came from the US, because that's sort of the industry standard.
That's the second one, these are related to each other. As you start digging into it, you start running into all these areas that you could do things differently, and there's sort of all these different variables that go into it. At some point, you have to start making some assumptions and some limitations to try to do better on it. Did any of you think any of these-- I mean, they did some sensitivity analysis, but which ones do you think would significantly have affected the outcome? Or were there any?
AUDIENCE: [INAUDIBLE] that usage data, too, was just a huge factor, because if you looked at the outputs. The overall ranking of the product was so largely driven by what was said, was estimated to be your usage. That was one of the least effectively tracked, or easily quantifiable metrics. So to me, that was just sort of [INAUDIBLE]
AUDIENCE: Going along those lines, I think that it was the number of papers, or the mass of the papers that is used for one usage. So that had a significant impact on how big of a GWP for the paper towels have compared to all the others. So that was a big assumption, too. They made some big assumptions on the hand dryers, but also on the paper towels.
PROFESSOR: And in some sense, this gets back to-- Different bathrooms have different types of paper towels, and so identifying one paper towel that you're going to choose to be representative of can be quite difficult to do.
AUDIENCE: I [? think also ?] throwing out the electric grid assumptions [INAUDIBLE] in use, and they had some pretty big impacts on the manufacturing for the paper towels. So the paper towels, if they weren't made in the United States and they weren't made on the average, that could have a huge impact. Likewise the usage, depending on where it was being used, [INAUDIBLE] would have a big impact on the electric dryers.
PROFESSOR: So if you were Xlerator which came in probably as the most similar, in terms of products that it would be competing with. I don't know if you've used the Xlerator dryer before, but just really powerful heated air coming out. A little bit different setup. But it generally, and the results showed us the second best after the Dyson one, but probably their direct competitor. So what would your response be if you were Xlerator and you saw Dyson sort of promoting this study?
AUDIENCE: [? I think I'd say ?] Xlerator was made in the US and Dyson was made in Malaysia, so you could point out that Dyson doesn't have to be-- Dyson using the [INAUDIBLE] [INAUDIBLE] the US electric grid for [? manual ?] [INAUDIBLE] and also have to ship the products across the ocean.
PROFESSOR: That's a big part of the marketing, they need to have a made in the US certified, whatever that means, on their webpage.
AUDIENCE: Also for Xlerator, the measured versus reported drying times were really different. For Dyson they were exactly the same, it was almost double for Xlerator. So they may want to get control of how much time they think people are spending and maybe make their machines a little bit more efficient. Because if they were to go to 12 seconds, which is lower, the difference between Dyson and Xlerator wasn't that big. But when you went with the 20-- I think it was 20 seconds-- that's where the difference was more severe.
PROFESSOR: So where did that measured drying time come from?
AUDIENCE: Because they looked for the measure, they were following the NSF standards of 0.1 gram of water on the hands when you dry it on a towel. But in [INAUDIBLE] I mean, it's really perceptions. The user doesn't actually measure 0.1 grams of water on my hand, you'd better stop using the dryer. It's really a perception issue.
PROFESSOR: We had that NSF protocol, all right. This is not National Science Foundation, it's National Standards or International Standards Institute, something like that. They have standards for a lot of things, and in this case, it's how do you define dry hands. So it's whatever it was, 0.01 grams of moisture per whatever is how they defined it. So the idea would be that if you were trying to objectively make some claim about how long it takes to dry the hands, that might be one way to do it. Who actually did the testing for that, though?
AUDIENCE: It was some study at University of Florida. I don't really know how they came up with those numbers.
PROFESSOR: Yes. I think, actually, Dyson reported it. So it was coming from a document that Dyson had claiming that somebody had done this test and come up with it. So you see, we're starting to get this paper trail going back and figuring out that so much of the study was dependent on this value, and that the person reporting these values is essentially Dyson, who's making the claim that it came from. Even though we have sort of a standard that makes sense in terms of, OK, we're going to try to find some way to do this objectively. It still would definitely leave some room open for Xlerator to probably take some issues with it.
AUDIENCE: If Xlerator was really concerned about the results of the study, too-- and something I've never noticed using one of the Airblades, they said that it has a digital motor, so it has no spin down time, so they didn't credit the Dyson with any 1.5 seconds spin down. Whereas the Xlerator was doing it out 3 over 750 watts, and assume 750 watts, which adds up over the 350,000 uses. So I guess if they wanted to borrow that idea and get rid of some energy consumption, they could do that.
PROFESSOR: So if you were Xlerator you'd seen, you read this study, and wanted to respond to that, what might you go do differently?
AUDIENCE: One, you might commission your own study. Since this was commissioned by Dyson. That's a pretty big starting point. Dyson commissioned-- not to say that the researchers at MIT weren't as objective as possible-- but as we noted, there was a good deal of data that the study was dependent on that came from Dyson and Dyson then commissioned the study with it. So if I'm Xlerator, I'd probably like to have a say in that as well.
PROFESSOR: Right, so that was one of the other issues with the data access, right. Because Dyson was behind this, they supplied the bill of materials and they supplied all of the data about the factory and things like that, whereas all the other products, they had to make use of publicly available information. So they didn't have access to that inside information.
So if you were Xlerator and saw this, and disagreed with it, you could commission your own study. You could now make use of the fact that you know about your supply chain, and you could start actually going and doing the details. You could go through the fact that you're actually made in the US, and you might want to redo the study under that assumption. That you're going look at it with your specific supply chain, no longer considering, say, a generic's supply chain from China.
So what would you do to get around this? Because that is still sort of the crux of it, was that you both claim similar drying times, but they're saying that in practice, yours is actually worse.
AUDIENCE: I don't know, it just depends on [INAUDIBLE] here's what they did. Putting cameras or individuals in bathrooms, but potentially building in some sort of chip that enables you to see what actual times are for drying, and then that could work in your favor.
PROFESSOR: I might go out and actually redo this study that Dyson was reporting. If I use this NSF study, to go in and at least verify that it really does take my machine 30 seconds versus their 12 seconds or something like that. So at MIT, if you wanted to do study of figuring out how long people actually dried it, you'd have to go through the human subject ethics board and all of that. So you might have trouble actually getting a study through where you actually went out and tried to observe it.
But you could at least try to reduce some of that uncertainty that comes in the measurement, since it's unfavorable to you and not really much hard information out there about it. So you might want to commission, say, an outside source to come in and repeat that testing, and see if they really got it.
In terms of what they're actually doing-- so they actually have commissioned a study to respond, to follow up on this. Because nobody's ever going to be happy with all the assumptions that go on in one of these, especially when they turn out to be-- if you look at two of these big ones that are certainly unfavorable to Xlerator, probably. When you look at them it makes sense that maybe you would want to come back in and revisit this to try to make sure that the assumptions that your competitor is making that are unfavorable to you actually are grounded in reality, or don't necessarily make a difference in the final outcome.
But you probably don't want them releasing a study that says this is your product and it's worse for the environment, it takes longer to dry, et cetera, without at least being prepared with some sort of follow up to dispute that, other than to just ignore it or claim that there's problems with it. You'd like to be able to come back with some sort of response that's grounded in some data or have some facts for it.
We're going to walk through this a little bit more in depth in terms of looking at it within the scope of the methodology of life cycle assessment. But to start off with, just what is life cycle assessment. And that's a good question to ask, because it took some time for the industry itself to figure out what exactly it was. But there are a set of ISO standards that I think were produced in the 1997, the first version.
Essentially it says this. LCA is a technique for assessing environmental aspects and potential impacts with a product by first, compiling an inventory of the inputs and outputs. So tracking, what's coming in and out of the environment. Second, evaluating the potential impacts associated with the inputs and outputs. So figuring out, now I know what I'm emitting to the air, what I'm taking out of the environment, actually figuring out what those impacts are. And one of the key points here is that it specifically mentions the potential environmental impacts. Because some of these studies are going to be forward looking. Trying to figure out what happens when we make a change as opposed to some of these studies that are looking backwards and trying to figure out what the actual impact is.
So that's a big one, is that a lot of times, we don't necessarily know what the exact environmental impact is. We're trying to forecast ahead of time what it's going to be. And finally, interpreting the results in the inventory analysis and impact assessment in relation to the objectives of the study. So the big thing about this is that it's sort of fluid, in terms of what an LCA is. Because you start off with creating some kind of objective of what you want to do with the study, and all of the results that you generate are going to be interpreted back in terms of that scope and that goal that you started with.
Some of the important things to remember is that it takes a life cycle view of the product, so raw materials all the way through to end of life. So to cradle to grave is typically the way that would be talked about. And while it specifically mentions products, it can be mentioned for services as well. So we can think of the hand drying example. It's not necessarily comparing products, it's more comparing a service, that of drying hands.
LCA generally credited to Coca Cola as being the first person to develop this back in 1969, when they commissioned a study that would look at the environmental impact of using cans or glass bottles. So this is when they were first thinking about going to cans, and they were trying to understand not just all the economic and supply chain impact, but they wanted to consider the environmental impacts as well.
They commissioned a study and actually hired some researchers to do it, and essentially they came up with what would eventually become life cycle assessment, in terms of quantifying that environmental impact in some manner by looking at the inputs and outputs. So that was in 1969. It started to gain attention in the '70s. There were other efforts going on in this area, especially in Europe with the energy crisis. There was a lot more concern about things like how much energy products were using, services, about what it was going to mean.
As that started to fade away-- the energy crisis-- in the '80s, it became a little bit more known for companies making environmental claims about their products, so trying to use it in advertising and things like that. It gave rise to what they referred to as hired gun studies. Which is basically, as we saw, there's a lot of assumptions and a lot of questionable assumptions, at times, that go into doing one of these studies. And what they were finding is that there are companies out there that you would hire them and they would construct a study in such a way that it said basically whatever you wanted, reaching the point that 11 state attorney generals issued guidelines saying that you couldn't use life cycle assessment to make a claim or a comparative claim about the environmental impact of your product in an advertisement, until LCA became standardized. Until a uniform methodology was developed.
So that sort of kicked it into gear of getting different companies, different organizations together to try to iron out what exactly a life cycle assessment was going to be. SETAC was probably the leading group, and then it's something like the something environmental toxicity and chemistry, society for environmental toxicity in chemistry, I think. Something like that. So it's generally chemists, people that study ecotoxicology, things like that, getting together and creating working groups, generally of academics and practitioners, trying to come up with a uniform methodology that can be used for life cycle assessment.
And eventually this developed into some standards from ISO. 14040 is the main one, but there are about four or five different offshoots from that that cover different aspects of it, from the guidelines for how you do it, to how you would use it, to making claims, or how you would use it for putting labels on products. So it's sort of grown quite a bit from those beginnings.
So essentially, the methodology that they developed is going to consist of four stages. The first of which is the goal and scope definition. Which is comprised of a couple of things, namely deciding what it is you're analyzing, looking at what the scope of the system is under consideration, identifying what falls within your system boundary.
The second step is referred to as the inventory analysis. This is where you're identifying and quantifying all the things that come in, raw materials that are coming in from the environment, all the emissions that are going back out, whether it's to air or water or land. Other products that you might be using, all of the inputs. And essentially creating an inventory of those, identifying how much there are.
The third step is the impact analysis. This is where we go from the inventory, from identifying everything that's going in, to figuring out what exactly the environmental impact from that is going to be, and what methodology is used to do that. And then finally, as we talked about, interpretation is the fourth step. So we want to go back and, after we've set the goal of the study-- identified the scope and gone through and quantified the environmental impact-- how do we take these results and relate them back all the things we set out from the beginning that were our goals. So if we start off with the goal and scope definition, right, the first thing is to decide the product you're going to be studying and what the purpose of the study is.
And that includes three points. What is the intended application of the study, so what are you trying to do with it. What's your reason for carrying it out. And third, for whom are the results going to be used. So if we look at the study that we looked at today, you'll notice when the study is laid out, as you read it, it was actually laid out in sections that followed along from our four step.
Let's talk about the product to be studied and the purpose of the study. What did they claim were the reasons for doing this? First off, what was the product that was being studied?
AUDIENCE: A dry set of hands.
PROFESSOR: So it's a Dyson Airblade and it's not a paper towel. What they set up was a per pair of dried hands. So this is what we would refer to as the functional unit. This is actually what we're going to study, and everything that's done throughout the study is in terms of this. So this is going to be our unit of analysis, it's going to be how we're going to compare all of these different options. And what did they say the intended application of the study was? What were they trying to do?
AUDIENCE: [INAUDIBLE] dry hands [INAUDIBLE]
PROFESSOR: So they actually had two uses. What was the target audience for this?
AUDIENCE: [? The engineer. ?]
PROFESSOR: Right. So if we look at in terms of this, one group of people that the results were for were the Dyson engineers. They wanted to go through and understand where were the impact-- what was the reason, it was to identify the impacts associated with the Airblade so that the engineers could take a look at that and start figuring out ways to reduce it. What was the other purpose?
AUDIENCE: [INAUDIBLE] environmental impacts.
PROFESSOR: Related to that. So if all I wanted to do was identify for my engineers where the impacts of the Airblade is, would I need to consider paper towels and the Xlerator and things like that? No. So that's our second reason, was comparative. They wanted to go through and say here's the environmental impact associated with a dry pair of hands when you use the Airblade, and here it is when you associated it with one of the other products under consideration. So they actually had the Airblade. What else did they study? The Xlerator.
AUDIENCE: Recycled paper towels.
PROFESSOR: Yeah, so they had two versions of the paper towel. And they had two versions of the Airblade, too. The aluminum and the plastic.
AUDIENCE: Standard, right?
PROFESSOR: Yeah, standard. Cotton Roll towels. So this gets back to our choice of a functional unit was the dry pair of hands, so this gives us the basis that we're going to do these comparisons on. But in terms of the goal and scope, that's important, because if I just wanted to use something internally, then I would never go to the trouble. There's no need to go to all of the trouble to do all of this.
The big thing that drove a lot of this is the idea that it's going to be comparative. That they actually want to make a comparison between what happens when you use the Airblade between when you use some of these other systems. And it's going to come back because once they did this, then they had to start making a lot of assumptions about how these other systems work, since they didn't necessarily have the data to that.
And then because you're doing that comparison, you sort of-- part of it is they're going to make this available externally. They've said that part of it might be to use this for making claims. And so once you've decided that you're going to start using it for public claims, you definitely want to be a little bit more rigorous in your application and your approach. Because as soon as you publish this and start making claims that X and all of the other companies are going to look at this and think, well, why didn't you do this, why this.
So this sort of drives a lot of what goes on in the next few steps. That by doing this, now we've expanded the scope of our study from just looking at my supply chain, which I have pretty good visibility to, to starting to look at a lot of different other products. And this brings a lot of extra complexity to it, because I have a number of different studies. And if each of these has different configurations or different assumptions that I make, the complexity starts really blowing up.
System boundary. What did they choose for the system boundary?
AUDIENCE: They chose not to include things like maintenance or transportation [INAUDIBLE] So they had to make a lot of assumptions [? around ?] the [INAUDIBLE]
PROFESSOR: So they start off by saying this is a life cycle assessment. So we're going to do cradle to grave. And you can think about that, that idea that you're doing cradle to grave creates a lot of problems, because they mentioned there's how many different screws in the Airblade? And you think that now you need to trace each of these little parts all the way up through their supply chain. So they started making some definitions about where they were going to sort of consider things outside of the system boundary. So there are a couple of issues that go into that.
So one was cut off rules. So what did they use as a cut off rule?
AUDIENCE: 1%, if it's less than 1% in effect then they didn't include it.
PROFESSOR: Right, and so this creates-- what's the issue here. If it had less than 1% of the total impact, they would exclude it. What's the issue with using this as a rule?
AUDIENCE: Number of [INAUDIBLE]?
PROFESSOR: That's part of it. How do you know when something only contributes 1% of the total impact? It's like a chicken and the egg problem. If you've quantified to identify that it's less than 1% of the impact, why wouldn't you include it. What they have actually done is for all those little things, they've done some basically back of the envelope or some other type of high level estimate to try to figure out what they are, without going to the trouble of digging down deep into the level of it to try to identify what it was.
They mentioned some of the other, right? They were using a lot of information from other previous studies, they mentioned some of the other rules that some of the other studies had used. Remember what some of those were?
AUDIENCE: Also on that, didn't they limit it to no more than like 5% of the total product on this 1% assumption so they couldn't say every screw didn't matter.
PROFESSOR: Right. So we do any individual process that was less than 1%, and in total, everything that I excluded had to be less than 5% of the total. These are pretty common rules. So you can think about this is if you had a bunch of things that were less than 1%, but then you estimated the total impact, they were only covering 90%. So you would just start adding more and more of those back into the system boundary until you hit that 95% percent point.
Some of the other studies used a slightly different rule than the impact. So some of them did this based on physical quantities. So anything that was less than 1% of the mass, I think one of the studies, was excluded.
This tends to be easier, because you don't actually have to go to the trouble of estimating what the impact is. You can start saying, well, I'm just going to exclude all of the tiny little things that go. But you're making an assumption that those don't contribute big, right. So if it's got-- I don't know, some uranium in it or something else that's radioactive, that may have a very high impact in terms of certain categories even if they don't contribute much the overall weight.
So generally, this would be preferred. We would like to make a ballpark estimate of what the impact is and then go through and exclude based on that rather than basically just using one of these physical quantities. There were some other things that they cut out from the system boundary.
AUDIENCE: Something about excluding raw materials [INAUDIBLE]
PROFESSOR: So I think they included that, or they should have, at least. I think it was mentioned earlier. We had capital goods, were not included. This is also a fairly common exclusion. Things that have a multiple year lifetime, not to include those. You could include in the same way that, when they made the decision to do a dry pair of hands, they had to start making assumptions about the lifetime of the product, how many uses it was going to get made. And then they allocated a portion of that to the life cycle. They included things like the bins and the liners they were using for paper towels and things like that.
You could include capital goods. You'd have to start making assumptions about how many years it existed, how many products it read. If you were to include the trucks or the ships that move the goods, how many trips a year does it make, how many containers does it move. You could start doing that. They tend to be pretty low. If you're including the transportation piece of the ocean, so you're capturing all of the fuel that's being burned, but you're just not capturing what goes into building the ship. So that's another one that's a pretty common one.
So they mentioned in the study that some of the studies they used had excluded the capital goods, and so they chose to exclude capital goods in this as well in order to maintain that consistency. So in this case, they might have otherwise chosen to include those. But because they were relying on past data, they went ahead and included them anyway. What about with the paper towels? This was specifically one of the issues that they had.
AUDIENCE: Whether or not to include the environmental impact of previous recycling.
PROFESSOR: Right, so this is another big one. Specifically, what was it about this type of recycling that was difficult? Remember what the term they used for it was? Open loop. So this is the problem with things like paper, is that something that is a paper towel, if you were to collect it and recycle it, usually the strength of the paper you get from the recycled fiber is not as strong as it was when it started. So if it started off as a paper towel when you recycled it, it's usually no longer strong enough to be a paper towel. So it might get made into toilet paper, or newsprint, or whatever else is on down the line.
You get into this situation where you have the product, and you recycle it, and then it's going to go get turned into some other product that has its own life cycle, and it's going through. As opposed to a closed loop recycling, that product would just get turned right back into an input. And if I were to do that, then I can draw my system boundary around this, and I don't have to worry about it, because it will get included in this system boundary.
But when I'm doing this, I start getting into multiple different types of products. This is a problem because the input-- when it mentioned they had the two types of paper towels. Some were made from virgin fibers and some were made from recycled fibers. If it's made from a recycled fiber, it had its whole life cycle before it became a paper towel. When it was maybe copy paper, or something similar to that.
That usually means you're going to have to make some decision about where to draw the line, in terms of how far we go chasing all of these other products that it might have been turned into. So, again, the goal-- this comes directly from what they wrote in the report. Compare the environmental impact of hand drying systems. Evaluate how the impact under different manufacturing and use scenarios.
So this is part of it, is that they, rather than consider it within the context of just one scenario, they want to try a couple of those scenarios. This related back to the assumptions that they had to make. Two, identify impact drivers and ways to target those with the idea to inform product decisions. We mentioned this comparative assertion, so this is sort of the important one, because that lets us know this is going to go to an external audience. This brings its own set of requirements.
So if you're going to use something for comparative assertions, the ISO standards generally require you to have a third party evaluation of it. So if you look through in one of the appendices, they mentioned the results of their third party, essentially, review of it.
And so they had a panel of three experts go through and review the initial draft of the life cycle assessment study, and then they had to go back, and usually you get critical-- it's like submitting something for peer review at an academic journal or something similar, where you would get back comments about what you might want to change and what you might want to do. So once you start making some of these selections upfront about the goal of your study, that's going to drive extra requirements and make some complications for what you need to do down the road.
Finally, they mentioned internal and external audiences. With the goal, the big thing to keep track of is, there's really two types of life cycle assessment studies and they go by different names. Attributional, first consequential is one of them. One I've heard is accounting based, that would be the attributional. And this would be change oriented. Really, these are little diagrams that somebody created that are useful to think of it. The attributional one, which is what they've done in this paper, is essentially taking a system and trying to figure out what portion of that system's environmental impact is related to whatever you're looking at. So it's taking a static snapshot of something and trying to attribute the various environmental impact to your product.
The consequential, the change oriented, is going to take a system and it's going to try to model what happens when you make changes to it. So the big thing of that is that it's not static. The picture's going to change. You might reduce impact in certain areas, but it might increase it in other areas. And so this attributional style is generally things that are backwards looking, whether it be estimating the environmental impact of one of your products. If you wanted to, say, do a carbon label, or to meet some certain standard to get an eco label. To estimate the environmental impacts of different systems as they are now, is the big thing.
The consequential is going to look at what happens when we make a change. So usually what this means is that could involve very big changes in the system. That if you make certain decisions, they're going to impact. That things might look this way now, if I look at just the system as it is, but say my demand is starting to increase and I start to have to start making different changes, that could shift the way it would be.
So one good example of this would be looking at, say, the impact of ethanol. If you look at US policy on ethanol. If you just look at a static snapshot of where we're at right now, or the way it exists right now. You'd look at the life cycle for, say, corn ethanol, and you measure its environmental impact. And then you compare that to, say, what it takes to produce gasoline or diesel fuel, and you come up with an environmental impact. And so that generally would tell you the ethanol is slightly better on, say, a greenhouse gas perspective.
But when you start looking at what happens when you start making big shifts, as we start converting crop land from other uses in order to turn it into corn, in order to turn that into ethanol, you start getting a cascade of change as they go on.
So in the case of the US, we've switched a lot of farmland over to corn, and so that has left a gap in wheat and soybeans and other crops that used to grow on land that were corn. So now we don't have as much to export to other countries. So those countries have to convert some of their land to uses to grow, to make up for our wheat shortage. And so then they may end up clearing rain forest or some other sort of bad impact, in order to turn that into land to grow these crops on.
So once you start looking at this cascade of system effects, people think that ethanol is no longer preferable. That once you include the indirect effects, it's better. So in that case, that's what happened when you start looking forward. Looking at what happens when I start to make a change to this. And so if you look at the characteristics of these different types of systems, they're going to have differences in what you're going to do.
Part of that is the choice of data, right. These we tend to based on average data, because we look at the average electricity grid in the US. You're usually not making, and we're not looking at, something that creates enough change in the system to really worry about whether it's impacting things at the margins. But once you start looking at energy policy or some of these big areas, if you start looking at, what if we were to shift a significant portion of steel production to aluminum production, which might be much more electricity intensive. Then we start needing more power plants. Maybe those new power plants come online, are gas powered as opposed to coal fired, and so the actual electricity and the actual emissions impact is less than if you looked at the average of the grid.
So these are the ideas between what type of study are you looking at. It's going to drive some of these choices and where you get the data, what the system boundary is. One of the nice things about doing a change oriented LCA is that if I have things that didn't change-- I'm comparing two systems, a before and after, and I have pieces of it that didn't change at all, I can ignore those pieces. Because they're not going to affect. I'm just looking at the changes that happened, so I can sort of exclude all of those from the system boundary. Whereas with this type of assessment, where I have to trace everything all the way up and back, I have to make sure that it's fully complete to make sure that I'm really getting that share of the emissions.
AUDIENCE: You mentioned the power grids. Is there, to your knowledge, any effort to track specifically which type of power is being used at the point in question? For example, if something's made in Pennsylvania it's probably coal, but if it's made in Idaho, it's almost exclusively hydraulic. Anyone ever account for that?
PROFESSOR: Yeah. So you can definitely get at that level. Even in things like the greenhouse gas protocol when we were talking about corporate level ones. If you go and look at the tools that they have for measuring the impact of electricity, you can get regional specific factors.
One thing to remember with electricity, it's on the grid. So even though you may locally be supplied by a nuclear or coal plant, you're part of, usually, a regional grid. So they tend to break it out into inter-regional ones. But you can get further down emissions factors and start looking.
We did a study with Chiquita looking at bananas. And so for that, we went-- part of that is that they're grown in Central and South America. So you can go out and look at the different electricity factors at a country level there, and they can make quite a bit of difference. There are, definitely. One, a lot of people of people already do that. Two is that you tend to be able to model that by looking at the percentage mix of coal versus nuclear versus gas that might be used in any specific area.
It's a really tricky question when you start thinking about what happens as I start to change things, because then you get into where's the power going to, which plants come online as demand starts to surge, and things like that. But that's definitely an area that people look at. Usually the big question is whether-- if you looked at, what if I did some small change where, say, I moved my corporate offices from one location to another. You probably wouldn't want to use the marginal data on electricity if you move from one region to the other, because you're not really large enough to have an effect on what's going on with the grid at that sort of level. So maybe it's better off to stick with averages and things like that. So there's some grey area in there in determining when it's right to use which factor.
We've covered this, the functional unit is a pair of dried hands. Once you've made that, it makes sense because as we saw, it's in the use where the impact comes from. So the question becomes, you don't really want to compare an Airblade versus an Xlerator. You want to compare what happens over the use. And definitely, when you start including things that are not a one to one correspondence-- comparing paper towels versus an Airblade, you want to put them on a unit of analysis that makes sense.
But once you start doing that, you start getting other-- right. So that's one of the things you decide up front, and then that creates a cascade of decisions about what you're going to have to do later on. Amongst that, making assumptions about the length. How many hands get dried, the lifespan of it. All the ancillary and secondary products to go along with it. So it's the kind of thing you want to decide upfront, because it creates consequences for what you do down the line. And that's why it's usually one of the first things that you'll do in the study.
Scope, we had seven different systems. Cradle to grave. They mention this within there, that they are including packaging. So the cardboard that they come in, or the plastic wrap when it's there, and the bins and liners and dispensers. So you want to be clear when you're setting the scope about what things you're including and what not. So they include the packaging, but they don't include the pallets that they get shipped on, things like that. Capital goods, et cetera.
And with the system boundary. We covered some of the allocation, what it means. So you had the end of life we talked about. Co-products, if you have a manufacturing plant or some other facility that's producing a number of different goods, you usually are going to run into that same allocation problem where you're going to have to figure out some way of apportioning the impacts of the production process to the different products that come out.
And again, there's various rules for that. Usually they're going to be simple things like mass, energy, or economic impact. One example in the textbook I have is a chicken processing plant. You're taking in chickens and you're producing breasts, thighs, wings, all of these different products. You can divide up the impact of what it takes to produce a chicken, to raise it, to give it feed, all of that. But at the end, you have all of these different types of products that all come from that same input, and then figure out how to divide those impacts amongst the different products that are coming out.
So mass or energy would be one way to do it. I just weigh the different products and I'll assign them each share of that burden based on their weight. But again, this may work well for some things, but not for others. Especially when you have some products that are coming out that are very high value. Since those tend to drive the decisions that you make.
So maybe you want to do it on the economic basis instead, which is to say, I'm going to portion my impacts based on the share of the value that each of these items generate. And these tend to come back down to accounting. So in the same way that our corporate carbon footprint last week followed our financial accounting rules. This is much more in the vein of managerial accounting, where I'm trying to make certain decisions that reflect what's actually going on as opposed to just necessarily following some rules.
Cut off rules we covered. One thing with the end of life-- so this was back in the appendix of the document. But this is essentially illustrating the four different cut off rules that they could apply for the waste management of the paper towels. So the base scenario used, essentially, this cut off rule. So what they're saying is that you have the first product that takes the virgin material in, you produce it, you use it, then it goes back to re-pulping. So it's essentially recycled and turned into a second product. Again, has its own life cycle. You might repulp it a third time, and again, it has its own life cycle. And then maybe it's reached the end of its useful life and it's going to go to waste management.
So all of these are essentially the same supply chain, but we can divide up the impacts between the three different product life cycles based on how we divide up who gets credit for which of these burdens. I think they used the cut off rule in this one, which is the first product took credit or took the impact for all of the production of the virgin material and all of the use. But then once it moved off into the recycling system, all of that impact was associated with the products that went on down the line.
The second product took care of all that repulping, the burden that went on there. And the third product took that second set of repulping and the final waste management disposal. So if you think of this as comes from the original forestry and paper production, and this is the final when it gets thrown away in the landfill. It's deciding who got those various impacts. And you can see that they've drawn, depending on where you want to draw the impact, there's a lot of different rules you can apply. And it's mainly a judgment call on which one you think makes sense. Since it's not entirely clear that one is better than the other, these decisions are, in essence, always going to be arbitrary. OK.
So that was the first step, the goal and scope. The second step is the inventory analysis. So this is when you have to go out and identify and quantify all of those impacts from the production system. So in this case, it's a pretty straightforward process, but not easy. So simple, but not easy. So the first is you build a model of the system. This is sort of their stylized version of what the model of the system is. If you look at a more detailed life cycle assessment study that's really getting down into the production process, you might see a list of, say, hundreds of different processes drawn out in a flow diagram.
A lot of the reason for this is that it's coming from people from a science background that have done this. So it tends to follow a mass or energy flow, where every time something comes in, you have to trace it and figure out where it exits the system or where it's going into, with the idea being that nothing should be getting lost along the way. If I have 100 kilograms of material coming in as input and 86 kilograms of it go into the production system, then that other 14 kilograms better get traced where it's going. Whether it's getting turned off into waste or burned or whatever happens to it.
So that's generally the first part. And this building a model of the system is something that goes on by looking at who are my suppliers, what are my inputs, where do I get different things from, where's energy coming into the system, electricity, all of that. Where are things being transported between, where are my different facilities located, interviews, studying the bill of materials, looking at your procurement, analyzing where your customers are. This tends to be going through the records of trying to figure out and map out the supply chain. So from that standpoint, it's pretty simple understanding what's going on, not necessarily always easy to do since this tends to be very data- and labor-intensive to go through and figure this out.
The even worse part is that once you've identified all these processes, is identifying the inputs and outputs. So in the inputs we have raw materials, energy, and other products that are coming in, and on the output, it's our emissions, to air, to water, and to waste. So everything that comes into the system and everything that goes out. I'm essentially drawing a boundary around my system which, inside it, may have a number of different processes going on. What I'm looking for is, once I've mapped out that process, I need to go through and identify where are all my inputs and outputs. If this is my system boundary, these should all be raw materials coming in, energy coming in, and all of the emissions going out.
And so one way to do that is to go through step by step. This is a production facility, start tracking. What energy are they using, how many units did they produce, things like that. So this tends to be the time consuming part of it, but pretty straightforward in terms of what you need to do.
So in terms of the study that we looked at, how did they go about doing this? They talk a little bit about it. It's kind of vague in some areas, but they talk about the main process that they use. What do they start with for the Dyson system.
AUDIENCE: Bill of materials?
PROFESSOR: Yeah, so the bill of materials. Are you familiar with the bill of materials? Or the B-O-M, the BOM. This is basically just a listing of all the parts that are going into it. If you were to look at a higher level, you might look at it as the fan, the motor, the housing, et cetera. These are the different parts that go into it. And these in themselves may have a number of other different materials that go into them. So if you look at it, it's sort of a diagram that sort of spreads out, where you have one piece here, and it might have a couple of inputs. And those might, in turn, have their own inputs. And essentially, it's going to expand out. I'm going to have a big list of all of the materials that I have.
So in this case, they went through and took the data that Dyson gave them and said, we have the bill of materials for all of this. And the trick was to turn that into the life cycle inventory. And so what they did is they took the bill of materials data-- and they call it the bill of activities because they also have data about the activities that are going on, the energy use that's going on in the production phase-- and they matched it up with inventory data.
In this case, you can think of the work that would go on to actually go and do one of these. Especially because you think of-- they mention in there something like the optic housing that was on one of them that detects when the hand goes in and when it comes out. You think, that has a lens, it has a microprocessor, it has whatever else goes into it. And all of those products have their own complex supply chains.
So the minute that we mention one of these, if we're taking this cradle to grave approach, then we need to start tracking each of those products up all the way through their suppliers. And so they're buying that assembly from some supplier who in turn is buying a processor from this company, and they're buying a lens from this company, and they're buying a plastic housing from this company. And that company does some kind of injection molding, and they buy raw plastic from here. So you can imagine they're going to have to start tracing all of that up, what a labor that would be to try to do that for a complex product, or even a relatively simple product.
So what they've done is they've gone out and found this inventory data, which generally comes from third party sources. So if you look at how you would do one of these life cycle inventories, usually there's a couple of big software vendors that make tools that help you do this. SimaPro, GaBi, are some of the big ones. They, in turn, license databases from other companies.
ecoinvent was the one that was used in this study, and they mention that in here. So ecoinvent is a company that goes out and actually tries to do that work. They try to go out and look for common products and go out and examine what types of inputs do they have, what goes on at the factory, how much energy does it take to make it, what are all the inputs and outputs to the environment, and they sort of start building up these.
So then they have a database that tells you things like this type of steel has this sort of impact, here are its inputs and outputs, different types of plastic, et cetera. And you go through and you match those up. So now it says, I don't necessarily need to go through and do all of this tracing of everything for me. The software, the database that I'm using, is going to have some of that in there. It's enough for me to say that this particular pieces is, say, 2.3 pounds of this type of material. I'm going to go out and match that up with the database to do all of that upstream tracking and tracing of what's going on.
They talk about this in the study, though, because this is going to run into some problems. What are some of the issues that they had with this? So there's a lot of different products that go into it. What do you do when you run into a product that's not in the database.
AUDIENCE: [INAUDIBLE] they didn't have specific data on the different material covers for the hand dryers, so they just averaged them.
PROFESSOR: Yeah. In that case, that was a slightly different issue, but related. Because that one, they were relying on-- they were taking the information from a third party that had done the study already, and had just already averaged the impact of the housing together. Rather than not being able to find-- I suppose it probably fits in there. Nobody had done this study and said, here is the impact for a Xlerator housing made of plastic or made of aluminum. So it is probably the same.
Some of the stuff, like there was no galvanized steel. They said there was none of this. There was no-- I forget which type. There was some type of bleached paper that they didn't have. And this was related to the recycled paper towels and some of these issues. So essentially, they had a list of maybe 20 different materials or so that were listed in these bill of materials or that were included in the system that they didn't have data for. So they had to go through and sort of create their own version of some of these.
So they said well, one thing you can do is use proxy data. So they said, we don't have this specific type of bleached paper, so we'll just use as a substitute this type of bleached paper. Or they said, we don't have galvanized steel, but we have regular, this type of steel, and then we're going to add to that the impact that would go with this process of applying the coating to it.
Even with this inventory data, you're going to run into trouble finding all the products that you need to do to do this. And so it's generally not something-- you're going to have to make some compromises as you go, in terms of figuring out what you can include and how to get around some of these limitations that you might have. Now, once they did that, they went through. They had this chart in here which was showing, essentially, what they had. This is the assumption that they used to generate the inventory, because part of it was that they had to go through the manufacturing and identify how much energy per unit. And then they had to go through, and then based on this, the life cycle, how many times would it draw. They had to go through and capture how much material was going into each of these.
One of the assumptions they had to make was data about the scrappage rates. Because part of this is that the goal is to understand all of those inputs that come into the system and the emissions, and then what is my useful output. So if I take in 100 kilograms of plastic, but only 85 kilograms of plastic end up in the final unit, because the other 15 goes to waste, you have to make sure to allocate the emissions related to that 15 kilograms of waste to those final products. So it's not enough just to look at the end bill of materials. They had to make some assumptions about what goes on the manufacturing process and how that relates to it.
And so at the end, once they've gone through this, they have enough to move on to the next stage. I'm going to skip this piece here and talk about our next stage, which is the impact analysis.
So this is where they go through and finally, now that they have, for each of those different products systems, they've made all of these assumptions, they've put together the impact. They're ready to go ahead and determine what the environmental impact of each of those products was. So there's essentially two steps that go on in the impact assessment, referred to as classification and characterization. So classification, the first one, essentially that inventory analysis you have is going to list things like all of the outputs, all of my emissions to air. How much carbon dioxide, how much carbon monoxide, all of these different types of gases.
But if I'm interested in the global warming potential, I really only need to know which of these are the greenhouse gases. So I'm going to go through in the classification step, and I'm going to look at all of these different outputs that I have to the atmosphere, and I'm going to gather together the ones that are contributing to global warming. That would be the first step. That would be the classification step.
And you're going to need to do that for each of the different environmental impact categories that you might have. So they'll go through and do that. And the second one is the characterization. So if we look at it in terms of the global warming potential, after I've classified and gotten together my listing of, OK, how much carbon dioxide, how much methane, how much nitrous oxide, characterization is when we apply those global warming potentials and actually turn that into a unit of output. Carbon dioxide equivalence. And this applies to a wide range of different environmental impacts that you might study.
What were some of the impacts that they chose to study in this one? They had a couple of basic ones and then some more complex ones.
AUDIENCE: [INAUDIBLE] CO2 emissions. And they didn't really understand how to get water. But they said they included water, and then they said they didn't, so I don't really understand what went on there.
PROFESSOR: So they mentioned a couple things in there. They had the global warming potential, they have the carbon footprint, essentially. They had water use.
AUDIENCE: Land occupation?
PROFESSOR: So we'll get to that in a second. So they had, they called it CED. Cumulative Energy-- yeah. Then they had-- remember what the name of the more complex one was?
AUDIENCE: [? Along the lines of ?] IMPACT 2002+.
PROFESSOR: They had something called IMPACT 2002+, I think it was called. So what they did, when they went through this, they made a distinction about some of these. So the global warming potential isn't environmental, it's an impact category, because it basically does these two steps.
I gather my greenhouse gases and then I characterize the impact using their global warming potentials. The water use and cumulative energy demand aren't really impact categories. They're treated as such by a lot of people, but they made the point in the study, they're not technically impact areas. And the reason for that is that there's no actual characterization that goes on.
So for water use and cumulative energy demand, they just went back through the inventory and said during all these steps in the process, I'll just sum up how much water was used at each stage. At the end, I get a total amount of water use. But there's no attempt to turn that into something more complicated. There's no attempt to rate. You can think of if I'm using good, clean drinking water or if I'm using salt water from the ocean.
You might think that those would have different environmental impacts, because most people don't care if I'm using up the salt water as opposed to using up the clean water. So you could think they're not actually really investigating the impact of those, they're just calculating them so you can see how much water and how much energy they use. And specifically for the cumulative energy demand, they mention that it's a proxy for a lot of other things. The more energy intensive the production process is, that's tied to a lot of other environmental impact categories. So they're sort of used as a proxy, but not really an impact category.
So we had the single use indicators. Cumulative energy demand on the side, and global warming potential, right. And we can go through, and they've calculated the carbon footprint for each of these different products based on that inventory analysis and broken it up into the different stages of life. And you can do the same thing for the cumulative energy demand where, in this case, they're measuring it in how many kilojoules of energy they use.
Those are pretty simple. But the more complicated ones are this one like IMPACT 2002. In this case, that's because it has number of different factors going in, and it's sort of divided up into a two stage approach. Which is the first thing you have, just like with global warming potential, they started with the inventory results. Then they went through and created what they called a number of midpoint categories.
So if you look at what IMPACT 2002 is, it's divided up into all of these different things that are counting everything from carcinogens to ozone depletion to how much mineral extraction you're doing, aquatic acidification. So a number of different environmental impacts. This is getting at a lot more broader scope than what we would get if we just looked at energy or global warming potential.
It goes through sort of the same process that you had before which is, now I'm going to look at my inventory and I'm going to classify all of my emissions that are carcinogens, and I'm going to group together and figure out how much I have of each different type. They're going to go through this for each of these different midpoint categories. Then what they've done is that they've combined these, then, into a second set.
So they figured out how many of the different types of carcinogens, how many of these. And then they relating these again to overall indicators that capture a broad set. All of these are things that impact human health. So they're grouping these together and one they're calling human health, they're grouping all of these together into ecosystem quality, climate change has its own, and then they have resources.
So that in itself is a pretty complicated process, because if you read about it or looked into some of these charts, this is what happened is that they-- and I'm not even sure how you do this. So the carcinogens are being measured in-- I don't know what that is. I need to brush up on my chemistry. But it's some kind of grams of some chemical equivalent.
So they've taken all of the different outputs that they have that are carcinogens, and they've found a way to measure them in terms of some equivalent units. I assume this is producing the same amount of carcinogens as 0.277 grams of whatever that compound is.
That's how they've gone through, and for each of these categories, they've created some measure that they're going to use, some equivalent. So respiratory inorganics, we can assume that these have the same impact as it would if you produced this many grams of particulate matter. 2.5 millimeter particulate matter, right. Or whatever that is.
They've gone through and, for each of these categories, they've come up with a way to do this. And so this is pretty complex and probably nobody on earth is enough of an expert to be an expert on each of these categories. So what they've done is they've gathered together experts from all of these different areas to figure out how you would go through and judge each of these.
And they actually go a step further from this, actually, two steps further from this. So you can see for each of these products, we're going to get all of these different ratings. And the question is, how do we put all of these together in terms of an indicator. Because I can look at what the value is for an Airblade aluminum, and I compare that to Cotton Roll towels, and I see, well, Cotton Roll towels have less carcinogens.
Does that make them better than the Dyson Airblade. And the question is, that depends. Because if you start looking at some of these other impact categories, you find ones where, well, that actually contributes a higher value, 290 to 118 in terms of terrestrial ecotoxicity. So then you have to come back through and figure out a way to balance all of these different impacts together. And that's what that impact system does.
It essentially produces two things. So first, they group all of those together. They took all those human health impacts together and created a single indicator. So they're bringing together the carcinogens, the inorganic respiration, all of these different types of impacts that they said impacts human health, and they put them all on a single unit of measure. Which I believe is disability adjusted life years.
So if any of you are following health care, that's how you measure when should we pay for this type of health care or not. This is one of the things that you do. You try to judge what the consequences of it will be, and then you have to make some trade offs about, would it be better to be blind in one eye or walk with a limp and all of these things. So it's very complicated and requires a lot of value judgments to get into some of these levels.
The nice thing about these categories like IMPACT 2002 is that they have what would hopefully be a panel of experts going through and doing this in sort of a peer reviewed fashion. And they're coming up, and so now this is giving me a human health comparison of these different products. So it's bringing together all of those different seven or eight different indicators that impacted human health and put them together on one scale. And they do the same thing for ecosystem quality. And in fact, you can take it a stage farther, which is that you can put these all together in terms of eco points. So then that makes the balance between how much human health would you be willing to trade off against how much ecosystem quality.
So the important thing to remember about this is that as you start-- so that's very nice when I have something like IMPACT 2002 because, theoretically, I can compare these systems and I can get a picture of the overall environmental quality. Not just looking at one single issue like global warming potential, and not having to look simultaneously at 27 different indicators and decide how I want to trade it off. I've hopefully got some experts out there that have done that already, and I can sum everything up in terms of, say, one unit of eco points.
But the important thing to remember is that when you do that, you're making value judgments. That's built into the system that scores that. It's deciding how much you're willing to trade off these different categories.
So finally, the last step we do is the interpretation phase. So this was actually the long one, when we looked at the study overall, because they went through and trying to interpret all those earlier results in terms of what the goal in the study was. And essentially to do that, they had to go through and do all sorts of uncertainty analysis on that to make sure all of those assumptions they made along the way were still valid when they tried to go back and interpret it. If you wanted to come back and make a claim that the Dyson Airblade was better than the others, you better make sure that all of the limitations in the study aren't going to impact that.
So they essentially did three types. They did a sensitivity analysis, they did uncertainty analysis-- which was really two different types, one was a scenario uncertainty and the other was a bill of activities analysis. So in terms of what they did, the sensitivity analysis was pretty straightforward. They basically went through all of those assumptions they made, and they had a lot of them. There were probably 12 or 15 different major assumptions that they made. And they did a one factor at a time change to test the impact of that.
So they went through and some of them, they tested. When it goes to waste, there's a percentage of that that gets incinerated. So they tested how that changed, and different countries that have different waste systems and different ways of taking care of the waste will do that differently. So they went back and tested the impact of changing that percentage on the scenarios.
If we look at the scenarios, none of these really get changed because they're not really sending much to waste that's getting burned. And these change a little bit. But overall, looking at these different scenarios, you get a very similar picture than you did before. So it's not particularly sensitive to the assumption that we have here.
The other one has to do with the intensity of the drying time on the system. And so in this case, you start to get a very different picture between different scenarios, where you have some of these that are getting definitely higher impact and changing as we go through. So the sensitivity analysis was pretty straightforward. Go through one at a time, changing those factors between some different values, seeing what impact it has in the system.
The two things we said it identified were the electricity and the drying time, which drying time scenario you used. So it identifies those two as sort of the major ones that can affect the system. But one of things it doesn't really capture is that it does all this one at a time. And especially those two are related, so if I'm off on how long I dry, then I'm also off on my assumption about the intensity of the electricity. Those two factors might compound together to make my results even worse.
So this only captures what happens when they did it one at a time. So they went through and did a scenario uncertainty analysis. And essentially, they had 20 different parameters, and they had a range for each of them. And they assumed the distribution, uniform or some other type, and they went through and did a Monte Carlo simulation, where they did 20,000 different simulation runs where they randomly picked a value from that distribution, and calculated the impact on each of the different product systems. So this is global warming potential, and you get some sort of distribution about what the impact of the different products looked like. You can see how it changes based on all the different assumptions of what the possible range was.
One of the important things they did with this is you get some overlap. But if we look at that, the Airblade is definitely the lowest in terms of where it's shifted. But there's definitely some overlap between some of these other systems, and one of the things they did is they went back and checked that just because there's overlap doesn't mean that Xlerator was lower than this. It just means in certain scenarios, the Xlerator was lower than what the Airblade had in certain scenarios.
So what they did is they did a check. Because you can think that some of the assumptions might affect only one product or the other. But I may have underestimated the drying time on the Xlerator and overestimated the drying time on my product, and that may cause the products to shift in terms of which was preferred.
Some of the others, like if the average electricity makes up the grid, I wouldn't expect those to be different between one product and the other. They're both using the same electrical supply, so my assumptions about the impact of the grid should be the same between them. So they made sure they did that check by each time they ran one of these scenarios, they compared. Did the Airblade have a better performance than, say, the Xlerator, and went through. And even though you get this overlapping like this, they still found something like 98% of the scenarios, the Airblade was better.
Finally, the last thing they did was looking at the bill of activities. So for every emissions factor, essentially, and for every input to each of those systems, they assigned an uncertainty level to it, and assumed a distribution, and did another simulation so they'd get a range of different products like this. So these are just three different types of analysis they went through to try to figure out how much those assumptions and that uncertainty impacted what the results were.
So the big thing, though, is that even after they went through with that, the Dyson Airblade still showed the lowest impact in general. Under certain scenarios, it may be that the Xlerator performs better, but under most scenarios, they were pretty confident that the Dyson showed up better. They broke it out by different types of scenarios and said when one was going to be better than the other.
So in the conclusion they identified what are the most sensitive parameters, what was the key driver, the environmental impact, and what was the general overall environmental performance comparing the two, taking into account all of that sensitivity. OK.
Free Downloads
Video
- iTunes U (MP4 - 180MB)
- Internet Archive (MP4 - 180MB)
Subtitle
- English - US (SRT)