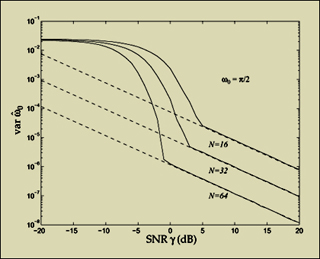
Example of threshold phenomenon in nonlinear estimation. (Image courtesy of Alan Willsky and Gregory Wornell.)
Instructor(s)
Prof. Gregory Wornell
Prof. Alan Willsky
MIT Course Number
6.432
As Taught In
Spring 2004
Level
Graduate
Course Description
Course Description
This course examines the fundamentals of detection and estimation for signal processing, communications, and control. Topics covered include: vector spaces of random variables; Bayesian and Neyman-Pearson hypothesis testing; Bayesian and nonrandom parameter estimation; minimum-variance unbiased estimators and the Cramer-Rao bounds; representations for stochastic processes, shaping and whitening filters, and Karhunen-Loeve expansions; and detection and estimation from waveform observations. Advanced topics include: linear prediction and spectral estimation, and Wiener and Kalman filters.