Flash and JavaScript are required for this feature.
Download the video from iTunes U or the Internet Archive.
Topics covered: Hard-decision and Soft-decision Decoding
Instructor: Prof. David Forney
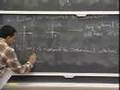
Lecture 3: Hard-decision an...
Related Resources
Hard-decision and Soft-decision Decoding (PDF)
PROFESSOR: And what we saw was the performance was quite different in the two regimes. In power-limited regime, typically our SNR is much smaller than one, whereas in the bandwidth-limited regime, the SNR is large. And because of this behavior of SNR, what we saw is that the Shannon spectral efficiency in the bandwidth-limited regime, it doubles for every -- if we double our SNR. For every 3 dB increase in SNR, the spectral efficiency increases by a factor of 2.
In the bandwidth limited regime, if we have a 3 dB increase in SNR, the spectral efficiency increases by one bit per two dimension. On the other hand, if we double our bandwidth, then the capacity in bits per second is not affected in the power-limited regime. But if we double our bandwidth, then in the bandwidth-limited regime, the capacity increases approximately by a factor of 2.
And that's really what motivated the name bandwidth-limited and power-limited regime. If we want a more operational definition, then we say that the flow is less than two bits per two dimensions, we will have this bandwidth-limited regime. And in this case, rho is greater than two bits per two dimensions.
The number two bits per two dimensions was chosen because it is like the largest spectral efficiency we can get through binary transmission. If it's an uncoded two-PAM over a channel, then we get two bits per two dimensions. If we are coding, the only thing we can do is reduce spectral efficiency. So typically, if we are going to operate in power-limited regime, the operational meaning is we can get away with binary transmission. In the bandwidth-limited regime, we have to resort to non-binary transmission.
So in other words, binary modulation is done in power-limited regime, whereas we need multi-level modulation in the bandwidth-limited regime.
The baseline system here is 2-PAM. The uncoded performance was of 2-PAM. In the bandwidth limited regime, it is M-PAM. And the way we measure performance in the power-limited regime is the probability of bit error as a function of EbN0. OK? In the bandwidth-limited regime, the performance measure is done by probability of error per two dimensions as a function of SNR norm.
And in this case, we saw that the gap to capacity -- or rather, to put it in other words, the ultimate limit on EbN0 is minus 1.59 dB. And here, the ultimate limit on SNR norm is 0 dB.
OK? Any questions on this? Yes.
AUDIENCE: Why do we use Eb over N_0 SNR norm for [INAUDIBLE] bandwidth limited?
PROFESSOR: That's a good question.
AUDIENCE: Why do we use Eb over N_0 for both regimes?
PROFESSOR: Or why don't use SNR norm for both regimes?
AUDIENCE: Yeah.
PROFESSOR: Now if we think about the bandwidth limited regime, what we really care about is spectral efficiency, right? What SNR norm does, if you remember the definition, is that it compass the amount of SNR we require for a practical system to that of the best possible system. So in other words, if you do care about spectral efficiency, SNR norm is the right measure to look for.
OK, now what happens in the power-limited regime? It turns out, probably more for historic reasons, people started with EbN0 in the power-limited regime. And if you look at the definition of EbN0, it is SNR over rho, right? So in the power limited regime, our rho is small, the SNR is going to be small, but if you look at -- because we are in the power limited regime so we have lots of bandwidth, so our SNR is going to be small and the spectral efficiency is going to be small. But if you look at the ratio between the two, it's going to be greater than minus 1.59 dB.
OK. so it turns out that the kind of limit we do take, our EbN0 remains constant as minus 1.59 dB, and that's probably one of the reasons that motivated to use EbN0 in the power limited regime. On the other hand, one could also argue is that what really happens in the power limited regime is that our bandwidth becomes really large. So if this [UNINTELLIGIBLE] stick with 2-PAM system, then we do get a spectral efficiency of two bits per two dimensions, but that's just because we are using a particular modulation scheme. If our bandwidth is really large, we are not really going to care about what spectral efficiency we use. What really matters is this energy per bit, and that's why this is a reasonable assumption.
Does that answer your question? Right, it's not completely clear as to why this EbN0 is the best here, and SNR norm is here if you don't take the limit rho going to zero here, but again, you can think of it more as a convention. OK.
AUDIENCE: [INAUDIBLE]
PROFESSOR: So if you look in the power-limited regime, you are saying rho is less than two bits per two dimensions. If you use an uncoded 2-PAM, what's your spectral efficiency? It's two bits per two dimension, right? Now the idea is, suppose we want to design a system with spectral efficiency greater than two bits per two dimensions? We cannot really use a 2-PAM system. Because if you put coding on top of it, all we are going to do is simply reduce the spectral efficiency below two bits per two dimension. So we have to start with a non-binary modulation, right? So that's how we distinguish between power-limited and bandwidth-limited
AUDIENCE: That's just because you're using the 2-PAM as your baseline [INAUDIBLE]?
PROFESSOR: Right. OK? All right. So let us do an example to finish off this analysis.
Now suppose -- say you are at a summer project, and you're assigned to design some system. Your boss gives you some specifications, like continuous time specifications. In particular, you have a baseband system. The baseband system has a bandwidth of one Megahertz. You have a power, P, which is one unit, so that's another resource you have. And if you measure your channel, it can be reasonably approximated as an AWGN channel, so there is no ISI or any filtering going on, just Additive White Gaussian Noise. And your noise a single sided spectral density of ten to the minus six units per Hertz of the bandwidth.
And what is your goal? So you have the following goal. Design a 2-PAM system, with a specified probability of bit error. And what you want to do is compare this to the ultimate Shannon limit. So that is your objective.
So since we have to compare it with Shannon limit, and we have already a formula for the Shannon limit, let's just start with that. So for this problem, we have the Shannon limit. You have rho is less than log base 2 of 1 plus SNR. For SNR, I can talk in terms of this continuous time parameters, it's P over N_0 W. My P is one unit, and nought is ten to the minus six, and my bandwidth, W, is one Megahertz here, which is ten to the six. So my P over N_0 W is basically one. So I have 1 plus 1, which is 2. This is log base 2 of 2, or it's one bit per two dimension.
So my capacity in bits per second is rho W, and rho is one bit per two dimension, the bandwidth is one Megahertz. So I get ten to the six bits per second. So this is my Shannon capacity for this particular AWGN system. OK.
The next thing we want to do is compare this with a practical system, and see how close we get to the Shannon limit. And since you only have to work with 2-PAM, the generic architecture is something we saw last time. You have input bits coming in, let's call them X sub k, where k is the kth bit. And they belong to a certain constellation, let's call the -- the constellation points are just -- it's a 2-PAM system, so we have minus alpha and alpha.
And this goes through a PAM modulator, and one parameter to specify for the PAM modulator is the symbol interval. Right, the time between sending consecutive signals over the channel. What you get out is X of t, this is the channel model, N of t. There's already noise over the channel, and what you get out is Y of t.
So this is the generic architecture. And now, your goal for the design problem is to select alpha and t in the right way, so that they satisfy this continuous time constraints, and at the same time, you have your probability of error of ten to the minus five.
So what would be an obvious choice for T?
AUDIENCE: [INAUDIBLE]
PROFESSOR: Right. So the first idea is you are given a certain amount of bandwidth, and you clearly want send your signals as fast as possible in order to get excellent data rate. Now because you have a certain amount of bandwidth, what Nyquist's criteria tells you is that you want to have zero ISI. And if you want to have zero ISI, what you do know is that the symbol interval should be greater than or equal to 1 over 2W. You cannot signal at a rate faster than one over T, and so if we look at this, it's 1 over 2 times 10 to the 6.
Now it I do use this particular value of T, then what's my alpha going to be? Well, alpha is simply the energy per symbol. So I know alpha squared is the power that I have times the symbol interval, T. It's just a definition. This comes from orthonormality of the PAM system that we have. Now P is one, because that's what I specified as a system specification, so this is just T, which is one over times ten to the six. So I can select this value of alpha and this value of T.
AUDIENCE: [INAUDIBLE]
PROFESSOR: Well, alpha squared is energy per symbol. So what will that be? What's the energy per symbol, if you're sending every T seconds, and if you have a power of P? Es, that I mentioned last time, or energy per two dimensions. So in PAM, it will be energy per symbols. In that case, it will be 2P.
OK. But now if I select these values of alpha and T, will my system work? Is this a reasonable design, or is there something wrong here? I'm clearly satisfying my --
AUDIENCE: [INAUDIBLE]
PROFESSOR: The probability of error, right, exactly. So in fact, I do know how to calculate it, right? What's the probability of bit error? Well, we saw that last time it was Q of root 2 Eb over N_0. Eb is same as alpha squared, because we have one bit per symbol. So alpha squared is this quantity here, 1 over 2 times 10 to the 6. So this is Q of square root of -- so 2 alpha squared is 10 to the 6, 1 over 10 to the 6. N_0, I know, is ten to the minus six, so this is actually Q of 1. And if I do calculate that, it's like 17 percent, which is nowhere close to ten to the minus five.
So any suggestions on how I can improve my system?
AUDIENCE: Increase T [INAUDIBLE]
PROFESSOR: Increase T, right? What's happening right now is -- the reason we selected this value of T in the first place is because we wanted to send our signals as fast as possible avoid ISI, but that's just one of criteria in my system, right? I have to also satisfy this probability of error criteria, so I want to make sure my probability of error is going to be small.
If I look at the expression for probability of error, it doesn't really look at T. All it looks at is this ratio of Eb/N0, right? So if I want to reduce my probability of error, I have to increase my energy per bit. Now my energy per bit is P times T, so the only hope of increasing my energy per bit will be to increase T, which means I have to signal at a slower rate.
OK? So we have probability of -- let's write the calculation down. It's ten to the minus five. Last time, we saw that the best way to solve this is to look at the waterfall curve, and EbN_0 in this case is approximately 9.6 dB. I will say that that's approximately ten on the linear scale. So this implies that energy per bit is ten to the minus five. So energy per bit is P times T, in this case, it's ten to the minus five. p is one, so this implies that t is ten to the minus five. So I can send one bit every ten to the minus five seconds. So my rate that I achieve -- just write it here -- which is ten to the five bits per second. OK?
If you compare this to the Shannon limit, the Shannon limit is right here, you have ten to the six bits per second. So you lose by a factor of ten in your data rate if you're going to use an uncoded 2-PAM system. So what this example tells you is that if you're going to do more sophisticated cording, you can gain up to a factor of ten in your data rate.
So if the 10 dB did not really impress you last time, hopefully this example throws more light on the value of coding. Are there any questions on this example?
AUDIENCE: Since the -- since we are signaling at a faster rate now, instead of using sink process, we can use something better.
PROFESSOR: That's a very good point, yes. Well, if you look at the nominal bandwidth here, it's 1 over 2T, right? T is 10 to the minus 5 seconds, so this says 1 over 2 times 10 to the minus 5. So it's going to be 5 times 10 to the 4, or 50 KHz. OK? The available bandwidth you have, the system bandwidth, if you will, is 1 Megahertz. But if you're going to do Nyquist's ideal sinks pulses, then you only need 50 KHz of bandwidth in your system, right?
So one advantage of this system, if you will, is that you're not required to do the complicated sink pulses. Do not need to send those pulses. You could simply send, for example, square pulses and, because your bandwidth is such low, you have a very low complexity system. Of course, the price you pay is you reduce the data rate by a factor of ten. OK, it's a good point.
In fact, there are many points that will come up in this example if you think about it later on, so feel free to ask me questions if you think about some issues later on.
Ok. So I think we have motivated the need for coding enough now, so let's look at our encoder design. So a typical encoder design takes bits in -- we saw this last time in the context of spectral efficiency -- and produces symbols out.
So I can represent my bits by, say a vector b, and I can represent my symbols by a vector x. So every sequence of b bits gets mapped to a sequence of N symbols. Now this output sequence of symbols is not any arbitrary sequence, but it lies in a set of all possible sequences, which I denote by C. And this set is essentially a set of permissible output symbol sequences, which I will write by C sub j, which is a vector in Rn, because there are N symbols being produced. And we can have set up to j, j goes from 1 to M. So we can have up to M symbols. And note that here, M has to be equal to 2 to the b, in order to be able to map every sequence of b bits to M symbols.
So this C is known as a codebook, and each C sub j is called a codeword. OK? The standard definition of an encoder.
Now in today's lecture and half of next week's lecture, we will be seeing at a very specific case when N equals 1 and 2. In that case, instead of using the letter C, we will be using a different letter, A. So C, in that case, we'll call it actually a constellation. So in particular if C is one, it's a PAM constellation. If N is 2, it's a QAM constellation. And we'll be denoting it by a letter A instead of C.
So A is again, a sequence of symbols a_j, which belongs to Rn, where one is less than j is less than M. OK, in this case a is a constellation. a_j's are known as symbols, or sometimes they're also known as signal points in the constellation.
There are a number of definitions that follow from this -- number of properties of the constellation, rather, that follow. So in particular N is known as the dimension of your constellation. The number N is this, and here, it's the number of symbol sequences you output for a sequence of b bits that are in. M is the size of your constellation. The energy per constellation is given by 1 over M times the summation the norm of a_j squared, where j goes from one to M. The minimum distance of your constellation is simply the Euclidean minimum distance between two points in the constellation. So if you take the norm of a_i minus a_j, and minimize it over all possible values i and j. The number of nearest neighbors, of the average number of nearest -- K_min of A is the average number of nearest neighbors in A.
In addition to this, there are some orthonormalized parameters that you saw last time. The spectral efficiency, which is in units of bits per two dimensions is 2b over N. And if you want to eliminate b, we use the relation that b is log M to the base 2 here. And so we have 2 log M to the base 2 over N.
The energy per two dimensions, denoted by Es, is simply 2 over N E(A). So E(A) is the average energy of your constellation. If you divide it by the number of dimensions you have, you get energy per dimension, and you multiply it by 2.
And finally, the energy per bit is Es over rho, or it can also be expressed as E(A), which is the energy per symbol over the number of bits per symbol, which is log M to the base 2.
It might seem like a lot of definitions, but you will see very soon that they have a very tight interplay among one another, so it's not nearly as overwhelming as it might seem at the first point.
AUDIENCE: [INAUDIBLE]
PROFESSOR: Yes.
AUDIENCE: Why is [UNINTELLIGIBLE]?
PROFESSOR: Because you have two [UNINTELLIGIBLE] b bits coming in, right, which you map to each --
AUDIENCE: [INAUDIBLE] There are two [INAUDIBLE] possible sequences, but all of them need not be used, right?
PROFESSOR: Well, we assume that there is no coding going on before the encoder. So you have the source code, for which there's a sequence of IID bits, and then they mapped to a sequence of symbols. So we'll see all of our possible input bits coming in here, because it's produced by a source code, like a Huffman code. Right? And the idea here is, perhaps what you're asking is -- this did not span the entire space of Rn. We want to select these sequences carefully here. Maybe we'll come back to that later on in the course.
OK, so let's do an example. So the example is, say we have A, which is a 2-PAM system, and you want to look at this constellation, B, which is denoted by A raised to K. The definition of A raised to k is it's a sequence of K symbols where each x_i belongs to A. So this is also known as the K-fold Cartesian product of A.
AUDIENCE: Another question. So it has been pre-decided that b bits will be encoded [UNINTELLIGIBLE]?
PROFESSOR: Right. So this is a specific structure we are imposing on the encoder. So this is the constellation, and you'll want to study the properties for this constellation. For this constellation, what's N going to be? What's the dimension going to be?
AUDIENCE: [INAUDIBLE]
PROFESSOR: For B, not A. It's going to be K. Well, the number of points in this constellation, how many points are there? There are K coordinates. Each coordinate can be plus or minus alpha. So we have 2 to the K possible points in this constellation. OK? What's E of A going to be?
AUDIENCE: [INAUDIBLE]
PROFESSOR: K alpha squared. right? Basically, we have K coordinates. The energy for each coordinate will simply add up. The energy across each coordinate is always going to be alpha squared. So each point in this constellation has an energy of K alpha squared. So regardless of how many points we have, the average energy is always going to be K alpha squared. Does everybody see this?
AUDIENCE: [INAUDIBLE]
PROFESSOR: You're right. Maybe that was the confusion. It's a good thing. Just getting too used to writing E of A. OK. What's d_min of b going to be?
AUDIENCE: [INAUDIBLE] 2 alpha.
PROFESSOR: 2 alpha. I think everybody had the right idea. So the minimum distance here is two alpha for A. If we look at this point B, we can fix K minus 1 coordinates for two points to be the same, they will only differ in one point. And so the minimum distance is across that point, which is 2 alpha.
What is K_min of B going to be? It's going to be K. For each point -- let's say the point which has all alphas, we can fix K minus 1 coordinate and find another point which is different in only one of the coordinates, say the first coordinate. We can do it for all K different coordinates, so K_min is going to be K for each point, and hence the average number of nearest neighbors is also K.
OK, so now in this case, let's first start with the normalized parameters. That's always good to start with spectral efficiency. That's 2 log M over N. Well, log of M is going to be K, so N is going to be K. So this is going to be two bits per two dimensions, and this is the same as that of the original constellation, A. Your energy per two dimensions is going to be 2 over N E(B). E(B) is K alpha squared, N equals K, so this is 2 alpha squared, and that is the same as the original constellation, A.
Finally. energy per bit is Es over rho, so it's 2 alpha squared over 2. So that's alpha squared, and that's same as the 2-PAM constellation.
So why did I go through all of these calculations? What we see is that the normalized parameters, rho, Es, and Eb, are the same for the Cartesian product as for the original constellation. And at some level, that should not be too surprising, right? Because what I'll be doing in this Cartesian product, we are not really doing any coding, right?
In this original constellation, we had one bit coming in, and we are mapping it to one symbol. All we are doing in the Cartesian product is we are taking K bits in and mapping them to K symbols. So we still have one bit per symbol. The noise is IID, so it's optimal to two decisions for each of the coordinates independently, and decide whether that coordinate corresponds to plus alpha or minus alpha. So in other words, there's nothing gained by doing this Cartesian product.
And we will see, the probability of error expression depends on these normalized parameters, if we want to look at Pb of E, and so we do not gain anything in terms of the probability of error, versus EbN_0, trade-off through Cartesian product.
So I'm making the note here because that's the only space I have. So the note is if I look at probability of bit error versus EbN_0, the curve we saw last time, it is the same for B and A. You should be able to convince yourself about this, and so there is really no coding going on here. Are there any questions on this?
Let's look at this problem a bit more carefully now.
AUDIENCE: [INAUDIBLE]
PROFESSOR: Yeah.
AUDIENCE: Why [INAUDIBLE]
PROFESSOR: Right.
AUDIENCE: What does he use?
PROFESSOR: Energy per two dimensions. Es will always be energy per two dimensions. Throughout the course, we'll be using these notations. Eb is the energy per bit, Es is the energy per two dimensions. And if you want to say energy per symbol, we'll be using this notation E sub the constellation.
AUDIENCE: Oh. It's not energy per bit.
PROFESSOR: No, this is energy -- B is my constellation. So that's why it's energy of that constellation, average energy per symbol in that constellation.
OK, so let us consider the special case when K equals 3. So in that case, B is A^q. So if I look at all possible points in B, they are going to lie on the vertices of a three-dimensional cube. That's a Cartesian product in three dimensions. And all my constellations points are basically on the vertices of this cube. The distance here is going to be 2 alpha. That's the length of each edge in my cube, and that's what B is going to be. Clearly, the minimum distances is 2 alpha, as we saw before.
Now let me define a different constellation, B prime, and only going to take four vertices from these possible eight vertices. I'm going to take this vertex here, I'm going to take this vertex here, this one, and this one. I'm only taking four vertices. If I want to tell you explicitly what the points are, I need to draw an axis, so I'm simply drawing the x, y, and z axis here. This is x-axis, this is y-axis, and z-axis. And B prime is a subset of the points in this three-dimensional Cartesian product. They will be alpha, alpha, alpha; minus alpha, minus alpha, alpha; alpha, minus alpha, minus alpha; and let's see, minus alpha, alpha, minus alpha. So two of the coordinates will be minus alpha here, in these three points, and we have one coordinate all alphas.
So this is my B prime. What is the minimum distance going to be for B prime?
AUDIENCE: [INAUDIBLE]
PROFESSOR: 2 over 2 alpha, right? It's basically the length of this edge here. This is 2 alpha, this is 2 alpha. So it's 2 over 2 alpha. So in other words, by simply selecting a subset of points, I have been able to increase my minimum distance. Because my minimum distance is larger, I hope that the probability of error will be smaller as opposed to the original constellation.
But this comes at the price, right? And what's the price? The spectral efficiency is smaller, right? What if I look at my spectral efficiency? Well, I'm only sending out two points, two bits per each point. So two bits, each point takes three dimensions, so my spectral efficiency is 2 times 2 over 3 bits per two dimensions, or it's 4 over 3 bits per two dimensions. And this is in contrast to the two bits per two dimensions we had for B.
So in other words, there is a trade-off between your spectral efficiency and the minimum distance. We'll start with a K-dimensional Cartesian product of A, which has all points. We took a subset of points, and if we chose them smartly, we were able to increase the minimum distance, but the price we had to pay was to reduce the spectral efficiency.
AUDIENCE: Where did this two / three come from?
PROFESSOR: This two here?
AUDIENCE: 2/3, yes.
PROFESSOR: 2/3, I am sending two bits per symbol, right? Each symbol has three dimensions. So it's 2/3 bit per dimension, or 4/3 bit per two dimension.
OK, so the point was it seems like there is a trade-off between minimum distance and spectral efficiency. And indeed, this might seem like a reasonable trade-off, and a lot of coding here that we will be seeing in the early part of the course is indeed motivated by this trade-off. You want to reduce your spectral efficiency in order to increase your probability of error. And this has in fact been quite a dominant design principle for a large number of codes that have come up in coding theory.
However, if you look at what Shannon says, Shannon says something quite different. In Shannon's theorem, all they say is, you have -- if your spectral efficiency is below a certain amount, then your probability of bit error can be made arbitrarily small. OK, so what Shannon is saying, it's something much stronger than this trade-off. It's saying if you reduce your spectral efficiency below a certain quantity which is finite, then the probability of error can be made arbitrarily small. There is no statement of minimum distance in this theorem here.
And indeed, if you look at the most modern codes which are capacity approaching, they are not designed to maximize the minimum distance. They are designed to work well with some practical decoding algorithms, like the belief propagation of algorithms and so on. So they are designed on a somewhat different principle than minimum distance.
But nevertheless, this is quite a powerful tool that we will be using in the early part of this course. We start with a K-dimensional Cartesian product, select a subset of points, and we want to increase the minimum distance at the cost of spectral efficiency. OK. Now are there any questions?
AUDIENCE: [INAUDIBLE]
PROFESSOR: That's a good question. Suppose I have a 2-PAM constellation, then I can easily write the probability of bit error as a function of Q function. If it is a more complicated expression, I have to integrate over the decision regions, which we'll be seeing later on in this lecture. And it's not usually possible to get an exact probability of error expression. We usually use an in-union bound, to bound it by a pair of [UNINTELLIGIBLE] error probability. We'll be doing all that just now.
OK. So now let us -- I have talked now enough now about encoder, and we'll be visiting it very soon, but let us switch gears and talk about the decoder now. OK, what does a decoder do? So the goal of a decoder is the following. You get your received vector Y, which is X plus N, and from Y, you want to estimate X-hat as a point in your signal constellation. So you receive a noisy version of X, and you want to estimate X-hat at the decoder. So this is the architecture of your decoder.
And the goal here is you want to minimize the probability of error. And what's the probability of error? It's basically probability that X is not equal to X-hat. So that is your general criteria at the decoder. Now what we'll doing next is basically going through this exercise to show that this minimum probability of error criteria is equivalent to a bunch of other criteria.
So the first criteria is the MAP criteria: Maximum A-Posteriori Rule. So our probability of error is basically -- I can track it as an integral of probability of error given Y times the density function of Y. So if I want to minimize my probability of error, I want to minimize each term in this integral. So this implies I want to minimize probability of error given Y for each possible value of Y. OK?
Now what's that going to be? Well, in order to look at what this term is, suppose I make a decision. I receive Y, and I decide a symbol a_j is sent. Then what's the probability of error going to be? My probability of error given Y is going to be 1 minus the probability that I was correct. Probability that I was correct is probability X equals a_j, given Y. This follows from the definition.
So if I want to minimize my probability of error given Y, I want to actually choose an a_j that maximizes the probability of a_j given Y. So this implies, choose a_j. And this is known as the MAP rule. So the idea behind the MAP rule is to choose the symbol in the constellation that maximizes the posterior probability, given the received symbol.
Now, this MAP rule is equivalent to the maximum likelihood rule, under the assumption that all the signal points a_j are equally likely. The proof is not hard, you just use Bayes Theorem for that. So suppose all a_j's are equally likely. Then probability of a_j given Y, which by Bayes Theorem is the density of Y given a_j, times the probability of a_j. But since all a_j's are equally likely, I will just write it as 1 over M, over the density of Y. Now because Y is fixed, the density of Y is fixed, so this quantity is just proportional to -- the proportionality symbol -- to the density of Y given a_j. I won't be writing all the vectors, I might be missing some. But please bear with me.
So this implies we want to choose a_j that maximizes the density of Y given a_j, and this is known as the maximum likelihood rule.
And there is one final rule. Basically if the noise is additive Gaussian, then the density of Y given a_j is simply proportional to E raised to minus the norm of Y minus a_j squared. So if we want to maximize this quantity, we want to minimize Y minus a_j squared. So we want to choose a_j that minimizes the Euclidean distance between Y minus a_j. And this is known as the minimum distance decision rule, MDD rule. Yes?
AUDIENCE: We are ignoring [UNINTELLIGIBLE]?
PROFESSOR: We are ignoring P value. Because for a given Y, Py is going to be fixed for all possible choices of a_j. The goal is I'm given Y, and I want to decide which signal point was set, because that's the probability of error given Y. This is my criteria now. So Y is fixed, so the density of Y is fixed.
AUDIENCE: [INAUDIBLE]
PROFESSOR: It's basically given by -- in order to find this density, we'll just condition it on a_j, and sum up over all possible values of a_j. Just take the marginal of Y, right? I mean, to write this explicitly. I'm writing it here. It's going to be sigma P of Y given a_j that's the probability of a_j. And this you can find by the Gaussian.
AUDIENCE: But then you have [INAUDIBLE].
PROFESSOR: But this is a summation over all possible a_j's. I should write, sorry -- a_k's. This is just a summation over all k's, right? So basically, Py is going to be a mixture of several Gaussians, OK? And it's fixed.
AUDIENCE: [INAUDIBLE]
PROFESSOR: Right. OK. So we want to choose the Minimum Distance Decision rule, and I should have the variance of noise here. OK, so what we have so far is we started with the Minimum Probability of Error rule, and that's the criteria of your decoder. Be sure this is equivalent to MAP rule, and that basically comes just from the definition. This integral here, we want to minimize each term in the integral, and that basically implies that the Maximum A-Posteriori rule is the best. This implied Maximum Likelihood rule, and Maximum Likelihood rule comes from the fact that all the signal points are equally likely. And this implies, then, the Minimum Distance Decision rule, and that comes from the fact that your noise is Additive Gaussian. So you have an exponential in the -- you have the Euclidean distance as an exponent, and you want to minimize the Euclidean distance.
So this is the story we have so far. And it turns out that the Minimum Distance Decision rule is actually quite nice, because it gives you a lot of geometrical insights. So say I have three points. We not even draw the coordinates. My constellation, A, has three points, and let me write them as a1, a2, a3. This is my constellation, for example. And say I receive a symbol Y. Then the job of the decoder is to figure out whether I sent a1, a2 or a3.
How will the decoder do that? Well, it will measure the distance from all the three constellation points and select the one with the smallest Euclidean distance. More generally, what we want to do is we want to look at the space of all received Y symbols, and partition it into decision regions. So that if the point falls in a certain decision region, we say that the constellation point corresponding to that decision region was sent.
And how do I find the decision region? Well, I start drawing hyperplanes between every two pair of constellation points. Say I want to find the decision region of point a1. I draw a hyperplane between a1 and a3, which is given by this line. I draw a hyperplane between a1 and a2 which is given by this point. And so the region -- the set of points which are closer to a1 than a2 and a3 is basically bounded by this region here. So this is my R1.
Similarly, if I want to find a decision region for a2 and a3, I will draw this line here. This will be R2 and this will be R3. So these are my decision regions. So if I want to write that formally, Rj is my decision region. And it is the set of points Y belong to Rn, such that the norm of Y minus a_j squared is going to be less than or equal to -- it doesn't matter if you have less than or equal to, because the point [UNINTELLIGIBLE] bound [UNINTELLIGIBLE] probability zero -- is radius squared. That's just the definition of Rj.
Now the way to construct Rj was to look at all the half planes which are closer to this point than any other point, and take the intersection of all the half planes. So in other words, I can also write Rj to be the intersection of these half planes -- the intersections over all points, j prime not equal to j -- of Rj, j prime. So Rj, j prime is your half plane where -- so norm of Y minus a_j prime squared is greater than or equal to norm of Y minus a_j squared. Note that this is a_j prime, and this is a_j here.
OK. So it turns out that this decision region has a somewhat nice structure, because they're intersection of a bunch of half planes, their shape is the convex polytope. And they're also known by the name Voronoi regions. OK, so these regions are known as Voronoi regions here.
Now the set of points whose hyperplanes are active in a certain decision region has a special name, too, and it's called the relevant subset. So in this case, the relevant subset of a1 is a2 and a3, because both of them have hyperplanes that are active in the decision region of a1. So let me write that down. So the relevant subset is the set of points, a_j prime, whose hyperplanes are active in this decision region Rj. There's a theorem which says that the nearest neighbors are always included in the relevant subset. It's asserted in your notes. OK?
So now that we have this Minimum Distance Decision rule, let us see if we can get a hang with the probability of error. Let me see the probability of error, given that I sent a symbol, a_j. I want the value that probability of error. That's simply the probability that Y does not belong to Rj, given that I sent the symbol a_j. That's when an error happens. That's same as probability that the noise vector -- because Y is a_j plus N now -- does not belong to the Rj minus a_j, and the noise is independent of a_j so I remove the conditioning. And that is 1 minus the probability that the noise does belong to Rj minus a_j.
If I want to find this integral, find this expression, I will integrate over the region Rj minus a_j, of the density of the noise, dN. No note that the noise has a spherical symmetry, but unfortunately despite that, the integral is not a straightforward integral, because this region here has sharp edges. The decision region is a convex polytope, so it's typically something like this, and if this was your point, a_j, your noise does have a spherical symmetry about these spheres, but when it intersects the decision boundary, things get ugly. And so this decision -- this is not a nice integral in general.
And so unfortunately, there is not much progress we can make beyond this point for the exact probability of error expression, but we can say some nice geometrical properties about the probability of error.
The first property is that probability of error is invariant to translations. And this should be quite obvious. You have, say, a constellation with two points here, and say I subtract off the mean. So I get a different constellation whose points are like this. This is my constellation, A, and this is my constellation, A prime. The probability of error will be same for the two constellations because the decision regions will have the same distance from both the points. This should be quite obvious.
And basically, what this really says is if I have any constellation, I can always subtract off the mean, and get another constellation with the same probability of error, but with smaller average energy. And so this implies that any optimal constellation will have zero mean.
The second point is, the probability of error is invariant to orthonormal rotations. So if I had, say, one point, one constellation, with these four points, and I rotate it by 45 degrees, what I get is another constellation with these four points. And both these constellations are simply rotations of one another, and they have the same probability of error. And the easiest way to see that is the decision regions here are simply the four quadrants. And if I want to integrate my probability of error, I will be integrating it over this region.
Here, my decision regions will be these 45 degree lines. And if I want to integrate the probability of error for this point here, it will be given by noise, which is symmetric about these circles. Basically, since the noise is invariant to orthonormal rotations, it should be quite obvious that the probability of error is invariant to rotations.
AUDIENCE: [INAUDIBLE]
PROFESSOR: Any rotation, right.
AUDIENCE: So what do you mean by [UNINTELLIGIBLE]?
PROFESSOR: Basically, you're preserving the distance. So you're just rotating the point, not scaling it.
AUDIENCE: [INAUDIBLE]
PROFESSOR: Unitarily. OK? I mean you will be proving these properties in the next homework, which is just handed out.
AUDIENCE: So those [UNINTELLIGIBLE] hold, because Minimum Distance rule is orthonormal, right? And that's because you assume Gaussian --
PROFESSOR: Because you assume Gaussian noise.
AUDIENCE: So that's the only assumption we make?
PROFESSOR: Right.
AUDIENCE: -- for those [INAUDIBLE], right?
PROFESSOR: I think so.
AUDIENCE: Why [INAUDIBLE] constellation must have zero mean?
PROFESSOR: Because if I have any constellation, there's a certain probability of error, right? Can always subtract out the mean from the constellation, I get a new constellation with a smaller average energy with the same probability of error.
AUDIENCE: Oh, so in terms of [INAUDIBLE]
PROFESSOR: Right. If you're looking at a trade-off of probability of error versus energy, usually what we look at. So maybe that's a good point. I should just mention it. For probability of error versus -- we're looking at this trade-off here.
Ok. The next idea is to basically bound the probability of error by a union bound, because we cannot compute an exact expression for the probability of error, so we might as well compute a bound which is tractable. So we'll look at what is known as the pairwise error probability.
So the idea behind pairwise error probability is suppose I send a point, a_j, what is the probability that instead of a_j at the receiver, I decide that a_j prime was sent. This is the pairwise error probability. So geometrically, say a_j and a_j prime are two points here. Let me draw some coordinate axis here. And say I sent point a_j, and there is noise on the channel, that takes me to this point. So this is my Y, and this is the noise vector. OK?
And now what I want to know is under what conditions will I decide a_j prime over a_j. What is the probability of deciding a_j prime over a_j? So let's draw a line joining a_j prime and a_j. So how would I decide -- suppose I receive this point, Y, and I wanted to decide between a_j and a_j prime. What would be my decision rule?
AUDIENCE: [INAUDIBLE]
PROFESSOR: Uh-huh.
AUDIENCE: [INAUDIBLE]
PROFESSOR: You select --
AUDIENCE: [INAUDIBLE] a_j prime.
PROFESSOR: Exactly. An equivalent way of saying it is to project Y onto this line, a_j prime minus a_j. We take two projections, one orthonormal to the line, one along on the line, and receive this projection. This is a straight line like this. Let's call it n tilde. I should change my chalk, it's getting too blunt now. This projection here is closer to a_j prime or a_j.
So in other words, this probability of error is same as the probability that this n tilde, which is the projection of Y onto a_j prime minus a_j, is greater than or equal to the norm of a_j prime minus a_j over 2. OK, now why did I use the notation n tilde here? Because the projection Y onto a_j, which is this. n tilde is same as the projection of the noise onto a line joining a_j prime minus a_j. So n tilde, I can write it as projection of N onto a_j prime minus a_j over the norm.
AUDIENCE: [INAUDIBLE]
PROFESSOR: Sorry?
AUDIENCE: Why, exactly?
PROFESSOR: You can just see geometrically, right? This is a 90 degree here. This is the noise. If I project the noise, it will be this component here.
AUDIENCE: [INAUDIBLE]
PROFESSOR: All right. This should be 90, I'm sorry. This is 90. I'm messing things up. OK, this is the noise here. This noise, if I project it onto a_j prime minus a_j, it's going to be this component. This is Y, if I project it, it's the same component.
Now, if the noise is IID with variance sigma squared in each coordinate, we are simply projecting the noise onto one orthonormal vector. So n tilde is also Gaussian, with zero mean variance sigma squared. So we can use that to find this probability of error. So in that case, the probability of error -- I should write this -- probability of a_j prime going to a_j is simply probability that this Gaussian is greater than some distance, and that's Q of norm of a_j prime minus a_j over two sigma. Yes?
AUDIENCE: What is sigma?
PROFESSOR: So the noise vector is IID, in each of the components, and has a variance of sigma squared. Sigma is basically N_0 over 2, if your noise is flat with -- so let me just write that down, sigma squared is N_0 over 2. If you have an AWGN channel, and you project it on each orthonormal signal, that's what you get.
AUDIENCE: What if you project the noise vector on [INAUDIBLE] why is [INAUDIBLE] you don't have --
PROFESSOR: So you have a noise vector. If you have a Gaussian vector, and you project it onto an orthonormal basis --
AUDIENCE: Yes. But [INAUDIBLE] normal?
PROFESSOR: Right. [INAUDIBLE] We are only projecting out on one vector which you need now.
AUDIENCE: So then you're saying -- OK, yeah. The assumption is the noise is symmetric in all dimensions?
PROFESSOR: Right. Let's do this algebraically, so you're convinced that there is no magic I'm doing here. So we can write this as you said, as the probability that the norm of Y minus a_j squared is greater than norm of Y minus a_j prime squared, given that Y [UNINTELLIGIBLE] a_j, so Y is a_j plus the noise vector. So I sub in for Y. What I get is probability that Y is a_j plus N. So here I have norm of N squared is greater than or equal to norm of a_j plus N minus a_j prime squared. And since the only random variable here is this noise, N, I can remove the conditioning [UNINTELLIGIBLE] down there. Let me expand this second norm term there. That's basically the norm of a_j minus a_j prime squared plus the norm of N squared minus two times the projection of N -- or rather, the inner product of N -- and a_j prime minus a_j.
So this is the probability that the inner product of N and a_j prime minus a_j is greater than or equal to norm of a_j prime minus a_j squared over 2. If you divide by the norm of a_j prime minus a_j, you get the same expression as we had. So it's the same thing. This was done geometrically, this is done algebraically. So this is the expression of the probability of error, and this is Q of the norm of a_j prime minus a_j over 2 sigma.
So now that we have the pairwise error probability, we can use it to bound the probability of error given a_j. Well by definition, the probably of error given a_j is simply the probability of the union of all the possible error events of the a_j goes to a_j prime over all possible j prime, not equal to j. This by the union bound is less than or equal to the summations of the probability that a_j goes to a_j prime. That's just using union bound. And now I can sub that expression over from there. This is the same as..So the summation is over j prime not equal to j times Q of the norm of a_j prime minus a_j over 2 sigma.
Now let me write the summation in a different way. I'm going to write the summation over all possible distances which belong to the set of distance, times K_D of a_j, times Q of D over 2 sigma. Where the set D is the set of all possible distances from a_j. Ok? And K_D of a_j is the number of points at distance D from a_j. That's just a straightforward change of variables.
Now if you look at this expression, then Q of B over 2 sigma basically behaves like an exponential, for an algebra use of the argument. So recall that Q of X is like half E to the minus X squared over 2, for X much larger than 1. So what you are really seeing here is that you have a sum of a bunch of exponentials, each written by this term, K_D of a_j.
Now if you think about the argument being large, then when you have a sum of exponentials, the term with the smallest exponent will dominate, because they are all decreasing exponentials. So this term can be written as approximately K_min of a_j times Q of d_min over 2 sigma. So what I am doing is I'm only picking up one term from this summation.
So far, we have a strict upper bound here, so this summation is a strict upper bound on the probability of error given a_j, But now what I am doing is I'm only going to keep one term in the summation, the term which has the smallest exponent here. So I'm looking at the smallest value of D in this set of possible distances from a_j.
AUDIENCE: So you're just looking at the nearest neighbor.
PROFESSOR: You're looking at essentially the nearest neighbor, geometrically speaking. And this approximation actually works quite well in practice. It's not a bound on the probability of error given a_j, but it's an approximation. And why did I do this? Well, if I want to look at the probability over all error, what's that going to be? It's going to be the average over all possible a_j's of probability of error given a_j.
Now, so I want to take an average of this quantity. So this is a constant. So I will just take the average over this, and that's going to be K_min of the constellation, which is the average number of nearest neighbors, times Q of D_min over 2 sigma. This is approximate here. So this is an approximation that will be used, and it's a very useful approximation, and it is known as the Union Bound Estimate.
It's no longer a bound on the probability of error, it's an estimate. And in fact, there is a homework problem where you will be showing that the Union Bound Estimate is in fact exact for an M-PAM constellation. And I will let you think why that is the case. I was going to do it, but then I realized it's a homework problem, so you might as well spend some time on it.
So the last thing that I wanted to do today is find a lower bound on the probability of error. So if I look at probability of error, it's a union of bunch of the events.
AUDIENCE: [INAUDIBLE]
PROFESSOR: Yes.
AUDIENCE: [INAUDIBLE] That union should be with a_j prime.
PROFESSOR: The union should be with a_j -- yeah. It's not what I have? So I'm taking a union over all possible events, but a_j's confused with a_j prime.
AUDIENCE: [INAUDIBLE] a_j going to union j prime not equal to j, a_j prime.
PROFESSOR: Oh, I see.
AUDIENCE: I think you need parentheses around the --
AUDIENCE: Brackets around the --
AUDIENCE: [INAUDIBLE] another set of parentheses behind the event a_j going to a_j prime. Because that's the event you were talking about there. At least that's [INAUDIBLE]
PROFESSOR: So you are saying that --
AUDIENCE: Put parentheses after the u.
PROFESSOR: After the u. Like this?
AUDIENCE: Yeah, right. That's the event.
PROFESSOR: Right. That's what I meant. OK, fine. Fair enough.
OK, so basically, the lower bound is actually quite simple. All I'm going to do is only take one event from that union. I'm only going to take one point, which is the minimum distance from a_j. So probability of error given a_j is greater than or equal to probability that a_j goes to a_j prime, where now a_j prime is the nearest neighbor of a_j. And this we know from PAM analysis is simply Q of d_min over 2 sigma.
So this is a strict lower bound on the probability of error, and it has the same exponent as the Union Bound Estimate. Of course, if I want to find the overall probability of error, I can just take an average of this. Since this is fixed, it's going to be the same quantity. So far what we have is a strict upper bound on the probability of error, which is this quantity here, a union bound estimate, and we have a lower bound on the probability of error.
In the next lecture, we will be looking at how to use these bounds to compute a probability of error for small signal constellations, and quantify the performance trade-off of the probability of error versus the EbN_0 and so on. I think this is a natural point to stop. It's almost time now.
Free Downloads
Video
- iTunes U (MP4 - 156MB)
- Internet Archive (MP4 - 156MB)
Subtitle
- English - US (SRT)