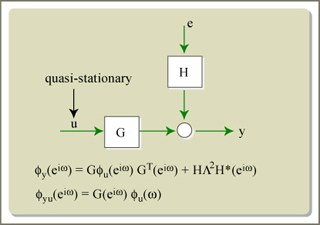
A model for noisy outputs taken from the course lecture notes. From this model, you can derive very important relations in system identification. (Figure by MIT OpenCourseWare.)
Instructor(s)
Prof. Munther Dahleh
MIT Course Number
6.435
As Taught In
Spring 2005
Level
Graduate
Course Description
Course Features
Course Highlights
This course features a complete set of lecture notes. The course also features homework assignments with solutions.
Course Description
This course is offered to graduates and includes topics such as mathematical models of systems from observations of their behavior; time series, state-space, and input-output models; model structures, parametrization, and identifiability; non-parametric methods; prediction error methods for parameter estimation, convergence, consistency, and asymptotic distribution; relations to maximum likelihood estimation; recursive estimation; relation to Kalman filters; structure determination; order estimation; Akaike criterion; bounded but unknown noise model; and robustness and practical issues.