Flash and JavaScript are required for this feature.
Download the video from iTunes U or the Internet Archive.
Description: After reviewing steady-state, this lecture discusses reversibility for Markov processes and for tandem M/M/1 queues. Random walks and their applications are then introduced.
Instructor: Prof. Robert Gallager
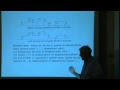
Lecture 20: Markov Processe...
The following content is provided under a Creative Commons license. Your support will help MIT OpenCourseWare continue to offer high quality educational resources for free. To make a donation or view additional materials from hundreds of MIT courses, visit MIT OpenCourseWare at ocw.mit.edu.
PROFESSOR: I want to review a little bit the things we did last time, and carry them just a little bit further, in some cases. Remember, we're talking about the Markov processes now. We defined a countable state Markov process in terms of an embedded Markov chain and in each state an embedded Markov chain.
There's a parameter nu sub i, which is the rate of an exponential process for leaving that state. And that describes what the Markov process does because knowing what the Markov chain does and knowing what these exponential random variables do that gives you everything you need, if you will, to simulate this process. It gives you, in principle, everything you need to calculate everything you want to know about what's going on in the process.
So if you remember, we found out that the-- we essentially defined steady state probabilities for the process in terms of the steady state probabilities for the Markov chain. And what we were doing there is to first, restrict ourselves to the case where the Markov chain is positive-- well, at least, is recurrent. But let's imagine this positive recurrence so we don't have to worry about those distinctions for the time being.
If it's positive recurrent there's a set of pi sub j, which gives the steady state probability that a transition, over the long term, is going to go into state j. If you look at the process overall time, if you look at a sample path of it, with probability one pi sub j, is a fraction of transitions that go into state j. Since when you're in state j, the expected amount of time that you stay in state j is 1 over nu sub j. Nu sub j is the rate of an exponential random variable so the expected value of that random variable is 1 over nu sub j, so the fraction of time that you should be in state j should be proportional to pi j over nu sub j.
But since these fractions have to add up to 1, we normalize it by dividing by the sum of pi k over-- pi sub k over nu sub k. That's the formula that we into it. We showed last time that this formula made sense. What we showed is that if you look at the-- What's that peculiar thing doing there? If you look at the rate at which transitions are occurring in this Markov chain, which is determined by everything going on, but this rate at which transitions are occurring, assuming we start in state i is equal to 1 over the sum over k of pi sub k over nu sub k.
Namely, it's the same denominator over here, and you can interpret this in the same way as you interpret this. This is independent of the state that you start in. These probabilities here, these fractions of time in each state, are independent of where you start. So Mi of t is a sample path, average rate at which transitions occur with probability 1. Namely, no matter where you start, and no matter what sample path you're looking at with probability one, what you wind up with is a number of transitions up to time t divided by t, which goes to a limit of probability one, and it's independent of the starting state.
OK, so if this sum here is infinite then something peculiar is happening because when this sum is infinite, even though the pi sub j's are positive, even though the embedded Markov chain is positive recurrent, these fractions of time in each state don't make any sense anymore. So that in fact, what you have is a Markov process which is getting slower and slower and slower.
That's what this equals infinity means. It means that these pi sub k's, the probability of being in certain states with very high-- If the probability is being high of states where you spend a long time in those states and that sum adds up to infinity, this transition rate is equal to zero. It means that the transitions rates are getting slower and slower and slower, we showed an example of that of an mm1q.
Well, it wasn't quite mm1, but it was like an mm1q, but it had the property that as you moved up to higher and higher states, both arrivals got slower and slower, and also the service time got slower and slower. We called this a rattled server, and also discouraged arrival. So with the two of those things, as you move up to higher and higher states, what happens is transitions get slower and slower and eventually, you settle down in a situation where nothing is getting done. And that's because of the sum here. It's not because of anything peculiar in any one given state.
Let's see, where we're we? OK, if this is equal to infinity, the transition rate goes to 0 and the process has no meaningful steady state. Otherwise, the steady state uniquely satisfies this equation. That's what we showed less time. This says that the rate in equals the rate out for each state, that's what this equation says.
This quantity over here p sub j is the probability that you're in state j, at any given time, nu sub j is expected amount of time you stay in there. So this is the, in a sense, this product here corresponds to the rate in state j. The sum over here corresponds to the rate at which you're going into state j. All of these P's are greater than 0, and the sum of the P's are equal to 1. This is the case though we normally call a positive recurrent process. I notice I didn't define that in the notes. I now notice, thinking about it even more, that I'm not sure I want to define it that way, but anyway that's what it's usually called. It's called a positive recurrent process. It requires both that this is less than infinity and the steady state probabilities pi sub j have a solution.
If you have a birth/death process, you can deal with the birth/death process in exactly the same way as we dealt with birth/death Markov chains. Namely, what goes up must eventually come down. And what that means is a P sub j, this is the fraction of time you're in state j. This is the rate at which you move up, so this combination here is the overall rate at which you're moving up, not conditional on being in state j. This is the overall probability of moving down. So the transition rate up, in any given state, equals the transition rate down. OK, so that's a useful equation. That's the way you usually solve for birth/death processes. The same as you use for solving for change, you can get it from the chain equation very easily.
If you have an irreducible process, an irreducible process is one where every state communicates with every other state, remember? Same as the definition for change. If there is a solution to these equations here, mainly, these are the equations where you directly solved for the average time in each state. The time spent in blah, blah, blah--
What this is saying again, is the rate at which you leave state j is what this is, is equal to the rate at which you enter state j, which is what this is. So this again, is saying what comes in must go out. And if this equation is satisfied, then the embedded chain is positive recurrent and instead of solving for the P's from the pi's, you're solving for the pi's from the P. It's the same kind of equation. Notice before P sub j was equal to pi sub j over nu sub j normalized. Now, pi sub j is equal to a P sub j, nu sub j normalized again. And you normalize this because the rate at which transitions are taking place is normally not 1.
We also found that this rate at which transitions are taking place, 1 over this quantity, is also equal to this sum here. So the sum of Pj nu sub j is the overall rate at which transitions are taking place. Now, if the sum of nu i times p sub i is infinite then, according to this formula, each pi sub j is equal to 0, which says that the embedded Markov chain has to be either transient or is null-recurrent, and something very, very strange is going on. Because here we've solved these equations, we found positive P sub j's, positive probabilities of being in each state or, at least, that's the way we try to interpret it. And then we find that as far as the transition rates are concerned all of the transition rates are equal to 0. So what's going on?
I must admit frankly, I don't know what's going on. Because, I mean, I know mathematically what's going on. This is what the equations say. There's a nice example of it, which I'll look at in the next slide, but to interpret this in any very satisfactory way seems to be very hard. OK, here's the example, and we talked about this a little bit last time.
If we look at the embedded chain, we can call this a hyperactive birth/death chain. It's hyperactive in the sense that the higher the state gets the faster the thing runs. So as you get more customers into this queue, you could imagine then the server runs faster, the customers arrive faster. And as it builds up, this keeps on going faster and faster and faster.
For this chain, it looks like it's stable, doesn't it? Because the probability of going up-- No, it looks like it's not stable. The probability of going up is 0.6. The probability of going down this 0.4. We have that in each state. This is what we've seen often for mm1q's, where things come in at a faster rate than they go out, and the only thing that could happen is the queue builds up. Namely, you start out down here, and you keep moving up and you move up forever, so something bazaar is happening.
But then, we solve these equations for the steady state fraction of time we spend in each state, and what do we get? Because this is the probability of going up, and this is the probability of going down from state 1. And the rate at which things happen from state 1 is 2. And the rate at which things happen in state 2 is 4, what's going on? Since the rate at which things happen here is 4, this rate of going down is 2 times 1.6. Here we are in--
If we're in state 1 and moving up, the rate at which transitions occur in state 1 is 2. The probability that the transition is up is 0.6, so the rate of an upper transition is 0.6 times 2, 1.2. If we're in this state, higher rate of transitions there twice as high, you're moving down with probability 0.4, but since the rate is twice as high, the overall rate at which things are going down is 1.6. This looks like a stable mm1q. OK, bizarre.
We can solve this. We can solve this with the formula for solving for the average time in each state. And what do we get using p sub j times q sub j, j plus 1. p sub j is the fraction of time in state j. q sub j, j plus 1, is the rate at which we move out of that state. That's equal to the fraction of time or in state j plus 1 times the rate at which we're moving down from j plus 1 to j, if these equations make any sense.
But at any rate, we can solve these equations p sub j plus 1 has to equal 3/4 p sub j. That ratio there is 3/4. And the average time in each state, according to these equations, which we can see there's something funny about it, but it's 1/4 times 3/4 to the j because of this relationship here. But the sum of p sub j times nu sub j is equal to infinity, which is why we can't get any steady state embedded chain probabilities.
OK, well, in an effort to understand this, what you can do is you can truncate this as mm1 Markov process to just k states. And it's very easy to truncate the Markov process because all you have to do is just cut off all the transition rates that go into these higher rate states. When you cut off everything going beyond here, what you get is just this Markov process shown here. Well, Markov chain shown here, Markov process shown here, and, at this point, we have a finite number of states. Nothing funny can happen.
And what we get if we actually go through all the calculations is that the fraction of time in state j is 1/4 times 1 minus 3/4 to the k. This term goes to 0 with k, so this whole term here is unimportant. Times 3/4 to the j. This 1/4 times 3/4 to the j was the result we had here when we looked at all the states there. Pi sub j, looking at this chain here, which is an unstable chain, you go up with higher probability than you go down. What you get is pi sub j is 1/3 times 1 minus 2/3 to the k, this term is going to 0 as k increases, times 2/3 to the k minus j.
In other words, when you truncate this change here, which is unstable, what's going to happen is that you're going to tend to stay in the higher states. And you're going to dribble down to the lower states with very little probability in the lower states. As you increase k and increase the number of states that you're dealing with what's happening is that you're increasingly spending most of your time and these higher ordered states. So as you increase k, you just move up one on which states are most likely.
So you get this kind of result here 2/3 to the k minus j, which is decreasing rapidly with k. Which says, as k goes to infinity, this goes to 0 for all j, and this goes to a sensible quantity for each j. When you sum up p sub j times nu sub j, this is giving you the rate at which transitions occur, what you get is this term, which doesn't amount to anything times 1/2 times 3/2 to the k minus 1. This term here is approaching infinity exponentially, which says that something doesn't make any sense here.
What's happening in this queue is that the rate at which transitions occur is going to infinity. If you start out at state 0, very rapidly the state builds up. The higher the state goes up, the faster the transitions become. So the transition rate is approaching infinity, and this solution here, which looks like it makes perfect sense, doesn't make any sense at all. Because in fact, what's happening is the transition rate is increasing as time goes on, whether the number of transitions in a finite time is infinite are not with probability 1. I don't know.
I can't figure out how to solve that problem. If I figure out how to do it, I will let you know. But anyway, the point is, for this embedded chain in this same process, the idea of steady state is totally meaningless. So the caution there is as you deal with Markov processes more and more, and any time you deal with killing a great deal, you deal with this kind of process all the time. What you normally do then is you start solving for these probabilities.
You simulate something, you figure out what these probabilities are from the simulation, everything looks fine until you look at the embedded chain. And then when you look at the embedded chain, you realize that this is all nonsense. I wish I could say more about this but I can't. But anyway, it's a note of caution when you're dealing with Markov processes. Check what the embedded chain is doing because it might not be doing something very nice.
Let's go onto reversibility for Markov processes. We talked about reversibility for Markov chains, sort of half understood what was going on there. Fortunately, for Markov processes, I think it's a little easier to see what's going on than it was for Markov chains. So if you almost understand reversibility for Markov chains then I'll be easy to get the extra things that you need here.
For any Markov chain in steady state, the backward transition probabilities were defined as pi sub i times Pi(j) star is equal to pi j times P(j)i star. In other words, the transition from i to j, the probability of being in state i and going to state j, which is this expression right here. You can write it in two different ways. And there's nothing magical or sophisticated here.
It's the probability that Xn plus 1 is equal to i times the probability that Xn is equal to j, given that the next state is equal to i. We can do that. There's nothing wrong with talking about the probability that the state now as j, given that the state one time from now, the state i. And that's also equal to the probability that Xn is equal to j times the probability. You go from j to i. This is just base law written in a particularly simple form.
This also holds for the embed chain of a Markov process. So to draw a picture for this, you're sitting here in state i, eventually, at some time t1, there's a transition. You go to state j. In state j, there's a transition rate nu sub j, so after some time, whose expected value is 1 over nu sub j at time t2, you go to another state. That new state say is state k, so you start out in state i, you go to state j, you stick there for a time, nu sub j, and then you go on to state k.
OK, so if we look at this picture again, and we look at it in terms of the sample time Markov chain, what's going on? If you're moving right, in other words, moving up in time. And I again, urge you if you have trouble thinking of time running backwards, think of left and right, because you will have no trouble thinking of things going to the left and things going to the right.
So moving to the right, which is the normal way to move after entering state j, the exit rate is nu sub j. In other words, we exit in each delta with a probability nu sub j times delta. The same holds moving left. In other words, if you're at time t2, if moving this way you move into state j, what happens? You leave state j going leftward in each delta unit time with-- There's this same rate here, which is the rate when you look at it either way, is this rate here at which you leave this state j, which is delta nu sub j, and all we're using here is the memorylessness of the exponential distribution. That's the only thing going on here.
So Poisson process is clearly reversible from the incremental definition, and that's what we're using here. And that what this means is that the steady state probabilities, the pi sub i's, and also, the nu sub i's, which are the rates at which transitions occur, are the same going left as going right. So this is the same kind of thing we had before, but I think now, you can see it more easily because there's a finite amount of time that you're sticking in state j, and it's a random amount of time. And moving in one direction, you're moving out of state j with this constant rate delta, delta times nu j. When you're going the other way, it's the same probability that you're going out, going backwards in time, over a period delta of delta times nu sub j. It's the same both ways. And I think this is easier to see than the thing we did before.
OK, so the probability of having a right transition from j to k in a little period of time as p sub j, fraction of time in state j, times q sub jk transition rating k times delta. Similarly, if q star sub kj is the left going process transition rate, the probability of having the same transition as pk times q star sub kj, thus we have this equation. And this equation, turns into this. The rate going backwards is going from k to j is equal to nu sub k times the rate for the embedding Markov chain going backwards.
So we define a Markov process as being reversible if q sub i j star, if the backward rate is equal to the forward rate for all i and j. And if we assume positive recurrence and we assume that pi sub i over nu sub i is less than infinity, which is the stability equation, which says that the rate of transitions has to be finite, then the Markov process is reversible, if and only if, the embedded chain is.
OK, so this gives you a nice easy condition to talk about reversibility. You can either show that the chain is reversible or the process is reversible. They both work the same way. And if you understand chains then you can use that to understand processes. If you understand processes, you can use that to understand chains.
OK, so from that we get what I like to call the guessing theorem. Suppose a Markov process is irreducible, this means every state communicates with every other state, it's easy to verify irreducibility. It's sometimes hard to verify whether a chain is positive recurrent or not and whether the process is positive recurrent or not.
So this guessing theorem says if a Markov process is irreducible and if p sub i is a set of probabilities that satisfy this equation here-- these are the reversibility equations-- p sub i times qij, rate at which you're going up is equal to the rate at which you're going down for all i and j. And when you find that p sub i that satisfies these equations here, if it also satisfies this equation, then, in fact, you're in business. And what the theorem says is first, all of these average probabilities are greater than zero for all i, 2, these average probabilities, this p sub i, is the sample-path fraction of time in state 1 with probability 1, 3, the process is reversible, and 4, the embedded chain is positive recurrent.
You get, all at once-- all you've got to do is find the solution to those equations. If you can find the solution to those equations and it satisfies this, then you're done. You don't need a birth/death chain or anything else. All you need to do is guess a solution to those equations. If you get one, then that establishes everything you need.
OK, useful application. All birth/death processes which satisfy this finite number of transition conditions are reversible. Remember, we talked about trees with the Markov condition. If you have a Markov graph which is a tree, and it satisfies this condition, then that process has to be reversible. You get it from this theorem also because, again, you have this condition that what goes over a branch in a tree, you can only go up on that branch one more time, then you can go down. So you've got the same equality and the argument is exactly the same. OK.
So what do we get out of this? What we get is Burke's theorem. And what this Burke's theorem says-- Burke's theorem here, for processes, is what you usually think of as Burke's theorem. It was the original statement of Burke's Theorem before people started to look at sample time chains. And what it says is, given an M/M/1 queue in steady-state with arrival rate lambda less than mu-- if you look at that condition, that's exactly what you need for the stability condition we've been talking about-- the departure process is Poisson with rate lambda.
Now you would think the process-- and when we're thinking about Markov chains, you all thought when you saw this that the departure process had rate mu because the server was operating at rate and mu, who said no, that's not right. Because the service rate is mu anytime the server is active. But is lambda is less than mu the server is not always active. The server is sometimes idle and therefore, what comes in is the same as what goes out. The only possibility is that this queue is gradually building up over time, and then what goes out is less than what comes in.
But otherwise, what comes in is what goes out. And the rate at which the server is working is not mu, that the server is working a rate lambda. Mainly, the server is working at rate mu when it has things to do but every time it doesn't have things to do it takes a coffee break. It's not doing anything.
And at that point, when you amortized over the time the server is taking coffee breaks and the time the server is working the rate is lambda because that's the rate at which things are coming in. You can only get one thing out for each thing in. So either the queue builds up forever, in which case, what's going out is less than what's coming in or the queue is not building up and what goes out is exactly what's coming in. I hope looking at it that way convinces you that what's coming out here is the same rate as what's going in.
OK, the next thing is that the state x of t is independent of the departures before t. This was the same as the condition we had when we were looking at Markov chains. The state x of t is independent of the departure before t. Whether you look at this thing forward or backward, the state is just the height of that graph there.
So when you're looking at it backwards, the state that you go through over the process is the same as the set of states when you're looking at it the other way. It's just that you have to flip it around. And it says that the state is independent of departures before t. The reason for that is that when you look at the backward process, every departure here is an arrival here. These arrivals here-- This is an arrival, this is an arrival, this is a third arrival. This is the fourth arrival and those depart later. When you're looking at it that way, what's going on?
These things that are called departures up in the top graph are called arrivals when you're looking at it going this way like. What this is saying is-- when you're looking at this as an M/M/1 process, what it's saying is for the backward M/M/1 process, the arrivals that come in later than me do not affect my service time. This is because we have first come first serve service, and if somebody with an enormous service requirement comes after me-- If I'm at the supermarket and somebody comes in with three big barrels of food, I breath a sigh of relief because I'm there first. And I get out quickly, and I don't have to wait for this person. And that's essentially, what this is saying.
OK, the third one for first come first serve service, a customer's arrival time, given that it departs at time t, is independent of the departures before time t. That's almost the same as two, but it's not quite the same as two. It only applies if you have first come first serve service. This is not quite true for Markov chains. You remember when we stated Burke's theorem for Markov chains. We only had these first two statements, and now we have this third statement.
Let me try to explain in the next slide why that's true. If I can explain this to you and make you believe it on the first time I feel very proud of myself. Because this is the kind of thing you have to have explained to you three times. You have to sit down and think about it. The first three times you think about it, you say, that's all baloney. The fourth time you think about it, you say, that's false. And maybe the fifth time you say, it's false again. And the sixth time you look at it just the right way, and it turns out to be true. And then you go back a week later, and it's false again.
But anyway, let me try. We're going to first look at the right moving sample path, OK? So departure at t up here is an arrival [? in ?] the departure in the right moving sample path is an arrival in the M/M/1 left moving sample path. For first come first service, looking at the left moving process-- that's the left moving process-- the departure time of an arrival at time t-- here's an arrival at time t-- the departure of that arrival, in this case, is that departure right there.
That departure time depends on the arrivals and the service requirements back over here because when we're looking at things going this way, this is what happens first. This is what happens later. So we have these arrivals coming in this way, this arrival here has nothing to do-- Well, this arrival here, in general, does have something to do with this arrival, because if this arrival were not finished by this time, we would have to wait for it. The waiting time of this arrival here depends on what happened before, which is over here. It does not depend on the arrivals after it because it's first come first serve service. And therefore, anything that comes after is sitting behind us. It doesn't bother us at all.
OK, for the corresponding right moving process then coming back the other way, the arrival time of that departure is independent as the departures before t, and that's exactly what this theorem is saying. In order to make sense of this you have to do something that we've been doing all along. We don't always talk about is very much, but I hope you're getting used to doing it. You talk about sample paths. When you're talking about sample paths, you're talking about one particular instantiation of the process.
You prove a result for that sample path, then you say, that's true for all sample paths. And then, you say, a ha, then it must be true for the random variables of which these sample paths are sample cases. That's an argument we go through so many times. In fact, when you take probability the first time, pretty soon it becomes second nature to you, and every once in while-- In fact it becomes so much second nature, that most people don't even distinguish between random variables and actual sample path numbers. They use the same symbol for both of them. Every once in a while I get confused by this, but eventually, they get it straightened out, and that's part of what this argument is here.
OK, let's test our understanding now. Let's look at tandem M/M/1 queues. First, we don't quite know what that means, but let's consider two queues, one sitting right after the other. Departures from the first queue move directly into the second queue. The partition here moved directly into here. I've shown these departures at rate lambda because what Burke's theorem says, is that these departures are, in fact, a Poisson process, we rate lambda. You think you're through at this point, but you're not. You still have some difficult thinking to go through.
So you're assuming originally, that the input is Poisson. You're assuming that the first queue has exponential services, the service times are independent of each other, they're all iid, and they're independent of the inter-arrival times. So this first thing here is just an M/M/1 queue like you're used to. We now know that what's coming out of that first queue is a Poisson process. It's a little hard to look at as a Poisson process but it is. It is not a Poisson process if you're conditioning on what's going on in the first queue.
It's not a Poisson process conditional on these arrival times here or on these service times here. It's only a Poisson process if we're looking at the departure times unconditional on anything else. Conditional only on the other departure times or iid, That's what we've proven. But conditional on what's going on in the first queues of those departures, are sure as hell not even close to doing iid. I mean, if you've got a lot of arrivals and you've got a lot of long service times, the queue is going to get busy. And what's coming out of that queue is going to be at rate mu, which is what all of you believe until you see this theorem, and think it through. And that's why you believe it, because you look normally, at time moving from left to right.
We're going to assume the service time at rates mu 1 and mu 2 are independent from queue to queue. And they're independent-- Ah, I didn't change that. This doesn't make any sense. The service rates at mu1 and mu2 are independent from this queue to this queue, and they're independent of the inter-arrival times back there. Now, why do I have to change that? Why can't I say that the service times at mu1 and mu2 are independent of both the arrivals to the first queue and the arrivals to the second queue?
Because first, I can't assume things that are not given by the model of the problem. The things going from queue 1 into queue 2, I know they're Poisson now, but I don't know that they're Poisson independent of what's going into the first queue, and independent of the service times in the first queue, and they certainly aren't. So what I have to say here is they're independent from queue to queue and independent of the arrivals at the first queue.
OK, we know that the arrivals at queue 2 are Poisson at rate lambda. That's true by Burke's theorem. And they're independent of the service times at 2. That alone makes the second queue M/M/1. Because the second queue has arrivals. It has service times. The arrivals are independent of the service times. It is, by definition, what an M/M/1 queue is. It might depend on what's going on in the first queue to a certain extent. Burke's theorem tells us, in one sense, it doesn't depend on it, and that's the next thing we have to deal with.
The states of the two systems are independent and the time of a customer in system one is independent of that in state two. I'm not going to try to convince you of that here. The text does, I think, do a pretty good job of convincing you of it. It's a more complicated argument than this. And s something which you just have to either say, I believe it because I'm too tired to do anything else. Or you have to go through it several times, sometimes disbelieving and sometimes believing until finally, saying, I guess it must be true.
OK, so enough of that. Let's go on to random walks, which is where we should have been 45 minutes ago. You see our problem here is that we would like to talk about martingales a little bit in this course because martingales are extremely important things. You can prove all sorts of things from them. An enormous amount of modern research in the field uses martingales, and you can do a great deal with martingales without knowing anything about measure theory.
And almost all the books that talk about martingales use measure theory, and therefore, require you to take a couple of extra terms of math courses before you can understand what's going on. So it's important to be able to say something about them, in this course, so you have enough-- so you know when you have to learn more about them at least. So I want to get to that point, which is why I've been speeding up a little bit from what I would like to do.
If you noticed, if you've been reading the notes, I have skipped the [INAUDIBLE] equations. I have skipped semi-Markov processes. We're not going to deal with them. The [INAUDIBLE] equations are quite important. You use them quite a bit. They play the same role for Markov processes that all the business with eigenvalues we went through play with Markov chains.
The trouble is we don't have time to do it. And the other trouble is all the time that we would spend understanding that would be time understanding linear systems of differential equations. And if you study that in math and you have a good feel for it, fine, you can read the section and notes. If you're not familiar with that, then you have to learn something about it. It's not something we should waste our time on because it's not something we will use at any other time in this course.
OK, so we want to get onto random walks. A random walk is, in fact, a very simple kind of animal. Let x sub i, i greater than or equal to 1, be a sequence of independent identically distributed random variables. And let s sub n be x1 plus x2 plus x sub n for n greater than or equal to 1. Is this something that we spent a 1/4 of the term talking about, or 1/2 the term talking about, or 3/4 of the term talking about? I don't know, but we've certainly spent an awful lot of time talking about the sums of iid random variables.
What is different here is that instead of being interested in these random variables s sub n, what we're interested in is this process of the s sub n's. I mean, we're interested in looking at the whole sequence of them, and saying things about that. What we would like to be able to do is say questions of this sort. You pick some alpha bigger than 0, doesn't matter what it is, 1. What's the probability that s sub n is greater than or equal to alpha for at least one in greater than or equal to 0? So we're not asking, what's the probability that s sub 10 is bigger than alpha. We're asking, if we look at this sequence, s1, s2, s3, s4, what's the probability that any one of those terms cross alpha?
In other words, when we look at the entire sample path, does the entire sample path lie below alpha or does the sample path, at some point, cross alpha, then perhaps, come back down again or continue to go up or do whatever it wants to do. What the question is, does it cross it at least once? The questions connected with that are, if it does cross the threshold, when does it cross the threshold? That's important. If it crosses the threshold, what's the overshoot with which it crosses the threshold? Does it go shooting way above the threshold when it crosses it?
If you have one of these random variables that has very long tails, then it's very likely that when you cross the threshold, you've crossed a threshold because of some sample value which is humongous. And therefore, you typically are going way above the threshold when you cross it. That makes the study of random walks very much more complicated than it would be otherwise. These overshoot problems are extremely difficult, extremely tedious, and unfortunately, very often, very important. So that's a bad combination, to be tedious and important. You would rather avoid things like that.
Next one is given two thresholds for a given alpha bigger than 0, and a given beta less than 0-- here we are, starting out at 0. We have a threshold up here and a threshold down here. And we want to know what's the probability that we ever cross one of the two thresholds. That, I hope, you can answer right away. Can you?
s sub n, after a long period of time, is going to look Gaussian. It's going to look Gaussian with a standard deviation, which is growing gradually. And as a standard deviation grows gradually, and we have these two finite limits, eventually, we're going to cross one of those thresholds. So the question is not do you cross one of the thresholds? The question is, which threshold do you cross? What's the probability of crossing each of them? At what end do you cross the one that you cross? And what's the overshoot when you cross it? So those are the questions there.
These threshold crossing problems are important. They're important in almost everything that you use stochastic processes for. They're familiar to me for studying errors in digital communications systems because that's sort of the basis of all of that study. They're important in studying the overflowing queues. Anytime you build a queue, I mean, what are you building? You're building a waiting room for customers, and you're building a service facility. And one of the first questions is, how big do I have to make the waiting room? How much storage do I need, is the question. So you really want to be able to answer the question, what's the probability that the queue will ever overflow the queue? That's a threshold problem.
In hypothesis testing, when you want to find out which of two hypotheses is true, this is important. When you want to look at, what's often a more important question, is you test for one or two hypotheses, and if you're smart, and if you can do it, you keep the test running until you're pretty sure that you have the right answer. If you've got the right answer right away, then you can stop, and save time from there on. If you have to go for a long time before you get the answer, then you go for a long time before you get the answer. That's called sequential hypothesis testing, and that's important.
There are these questions or ruin and other catastrophes, which, as you've observed in this paper over the last few years, most people don't know how to deal with them. If they do know how to deal with them, they're too affected by short term profits and things like that that do what they should do. But part of the trouble is people don't know how to deal with those problems. And therefore, if you're trying to maximize your profits and you can say, I've talked to 10 experts, they've told me five different things. It gives you a perfectly good excuse for maximizing your profits rather than doing something reasonably sensible or reasonably safe. So these are important problems that we're dealing with.
I'm going to start with a brief discussion of three simple cases. First one is called simple random walks. The next one is called integer random walks, and the third one is called renewal processes. These random walks we're talking about sound like very simple minded things, but if you think about it for a minute, all of these renewal processes we've spent all this time studying and struggling with, are just a special case of random walks.
I mean, a renewal process has non-negative random variables. We're adding up those non-negative random variables to see what's going on. So a renewal process is just dealing with sums of iid non-negative random variables, where this is dealing with a case where the random variables can be either positive or negative. OK, so we're going to start with a little bit about them.
A random walk is called simple if the underlying random variable xn n is the simplest random random variable of all. Mainly, it's binary. The probability that it's 1, that it's equal to 1, and the probability is minus 1 is equal to q. You could make it just 0 or 1, namely, make it Bernoulli, but that makes it too special. I mean, since we've been studying renewal processes, where all these random variables are non-negative, let's take the plunge and study the simplest case of random variables, which can be either positive or negative. The simplest case is this binary case, where the random variables can be 1 or minus 1. That's what a simple random walk is.
OK, it's just a scaling variation on our Bernoulli process though. The probability that xi is equal to 1 for m out of the n trials. Mainly, you're going to flip this coin x sub i, heads is 1, tails is minus 1. The probability that you get heads for m out of the n trials is n factorial over m factorial times n minus m factorial. Number of combinations of n things taken m at a time times p to the m times 1 minus p to the n minus m. We've known that forever. You probably knew in high school. You relearned it when you were taking elementary probability. We've talked about it in this course. We can also view this as a Markov chain.
You start off in state 0 with probability p. You go to state 1 with probability 1 minus p. You go to state minus 1. On the second trial, you either go to state 2 or to 1, 2 or to 0, or to minus 2, and so forth. That's just another way of analyzing the same simple random walk. Just like in the Stop When You're Ahead game that we talked about in class, the probability that we ever cross a threshold at k equal to p over 1 minus p to the k. Let me remind you of why this is true, remind you of something else. I want to find the probability that any s sub n is greater than or equal to k, so I need this union here.
Many people write that as a maximum over n as the probability that the maximum over the s sub n's is greater than or equal to k. Other people write it as the probability that the supremum of this sum is greater than or equal to k. Why do I like to use the union, other than the fact that I like set theory more than algebra? Anybody know what some simple reasons for that are? Yeah?
AUDIENCE: It might not be the max [INAUDIBLE].
PROFESSOR: What?
AUDIENCE: You were just trying to decide whether it crosses-- the sum-- that k right now, whether it-- the maximum implied that was as high as it [INAUDIBLE].
PROFESSOR: Not necessarily, no. I mean, I might not be able to find the maximum. If I'm not dealing with a simple random walk, and I look at the sequence S1, S2, S3, S4, and I ask what's the maximum of that sequence, the maximum might not exist. The supremum always exists if you include infinity as a possible value for it.
But the supremum is not very nice either. Because suppose I had a random walk where it was possible for the random variables to take on arbitrarily small values. And suppose I had a sequence which went crawling up towards 1, as n increases, it keeps climbing up towards 1, but it never quite gets there. What's the supremum of that set of numbers? 1. So the supremum is greater than or equal to 1. But that's not what I'm interested in. I'm interested in the question, what's the probability that any one of these random variables is greater than or equal to 1? So that's the straightforward way to write it.
Now does anybody care about these minor differences of a maximum or a supremum or what have you? No. The only reason you care about it is when you write a maximum, after you become a little bit careful with your mathematics, you say, is that a maximum? Or is it a supremum? So you write it as a supremum and then you go through the argument I just went through. And the point is, you're not interested in that at all. All you're interested in is do you cross the threshold or don't you? And this is the, natural way to write it.
OK. Now the next thing is, how do we get this formula here? Well, if we're going to cross a threshold at k, and k is an integer, there's not going to be any overshoot. We're either going to get there and hit it, and then we might go on beyond there. We'll either hit it on [INAUDIBLE] or we won't hit it. Now if we're going to hit it at [INAUDIBLE], how are we going to do that?
Well we have to hit 1 at some point. Because you can only move up or down by one at a time. So you're going to have to hit 1 at some point. Given that you've hit 1, you have to go on and hit 2 at some point. Given that you've hit 2, you have to move up and hit 3 at some point. Those events are independent of each other. So the probability that you ever get to k is the probability that you can stop when you're ahead. Namely, it's the probability that you go from 0 to 1 up to the k-th power. So I'm saying that p over 1 minus p is the probability you ever move from state 0 up to state 1.
And when we were looking at Stop When You're Ahead, it was exactly this game here. We moved up with probability p, down with probability q. We were looking at the probability we'd ever be ahead in this gambling game where we kept betting money, and we always had an infinite capital. So we could keep on betting forever. And we stopped as soon as we hit 1.
So it's exactly this question here. The way we solved it at that point was to write an equation for it. On the first step, you either move up, and you're there. Or you move down. And if you move down, you have to move up twice. So the probability you ever get from here to here is p plus q times the probability you get from here squared. You solve that quadratic equation. You might remember solving that before. And that's where you get to p over 1 minus p.
OK, so that's not terribly important. What is important is that this is the only problem in random walks I know which has a trivial solution. Most problems have a harder solution than that. But there is at least one problem which is easy. Now for those of you worried about a final exam, final exams have to find problems which are easy. So that tells you something. I mean, we have to take the easy results in this course, and we have to use them in someway. And since I'm telling you that, I probably will include it, so-- OK.
Integer random walks. That's the next kind of random walk to talk about. x is an integer random variable. So similarly, s sub n is an integer random variable. So when we're moving up, we move up in integer values, which makes things a little bit easier. This means, again, that we can model this as a Markov chain.
In the Markov chain, we start at 0, we move up whatever number of values x sub n can have, or we move down whatever number of values it can have. And then from there, we keep moving again. So it's this Markov chain with a very regular structure, where for each state, the set of various states of up transition and set of down transitions are all the same for all the states. So that's a simple Markov chain to deal with.
OK, and then we said that renewal processes are special cases of random walks where x is a positive random variable. When you're sketching sample paths, the axes are usually reversed from random processes to random walks. I'm just pointing this out because every time you try to go from a random walk to a two-way renewal process, you will be happily writing equations and drawing pictures of what you're doing. And you will suddenly come up against this problem, which is that whenever you draw a figure for a renewal process, you draw it this way. OK?
In other words, what you're looking at is the time at which the n-th arrival occurs. Time is going this way. The time at which the first arrival occurs is S1. The time at which the second arrival occurs is S2, S3, and so forth. These intervals here-- x1, x2, x3, x4, x5, and so forth-- whenever you're dealing with a random walk, what you're interested in directly these s's and x's. And you always draw it the opposite way with the axes reversed.
And what's confusing to you at first is that when you see this picture, it doesn't look like this picture at all. These two pictures are identical with the axes reversed. One is the way you draw renewal processes. The other is the way you draw random walks-- which also suggests that sometimes when you're dealing with random walks, you want to draw a picture like the picture you draw for renewal processes and vice versa. So the two are very closely related.
OK, so I want to do a little bit about the queuing delay in a G/G/1 queue. We've talked about G/G/1 queues a little bit in terms of Little's Theorem and various other things. And there's one very simple thing about G/G/1 queues that follows from looking at random walks. And there's a nice part of the problem you can solve dealing with random walks.
Let's let x sub i be the IID random variables, which are inter-arrival intervals for the G/G/1 queue. Here's the first inter-arrival interval. Here's the second. Here's the third. Here's the fourth up here, and so forth. The departure occurs-- there's an inherent arrival that we visualize at time 0. y 0 is a service time that that arrival requires. y1 is the service time that this first arrival requires. y2 is the service time of the third arrival. These service times are all independent of each other. We've looked at this picture before. What we haven't done is to draw the obvious conclusion from it.
If you try to talk about what is the waiting time in queue of the n-th customer, how can we draw that in a figure? Well here it is in this figure. The 0-th customer has no waiting time at all, because there's nothing in the queue. It goes directly into the server. I'm just talking about queueing time, not system time. So 0 goes directly into the server, takes some long service time, ends here. The next arrival occurs at this time here after this inter-arrival time. And what's it have to do? It has to wait until this customer finishes. And then it has to wait for its own service time before it gets out of the system.
The next customer comes in x2 units of time after the first customer comes in. So it comes in here. It has to wait in queue until after the first customer gets finished, and so forth. Now look at the time from the arrival of the second customer until the second customer goes into service. What is that? It can be written in two different ways. And this is the observation that makes it possible to analyze G/G/1 queues. The inter-arrival time of the second customer plus the queueing time of the second customer is equal to the queuing time of the first customer plus the service time of the first customer. OK?
This time is equal to that time. Put little ovals around them so you can spot it. So in general, if the arrival n is queued, then xn plus wn, the inter-arrival time for the n-th customer plus its waiting time in queue is equal to the service time of the previous customer plus the waiting time of the previous customer in queue. If arrival n sees an empty queue, then wn equals 0. OK? This is easy.
If you didn't draw this picture, it would be very hard. If you tried to do this by algebra, you would never get done. But when you draw a picture, there's nothing to it. OK, so that says w sub n is equal to y sub n minus 1 minus xn plus w sub n minus 1 if w sub n minus 1 plus yn minus 1 is greater than or equal to xn, namely, if xn gets queued at all. And otherwise, w sub n is equal to 0. In other words, if the n-th customer enters a queue which is empty, it doesn't wait in queue at all. It goes right into service if that condition is satisfied.
So we can write this whole thing as w sub n is equal to the maximum of w sub n minus 1 plus yn minus 1 minus xn or 0. Namely, what's happening is that the amount of time that each customer has to wait in the queue, not in the system-- waiting time in the queue is either this quantity here that's related to the waiting time of the previous customer added to the service time of the previous customer minus the n arrival time of the customer before.
If you define u sub n as y sub n minus 1 minus plus xn-- y sub n minus 1 minus xn-- namely, the service time of the n minus first customer minus the inter-arrival time for the n-th customer, this is independent over n. So this is a IID random variable. Each of the arrivals x sub n are IID. Each of the departures and each of the service times are IID. Arrivals and services are independent of each other. So this u sub n random variable is a sequence of IID random variables. And what this is saying then, is that w sub n is equal to the maximum of w sub n minus 1 plus un or 0.
If for the time being, ignore that maximum. I mean, suppose the queue is very, very long. The queue is very, very long. You don't have 0. Everybody has some service time. This would be a random walk then. w sub n, the waiting time for the n-th customer, is equal to the waiting time of the n minus first customer plus u sub n. u sub n is playing the role of the inter-arrival in the random walk. That's the x's that we had in the random walk. w sub n minus 1 is the sum. w sub n is equal to the previous sum plus the new random variable coming in. So without the maximum, this is just a random walk. The w sub n's are the random walk based on these peculiar random variables u sub i, which are the peculiar random variables of service times minus inter-arrival times.
OK. With the max, this says w sub n is like a random walk. What does it do? It keeps going up for a while you have a queue. Then it might start dropping. But it can't go negative. Whenever it gets down to 0, it gets down below 0, the next time you start at 0 again. And you start going again. The next time it goes negative, you get bumped up to 0 again. It's like a naughty kid who goes through all sorts of problems, but as soon as he goes negative, somebody picks him up and starts him back up again. So it's a-- well, it's a different kind of process.
But anyway, it's like a random walk, except it has this peculiar characteristic that any time it crosses the 0 threshold, it gets bumped back up, and you start over again. So you have these segments which make it a renewal process also. You have renewals whenever you get to 0 and you start over again. And we'll talk about how those processes work later. The text has another way of looking at it, where you look at this random walk going backwards in time rather than forward in time. But we don't want to spend a lot of time on that. We just want to see this does have something to do with random walks.
I want to spend some real time studying detection and decisions and hypothesis testing, because each one of these things are very important in a number of different fields. If you study radar or any kind of military problem, detection is very important. You want to see whether something has happened or not. And often you have to see whether it's happened in the presence of a great deal of noise or something. So you're not sure of whether it's happened or not. So you make many observations to see whether it's happened. And then you try to make a decision on the basis of all of those things.
Decisions-- that's what control people think about all the time. Control freaks always want to make decisions. They don't want you to make decisions. They want to make the decisions themselves. But that's part of what studying random processes is all about. How do you make sensible decisions?
You generally-- when you make a decision, you make it with some uncertainty connected to it. I mean, people respond to this by pretending there isn't any uncertainty. They pretend when they made a decision that they must be right. But actually, they could be wrong. And sensible people face the fact that they might be wrong. And therefore, they try to analyze what's the probability of being wrong, what's the probability of being right, and all of these things.
Hypothesis testing-- all scientists deal with this. There are competing theories in some field, and in these competing theories, you want to find out which is the right hypothesis. So you do a lot of tests. And after all those tests, you try to decide which hypothesis you believe in. Why is it important to make a choice?
Well it's important to make a choice in all sorts of reasons and all sorts of areas. Even in a scientific area, I mean-- the field has to move somehow. If the field has all sorts of open questions and if everybody says, well, there might be quantum theory, or there might not be quantum theory. It might all be wrong. It might all be right. I don't know. I mean there's noise in the system, we can't tell anything. So all they're dealing with is a set of very complicated probabilities.
Instead of that, people do make decisions. They say, let's proceed on the basis of what they think is the best course of action. You're voting for a candidate for public office. Well you can say, I don't care. But you can't vote for both. You have to choose one or the other. And you have to make a decision, what's the best choice?
So these problems arise everywhere. And if you leave these problems out of the study of random processes, what are you left with? A purely academic exercise. OK? All you're doing is you're establishing probabilities, but you're not using them. So this is sort of where the rubber hits the road, when you study decision making or hypothesis testing or detection, whichever one you want to call it. But you absolutely need to make these hypothesis tests at some point.
We're going to call them hypothesis tests because the language used there seems to be easier to live with than the language in other places. Not that I like the way the statisticians do these things. Statisticians talk about errors of the first kind and errors of the second kind. And I and everyone else I know never knows what's the first kind and what's the second kind? But they never talk about giving names to these things. It's always first kind and seconds kind and so forth.
But we won't bother ourselves about that. What we're going to do is we'll consider only problems where you have two possible decisions. So it's a binary decision problem, binary hypothesis testing problem. We're going to consider a sample space which has a random variable called h in it. The random variable h can have two possible values, 0 or 1. And you're going to have the multiple observations that you're going to make, Y1, Y2, Y3, Y4, up to y sub n, say.
To make the problem easy and to make it correspond to random walks, we're going to assume that the observations are IID conditional on h equals 0, and they're also ID conditional on h equals 1. If you're used to studying noise problems, a nice example of this is you send one of two binary values over some communication channel. There's additive noise added to it. At the receiver, what you see is what you sent plus some additive noise. You try to figure out from that sum of signal plus noise was a 0 sent, or was a 1 sent? And you don't know which was sent, and you have to take a guess.
And usually if the noise is not too big, you can make a very good guess, so you don't make many errors. This is exactly the problem that we're concerned with here. If you have multiple observations, you can think of sending that same binary digit n times. And then on the basis of all of those observations, see what you want to guess.
I mean scientific experiments-- does a scientific theory ever get resolved by one experiment? I mean sometimes you read in textbooks that it does. But it never does. Somebody does an experiment. They come up with some conclusion. And immediately 10 other groups around the world are all doing the same experiment to validate it or not validate it. Maybe they don't all publish papers about it. But pretty soon, that experiment is done so many times with so many variations on it that you can think of having multiple observations of the same thing.
OK. So when we got all done with it, we're going to assume that the observations will have probability density functions. They're analog and the hypothesis is binary. Doesn't make any difference, you can have observations that are discrete also. It's just whether you want to use probability mass functions or probability density functions. It looks a little bit easier to do it this way. So the probability density of having n observations given that the hypothesis l is the correct one is this product here. And that's true for both l equals 1 and l equals 0.
Let me go just a little bit further. Baye's law then says that the probability that h is equal to the hypothesis l is equal to the a priori probability that the alt hypothesis is correct times this density divided by the sum of the two densities. Nothing fancy here at all. If you compare these two probabilities-- probability that 0 is a correct hypothesis with the probability that 1 is the correct hypothesis-- you take the ratio of these. What you get this p0 over p1 times this density divided by that density.
Well this was thought of well over 100 years ago, probably 150 years ago. People fought about it terribly, terrible fights about this. One of the worst fights in science that's ever happened. Then we had Bayesian statisticians and non-Bayesian statisticians. And you know, these people were all perfectly willing to talk about conditional probabilities so long as they were looking forward in time. When they started looking backward in time, namely looking at these conditional probabilities going backwards, and saying, we have hypotheses in our model. These have probabilities.
We will talk about these probabilities of everything involved. We have a complete probabilistic model. We will talk about-- in this probabilistic model, when you get a certain observation, what's the probability the one hypothesis is correct? What's the probability the other hypothesis is correct? People suddenly lost everything they'd learned about probability, and said this can't be right. So there were enormous fights about it.
Anyway, I wanted to get to that point. Think about that. Next time we're going to start out and do a little bit with this. And suddenly a random walk is going to emerge. So we will do that next time.
Markov Processes and Random Walks (PDF)
Free Downloads
Video
- iTunes U (MP4 - 203MB)
- Internet Archive (MP4 - 203MB)
Subtitle
- English - US (SRT)