Flash and JavaScript are required for this feature.
Download the video from iTunes U or the Internet Archive.
Description: Lecture covering parallelism, scheduling theory, the Cilk++ runtime system, and Cilk chess programs.
Speaker: Charles Leiserson
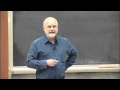
Lecture 13: Parallelism and...
The following content is provided under a Creative Commons license. Your support help MIT OpenCourseWare continue to offer high quality educational resources for free. To make a donation or view additional materials from hundreds of MIT courses, visit MIT OpenCourseWare at ocw.mit.edu.
PROFESSOR: So today, we're going to talk a bit more about parallelism and about how you get performance out of parallel codes. And also, we're going to take a little bit of a tour underneath the Cilk++ runtime system so you can get an idea of what's going on underneath and why it is that when you code stuff, how it is that it gets mapped, scheduled on the processors.
So when people talk about parallelism, one of the first things that often comes up is what's called Amdahl's Law. Gene Amdahl was the architect of the IBM360 computers who then left IBM and formed his own company that made competing machines and he made the following observation about parallel computing, he said-- and I'm paraphrasing here-- half your application is parallel and half is serial. You can't get more than a factor of two speed up, no matter how many processors it runs on.
So if you think about it, if it's half parallel and you managed to make that parallel part run in zero time, still the serial part will be half of the time and you only get a factor of two speedup. You can generalize that to say if some fraction alpha can be run in parallel and the rest must be run serially, the speedup is at most 1 over 1 minus alpha. OK, so this was used in the 1980s in particular to say why it was that parallel computing had no future, because you simply weren't going to be able to get very much speedups from parallel computing. You're going to spend extra hardware on the parallel parts of the system and yet you might be limited in terms of how much parallelism there is in a particular application and you wouldn't get very much speedup. You wouldn't get the bang for the buck, if you will.
So things have changed today that make that not quite the same story. The first thing is that with multicore computers, it is pretty much just as inexpensive to produce a p processor right now, like six processor machine as it is a one processor machine. so it's not like you're actually paying for those extra processing cores. They come for free. Because what else are you're going to use that silicon for?
And the other thing is that we've had a large growth of understanding of problems for which there's ample parallelism, where that amount of time is, in fact, quite small. And the main place these things come from, it turns out, this analysis is kind of a throughput kind of analysis. OK, it says, gee, I only get 50% speedup for that application, but what most people care about in most interactive applications, at least for a client side programming, is response time. And for any problem that you have that has a response time that's too long and its compute intensive, using parallelism to make it so that the response is much zippier is definitely worthwhile. And so this is true, even for things like game programs. So in game programs, they don't have quite a response time problem, they have what's called a time box problem, where you have a certain amount of time-- 13 milliseconds typically-- because you need some slop to make sure that you can go from one frame to another, but about 13 milliseconds to do a rendering of whatever the frame is that the game player is going to see on his computer or her computer.
And so in that time, you want to do as much as you possibly can, and so there's a big opportunity there to take advantage of parallelism in order to do more, have more quality graphics, have better AI, have better physics and all the other components that make up a game engine.
But one of the issues with Amdahl's Law-- and this analysis is a cogent analysis that Amdahl made-- but one of the issues here is that it doesn't really say anything about how fast you can expect your application to run. In other words, this is a nice sort of thing, but who really can decompose their application into the serial part and the part that can be parallel? Well fortunately, there's been a lot of work in the theory of parallel systems to answer this question, and we're going to go over some of that really outstanding research that helps us understand what parallelism is.
So we're going to talk a little bit about what parallelism is and come up with a very specific measure of parallelism, quantify parallelism, OK? We're also going to talk a little bit about scheduling theory and how the Cilk++ runtime system works. And then we're going to have a little chess lesson. So who here plays chess? Nobody plays chess anymore. Who plays Angry Birds? [LAUGHTER] OK. So you don't have to know anything about chess to learn this chess lesson, that's OK.
So we'll start out with what is parallelism? So let's recall first the basics of Cilk++. So here's the example of the lousy Fibonacci that everybody parallelizes because it's good didactically. We have the Cilk spawn statement that says that the child can execute in parallel with the parent caller and the sync that says don't go past this point until all your spawn children have returned. And that's a local sync, that's just a sync for that function. It's not a sync across the whole machine. So some of you may have had experience with open MP barriers, for example, that's a sync across the whole machine. This is not, this is just a local sync for this function saying when I sync, make sure all my children have returned before going past this point.
And just remember also that Cilk keywords grant permission for parallel execution. They don't command parallel execution. OK so we can always execute our code serially if we choose to. Yes?
AUDIENCE: [UNINTELLIGIBLE] Can't this runtime figure that spawning an extra child would be more expensive? Can't it like look at this and be like--
PROFESSOR: We'll go into it. I'll show you how it works later in the lecture. I'll show you how it works and then we can talk about what knobs you have to tune, OK?
So it's helpful to have an execution model for something like this. And so we're going to look at an abstract execution model, which is basically asking what does the instruction trace look like for this program? So normally when you execute a program, you can imagine one instruction executing after the other. And if it's a serial program, all those instructions essentially form a long chain. Well there's a similar thing for parallel computers, which is that instead of a chain as you'll see, it gets bushier and it's going to be a directed acyclic graph.
So let's take a look at how we do this. So we'll the example of fib of four. So what we're going to do is start out here with a rectangle here that I want you think about as sort of a function call activation record. So it's a record on a stack. It's got variables associated with it. The only variable I'm going to keep track of is n, so that's what the four is there. OK, so we're going to do fib of four. So we've got in this activation frame, we have the variable four and now what I've done is I've color coded the fib function here and into the parts that are all serial. So there's a serial part up to where it spawns, then there's recursively calling the fib and then there's returning. So there's sort of three parts to this function, each of which is, in fact, a chain of serial instruction.
I'm going to collapse those chains into a single circle here that I'm going to call a strand. OK, now what we do is we execute the strand, which corresponds to executing the instructions and advancing the program calendar up until the point we hit this fib of n minus 1. At that point, I basically call fib of n minus 1. So in this case, it's now going to be fib of 3. So that means I create a child and start executing in the child, this prefix part of the function.
However, unlike I were doing an ordinary function call, I would make this call and then this guy would just sit here and wait until this frame was done. But since it's a spawn, what happens is I'm actually going to continue executing in the parent and execute, in fact, the green part. So in this case, evaluating the arguments, etc. Then it's going to spawn here, but this guy, in fact, is going to what it does when it gets here is it evaluates n minus 2, it does a call of fib of n minus 2. So I've indicated that this was a called frame by showing it in a light color. So these are spawn, spawn, call, meanwhile this thing is going.
So at this point, we now have one, two, three things that are operating in parallel at the same time. We keep going on, OK? So this guy that does a spawn and has a continuation, this one does a call, but while he's doing a call, he's waiting for the return so he doesn't start executing the successor. He stalled at the Cilk sink here. And we keep executing and so as you can see, what's happening is we're actually creating a directed acyclic graph of these strands. So here basically, this guy was able to execute because both of the children, one that he had spawned and one that he had called, have returned. And so this fella, therefore, is able then to execute the return. OK, so the addition of x plus y in particular, and then the return to the parent. And so what we end up with is of all these serial chains of instructions that are represented by these strands, all these circles, they're embedded in the call tree like you would have in an ordinary serial execution. You have a call tree that you execute up and down, you walk it like a stack normally. Now, in fact, what we have is embedded in there is the parallel execution which form a DAG, directed acyclic graph.
So when you start thinking in parallel, you have to start thinking about the DAG as your execution model, not a chain of instructions. And the nice thing about this particular execution model we're going to be looking at is nowhere did I say how many processors we were running on. This is a processor oblivious model. It doesn't know how many processors you're running on. We simply in the execution model, are thinking about abstractly what can run in parallel, not what actually does run in parallel in an execution.
So any questions about this execution model? OK. So just so that we have some terminology, so the parallel instruction stream is a DAG with vertices and edges. Each vertex is a strand, OK? Which is a sequence of instructions not containing a call spawn sync, a return or thrown exception, if you're doing exceptions. We're not going to really talk about exceptions much. So they are supported in the software that we'll be using, but for most part, we're not going to have to worry about them.
OK so there's an initial strand where you start, and a final strand where you end. Then each edge is a spawn or a call or return or what's called a continue edge or a continuation edge, which goes from the parent, when a parent spawns something to the next instruction after the spawn. So we can classify the edges in that fashion. And I've only explain this for spawm and sync, as you recall from last time, we also talked about Cilk four. It turns out Cilk four is converted to spawns and syncs using a recursive divide and conquer approach. We'll talk about that next time on Thursday. So we'll talk more about Cilk four and how it's implemented and the implications of how loop parallelism works.
So at the fundamental level, the runtime system is only concerned about spawns and syncs. Now given that we have a DAG, so I've taken away the call tree and just left the strands of a computation. It's actually not the same as the computation we saw before. We would like to understand, is this a good parallel program or not? Based on if I understand the logical parallelism that I've exposed. So how much parallelism do you think is in here? Give me a number. How many processors does it make sense to run this on? Five? That's as parallel as it gets. Let's take a look. We're going to do an analysis. At the end of it, we'll know what the answer is.
So for that, let tp be the execution time on p processors for this particular program. It turns but there are two measures that are really important. The first is called the work. OK, so of course, we know that real machines have caches, etc. Let's forget all of that. Just very simple algorithmic model where every strand, let's say, costs us unit time as opposed to in practice, they may be many instructions and so forth. We can take that into account. Let's take that into account separately.
So T1 is the work. It's the time it if I had to execute it on one processor, I've got to do all the work that's in here. So what's the work of this particular computation? I think it's 18, right? Yeah, 18. So T1 is the work. So even though I'm executing a parallel, I could it execute it serially and then T1 is the amount of work it would take.
The other measure is called the span, and sometimes called critical path length or computational depth. And it corresponds to the longest path of dependencies in the DAG. We call it T infinity because even if you had an infinite number of processors, you still can't do this one until you finish that one. You can't do this one until you finish that one, can't do this one till you've finished that one and so forth. So even with an infinite number of processors, I still wouldn't go faster than the span. So that's why we denote by T infinity.
So these are the two important measures. Now what we're really interested in is Tp for a given p. As you'll see, we actually can get some bounds on the performance on p processors just by looking at the work, the span and the number of processors we're executing on. So the first bound is the following, it's called the Work Law. The Work Law says that the time on p processors is at least the time on one processor divided by p. So why does that Work Law make sense? What's that saying? Sorry?
AUDIENCE: Like work is conserved sort of? I mean, you have to do the same amount of work.
PROFESSOR: You have to do the same amount of work, so on every time step, you can get p pieces of work done. So if you're running for fewer than T1 over p steps, you've done less than T1 work over and time Tp. So you won't have done all the work if you run for less than this. So the time must be at least Tp, time Tp must be at least T1 over p. You only get to do p work on one step. Is that pretty clear?
The second one should be even clearer, the Span Law. On p processors, you're not going to go faster than if you had an infinite number of processors because the infinite processor could always use fewer processors if it's scheduled. Once again, this is a very simple model. We're not taking into account scheduling, we're not taking into account overheads or whatever, just a simple conceptual model for understanding parallelism.
So any questions about these two laws? There's going to be a couple of formulas in this lecture today that you should write down and play with. So these two, they may seem simple, but these are hugely important formulas. So you should know that Tp is at least T1 over p, that's the Work Law and that Tp is at least T infinity. Those are bounds on how fast you could execute. Do I have a question in that back there?
OK so let's see what happens to work in span in terms of how we can understand our programs and decompose them. So suppose that I have a computation A followed by computation B and I connect them in series. What happens to the work? How does the work of all this whole thing correspond to the work of A and the work of B? What's that?
AUDIENCE: [UNINTELLIGIBLE]
PROFESSOR: Yeah, add them together. You get T1 of A plus T1 of B. Take the work of this and the work of this. OK, that's pretty easy. What about the span? So the span is the longest path of dependencies. What happens to the span when I connect two things in a series? Yeah, it just sums as well because I take whatever the longest path is from here to here and then the longest one from here to here, it just adds.
But now let's look at parallel composition, So now suppose that I can execute these two things in parallel. What happens to the work? It just adds, just as before. The work always adds. The work is easy because it's additive. What happens to the span? What's that?
AUDIENCE: [UNINTELLIGIBLE]
PROFESSOR: It's the max of the spans. Right, so whatever is the longest, whichever one of these ones has a longer span, that's going to be the span of the total. Does that give you some Intuition So we're going to see when we analyze the spans of things that in fact, we're going to see maxes occurring all over the place.
So speedup is defined to be T1 over Tp. So speedup is how much faster am I on p processors than I am on one processor? Pretty easy. So if T1 over Tp is equal to p, we say we have perfect linear speedup, or linear speedup. That's good, right? Because if I put on use p processors, I'd like to have things go p times faster. OK, that would be the ideal world. If T1 over Tp, which is the speedup, is greater than p, that says we have super linear speedup. And in our model, we don't get that because of the work law. Because the work law says Tp is greater than or equal to T1 over p and just do a little algebra here, you get T1 over Tp must be less than or equal to p.
So you can't get super linear speedup. In practice, there are situations where you can get super linear speedup due to caching effects and a variety of things. We'll talk about some of those things. But in this simple model, we don't get that kind of behavior. And of course, the case I left out is the common case, which is the T1 over Tp is less than p, and that's very common people write code which doesn't give them linear speedup. We're mostly interested in getting linear speedup here. That's our goal. So that we're getting the most bang for the buck out of the processors we're using.
OK, parallelism. So we're finally to the point where I can talk about parallelism and give a quantitative definition of parallelism. So the Span Law says that Tp is at least T infinity, right? The time on p processors is at least the time on an infinite number of processors. So the maximum possible speedup, that's T1 over Tp, given T1 and T infinity is T1 over T infinity. And we call that the parallelism. It's the maximum amount of speedup we could possibly attain. So we have the speedup and the speedup by the Span Law that says this is the maximum amount we can get, we could also view it as if I look along the critical path of the computation. It's sort of what's the average amount of work at every level. The work, the total amount of stuff here divided by that length there that sort of tells us the width, what's the average amount of stuff that's going on in every step.
So for this example, what is the-- I forgot to put this on my slide-- what is the parallelism of this particular DAG here? Two, right? So the span has length nine-- this is assuming everything was unit time-- obviously in reality, when you have more instructions, you in fact would make it be whatever the length of this was in terms of number of instructions or what have you, of execution time of all these things. So this is length 9, there's 18 things here, parallelism is 2. So we can quantify parallelism precisely. We'll see why it's important to quantify it.
So that the maximum speedup we're going to get when we run this application. Here's another example we did before. Fib of four. So let's assume again that each strand takes unit time to execute. So what is the work in this particular computation? Assume every strand takes unit time to execute, which of course it doesn't, but-- anybody care to hazard a guess? 17, yeah, because there's four nodes here that have 3 plus 5. So 3 times 4 plus 5 is 17. So the work is 17.
OK, what's the span? This one's tricky. Too bad it's not a little bit more focused. What the span?
AUDIENCE: 8.
PROFESSOR: 8, that's correct. Who got 7? Yeah, so I got 7 when I did this and then I looked harder and it was 8. It's 8, so here it is. Here's the span. There is goes. Ooh that little sidestep there, that's what makes it 8.
OK so basically, it comes down here and I had gone down like that when I did it, but in fact, you've got to go over and back up. So it's actually 8. So that says that the parallelism is a little bit more than 2, 2 and 1/8. What that says is that if I use many more than two processors, I can't get linear speedup anymore. I'm only going to get marginal performance gains. If I use more than 2, because the maximum speedup I can get is like 2.125 if I had an infinite number of processors.
So any questions about this? So this by the way deceptively simple and yet, if you don't play around with it a little bit, you can get confused very easily. Deceptively simple, very powerful to be able to do this.
So here we have for the analysis of parallelism, one of the things that we have going for us in using the Cilk tool suite is a program called Cilkview, which has a scalability analyzer. And it is like the race detector that I talked to you about last time in that it uses dynamic instrumentation. So you run it under Cilkview, it's like running it under [? Valgrhen ?] for example, or what have you. So basically you run your program under it, and it analyzes your program for scalability. It computes the work and span of your program to derive some upper bounds on parallel performance and it also estimates a scheduling overhead to compute what's called a burden span for lower bounds.
So let's take a look. So here's, for example, here's a quick sort program. So let's just see this is a c++ program. So here we're using a template so that the type of items that I'm sorting I can make be a variable. So tightening-- can we shut the back door there? One of the TAs? Somebody run up to-- thank you.
So we have the variable T And we're going to quick sort from the beginning to the end of the array. And what we do is, just as you're familiar with quick sort, if there's actually something to be sorted, more than one thing, then we find the middle by partitioning the thing and this is a bit of a c++ magic to find the middle element. And then the important part from our point of view is after we've done this partition, we quick sort the first part of the array, from beginning to middle and then from the beginning plus 1 or the middle, whichever is greater to the end. And then we sync.
So what we're doing is quick sort where we're spawning off the two sub problems to be solved in parallel recursively. So they're going to execute in parallel and they're going to execute in parallel and so forth. So a fairly natural thing to divide, to do divide and conquer on quick sort because the two some problems can be operated on independently. We just sort them recursively. But we can sort them in parallel. OK, so suppose that we are sorting 100,000 numbers. How much parallelism do you think is in this code? So remember that we're getting this recursive stuff done. How many people think-- well, it's not going to be more than 100,000, I promise you. So how many people think more than a million parallels? Raise your hand, more than a million? And how many people think more than 100,000? And how many people think more than 10,000? OK, between the two. More than 1,000? OK, how about more than 100? 100 to 1,000? How about 10 to 100? How about between 1 and 10?
So a lot of people think between 1 and 10. Why do you think that there's so little parallels in this? You don't have to justify yourself, OK. Well let's see how much there is according to Cilkview. So here's the type of output that you'll get. You'll get a graphical curve. You'll also get a textual output. But this is sort of the graphical output. And this is basically showing what the running time here is. So the first thing it shows is it will actually run your program, benchmark your program, on in this case, up to 8 course. We ran it. So we ran up to 8 course and give you what your measured speedup is.
So the second thing is it tells you the parallels. If you can't read that it's, 11.21. So we get about 11. Why do you think it's not higher? What's that?
AUDIENCE: It's the log.
PROFESSOR: What's the log?
AUDIENCE: [UNINTELLIGIBLE]
PROFESSOR: Yeah, but you're doing the two things in parallel, right? We'll actually analyze this. So it has to do with the fact that the partition routine is a serial piece of code and it's big. So the initial partitioning takes you 100,000-- sorry, 100 million steps of doing a partition-- before you get to do any parallelism at all. And we'll see that in just a minute. So it gives you the parallelism. It also plots this. So this is the parallelism. Notice that's the same number, 11.21 is plotted as this bound. So it tells you the span law and it tells you the work law. This is the linear speedup. If you were having linear speedup, this is what your program would give you. So it gives you these two bounds, the work law and span law on your speedup. And then it also computes what's called a burden parallelism, estimating scheduling overheads to sort of give you a lower bound.
Now that's not to say that your numbers can't fall outside this range. But when they do, it will tell you essentially what the issues are with your program. And we'll discuss how you diagnose some of those issues. Actually that's in one of the handouts that we've provided. I think that's in one of the handouts. If not, we'll make sure it's among the handouts.
So basically, this gives you a range for what you can expect. So the important thing here is to notice here for example, that we're losing performance, but it's not due to the parallelism, to the work law. Basically, in some sense, what's happening is we are losing it because the Span Law because we're starting to approach the point where the span is going to be the issue. So we'll talk more about this. So the main thing is you have a tool that can tell you the work and span and so that you can analyze your own programs to understand are you bounded by parallelism, for example, in particular, in the code that you've written.
OK let's do a theoretical analysis of this to understand why that number is small. So the main thing here is that the expected work, as you recall, of quick sort is order n log n. You tend to do order n log n work, you partition and then you're solving two problems of the same size. If you actually draw out the recursion tree, it's log height with linear amount of work on every level for n log end total work.
The expected span, however, is order n because the partition routine is a serial program that partitions up the thing of size n in order n time. So when you compute the parallelism, you get parallelism of order log n and log n is kind of puny parallelism, and that's our technical word for it. So puny parallelism is what we get out of quick sort.
So it turns out there are lots of things that you can analyze. Here's just a selection of some of the interesting practical algorithms and the kinds of analyses that you can do showing that, for example, with merge sort you can do it with work n log n. You can get a span of log qn and so then the parallelism is the ratio of the two. In fact, you can actually theoretically get log squared n span, but that's not as practical an algorithm as the one that gives you log cubed n. And you can go through and there are a whole bunch of algorithms for which you can get very good parallelism.
So all of these, if you look at the ratio of these, the parallelism is quite high. So let's talk a little bit about what's going on underneath and why parallelism is important. So when you describe your program in Cilk, you express the potential parallelism of your application. You don't say exactly how it's going to be scheduled, that's done by the Cilk++ scheduler, which maps the strands dynamically onto the processors at run time. So it's going to do the load balancing and everything necessary to balance your computation off the number of processors. We want to understand how that process works, because that's going to help us to understand how it is that we can build codes that will map very effectively on to the number of processors.
Now it turns out that the theory of the distributed schedulers such as is in Cilk++ is complicated. I'll wave my hands about it towards the end, but the analysis of it is advanced. You have to take a graduate course to get that stuff. So instead, we're going to explore the ideas with a centralized, much simpler, scheduler which serves as a surrogate for understanding what's going on.
So the basic idea of almost all scheduling theory in this domain is greedy scheduling. And so this is-- by the way, we're coming to the second thing you have to understand really well in order to be able to generate good code, the second sort of theoretical thing-- so the idea of a greedy scheduler is you want to do as much work as possible on each step. So the idea here is let's take a look, for example, suppose that we've executed this part of the DAG already. Then there are certain number of strands that are ready to execute, meaning all their predecessors have exited. How many strands are ready to execute on this DAG? Five, right? These guys. So those five strands are ready to execute.
So the idea is-- and let me illustrate for p equals 3-- the idea is to understand the execution in terms of two types of steps. So in a greed schedule, you always do as much as possible. So is what would be called a complete step because I can schedule all three processors to have some work to do on that step. So which are the best three guys to be able to execute? Yes, so I'm not sure what the best three are, but for sure, you want to get this guy and this guy, right? Maybe that guy's not, but this guy, you definitely want to execute. And these guys, I guess, OK. So in a greedy schedule, no, you're not allowed to look to see which ones are the best execute. You don't know what the future is, the scheduler isn't going to know what the future is so it just executes any p course. You just execute any p course. In this case, I executed the p strand. In this case, I executed these three guys even though they weren't necessarily the best. And in a greedy scheduler, it doesn't look to see what's the best one to execute, it just executes as many as it can this case. In this case, it's p.
Now we have what's called an incomplete step. Notice nothing got enabled. That was sort of too bad. So there's only two guys that are ready to go. What do you think happens if I have an incomplete step, namely p strands are ready, fewer than p strands are ready? I just to execute all of them, as many as I can. Run all of them.
So that's what a greedy scheduler does. Just at every step, it executes as many as it can and we can classify the steps as ones which are complete, meaning we used all our processors versus incomplete, meaning we only used a subset of our processors in scheduling it. So that's what a greedy scheduler does.
Now the important thing, which is the analysis of this program. And this is, by the way, the single most important thing in scheduling theory but you're going to ever learn is this particular theory. It goes all the way back to 1968 and what it basically says it is any greedy scheduler achieves a bound of T1 over p plus T infinity. So why is that an interesting upper bound? Yeah?
AUDIENCE: That says that it's got the refinement of what you said before, even if you add as many processors as you can, basically you're bounded by T infinity.
PROFESSOR: Yeah.
AUDIENCE: It's compulsory.
PROFESSOR: So basically, each of these, this term here is the term in the Work Law. This is the term in the Span Law, and we're saying you can always achieve the sum of those two lower bounds as an upper bound. So let's see how we do this and then we'll look at some of the implications. Question, do you have a question? No?
So here's the proof that you meet this. So that the proof says-- and I'll illustrate for P equals 3-- how many complete steps could we have? So I'll argue that the number of complete steps is at most T1 over p. Why is that? Every complete step performs p work. So if I had more complete steps than T1 over p, I'd be doing more than T1 work. But I only have T1 work to do. OK, so the maximum number of complete steps I could have is at most T1 over p. Do people follow that?
So the trickier part of the proof, which is not all that tricky but it's a little bit trickier, is the other side. How many incomplete steps could I have? So we execute those. So I claim that the number of incomplete steps is bounded by the critical path length, by the span. Why is that? Well let's take a look at the part of DAG that has yet to be executed. So that this gray part here. There's some span associated with that. In this case, it's this longest path. When I execute all of the ready threads that are ready to go, I guarantee to reduce the span of that unexecuted DAG by at least one. So as I do here, so I reduce it by one when I execute.
So if I have a complete step, I don't guaranteed to reduce the span of the unexecuted DAG, because I may execute things as I showed you in this example, you don't actually advance anything. But I execute all the ready threads on an incomplete step, and that's going to reduce it by one. So the number of incomplete steps is at most infinity. So the total number of steps is at most the sum. So as I say, this proof you should understand in your sleep because it's the most important scheduling theory proof that you're going to probably see in your lifetime. It's very old, and really, very, very simple and yet, there's a huge amount of scheduling theory if you have a look at scheduling theory, that comes out of this just making this same problem more complicated and more real and more interesting and so forth. But this is really the crux of what's going on. Any questions about this proof?
So one corollary of the greedy scheduling algorithm is that any greedy scheduler achieves within a factor of two of optimal scheduling. So let's see why that is. So it's guaranteed as an upper bound to get within a factor of two of optimal. So here's the proof. So let's Tp star be the execution time produced by the optimal scheduler. This is the schedule that knows the whole DAG in advance and can schedule things exactly where they need to be scheduled to minimize the total amount of time. Now even though the optimal scheduler can schedule very officially, it's still bound by the Work Law and the Span Law. So therefore, Tp star has still got to be greater than T1 over p and greater than T infinity by the Work and Span Laws. Even though it's optimal, every scheduler must obey the Work Laws and Spam Law.
So then we have, by the greedy scheduling theorem, Tp is at most T1 over p plus T infinity. Well that's at most twice the maximum of these two values, whichever is larger. I've just plugged in to get the maximum of those two and that's at most, by this equation, twice the optimal time. So this is a very simple corollary says oh, greedy scheduling is actually pretty good. It's not optimal, in fact, optimal scheduling is mP complete. Very hard problem to solve. But getting within a factor of two, you just do greedy scheduling, it works just fine.
More importantly is the next corollary, which has to do is when do you get linear speedup? And this is, I think, the most important thing to get out of this. So any greedy scheduler achieves near perfect linear speedup whenever-- what's this thing on the left-hand side? What's the name we call that?-- the parallelism, right? That's the parallelism, is much bigger than the number of processors you're running on. So if the number of processors are running on is smaller than the parallelism of your code says you can expect near perfect linear speedup. OK, so what does that say you want to do in your program? You want to make sure you have ample parallelism and then the scheduler will be able to schedule it so that you get near perfect linear speedup. Let's see why that's true.
So T1 over T infinity is much bigger than p is equivalent to saying that T infinity is much less than T1 over p. That's just algebra. Well what does that mean? The greedy scheduling theorem says Tp is at most T1 over p plus T infinity. We just said that if we have this condition, then T infinity is very small compared to T1 over p. So if this is negligible, then the whole thing is about T1 over p. Well that just says that the speedup is about p. So the name of the game is to make sure that your span is relatively short compared to the amount of work per processor that you're doing. And in that case, you'll get linear speedup. And that happens when you've got enough parallelism compared to the number processors you're running on. Any questions about this? This is like the most important thing you're going to learn about parallel computing.
Everything else we're going to do is going to be derivatives of this, so if you don't understand this, you have a hard time with the other stuff. So in some sense, it's deceptively simple, right? We just have a few variables, T1, Tp, T infinity, p, there's not much else going on. But there are these bounds and these elegant theorems that tell us something about how no matter what the shape of the DAG is or whatever, these two values, the work and the span, really characterize very closely where it is that you can expect to get linear speedup. Any questions? OK, good.
So the quantity T1 over PT infinity, so what is that? That's just the parallelism divided by p. That's called the parallel slackness. So this parallel slackness is 10, means you have 10 times more parallelism than processors. So if you have high slackness, you can expect to get linear speedup. If you have low slackness, don't expect to get linear speedup. OK. Now the scheduler we're using is not a greedy scheduler. It's better in many ways, because it's a distributed, what's called work stealing scheduler and I'll show you how it works in a little bit. But it's based on the same theory. Even though it's a more complicated scheduler from an analytical point of view, it's really based on the same theory as greedy scheduling. It guarantees that the time on p processors is at most T1 over p plus order T infinity. So there's a constant here.
And it's a randomized scheduler, so it actually only guarantees this in expectation. It actually guarantees very close to this with high probability. OK so the difference is the big O, but if you look at any of the formulas that we did with the greedy scheduler, the fact that there's a constant there doesn't really matter. You get the same effect, it just means that the slackness that you need to get linear speedup has to not only overcome the T infinity, it's also got to overcome the constant there. And empirically, it actually turns out this is not bad as an estimate using the greedy bound. Not bad as an estimate, so this is sort of a model that we'll take as if we're doing things with a greedy scheduler. And that will be very close for what we're actually going to see in practice with the Cilk++ scheduler.
So once again, it means near perfect linear speedup as long as p is much less than T1 over T infinity generally. And so Cilkview allows us to measure T1 and T infinity. So that's going to be good, because then we can figure out what our parallelism is and look to see how we're running on typically 12 cores, how much parallels do we have? If our parallelism is 12, we don't have a lot of slackness. We won't get very good speedup. But if we have a parallelism of say, 10 times more, say 120, we should get very, very good parallelism, very, very good speedup on 12 cores. We should get close to perfect speedup.
So let's talk about the runtime system and how this work stealing scheduler works, because it different from the other one. And this will be helpful also for understanding when you program these things what you can expect. So the basic idea of the schedule is there's two strategies the people have explored for doing scheduling. One is called work sharing, which is not what Cilk++ does. But let me explain what work sharing is because it's helpful to contrast it with work stealing.
So in works sharing, what you do is when you spawn off some work, you say let me go find some low utilized processor and put that worked there for it to operate on. The problem with work sharing is that you have to do some communication and synchronization every time you do a spawn. Every time you do a spawn, you're going to go out. This is kind of what Pthreads does, when you do Pthread create. It goes out and says OK, let me create all of the things it needs to do and get it schedule then on a processor.
Work stealing, on the other hand, takes the opposite approach. Whenever it spawns work, it's just going to keep that work local to it, but make it available for stealing. A processor that runs out of work is going to go looking for work to steal, to bring back. The advantage of work stealing is that the processor doesn't do any synchronization except when it's actually load balancing. So if all of the processors have ample work to do, then what happens is there's no overhead for scheduling whatsoever. They all just crank away. And so you get very, very low overheads when there's ample work to do on each processor.
So let's see how this works. So the particular way that it maintains it is that basically, each processor maintains a work deck. So a deck is a double-ended queue of the ready strands. It manipulates the bottom of the deck like a stack. So what that says is, for example, here, we had a spawn followed by two calls. And basically, it's operating just as it would have to operate in an ordinary stack, an ordinary call stack. So, for example, this guy says call, well it pushes a frame on the bottom of the call stack just like normal. It says spawn, it pushes a spawn frame on the bottom of the deck.
In fact, of course, it's running in parallel, so you can have a bunch of guys that are both calling and spawning and they all push whatever their frames are. When somebody says return, you just pop it off. So in the common case, each of these guys is just executing the code serially the way that it would normally executing in C or C++. However, if somebody runs out of work, then it becomes a thief and it looks for a victim and the strategy that's used by Cilk++ is to look at random. It says let me just go to any other processor or any other workers-- I call these workers-- and grab away some of their work. But when it grabs it away, what it does is it steals it from the opposite end of the deck from where this particular victim is actually doing its work. So it steals the oldest stuff first. So it moves that over and now here what it's doing is it's stealing up to the point that it spawns. So it steals from the top of the deck down to where there's a spawn on top. Yes?
AUDIENCE: Is there always a spawn on the top of every deck?
PROFESSOR: Close, almost always. Yes, so I think that you could say that there are. So the initial deck does not have a spawn on top of it, but you could imagine that it did. And then when you steal, you're always stealing from the top down to a spawn. If there isn't something, if this is just a call here, this cannot any longer be stolen. There's no work there to be stolen because this is just a single execution, there's nothing that's been spawned off at this point. This is the result of having been spawned as opposed to that it's doing a spawn. So yes, basically you're right. There's a spawn on the top.
So it basically steals that off and then it resumes execution afterwards and starts then operating just like an ordinary deck. So the theorem that you can prove for this type of scheduler is that if you have sufficient parallelism, so you all know what parallelism is at this point, you can prove that the workers steal infrequently. So in a a typical execution, you might have a few hundred load balancing operations of this nature for something which is doing billions and billions of instructions. So you steal infrequently. If you're stealing infrequently and all the rest of the time you're just executing like the C or C++, hey, now you've got linear speedup because you've got all of these guys working all the time.
And so as I say, the main thing to understand is that there's this work stealing scheduler running underneath. It's more complicated to analyze then the greedy scheduler, but it gives you pretty much the same qualitative kinds of results. And the idea then is that the stealing occurs infrequently so you get linear speedup. So the idea then is just as with greedy scheduling, make sure you have enough parallelism, because then the load balancing is a small fraction of the time these processors are spending executing the code. Because whenever it's doing things like work stealing, it's not working on your code executing, making it go fast. It's doing bookkeeping and overhead and stuff. So you want to make sure that stays low. So any questions about that?
So specifically, we have these bounds. You have achieved this expected running time, which I mentioned before. Let me give you a pseudo-proof of this. So this is not a real proof because it ignores things like independence of probabilities. So when you do a probability analysis, you're not allowed to multiply probabilities unless they're independent. So anyway, here I'm multiplying probabilities that are independent. So the idea is you can view a processor as either working or stealing. So it goes into one of two modes. It's going to be stealing if it's run out of work, otherwise it's working. So the total time all processors spend working is T1, hooray, that's at least a bound.
Now it turns out that every steal has a 1 over p chance of reducing the span by one. So you can prove that of all of the work that's in the top of all those decks that those are where any of the ready threads are going to be there are in a position of reducing the span if you execute them. And so whenever you steal, you have a 1 over p chance of hitting the guy that matters for the span of unexecuted DAG. So the same kind of thing as in theory. You have a 1 over p chance. So the expected cost of all steals is order PT infinity. So this is true, but not for this reason. But it's kind, the intuition is right. So therefore the cost of all steals is PT infinity and the cost of the work is T1, so that's the total amount of work and time spent stealing by all the p processors. So to get the time spent doing that, we divide by p, because they're p processors. And when I do that, I get T1 over p plus order T infinity. So that's kind of where that bound is coming from.
So you can see what's important here is that the term, that order T infinity term, this the one where all the overhead of scheduling and synchronization is. There's no overhead for scheduling and synchronization in the T1 over p term. The only overhead there is to do things like mark the frames as being a steel frame or a spawn frame and do the bookkeeping of the deck as you're executing so the spawn can be implemented very cheaply.
Now in addition to the scheduling things, there are some other things to understand a little bit about the scheduler and that is that it supports the C, C++ rule for pointers. So remember in C and C++, you can pass a pointer to stack space down, but you can't pass a pointer to stack space back to your parent, right? Because it popped off. So if you think about a C or C++ execution, let's say we have this call structure here. A really cannot see any of the stack space of B,C,D or E. So this is what A gets to see. And B, meanwhile, can see A space, because that's down on the stack, but it can't see C, D or E. Particularly if you're executing this serially, it can't see C because C hasn't executed yet when B executes.
However, C, it turns out, the same thing. I can't see any of the variables that might be allocated in the space for B when I'm executing here on a stack. You can see them in a heap, but not on the stack, because B has been popped off at that point and so forth. So this is basically the normal rule, the normal views of stack that you get in C or C++. In Cilk++, you get exactly the same behavior except that multiple ones of these views may exist at the same time. So if, for example, B and C are both executing at the same time, they each will see their own stack space and a stack space. And so the cactus stack maintains that fiction that you can sort of look at your ancestors and see your ancestors, but now it's maintained. It's called a cactus stack because it's kind of like a tree structure upside down, like a what's the name of that big cactus out West? Yes, saguaro. The saguaro cactus, yep. This kind of looks like that if you look at the stacks.
This leads to a very powerful bound on how much space your program is using. So normally, if you do a greedy scheduler, you could end up using gobs more space then you would in a serial execution, gobs more stack space. In Cilk++ programs, you have a bound. It's p times s1 is the maximum amount of stack space you'll ever use where s1 is the stack space used by serial execution. So if you can keep your serial execution to use a reasonable amount of stack space-- and usually it does-- then in parallel, you don't use more than p times that amount of stack space.
And the proof for that is sort of by induction, which basically says there's a property called the Busy Leaves Property that says that if you have a leaf that's being worked on but hasn't been completed-- so I've indicated those by the purple and pink ones-- then if it's a leaf, it has a worker executing on it. And so therefore, if you look at how much stack space you're using, each of these guys can trace up and they may double count the stack space, but it'll still be bounded by p times the depth that they're at, or p times s1, which is the maximum amount. So it has good space bounds. That's not so crucial for you folks to know as a practical matter, but it would be if this didn't hold. If this didn't hold, then you would have more programming problems than you'll have.
The implications of this work stealing scheduler is interesting from the linguistic point of view, because you can write a code like this, so for i gets one to a billion, spawn some sub-routine foo of i and then sync. So one way of executing this, the way that the work sharing schedulers tend to do this, is they say oh, I've got a billion tasks to do. So let me create a billion tasks and now schedule them and the space just vrooms to store all those billion tasks, it gets to be huge. Now of course, they have some strategies they can use to reduce it by bunching tasks together and so forth. But in principle, you got a billion pieces of work to do even if you execute on one processor. Whereas in the work stealing type execution, what happens is you execute this in fact depth research. So basically, you're going to execute foo of 1 and then you'll return. And then you'll increment i and you'll execute foo of 2, and you'll return. At no time are you using more than in this case two stack frames, one for this routine here and one for foo because you basically keep going up. You're using your stack up on demand, rather than creating all the work up front to be scheduled.
So the work stealing scheduler is very good from that point of view. The tricky thing for people to understand is that if executing on multiple processors, when you do Cilk spawn, the processor, the worker that you're running on, is going to execute foo of 1. The next statement-- which would basically be incrementing the counter and so forth-- is executed by whatever processor comes in and steals that continuation. So if you had two processors, they're each going to basically be executing. The first processor isn't the one that excuse everything in this function. This function has its execution shared, the strands are going to be shared where the first part of it would be done by processor one and the latter part of it would be done by processor two. And then when processor one finishes this off, it might go back and steal back from processor two. So the important thing there is it's generating its stack needs sort of on demand rather than all up front, and that keeps the amount of stack space small as it executes.
So the moral is it's better to steal your parents from their children than stealing children from their parents. So that's the advantage of doing this sort of parent stealing, because you're always doing the frame which is an ancestor of where that worker is working and that means resuming a function right in the middle on a different processor. That's kind of the magic of the technologies is how do you actually move a stack frame from one place to another and resume it in the middle?
Let's finish up here with a chess lesson. I promised a chess lesson, so we might as well do some fun and games. We have a lot of experience at MIT with chess programs. We've had a lot of success, probably our closest one was Star Socrates 2.0, which took second place in the world computer chess championship running on an 1824 node Intel Paragon, so a big supercomputer running with a Cilk scheduler. We actually almost won that competition, and it's a sad story that maybe be sometime around dinner or something I will tell you the sad story behind it, but I'm not going to tell you why we didn't take first place. And we've had a bunch of other successes over the years. Right now our chess programming is dormant, we're not doing that in my group anymore, but in the past, we had some very strong chess playing programs.
So what we did with Star Socrates, which is one of our programs, was we wanted to understand the Cilk scheduler. And so what we did is we ran a whole bunch of different positions on different numbers of processors which ran for different amounts of time. We wanted to plot them all on the same chart, and here's our strategy for doing it. What decided to do was do a standard speedup curve. So a standard speedup curve says let's plot the number of processors along this axis and the speed up along that axis. But in order to fit all these things on the same processor curve, what we did was we normalize the speedup. So what's the maximum pot? So here's the speedup. If you look the numerator here, this is the speedup, T1 over Tp. What we did is we normalized by the parallelism. So we said what fraction of perfect speedup can we get?
So here one says that I got exactly a speedup, this is the maximum possible speed up that I can get because the maximum possible value of T1 over p is T1 over T infinity. So that's sort of the maximum. On this axis, we said how many processors are you running on it? Well, we looked at that relative to essentially the slackness. So notice by normalizing, we essentially have here the inverse of the slackness. So 1 here says that I'm running on exactly the same number of processors as my parallelism. A tenth here says I've got a slackness of 10, I'm running on 10 times fewer processors then parallelism. Out here, I'm saying I got way more processors than I have parallelism. So I plotted all the points. So it doesn't show up very well here, but all those green points, there are a lot of green points here, that's our performance, measured performance. You can sort of see they're green there, not the best color for this projector. So we plot on this essentially the Work Law and the Span Law. So this is the Work Law, it says linear speedup, and this is the Span Law. And you can see that we're getting very close to perfect linear speedup as long as our slackness is 10 or greater. See that? It's hugging that curve really tightly.
As we approach a slackness of 1, you can see that it starts to go away from the linear speedup curve. So for this program, if you look, it says, gee, if we were running with 10 time, slackness of 10, 10 times more parallelism than processors, we're getting almost perfect linear speedup in the number of processors we're running on across a wide range of number of processors, wide range of benchmarks for this chess program. And in fact, this curve is the curve. This is not an interpolation here, but rather it is just the greedy scheduling curve, and you can see it does a pretty good job of going through all the points here. Greedy scheduling does a pretty good job of predicting the performance.
The other thing you should notice is that although things are very tight down here, as you approach up here, they start getting more spread. And the reason is that as you start having more of the span mattering in the calculation, that's where all the synchronization, communication, all the overhead of actually doing the mechanics of moving a frame from one processor to another take into account, so you get a lot more spread as you go up here.
So that's just the first part of the lesson. The first part was, oh, the theory works out in practice for real programs. You have like 10 times more parallelisms than processors, you're going to do a pretty good job of getting linear speedup. So that says you guys should be shooting for parallelisms on the order of 100 for running on 12 cores. Somewhere in that vicinity you should be doing pretty well if you've got parallelism of 100 when you measure it for your codes. So we normalize by the parallel there.
Now the real lesson though was understanding how to use things like work and span to make decisions in the design of our program. So as it turned out, Socrates for this particular competition was to run on a 512 processor connection machine at the University of Illinois. So this was in the mid in the early 1990's. It was one of the most powerful machines in the world, and this thing is probably more powerful today. But in those days, it was a pretty powerful machine. I don't know whether this thing is, but this thing probably I'm pretty sure is more powerful. So this was a big machine.
However here at MIT, we didn't have a great big machine like that. We only had a 32 processor CM5. So we were developing on a little machine expecting to run on a big machine. So one of the developers proposed to change the program that produced a speedup of over 20% on the MIT machine. So we said, oh that's pretty good, 25% improvement. But we did a back of the envelope calculation and rejected that improvement because we were able to use work and span to predict the behavior on the big machine. So let's see how that worked out, why that worked out.
So I've fudged these numbers so that they're easy to do the math on and easy to understand. The real numbers actually though did sort out very, very similar to what I'm saying, just they weren't round numbers like I'm going to give you. So the original program ran for let's say 65 seconds on 32 cores. The proposed program ran for 40 seconds on 32 cores. Sounds like a good improvement to me. Let's go for the faster program. Well, let's hold your horses. Let's take a look at our performance model based on greedy scheduling. That Tp is T1 over p plus infinity. What component we really need to understand the scale this, what component of each of these things is work and which is span? Because that's how we're going to be able to predict what's going to happen on the big machine.
So indeed, this original program had a work of 2048 seconds and a span of one second. Now chess, it turns out, is a non-deterministic type of program where you use speculative parallelism, and so in order to get more parallelism, you can sacrifice and do more work versus less work. So this one over here that we improved it to had less work on the benchmark, but it had a longer span. So it had less work but a longer span. So when we actually were going to run this, well first of all, we did the calculation and it actually came out pretty close. I was kind of surprised how close the theory matched. We actually on 32 processors when you do the work spanned calculation, you get the 65 seconds on a 32 processor machine, here we had 40 seconds.
But now what happens when we scale this to the big machine? Here we scaled it to 512 cores. So now we take the work divided by the number of processors, 512, plus 1, that's 5 seconds for this. Here we have the work but we now have a much larger span. So we have two seconds of work for processor, but now eight seconds of span for a total of 10 seconds. So had we made this quote "improvement," our code would have been half as fast. It would not have scaled.
And so the point is that work and span typically will beat running times for predicting scalability of performance. So you can measure a particular thing, but what you really want to know is this thing this going to scale and how is it going to scale into the future. So people building multicore applications today want to know that they coded up. They don't want to be told in two years that they've got to recode it all because the number of cores doubled. They want to have some future-proof notion that hey, there's a lot of parallelism in this program.
So work and span, work and span, eat it, drink it, sleep it. Work and span, work and span, work and span, work and span, work and span, OK? Work and span.
Parallelism and Performance (PDF - 2.7MB)
Free Downloads
Video
- iTunes U (MP4 - 178MB)
- Internet Archive (MP4 - 178MB)
Subtitle
- English - US (SRT)