Flash and JavaScript are required for this feature.
Download the video from iTunes U or the Internet Archive.
Description: In this recitation, problems related to 2-3 Trees and B-Trees are discussed.
Instructors: Amartya Shankha Biswas
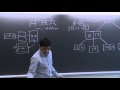
Recitation 2: B-trees
The following content is provided under a Creative Commons license. Your support will help MIT OpenCourseWare continue to offer high quality educational resources for free. To make a donation or view additional materials from hundreds of MIT courses, visit MIT OpenCourseWare at ocw.mit.edu.
PROFESSOR: So this is a 2-3 tree. So as you can see, every node has-- so the 2-3 is either two children or three children. Every node can have either one key or two keys. And the correlation is that every-- so if there are n keys in a node, it has n plus 1 children.
So the way that works is similar to binary search trees. So if you have value here, the two children surrounding it-- so this side is less, this side is more. So it's essentially sort of going in order reversal, left child, root, right child. So [INAUDIBLE], it's ordered.
So generally a B tree will have some nodes. So let's say n and n plus 1 children. And if you take anything in the middle, look at the two children, all the keys in this sub-tree are smaller than the key here, and all the keys in this sub-tree are larger than the key here. So that's the general node.
So before we go into more details of the properties and everything, the question is why use B-trees. So if we do a quick depth analysis, we can see that the depth is to log n rate. Is that clear to everyone sort of, why the depth is log n? Because you have branching just like in binary search trees. In fact, you have more branching. But in any case, depth is to log n.
But why use B-trees over binary search trees? Anyone have a reason why you would prefer to use B-trees or not? So all the operations are still log n. Any guesses? None. OK.
Well, OK, the reason is memory hierarchy. So normally in [INAUDIBLE], we just assume that the computer has access to memory, and you can just pick up things from disk and constant time and do your operations with it, and you don't worry about caches and everything. But that's not how computers work. So in a computer, you have-- so those of you who have taken some computer architecture class [INAUDIBLE] or something, you will know that hierarchy.
So there's a CPU-- so let's draw it somewhere. So you have your CPU. And [INAUDIBLE] CPU, you have some registers. You have your caches, L1, L2, L3, whatever. You have your RAM. You have disk after that. So disk [? loads. ?] Then you have your, I don't know, your cloud, whatever. So each level, your memory size grows and your access time grows as well.
So in the basic memory hierarchy model, we have just two levels of hierarchy, let's say. So you have cache connected by a high bandwidth channel to the CPU, and you have a low bandwidth channel to disk. So the difference is-- so essentially you can consider that cache just has infinite speed. Cache, just like, whatever you can take it. You don't have any cost for bringing in stuff from cache. But it's finite size. So the way cache works is it has a bunch of words, which is a finite number of words. So each word has size B, and let's say you have m words.
However, hard disk is just, let's say, infinite memory, but it has some cost associated to accessing things. Also when you access things from hard disk, you copy them into cache. When you copy a block of size b, you take it up from the hard disk, and you take a block, and you put it into cache. And you have to get rid of something because it's fine.
So what you want to do is you want to utilize that b block efficiently. You just want to bring a b block every time you want to access a new node. In a binary search tree, normal operations are what? You start in the root and go to a node. But that's not very easily correlated with this. Right? So if you want to utilize an entire block, you would want something like a block which sort of goes down the tree. But that's not how binary trees are stored. Binary trees are stored this way.
So that's the nice thing about B-trees. So this is just a 2-3 tree. This is not a general B-tree. A general B-tree will have a bunch of nodes, and we'll come to that number. But generally you want to make that number of nodes something like the cache-- what is it? The word size in the cache.
So once you do that, you can get an entire node from disk, like work on that, and then get another [INAUDIBLE], so your height is reduced. And you can do your operation much quicker, because you're not accessing disk every time you're going down a level. Sorry. You are accessing disk every time you go down a level, but you're utilizing the whole block when you're accessing disk. Good? Sort of make sense? OK.
So let's write down the specifications for B-trees now. All right. So number of children. So first of all, a B-tree has something called a branching factor. So in the 2-3 tree, the branching factor is two. So what that means is simply that it just balances the number of children. So the number of children has to be greater than or equal to 2. Other than the root node. The root node can have less than B children. It's fine.
Also it's upper bounded by 2B [? plus ?] 2B. Notice that this is a strict upper bound. So you can have at most 2B minus 1 children from a node.
Also remember that the number of keys, the number of keys is just 1 less than the number of children. Therefore, these inequalities are just reduced by 1. So you have minus 1 and you have 2B minus 1. So the number of keys can be between minus 1 and 2B minus 2. The rationale for that will become clear-- yeah?
AUDIENCE: Is B the height of the tree?
PROFESSOR: No, B is the branching. B is the branching factor. So that is the number of children. It's not the number of children. It's a bound of the number of children. So like in the 2-3 tree, B is equal to 2, and this is a 2-3 tree. So the 2 refers to-- you can have either two children or you can have three children. And so the upper bound on children is 2B minus 1. 2B minus 1 is equal to 3. So you can have two or three children.
And correspondingly, you can have either one or two keys in a node. Make sense?
AUDIENCE: Yeah.
PROFESSOR: Cool. OK So coming back to this. So the root does not have a lower bound. The root can have one child in any tree. So you have a B equal to 5 tree, the root can still have one child-- sorry. Not one child, one key element, two children. All right. It's good.
Also it's completely balanced. So all the leaves are the same depth. So you can see it here, right? So you can't have a dangling node here. This is not allowed. You have to have a leaf. You have to have something going down, and everything ends at the same level. All right. So that's the thing. So also the leaves obviously don't have children, so this condition is violated by the leaf. So that's the basic structure of a B-tree.
So the first operation we'll consider on B-trees is searching. So that should be relatively straightforward. So remember how searching is done in the binary search tree. You bring in a value x compared to the key. Let's say x is less than K, you go down this path. Let's say x is greater than K, you go down this path. So similarly in a B-tree.
So let's say we bring in a value. Let's say you are looking for 20. So you bring in 20 compared to this. 20 is less than 30, so you go down here. Now you have two values. So where does 20 fit in here? Not here. Not here. It fits here. OK. Go down this tree. You find 20, that's it.
So in general, you bring in a key K, you look at this node, and you go through all the values. So something I forgot to mention, which should be clear. All the keys in a node, they're sorted, one after the other. So your values go like this. So they're increasing in this way. Make sense?
So you bring in a key. Look at all the keys in the node you're looking at, pick the place where K fits in, unless it's already in the node. Then you're done. You've found it. Otherwise, let's say K fits in between these two guys. So you go down this child and continue. So searching with log n, similar to BSTs.
So searching is not very interesting. So next is insertion. So insertion is a little more interesting than searching. So what you do in insertion is you--
[SIDE CONVERSATION]
PROFESSOR: So before we resume, does anyone have any questions about the structure of B-trees. We rushed through that quite fast. About how B-trees are structured, everyone good with that? OK, also any questions about searching in a B-tree or a BST? Go ahead.
AUDIENCE: Just a random question. So the 38 there, it can only have two children.
PROFESSOR: Yep. So one value, two children. So you have some node in the B-tree, and whatever is below it is split into parts by the elements. So if you have n elements, it splits it up into n plus 1 segments.
AUDIENCE: You said that the root didn't have to follow the root.
PROFESSOR: No.
AUDIENCE: Why is that?
PROFESSOR: Well, you'll see when we do insertion and deletion why that's necessary. But essentially you can consider that it's an invariant. And all we have to do is preserve that invariant. So the root, it has to still have less than two-- it still has to have the upper bound. But it doesn't need to have a lower bound.
AUDIENCE: How do you choose B?
PROFESSOR: Well, the whole [INAUDIBLE] cache size, so something with that. So you probably want 2B to be about your cache size so you can get the whole block in one go. I've never implemented a B-tree, so I don't know how it's actually done in practice. But that is the reason, so I'm assuming it's something to do with the cache length.
AUDIENCE: Is the 14, is it a child of both 10 and 17?
PROFESSOR: Well, it's not a child of either. It's a child of this node. So this node has two elements, so it's being divided-- dividing the interval up into three parts. So it's in between 10 and 17 is the point here.
AUDIENCE: So then this node has five children?
PROFESSOR: Sorry? No, it has three children. So don't think of every key as a node. Think of the whole unit as a node. So it's not necessarily-- in a binary search tree, you have one element, but here every node has multiple elements. That's the point of it. Anyone else?
OK, let's start with searching. So let's leave this here. Well, you have the formulas up there, so that's good.
Insertion. Let's start with insertion. We already did searching.
So insertion is you bring in a new key K, and you want to insert it into the tree. So what's the problem that could happen? You can find the location where you want to insert it, just like searching. You just go down the tree and find where it should be placed. But once you do place it, you have a problem. What is the problem? The problem is that one of your nodes will become overfull. Whatever. It'll overflow, and that's not what you want.
So you want some way so you can manage this. How do you manage this? So I have this lovely prop here, which I hope to demonstrate. OK. So here we have B equal to 4. So let's first figure out the number of keys. So what is the minimum number of keys, anyone for B equal to 4?
AUDIENCE: Three.
PROFESSOR: Three, precisely. So what is the maximum number of keys?
AUDIENCE: Six.
PROFESSOR: 4 into 2 minus 3, yeah. Correct. 3, 4. It's not seven, there's a strictly less than sign somewhere. Yes. And you'll see why it's not seven in a minute.
[LAUGHTER]
Oh. Hypocritical of me. All right. So as you can see, 1, 2, 3, 4, 5, 6, 7. So some insertion happened. Is the writing clear? Can everyone read the numbers? 49 looks a little skewed. Anyway, essentially these are all sorted. This is the parent node. Doesn't matter what's over here. All that matters is 8, 56, and whatever's in between.
So what we do when we have an overfull node is something that's called a split operation. So split. And there's something which is called a merge, which we'll come to later when we're doing deletion.
But a split is-- very intuitively, it splits the node into two parts. So what it does is when you have an overfull node-- so the number of elements here is what? 2B minus 1, which is just 1 over the max. So what do you do is you take the middle element and remove it. and now you split the node into two parts. Observe that there are three here and three here, which is perfect.
And now what you do with the middle node-- so now you're actually disrupting the structure of the tree, because there was one pointer going in. There was one child. And now you have two children.
So somehow you need to adjust the parent node, because the parent node had only one child. Well, at least there are other children off to the side. But here it had only one child, and now it's split apart. So you do something very simple. You just insert this guy in here. And then you say, oh, this points here, and this points here. Make sense? I'm going to get rid of these two.
And you can even convince yourself that this preserves all the nice properties. So your children have nicely fallen back into their interval. Your sequence is completely correct, because this was the middle element of this. So this divides this interval properly. This is also between 8 and 56, because this was in this node. So all the properties.
But there's one property that is a problem. So you have just increased the size of the parent node by 1. So now it's possible that the parent node has overflowed. So what do you do? You split it again. And split it again. And if at any point, you're fine, you look at the parent node and go, OK, that's fine. That's in the range. But every time it overflows, you can keep going.
And how many times can you do this? You can do this all the way up to the root. And when you reach the root, either it's fine or the root is too big. It's reached 2B minus 1. And then you split the root, and you get one single [INAUDIBLE] up there. So that, in answer to your question, that is why you need that property in some sense. Not a very convincing argument, but sort of.
So let's actually do an insertion in this tree we have here. So we are going to insert 16. So 16 comes in here. It's less than 30, it goes to the left. It's between 10 and 17, it goes in the middle. 16. And it's greater than 14, so we add 16 here.
All right. That seems good. All the properties fine. This still has two elements, which is the maximum, but it's good. It doesn't overflow.
Let's insert something else. Let's insert 2. So 2 goes to 30, goes down, goes down. And we have a problem, because 2 has overflowed this node.
So we split. And the way we split is we take the middle element. So we split the node here. And 3 goes up to the parent, so 3 goes here. And all good, except for the parent has overflowed.
So what do we do with the parent? We split the parent again. And this time, it's right down the middle, the 10 goes up. So OK, let's get rid of this. So now that we split the parent, the 10 goes up here. And you're good. It's a bit cluttered, so let me reposition the 17.
Did those two operations make sense? Questions?
AUDIENCE: If your node size [INAUDIBLE] number of--
PROFESSOR: So just pick the-- first of all-- OK. If the way we're doing it-- when your node is overflowing, it's returning only one thing at a time, right?
AUDIENCE: Yeah.
PROFESSOR: So if your node is overflowing, it'll be 2t minus 1, which is an odd number always. There might be a case where you get an even number if you do something weird. Maybe you have a-- there are different ways to do B-trees. But if it does, you can probably pick the one, either of them, and then [INAUDIBLE]. I'm not sure about that. I'll look into it.
But in general, if you're doing it this way, it's always odd. So you don't have to worry about that. Anything else?
AUDIENCE: If we did reach all the way to the root and then went one more up--
PROFESSOR: So what you would do is--
AUDIENCE: That root would have--
PROFESSOR: That root would have two children, one element and two children, which is fine because we didn't put that restriction on the root. That's good. How we doing on time? OK, we have some time.
Let's jump into deletion, unless anyone else has questions.
AUDIENCE: [INAUDIBLE] any point?
PROFESSOR: So-- oh, yeah. That's a good-- thank you. So you are going down to the leaves at most-- at most of the leaf ones, and you're going back up one. So it's like log n plus log n, and you're good.
Let's do deletion. So deletion is more complicated. So the reason, it'll be clear. So the problem in deletion will be remove a node and a node is now underfull. So it has less than B minus 1 keys in it suddenly.
So let's turn this around. So again B equal to 4. This node is a problem. Only two things in it. So what do we do?
So before we go into that, let's make this assumption that-- there are two steps to deletion. The first step is making the deletion at a leaf. How do you do that? So the way you make a deletion at a leaf is, let's say, you have a key. You come down in your B-tree, and you add a node. Oh, this key needs to be deleted. But it's not a leaf. So what do you do?
So essentially what you do is you look at these two subtrees. So it might have only one subtree. If it's at the end, it will have only one. Actually, no, that's not true. Ignore that.
If it's not a leaf, it has two subtrees. So either take the rightmost element in this subtree, which is a leaf, because you can always keep going down, right, right, right, right till you get to a leaf, or the leftmost element in this subtree. So that is just the next element after this guy.
So you delete this, and you bring this up to here. We'll do an example of this, and it'll be clearer. So you take either the rightmost element in the left subtree or the leftmost element in the right subtree and bring it up here. So you sort of like move the deletion to the leaf. And now it's easier to deal with. So we will come to that.
Also just note that this is not what is done in the recitation. This algorithm for deletion, I think, is not done in the recitation notes. This is a different thing, which I'll send out a link for later. But I believe it works, because I got it from the [INAUDIBLE] reference.
So once you move to the leaf-- so now let's look at this. So this is a node that is underfull. And you want to fix it. So how do you fix it?
So what do is you look at its siblings. So in this case, it has one sibling. It can have up to two siblings. It can have left or right.
So what you do is you look at a sibling. And this sibling is actually 1 over the minimum. And if it's 1 over the minimum, then it's really easy. All you have to do is take the leftmost thing here-- or if it's the sibling on this side, take the rightmost thing here. And look at its parent. So you bring the parent down, and you move the sibling up. And there we go.
So you basically are rotating the thing into place. So you move the parent down into the underfull node, and you replace the parent by the leftmost thing here. Everyone see why that preserves everything?
And the child is also shifted. Make sure you see that. So the child which was in this subtree is now in this subtree.
But then you can have the situation where you don't have a nice sibling to take care of your problems. So in this scenario, the sibling is barely full. It has three things, and it can't donate anything to you. So what do you do in that case?
So then you do something which is a parallel of the split operation. You do a merge. So what do you have? So here you have B minus 2, and here you have B minus 1. And you get 2B minus 3. Well, you've got another element. You also take the parent.
So how do you do the merge. I just want to show you the merge first. So the way you do it is you move the parent down, and you merge these two. Seems OK? So you move the parent node down and merge these two. And, well, now this comes together, and this points into the new node. Sort of clear what's going on? Questions? Yes?
AUDIENCE: So now the parent is underfull?
PROFESSOR: Well, so you have-- yeah, exactly. So you have decreased the size of the parent, so it might be underfull. So you propagate. Anything else?
AUDIENCE: So are these all different techniques for doing that?
PROFESSOR: So there are two cases. So either you have a sibling which has extra nodes to donate to you or you don't. If you don't, then you have to do this.
AUDIENCE: But what about that case? Or is that just like--
PROFESSOR: No, that is moving it down to the leaf. Once you move the deletion down to the leaf, so here we have something now. And now you move it all the way back up. So there are two cases. Let's do an example. That'll make it clearer. How are we doing on time? Five minutes, all right.
So we are going to delete 38. 38 is gone. But we want to move it down to the leaf. So let's take an element. Let's say we take 41. So we take 41 and move it up here. 41 is the leftmost thing in the right subtree.
So this vacancy doesn't really affect anything, because this node still has the right number of things, because it's still got one thing in it, which is good. So you're fine. This is now just 48.
Let's say we now delete 41. So 41 is gone. So now that 41 is gone, what do you replace this blank spot with? Either this or this, right? Doesn't matter. So let's just do this one for consistency. So you have 48 here.
And now you a problem because you have a blank box. So can you rotate? Yes, no? No, right? Because sibling is barely full. So what can you do? So you merge. And how do you merge? You move the 48 down, and you combine everything.
So this is kind of hard to understand, but this is like a zero-element node. So when you merge, you have 32, 48, and nothing, so it's just 32 and 48. So what you do is-- so this seems weird, but this is just another empty node. You just propagated the emptiness upwards.
Now you take this empty node, and you look for its siblings. Again, its sibling is-- well, it's barely full. So what do you do now? You bring the 30 down, and you merge this. So let's do that.
30 comes down, and there we go. Looks fine? Does that tree look good? Questions about the operation? I'm sure it was not clear, but-- anything? Make sense?
OK, let's do a deletion where we can actually do a rotation. So let's go ahead and delete 20. So you do your searching, go down the tree. You find the 20 under here. So now, OK. So you're left with just-- actually never mind. We'll do another one. So this doesn't do anything. You lost the 20, and you're left with the 24 this time.
So now you delete the 24. So now that you've got rid of the 24, you have a blank box here now. But its sibling is not barely full. It has something to donate. So anyone, which elements are going to rotate?
AUDIENCE: 17 and 16.
PROFESSOR: 16 and 17, right. Cool. So 16 goes up, 17 goes down. And you're done. You're consistent again. So that was deletion. Those are the two cases for deletion. Does that make sense? Anyone? Any questions?
OK. So that's all the topics we were supposed to cover today. Any questions about any of the operations, any of the other topics, lecture, anything?
Notes for Recitation 2 (PDF) are available.
Free Downloads
Video
- iTunes U (MP4 - 70MB)
- Internet Archive (MP4 - 70MB)
Subtitle
- English - US (SRT)