Flash and JavaScript are required for this feature.
Download the video from iTunes U or the Internet Archive.
Description: In this lecture, Professor Devadas continues with randomization, introducing skip lists as a randomized data structure.
Instructors: Srinivas Devadas
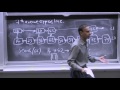
Lecture 7: Randomization: S...
The following content is provided under a Creative Commons license. Your support will help MIT OpenCourseWare continue to offer high quality educational resources for free. To make a donation or view additional materials from hundreds of MIT courses, visit MIT OpenCourseWare at ocw.mit.edu.
SRINIVAS DEVADAS: All right. Good morning, everyone. And let's get started. Today's lecture is about a randomized data structure called the skip list. And it's a data structure that, obviously because it's randomized, we'd have to do a probabilistic analysis for.
And we're going to sort of raise the stakes here a little bit with respect to our expectation-- the pun intended of this data structure-- in the sense that we're not going to be happy with just doing an expected value analysis or to get what the expectation is of the search complexity in a skip list.
We're going to introduce this notion with high probability, which is a stronger notion than just giving you the expected value or the expectation for the complexity of a search algorithm. And we're going to prove that under this notion, that search has a particular complexity with high probability. So we'll get to the with high probability part a little bit later in the lecture, but we're just going to start off doing some cool data structure design, I guess, [INAUDIBLE] pointing to the skip list.
The skip list is a relatively young data structure invented by a guy called Bill Pugh in 1989, so not much older than you guys. It's relatively easy to implement as you'll see. I won't really claim that, but hopefully you'll be convinced by the time you're done describing the structure. Especially in comparison to balanced trees.
And we can do a comparison after we do our analysis of the data structure as to what the complexity comparisons are for search and insert when you take a skip list and compare it to an AVL tree, for example, or a red black tree, et cetera. In general, when we have a data structure, we want it to be dynamic. The skip list maintains a dynamic set.
What that means is not only do you want to search on it-- obviously it's uninteresting to have a static data structure and do a search. You want to be able to change it, want to be able to insert values into it. There's a complexity of insert to worry about. You want to be able to delete values. And the richness of the data structure comes from the operations and the augmentations you can do on it, and the skip lists are no exception to that.
So if you want to maintain a dynamic set of n elements, and you obviously know a ton of data structures to do this, each of which has different characteristics. And this is, if you ignore hash tables, this is your first real randomized data structure, if you're just taking 6006 and this class might have seen randomized structures in other classes.
So we're going to try and do this in order log n time. As you know with balanced binary trees, you can do things in order log n time, a ton of things, pretty much everything that is interesting. And this, given that it's randomized, it's a relatively easy analysis to show that the expectation or the expected value of a search would be order log n expected time.
But we're going to, as I said, raise the stakes, and we're going to spend a ton of time the second half of this showing the with high probability result. And that's a stronger result than just saying that search takes expected order log n time. All right, so that's the context.
You can think of a skip list as beginning with a simple linked list. So if we have one link list and that link list-- let's first think of this as being unsorted. So suppose I have a link list which is unsorted and I want to search for a particular value in this link list. And we can assume that this is a doubly-linked list, so the arrows go both ways. You have a pointer, let's say, just to the first element.
So if you have a list that's unsorted and you want to search for an element, you would want to do a membership query. If there's n elements, the complexity is?
AUDIENCE: Order n.
SRINIVAS DEVADAS: Order n. So a linked list, the search takes the order n time. Now let's go ahead and say that we are sorting this list, so it's a sorted linked list. So your values here, 14, 23, 34, 42, 50, 59. They're sorted in ascending order. You still only have a pointer to the front of the list and it's a doubly-linked list, what is the complexity of search in the sorted link list?
AUDIENCE: Log n.
SRINIVAS DEVADAS: Log n. Oh, I wanted to hear that. Because it is?
AUDIENCE: Order n.
SRINIVAS DEVADAS: It's order n. log n is-- yeah, that was a trick question. Because I liked that answer, the person who said log n gets a Frisbee. This person won't admit.
[LAUGHTER]
Oh, it was you. OK, all right. There you go. All right.
So log n would imply that you have random access. If you have an array that's sorted and you can go [? AFi, ?] and you can go [? AFi ?] divided by 2, or [? AF2i ?] and you can go directly to that element, then you can do binary search and you can get a log n, order log in.
But here, the sorting actually doesn't help you with respect to the search simply because you have to start from the beginning, from the front of the list, and you've got to keep walking. The only place that it helps you is that if you know it's sorted and you're looking for 37, you can stop after you see 42, right?
That's pretty much the only place that it helps you. But it's still order n because that could happen-- on average is going to happen halfway through the list for a given membership query. So it's still order n for a sorted link list.
But now let's say that we had two sorted link lists. And how are these two link lists structured? Well, they're structured in a certain way, and let me draw our canonical example for skip list that I'm going to keep coming back to. So I won't erase this, but I'll draw one out-- 1, 2, 3, 4, 5, 6, 7, 8, 9-- 9 elements.
So that's my first list which is sorted, and so I have 14, 23, 34, 42, 50, 59, 66, 72, and 79. What I'm going to have now is another list sort of on top of this. I can move from top to bottom, et cetera. But I'm not going to have elements on top of each bottom element.
By convention, I'm going to have elements on top of the first element, regardless of how many lists I have. We only have two at this point. And so I see a 14, which is exactly the same element duplicated up on the top list. And that list is also sorted, but I won't have all of the elements in the top list.
I'm just picking a couple here. So I've got 34, 42-- they're adjacent here-- and then I go all the way up to 72, and I duplicate it, et cetera. Now, this looks kind of random. Anybody recognize these numbers? No one from the great City of New York? No? Yup, yup.
AUDIENCE: On the subway stops?
SRINIVAS DEVADAS: Yeah, subway stops on the Seventh Avenue Express Line. So this is exactly the notion of a skip list, the fact that you have-- could you stand up? Great. All right.
So the notion here is that you don't have to make a lot of stops if you know you have to go far. So if you want to go from 14th Street to 72nd Street, you just take the express line. But if you want to go to 66th Street, what would you do?
AUDIENCE: Go to 72nd and then go back.
SRINIVAS DEVADAS: Well, that's one way. That's one way. That's not the way I wanted. The way we're going to do this is we're not going to overshoot. So we want to minimize distance, let's say. So our secondary thing is going to be minimizing distance travel.
And so you're going to pop up the express line, go all the way to 42nd Street, and you're going to say if I go to the next stop on the Express Line, I'm going too far. And so you're going to pop down to the local line. So you can think of this as being link list L0 and link list L1. You're going to pop down, and then you're going to go to 66th Street.
So search 66 will be going from 14 to 42 on L1, and then from 42, let's just say that's walking. 42 to 42, L1 to L0. And then 42 to 66 on L0.
So that's the basic notion of a skip list. So you can see that it's really pretty simple. What we're going to do now is do two things. I want to think about this double-sorted list as a data structure in its own right before I dive into skip lists in general. And I want to analyze at some level, the best case situation for worst case complexity.
And by that I mean I want to structure the express stops in the best manner possible. These stops are very structured for passengers because they figured fancy stops on 42nd Avenue, whatever-- fancy stores. Everybody wants to go there and so on and so forth. So you have 34 pretty close to 42 because they're both popular destinations.
But let's say that things where I guess more egalitarian and randomized, if you will. And what I want to do is I want to structure this double-sorted list so I get the best worst case complexity for search. And so let's do that.
And before I do that, let me write out the search algorithm, which is going to be important. I want you to assimilate this, keep it in your head because we're going to analyze search pretty much for the rest of the morning here. And so I'll write this down. You've got a sense of what it is based on what I just did here with this example of 66, but worth writing down.
We're going to walk right in the top linked list, so this is simply for two linked lists, and we'll generalize at some point. So we want to walk right in the top linked list, L1, until going right would go too far.
Now, there was this answer with 72, which I kind of dismissed. But there's no reason why you can't overshoot one stop and go backwards. It would just be a different search algorithm. It's not something we're going to analyze here. It turns out in analyzing that with high probability would be even more painful than the painful analysis we're going to do. So we won't go there.
And then we walk down to the bottom list. And the bottom list we'll call L0. And walk right in L0 until the element is found or not. And you know that if you've overshot.
So if you're looking here for route 67, when you get to 72 here-- you've seen 66 and you get to 72 and you're looking for 67, search fails. It stops and fails. Doesn't succeed in this case.
So that's what we got for search. And that's our two linked list argument. Now, our analysis essentially says what I have is I'm walking right at the bottom list here, and my top list is L1, so I start with L1. And my search cost is going to be approximately the length of L1.
The worst case analysis, I could go all the way on the top list-- it's possible. But for a given value, I'm going to be looking at only a portion of the bottom list. I'm not going to go all the way on the bottom list ever. I'm only going to be looking at a portion of it.
So it's going to be L0 divided by L1, if I have interspersed my express stops in a uniform way. So there's no reason-- if I have 100 elements in the bottom list, and if I had five, just for argument sake, five in the top list, then I'd put them at, let's say, the 0 position, 20, 40, 60, et cetera. So I want to have roughly equal spacings.
But we need to make that a little more concrete, and a little more precise. And what I'm saying here simply is that this is the cost of traversal in the top list, and this is the cost of traversal in the bottom list, because I'm not going to go all the way in the bottom list. I'm only going to go a portion on the bottom list. Everybody gets that? Yup? All right, good.
So if I want to minimize this cost, which is going to tell me how to scatter these elements in the top list, how to choose my express stops, if you will-- I want to scatter these in a uniform way, then this is minimized when terms are equal. You could go off and differentiate and do that. It's fairly standard.
And what you end up getting is you want to get L1 square equals L0 equals n. So all of the elements are down at the bottom list, and so the cardinality of the bottom list is n. And roughly speaking, you're going to end up optimizing, if you have this satisfied, which means that L1 is going to be square root of n. OK?
So what you've done here is you've said a bunch of things, actually. You've decided how many elements are going to be in your top list. If there's n elements in the bottom list, you want to have the square root of n elements in the top list.
And not only that, in order to make sure that this works properly, and that you don't get a worse case cost that is not optimal, you do have to intersperse the square root of n elements at regular intervals in relation to the bottom list on the top list. OK, so pictorially what this means is it's not what you have here.
What you really want is something that, let's say, looks like this where this part here is square root of n elements up until that point, and then let's say we go from here to here or square root of n elements, and maybe I'll have a 66 here because that's exactly where I want my square root of n. Basically, three elements in between. So I got 66 here, et cetera. I mean I chose n to be a particular value here, but you get the picture.
So the search now, as you can see if you just add those up you get square root of n here, and you got n divided by square root of n here. So that's square root of n as well. So the search cost is order square root of n.
And so that's it. That's the first generalization, and really the most important one, that comes from going from a single sorted list to an approximation of a skip list. So what do you do if you want to make things better? So we want to make things better? Are we happy with square root of n?
AUDIENCE: No.
SRINIVAS DEVADAS: No. Well, what's our target?
AUDIENCE: Log n.
SRINIVAS DEVADAS: Log n, obviously. Well, I guess you can argue that our target may be order 1 at some point, but for today's lecture it is order log n with high probability. We'll leave it at that.
And so what do you do if you want to go this way and generalize? You simply add more lists. I mean it seems to be pretty much the only thing we could do here. So let's go ahead and add a third list.
So if you have two sorted lists, that implies I have 2 square root of n. If I want to be explicit about the constant in terms of the search cost, assuming things are interspersed exactly right. Keep that in mind because that is going to go away when we go and randomize. We're going to be flipping coins and things like that. But so far, things are very structured.
What do you think-- we won't do this analysis-- the cost is going to be if I intersperse optimally, what is the cost going to be for a search when I have three sorted lists?
AUDIENCE: Cube root.
SRINIVAS DEVADAS: Cube root. Great guess. Who said cube root?
AUDIENCE: [INAUDIBLE].
SRINIVAS DEVADAS: You already have a Frisbee. Give it to a friend. I need to get rid of these.
So it's going to be cube root, and the constant in front of that is going to be?
AUDIENCE: 3.
SRINIVAS DEVADAS: 3. So you have-- right? So let's just keep going. You have k sorted lists. You're going to have k times the k-th root of n. That's what you got. And I'm not going to bother drawing this, but essentially what happens is you are making the same number of moves which corresponds to the root of n, the corresponding root of n, at every level.
And the last thing we have to do to get a sense for what happens here is we have log n sorted lists, so the number of levels here is log n. So this is starting to look kind of familiar because it borrows from other data structures. And what this is I'm just going to substitute log n for k, and I got this kind of scary looking-- I was scared the first time I saw this. Oh, this is n.
It's the log n-th root of n, OK? And so it's kind of scary looking. But what is the log n-th root of n-- and we can assume that n is a power of two?
AUDIENCE: 2.
SRINIVAS DEVADAS: 2, exactly. It's not that scary looking, and that's because I'm not a mathematician. That's why I was scared. So 2 log n.
All right. So that's it. So you get a sense of how this works now, right? We haven't talked about randomized structures yet, but I've given you the template that's associated with the skip list, which essentially says what I'm going to have are-- if it was static data items and n was a power of two, then essentially what I'm saying is I'm going to have a bunch of items, n items, at the bottom.
I'm going to have n over 2 items at the list that's just immediately above. And each of them are going to be alternating. You're going to have an item in between. And then on the top I'm going to see n over 4 items, and so on and so forth.
What does that look like? Kind of looks like a tree, right? I mean it doesn't have the structure of a tree in the sense of the edges of a tree. It's quite different because you're connecting things differently. You have all the leaves connected down at the bottom of this so-called tree with this doubly linked list, but it has the triangle structure of a tree. And that's where the log n comes from.
So this is would all be wonderful if this were a static set. And n doesn't have to be a power of 2-- you could pad it, and so on and so forth. But the big thing here is that we haven't quite accomplished what we set out to do, even though we seem to have this log n cost for search. But it's all based on a static set which doesn't change.
And the problem, of course, is that you could have deletions. You want to take away 42. For some reason you can't go to 42nd Avenue, or I guess art-- you can't go to [INAUDIBLE] would be a better example. So stuff breaks, right? And so you take stuff out and you insert things in.
Suppose I wanted to insert 60, 61, 62, 63, and 64 into that list that I have? What would happen? Yeah, you're shaking your head. I mean that log n would go away, so it would be a problem.
But what we have to do now is move to the probabilistic domain. We have to think about what happens when we insert elements. We need an algorithm for insert. So then we can start with the null list and build it up. And then you start with a null list and you have a randomized algorithm for insert, it ain't going to look that pretty. It's going to look random.
But you have to have a certain amount of structure so you can still get your order log n. So you have to do the insertion appropriately. So that's what we have to do next. But any questions about that complexity that I have up there? All right, good.
I want a canonical example of a list here, and I kind of ran out of room over there, so bear with me as I draw you a more sophisticated skip list that has a few more levels. And the reason for this is it's only interesting when you have three or more levels. The search algorithm is kind of the same. You go up top and when you overshoot you pop down one level, and then you do the same thing over and over.
But we are going to have to bound the number of levels in the skip list in a probabilistic way. We have to actually discover the expected number of levels because we're going to be doing inserts in a randomized way. And so it's worthwhile having a picture that's a little more interesting than the picture of the two linked lists that I had up there. So I'm going to leave this on for the rest of the lecture.
So that's our bottom, and that hasn't changed from our previous examples. I'm not going to bother drawing the horizontal connections. When you see things adjacent horizontally at the same level, assume that they're all connected-- all of them.
And so I have four levels here. And you can think of this as being the entire list or part of it. Just to delineate things nicely, we'll assume that 79, which is the last element, is all the way up at the top as well. Sort of the terminus, termini, corresponding to our analogy of subways.
And so that's our top-most level. And then I might have 50 here at this level, or so that looks like. I will have 50, so the invariant here, and that's another reason I want to draw this out, is that if you have a station at highest level, then you will have-- it's got to be sitting on something.
So if you've got a 79 at level four, or level three here if this is L0, then you will see 79 at L2, L1, and L0. And if you see 50 here, it's not in L3 so that's OK, but it's in L2, so it's got to be at L1 as well.
Of course you know that everything is down at L1, so this is interesting from a standpoint of the relationship between Li and Li plus 1 where i is greater than or equal to 1. So the implication is that if you see it at at Li plus 1, it's going to be at Li and Li minus 1 if that happens to exist, et cetera.
And so one last thing here just to finish it up. I got 34 here, which is an additional thing which ends there. So the highest level is this second level or L1. This is 66. And then that's it. So that's our skip list.
So if you wanted to search for 72, you would start here, and then you'd go to 79, or you'd look and say, oh, 79 is too far, so I'm going to pop down a level. And then you'd say 50, oh, good. I can get to 50. 79 is too far, so I'm going to pop down a level. And then you go to 66-- 79 is too far-- and at 66, you pop down a level and then you go 66 to 72.
So same as what we had before. Hopefully it's not too complicated. So that's our skip list. It's still looking pretty structured, looking pretty regular. But if I start taking that and start inserting things and deleting things, it could become quite irregular.
I could take away 23, for example. And there's nothing that's stopping me from taking away 34 or 79. You've got to delete an element, you've got to delete an element. I mean the fact that it's in four levels shouldn't make a difference. And so that's something to keep in mind. So this could get pretty messy.
So let's talk about insert, and I've spent a bunch of time skirting around the issue of what exactly happens when you insert an element. Turns out delete is pretty easy. Insert is more interesting. Let's do insert.
To insert an element x into a skip list, the first thing we're going to do is search to figure out where x fits into the bottom list. So you do a search just like you would if you were just doing a search.
You always insert into the appropriate position. So if there's a single sorted list, that would pretty much be it. And so that part is easy. If you want to insert 67, you do all of the search operations that I just went over, and then you insert 67 between 66 and 72. So do your pointer manipulations, what have you, and you're good.
But you're not done yet, because you want this to be a skip list and you want this to have expected search over any random query as the list grows and shrinks of order log n, expectation, and also with high probability. So what you're going to have to do is when you start inserting, you're going to have to decide if you're going to what is called promote these elements or not.
And the notion of a promotion is that you are going up and duplicating this inserted element some number of levels up. So if you just look at how this works, it's really pretty straightforward. What is going to happen is simply that let's say I have 67 and I'm going to insert it between 66 and 72. That much is a given. That is deterministic.
Then I'm going to flip a coin or spin a Frisbee. I like this better. I'm not sure if this is biased or not. It's probably seriously biased.
[LAUGHTER]
Would it ever go the other way is the question. Would it ever? No. All right. So we've got a problem here. I think we might have to do something like that.
[LAUGHTER]
I'm procrastinating. I don't want to teach the rest of this material.
[LAUGHTER]
All right. Let's go, let's go. So I'd like to insert into some of the lists, and the big question is which ones? It's going to be really cool. I'm just going to flip coins, fair coins, and decide how much to promote these elements.
So flip fair coin. If heads, promote x to the next level up, and repeat. Else, if you ever get a tails, you stop. And this next level up may be newly created.
So what might happen with the 67 is that you stick it in here, and it might happen that the first time you flip you get a tails, in which case, 67 is going to just be at the bottom list. But if you get one heads, then you're not only going to put 67 in here, you're going to put 67 up here as well. And you're going to flip again.
And if you get a heads again, you're going to put 67 up here. And if you get a heads again, you're going to put 67 up here. And if you get a heads again, you're going to create a new list up there, and at this point when you create the new list, it's only going to be 67 up there. And that's going to be the front of your list, because that's the one element that you're duplicating.
So you're going to keep going until you get a tails. Now, that's why this coin had better be fair. So you're going to keep going and you're going to keep adding. Every time you insert there's a potential for increasing the number of levels in this list.
Now, the number of levels is going to be bounded in expectation with a high probability of regular expectation, but I want to make it clear that every time you insert, if you get a chain of heads, you're going to be adding levels. And so the first time you get a tails, you just stop. You just stop.
So you can see that this can get pretty messy pretty quick. And especially if you were starting from ground zero and adding 14, 23-- all of those things, the bottom is going to look exactly like it looks now because you're going to put it in there. It's deterministic. But the very next level after that looked pretty messy. You could have all of them chunked up here, and a big gap, et cetera, et cetera. So it's all about randomized search cost.
The worse case cost here is going to be order n. Worst case cost is going to be order n, because you have no idea where these things are going to end up. But the randomized cost is what's cool about this. Any questions about insert or anything I said? Yeah, go ahead.
AUDIENCE: Is worse case really order n? What if you had a really long, like a lot of lists on top of each other, and you start at the top of that and you had to walk all the way [INAUDIBLE]?
SRINIVAS DEVADAS: Well, you go n down and n this way, right? You would be checking so it would be order n.
AUDIENCE: So it's [? bounded ?] by n?
SRINIVAS DEVADAS: Yeah, the worst case.
AUDIENCE: Worse case is infinity.
SRINIVAS DEVADAS: Worse case is infinity. Oh, in that sense, yeah. OK. Well, n elements, Eric is right. So what is happening here is that you have a small probability that you will keep flipping heads forever. So at some level, if you somehow take that away and use Frisbees instead or you truncate it.
Let's say at some point you ended up saying that you only have n levels total. So it's not a-- I should have gone there. The question has to be posed a little more precisely for the answer to be order n. You have to have some more limitations to avoid the case that Eric just mentioned, which is in the randomized situation you will have the possibility of getting an infinite number of heads. Yeah, question back there.
AUDIENCE: [INAUDIBLE].
SRINIVAS DEVADAS: Yes, you can certainly do capping and you can do a bunch of other things. It ends up becoming something which is not as clean as what you have here. The analysis is messy. And it's sort of in between a randomized data structure, a purely randomized data structure, and a deterministic one.
I think the important thing to bring out here is the worst case is much worse than order log n, OK? Cool. Good. Thanks for those questions.
And so what we have here now is an insert algorithm that could make things look pretty messy. I'm going to leave the insert up here, and that, of course, is part of that.
Now, for the rest of the lecture we're going to talk about why skip lists are good. And we're going to justify this randomized data structure and show lots of nice results with respect to the expectation on the number of levels, expectation on the number of moves in a search, regardless of what items you're inserting and deleting.
One last thing. To delete an item, you just delete it. You find it, search, and delete at all levels. So you can't leave it in any of the levels. So you find it, and you have to have the pointers set up properly-- move the previous pointer over to the next one, et cetera, et cetera. We won't get into that here, but you have to do the delete at every level. Yeah, question.
AUDIENCE: So what happens if you inserted 10s and you flip off a tail? So that's like your first element is not going to go up all the way, and then have you do search.
SRINIVAS DEVADAS: So typically what happens is you need to have a minus infinity here. And that's a good point. It's a corner case. You have to have a minus infinity that goes up all the way. Good question.
So the question was what happens if I had something less than 14 and I inserted it? Well, that doesn't happen because nothing is less than minus infinity, and that goes up all the way. But thanks for bringing it up.
And so we're going to do a little warm-up Lemma. I don't know if you've ever heard these two terms in juxtaposition like this-- warm up and Lemma. But here you go, your first warm-up Lemma.
I guess you'd never have a warm-up theorem. It's a warm-up Lemma for this theorem, which is going to take a while to prove. This comes down to trying to get a sense of how many levels you're going to have from a probabilistic standpoint. The number of levels in an n element skip list is order log n. And I'm going to now define the term with high probability.
So what does this mean exactly? Well, what this means is order log n is something like c log n plus a constant. Let's ignore the constant and let's stick with c log n. And with high probability is a probability that is really a function of n and alpha.
And you have this inverse polynomial relationship in the sense that obviously as n grows here, an alpha-- we'll assume that alpha is greater than the 1-- you are going to get a decrease in this quantity. So this is going to get closer and closer to 1 as n grows. So that's the difference between with high probability and just sort of giving you an expectation number where you have no such guarantees.
What is interesting about this is that as n grows, you're going to get a higher and higher probability. And this constant c is going to be related to alpha. That's the other thing that's interesting about this.
So it's like saying-- and you can kind of say this for using Chernoff bounds that we'll get to in a few minutes, even for expectation as well. But what this says is that if, for example, c doubled, then you are saying that your number of levels is order 4 log n. I mean I understand that that doesn't make too much sense, but it's less than or equal to 4 log n plus a constant.
And that 4 is going to get reflected in the alpha here. When the 4 goes from 4 to 8, the alpha increases. So the more room that you have with respect to this constant, the higher the probability. It becomes an overwhelming probability that you're going to be within those number of levels.
So maybe there's an 80% probability that you're within 2 log n. But there's a 99.99999% probability that you're within 4 log n, and so on and so forth. So that's the kind of thing that with the high probability analysis tells you explicitly.
And so you can do that, you can do this analysis fairly straightforwardly. And let me do that on a different board. Let me go ahead and do that over here. Actually, I don't really need this. So let's do that over here.
And so this is our first with high probability analysis. And I want to prove that warm-up Lemma. So usually what you do here is you look at the failure probability. So with high probability is typically something that looks like 1 minus 1 divided by n raised to alpha. And this part here is the failure probability. And that's typically what you analyze and what we're going to do today.
So the failure probability is that it's not less than c log n levels, is the complement of what we just looked at, which is the probability that it's strictly greater than c log n levels. And that's the probability that some element gets promoted greater than c log n times.
So why would you have more than c log n levels? It's essentially because you inserted something and that element got promoted strictly greater than c log n times, which obviously implies that you had the sequence of heads, and we'll get to that in just a second.
But before we go to that step of figuring out exactly what's going on here as to why this got promoted and what the probability of each promotion is, what I have here is I have a sequence of inserts potentially that I have to analyze. And in general, when I have an n element list, I'm going to assume that each of these elements got inserted into the list at some point. So I've had n inserts. And we just look at the case where you have n inserts, you could have deletes, and so you could have more inserts, but it won't really change anything.
You have n inserts corresponding to each of these elements, and one of those n elements got promoted in this failure case greater than c log n times. That's essentially what's happened here.
And so you don't know which one, but you can typically do this in with high probability analysis because the probabilities are so small and they're inverse polynomials, polynomials like n raised to alpha. You can use what's called the union bound that I'm sure you've used before in some context or the other. And you essentially say that this is less than or equal to the probability that a particular element x.
So you just pick an element, arbitrary element x, but you pick one. Gets promoted greater than c log n times. So you have a small probability. You have no idea whether these events are independent or not.
The union bound doesn't care about it. It's like saying you've got a 0.001 probability that any of these elements could get promoted greater than c log n times, and there's 10 of those elements. You don't know whether they're independent events or not, but you can certainly use the union bound that says the overall failure probability is going to be less than or equal to n equals 10, in my example, times that 0.001. That's basically it.
Now you can go off and say, what does it mean for an element to get promoted? What actually has to happen for an element to get promoted? And you have n times 1 over 2, because you're flipping a fair coin, and you are getting a c log n heads here. You flip and you get one promotion. There's two levels associated with a promotion, the level you came from and the level you went to.
And so a promotion is a move, so you're going to have one more level. If you count levels, then you have the number of promotions, right? That's just simply corresponds to taking this 1/2 and raising it to c log n, because that's essentially the number of promotions you have.
And you got n 1/2 c log n, and what does that turn into? What is n times 1/2 c log n? 1 over 2 raised to log n would give you? 2 raised to log ns? Is n, right?
So you got n divided by n raised to c, which is 1 divided by n raised to c minus 1, which is 1 divided by n raised to alpha where alpha is c minus 1. So that's it. That's our first with high probability analysis. Not too hard.
What I've done is done exactly what I just told you that the notion of with high probability is. You have a failure probability that is related. Inverse polynomial and the degree of the polynomial alpha is related to c. And so that's what I have out there, but c equals-- what did it have? Alpha equals c minus 1 or c equals alpha plus 1.
So what I've done here is done an analysis that tells you with high probability how many levels I'm going to have given my insert algorithm. So this is the first part of what we'd like to show. This just tells us how big this skip list is going to grow vertically.
It doesn't tell us anything about the structure of the list internally as to whether the randomization is going to cause that pretty structure that you see up here to be completely messed up to the point where we don't get order log n search complexity, because we are spending way too much time let's say on the bottom list or the list just above the bottom list, et cetera.
So we need to get a sense of how the structure corresponding to the skip list, whether it's going to look somewhat uniform or not. We have to categorize that, and the only way we're going to characterize that is by analyzing search and counting the number of moves that a search makes.
And the reason it's more complicated than what you see up there is that in a search, as you can see, you're going to be moving at different levels. You're going to be moving at the top level. Maybe at relatively small number of moves, you're going to pop down one, move a few moves at that level, pop down, et cetera, et cetera.
So there's a lot of things going on in search which happen at different levels, and the total cost is going to have to be all of the moves. So we're going to think about all of the moves-- up moves, down moves, and add them all up. They all have to be order log n with high probability. There's no getting around that because each of them costs you.
So that's the thing that we'll spend the next 20 minutes on. And the theorem that we like to prove for search is that-- this is what I just said-- any search in an n element skip list costs order log n w.h.p.
So it doesn't matter how this skip list looks. There's n elements, they got inserted using the insert algorithm-- that's important to know if you're going to have to use that. And when I do a search for an element, it may be in there, it may not be in there. Doesn't really matter. We'll assume a successful search. That is going to cost me order log n with high probability.
And the cool idea here in terms of analyzing the search in order to figure out how we're going to add up all of these moves is we're going to analyze the search backwards. So that's a cool idea. So what does that mean exactly?
Well, what that means is that we're going to think about this b search, which think of it as the backward search, starts-- it actually ends, so that's what I'm writing in brackets here, at the node in the bottom list. So we're assuming a successful search, as I mentioned before. Otherwise, the point would just be in between two members.
You know that it's not in there because you're looking for 67 and you see 66 to your left and 72 to your right. So either way it works, but keep in mind that it's a successful search because it just makes things a little bit easier.
Now, at each node that we visit, what we're going to do is we're going to say that if the node was not promoted higher, then what actually happened here was that when you inserted that particular element, you got a tails. Because otherwise you would have gotten a heads, that element would have been promoted higher.
Then you go-- and that really means that you came from the left-hand side, so you make a left move. Now, search of course makes down moves and right moves, but this is a backward search so it's going to make left moves and up moves.
What else do I have here? Running out of room, so let me-- let's continue with that.
All right. And now the case is if the node was promoted higher, that means we got heads here in that particular insertion. Then we go, and that means that during the search we came from upstairs.
And then lastly, we stop, which means we start when we reach the top level or minus infinity if we go all the way back. So that's it. A lot of writing here, but this should make things clear.
So let's say that we're searching for 66. I want to trace through what the backwards path would look like, and keep that code in mind as I do this. So I'm searching for 66, and obviously, we know how to find it. We've done that. But let's go backwards as to what exactly happened when we look for 66.
When we look for 66, right at this point when you see 66, where would you have come from?
AUDIENCE: [INAUDIBLE]
SRINIVAS DEVADAS: You'd have come from the top. And so if you go look at what happens here, the node when it got inserted was promoted one level. So that means that you would go up top in the backward search first. Your first move would be going up like that.
Now, if there's a 66 up there, you would go up one more. But there's not, so you go left. You go to 50. And when you have a 50 up here, would you stay on this level?
AUDIENCE: No.
SRINIVAS DEVADAS: No. You'd go up to 50 because the first chance you get you want to get up to the higher levels. And again, this 50 was promoted so you go up there, and you go to 14, and pretty much that's the end of that.
So this would look like you go like that, you have an up move, then you have a left move-- different colors here would be good-- then you have an up move, and a left, and then an up. So that's our backward search. And it's not that complicated, hopefully. If you're looking for 66 or 59, you do that. So it's much more natural, and you just need to flip it.
Why am I doing all this? Well, the reason I'm doing all this is that I have to do some bounding of the moves, and I know that the moves that correspond to the up moves are probabilistic in the sense that the reason I'm making them is because I flipped heads at some point. So all of this is going to turn into counting how many coin flips come out heads in a long stream of coin flips. So that's what this backward search is going to allow us to do.
And that crucial thing is what we'll look at next. So the analysis itself is a bit painful, but there's a bunch of algebra. But what I want to do is to make sure that you get the high level picture, number one, and the insights as to why the expected value or the with high probability value is going to be order log n. But the key is the strategy.
So we're going to go off and we're going to prove this theorem. Our backward search makes up moves and left moves. We know that. Each with probability 1/2. And the reason for that is when you go up is because you got a heads, and if you didn't get a heads in you got a tails, that meant you go left. Because of the previous element, every time you're passing these elements that are inserted, and they were inserted by flipping coins.
So that's key point number one. All of that, if you look at what happens here when I drew this out, you got heads here and you got tails there. So each of those things for a fair coin is happening with probability 1/2. And it's all about coin flips here.
Now, the number of moves going up is less than the number of levels-- the number of levels is one more than that. And we've shown that that's c log n with high probability by the warm-up Lemma. That's what this just did.
The number of up moves-- I mean you can't go off the list here. This list is now you're not inserting anymore, you're doing a search. So it's not like you're going to be adding levels or anything like that. So the number of up moves we've taken care of.
So this last thing here which I'm going to write out here is the key observation, which is going to make the whole analysis possible. And so this last thing it says that the total number of moves-- so now the total number of moves has to include, obviously, the up moves and the left moves, and there's no other kind.
The total number of moves is going to correspond to the number of moves till you get c log n up moves. So what does that mean? There's some sequence of heads and tails that I'm getting, each of them with probability 1/2. Every time that I got a heads, I moved up a level.
The fact of the matter is that I can't get more than c log n heads because I'm going to run out of levels. That's it. I'm going to run out of room vertically if I keep popping up and keep doing up moves. So at that point I'm forced to go left. Maybe I'm going left in the middle there when I still had a chance to go up. That corresponds to getting a tails as opposed to a heads.
But I can limit the total number of moves from a probabilistic standpoint by saying during that sequence of coin flips I only have a certain number of heads that I could have possibly gotten. Because if I got more heads than that, I would be up top. I'd be out of the skip list, and that doesn't work.
So the total number of moves is the number of moves till you get c log n up moves, which essentially corresponds to-- now, forget about skip lists for a second. Our claim is the total number of moves is the number of coin flips, so these are the same, because every move corresponds to a coin flip. Until-- it's a fair coin, probability 1/2-- until c log n heads have been obtained.
So the number of coin flips until c log n heads is the total number of moves. This equals that. And what we now want to show, if you believe that, and hopefully you do because the argument is simply that you run out of levels, that this is order log n w.h.p. That's why it's a claim.
So the observation is that the number of coin flips, as you flip a fair coin, until you get c log n heads will give you the number of moves in your search, total number of moves in your search. It includes the up moves as well as the left moves. And now what we have to show is that that is going to be order log n with high probability. OK?
And then once you do that you've done two things. You've bounded the number of levels in the skip list to be order log n with high probability. And you've said the number of moves in the search is order log n with high probability assuming that the number of levels is c log n, obviously.
So it's not that the bottom one subsumes the top one. It's the last thing to keep in mind as we get all of these items out of the way. This assumes that there are less than or equal to c log n levels. That's the only reason why I could make an argument that I've run out of levels.
So if I have this event A here-- if I call this event A, and I have this event B, what I really want is-- I've shown you that event A happens with high probability. That's the warm-up Lemma. I need to show you that event B happens with high probability. And then I have to show you that event A and event B happen with high probability, because I need both.
Any questions? We're stopping a minute here. The rest of the analysis, a bunch of algebra, we'll get through it, you can look at the notes. This is the key point. If you got this, you got it. Yeah.
AUDIENCE: Can you just say that because the probability of drawing an up move instead of a left move is 1/2, that the expected number of left moves should be equal to the number of up moves, [INAUDIBLE] bound the up moves?
SRINIVAS DEVADAS: So the argument is that since you have 1/2, can you simply say that the expected number of left moves is going to be the same as the same as the up moves?
You can make arguments about expectation. You can say that at any level, the number of left moves that you're going to have is going to be two in expectation. It's not going to give you your with high probability proof. It's not going to relate that to the 1 divided by n raised to alpha.
But I will tell you that if you just wanted to show expectation for search is order log n, you won't have to jump through all of these hoops. At some level you'll be making the assumptions that I've made explicit here through my observations when you do that expectation. So if you really want to write a precise proof of expected value for search complexity, you would have to do a lot of the things that I'm doing here.
I'm not saying you waved your hands. You did not. But it needed more to than what you just said. OK?
So this is pretty much what the analysis is. With high probability analysis we bounded the vertical, we bounded the number of moves. Assuming the vertical was bounded, we got the result for the number of moves. So both of those happen with high probability. You got your result, which is the theorem that we have somewhere. Woah, did I erase the theorem?
AUDIENCE: [INAUDIBLE].
SRINIVAS DEVADAS: It's somewhere. All right. Good.
So let's do what we can with respect to showing this theorem. There's a couple ways that you could prove this. There's a way that you could use a Chernoff bound. And this is kind of a cool result that I think is worth knowing.
I don't know if you've seen this, but this is a seminal theorem by Chernoff that says if you have a random variable representing the total number of tails, let's say-- it could be heads as well-- in a series of m-- not n, m-- independent coin flips where each flip has a probability p of coming up heads, then for all r greater than 0, we have this beautiful result that says the probability that y, which is a random variable-- a particular instance when you evaluate it-- that it is larger than the expectation by r is bounded.
So just a beautiful result that says here's a random variable that corresponds to flipping a coin. I'm going to flip this a bunch of times, and I know what the expectation is. If it's a fair coin of 1/2, then I'm going to get m over 2-- expected number of heads is going to be m over 2. Expected number of tails is going to be m over 2. If it's p, then obviously it's a little bit different-- p times m.
But what I have here is if you tell me what the probability is that I'm 10 away from the expectation and that would imply that r is 10, then that is bounded by e raised to minus 2 times 10 square divided by m. So that's Chernoff's bound.
And you can see how this relates to our with high probability analysis. Because our with high probability analysis is exactly this. This is the hammer that you can use to do with high probability analysis. Because this tells you as you get further and further away from the average or you get further and further away from the expectation, what the probability is that you're going to be so far away.
What is the probability that in 100 coin flips that are fair, you get 50 heads? It's a reasonably large number because the expected value corresponds to 50. So r is 0. So that just says this is a-- well, it doesn't tell you much because this says it's less than or equal to 1. That's all it's says.
But if you had 75, what are the probability that you get 75 heads when you flip a coin 100 times? Then e of y for a fair coin would be 50, r would be 25, and you'd go off and you could do the math for that.
So it's a beautiful relationship that tells you how the probabilities change as your random variable value is further and further away from the expectation. And you can imagine that this is going to be very useful in showing our with high probability result. And I think what I have time for is just to give you a sense of how this result works out-- I'm not going to do the algebra. I don't think it's worth it to write all of this on the board when you can read it in the notes.
But the bottom line is we're going to show this little Lemma that says for any c, invoking this Chernoff bound, there's a constant d, such that with high probability, the number of heads in flipping d log n. So I have a new constant here. d log n fair coins, or a single fair coin, d log n times, assuming independence, is at least c log n.
So what does this say? A lot of words. It just says, hey, you want an order log n bound here eventually. The beauty of order log n is that there's a constant in there that you control. That constant is d. So you tell me that c log n is 50. So c log n is 50.
Then what I'm going to do is I'm going to say something like, well, if I flip a coin 1,000 times, then I'm going to have an overwhelming probability that I'm going to get 50 heads. And that's it. That's what the Lemma says.
It says tell me what c log n is. Give me that value. And I will find you a d, such that by invoking Chernoff, I'm going to show you an overwhelming probability that for that d you're going to get at least c log n heads. So everybody buy that? Make sense from what you see up there? Yup?
So this essentially can be shown-- it turns out that what you have to do is-- and you don't have to choose 8, but you can choose d equals 8c. Just choose d equals 8c and you'll see the algebra in the notes corresponding to what each of these values are.
So e of y, just to tell you, would be m over 2. You're flipping m coins, fair coin with probability 1/2. So you got m over 2. And then the last thing that I'll tell you is that what you want in terms of invoking that, you want r-- remember we were talking about tails here-- so r is going to be d log n minus c log n.
So you just invoke Chernoff with e of y equals m over 2. And what you're saying here is you want c log n heads. You want to make sure you get c log n heads, which means that the number of tails is going to be d log n minus c log n.
And typically we analyze failure probability, so what this is is this is going to be a tiny number. So the failure is when you get fewer than c log n heads. So the failure is when you get fewer than c log n heads. And so that means that you're getting more than d log n minus c log n tails as you're flipping this coin. Fewer than c log n heads means you're getting at least d log n minus c log n tails. So that's why this is your r here.
And then when your r gets that large, and you can play around with the d and the c and choose d equals 8c, you realize that this is going to be a minuscule probability. And you can turn that around to a polynomial-- again, a little bit of algebra. But you can show this result on here that says that the number of coin flips until c log n heads is order log n with high probability by appropriately choosing the constant d to be some time/number over c.
So I'll let you do that algebra. But this one last thing that-- we're not quite done. So you thought we were done, but we're not quite done. And why is it that we're not quite done? Real quick question worth five Frisbees. Why is it that we're not quite done? What did I say? I have done event A and event B, right?
AUDIENCE: [INAUDIBLE].
SRINIVAS DEVADAS: I haven't done the last thing which is to show that probability of event A-- this is with high probability happens-- and I need to show that probability of event A and event B happens-- or this is with high probability. Or I should just say event A and event B happen with high probability.
And you can see that. It turns out it's pretty straightforward, but you got the gist of it. Thanks for being so patient. And there you go guys. Woah.
Notes for Lecture 7 (PDF) are available.
Free Downloads
Video
- iTunes U (MP4 - 182MB)
- Internet Archive (MP4 - 182MB)
Subtitle
- English - US (SRT)